1. Identifying Novel Therapeutic Targets
AI algorithms now integrate multi-omics (genomic, proteomic, etc.) to uncover cancer-specific drug targets. By mining large datasets, AI can flag subtle genomic alterations and protein structures that indicate actionable targets. These methods drastically cut the discovery timeline: for example, a recent AI workflow used protein folding predictions to generate a new drug candidate in ~30 days. AI can also suggest novel uses for existing molecules by linking molecular networks and pathways. Overall, AI-driven analyses help prioritize promising targets from vast molecular data, accelerating the path from biological insights to new therapies.
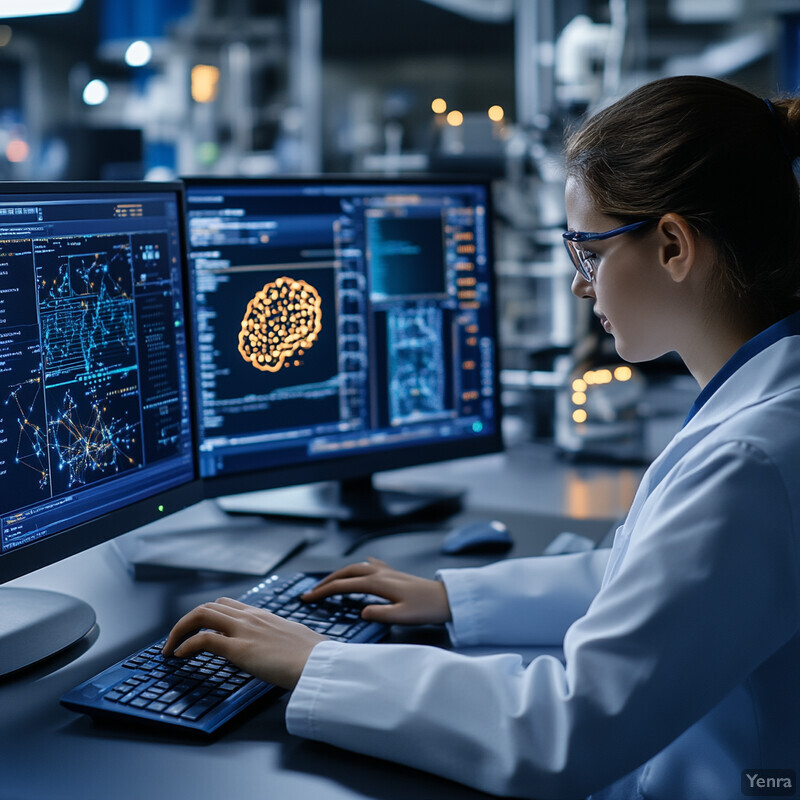
O’Connor and McVeigh (2025) demonstrated that an AI platform (AlphaFold-based) predicted the structure of a novel liver cancer protein (CDK20) and enabled a targeted drug to be designed in ~30 days, vastly faster than traditional methods. In the same study, a deep learning tool (CDRscan) analyzed genomics and chemoinformatics data and identified 14 oncology and 23 non-oncology approved drugs with previously unrecognized anti-cancer potential. In a broader review, Fountzilas et al. (2025) noted that AI analysis of large-scale genomic and epigenomic datasets can reveal new epigenetic drug candidates and pinpoint compounds targeting specific molecular modifications. Together, these findings illustrate concrete cases where AI has pinpointed actionable targets or drug candidates by sifting through complex biological data.
2. Predicting Treatment Response
AI models are increasingly accurate at forecasting how individual tumors will respond to treatments. By combining genomic profiles, clinical data, and even lab values, ML can identify which patients are likely to benefit from a given therapy. For example, models have been developed to predict response to chemotherapy and immunotherapy before treatment begins. These AI-based predictions outperform many standard methods, helping to avoid ineffective therapies and personalize treatment plans. In practice, AI tools have matched or exceeded physician judgment in predicting survival or drug response in multiple cancers. As a result, such models can guide oncologists to choose therapies with the highest chance of success for each patient.
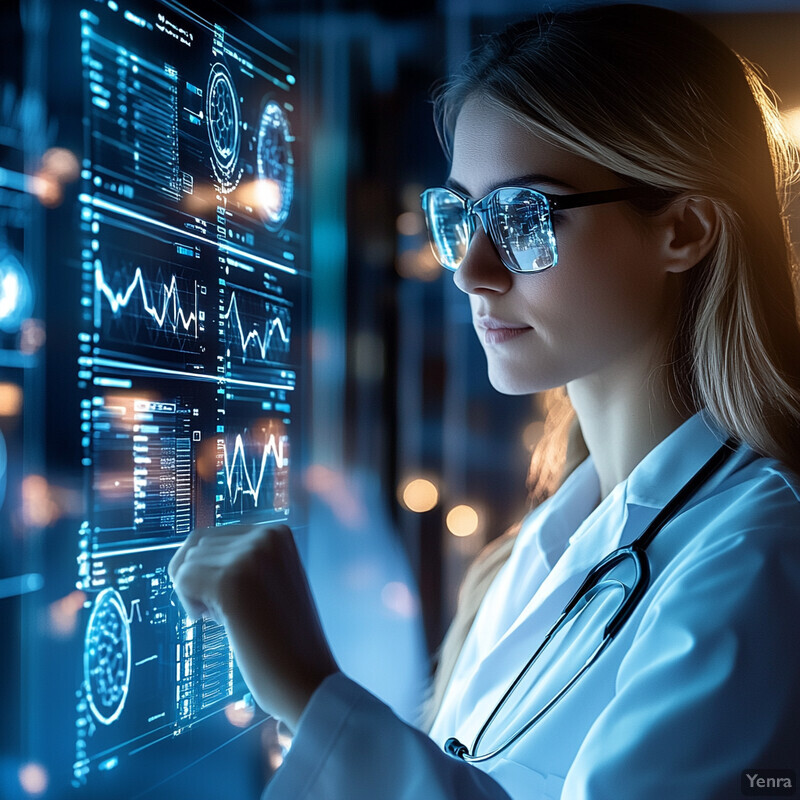
In muscle-invasive bladder cancer, a machine-learning model accurately predicted which patients would respond to neoadjuvant chemotherapy translational-medicine.biomedcentral.com . In ovarian cancer, the same study reported an AI model achieving over 80% accuracy in forecasting platinum-based chemotherapy effectiveness translational-medicine.biomedcentral.com . For immunotherapy, Chowell et al. (2025) trained an AI using routine blood tests (the SCORPIO model) and reported pan-cancer AUC values of 0.714 and 0.641 for predicting immune checkpoint inhibitor (ICI) benefit, substantially higher than tumor mutational burden (TMB) biomarkers (AUC ~0.55). These results, validated on thousands of patients across clinical trials, show AI’s advantage in predicting treatment efficacy from readily available clinical data.
3. Drug Repositioning
AI-driven analysis can rapidly repurpose approved drugs or experimental compounds for new anti-cancer uses. By mining drug-target databases, chemical libraries, and biomedical literature, machine learning can match existing compounds to novel cancer vulnerabilities. This reuse of safe, approved drugs potentially shortens development time and cost. For instance, AI models can suggest that a drug originally for one disease might inhibit a cancer-specific pathway. These insights have already led to the identification of several candidate repurposed therapies for cancer. Overall, AI-facilitated repurposing leverages existing molecules to expand treatment options without starting from scratch.
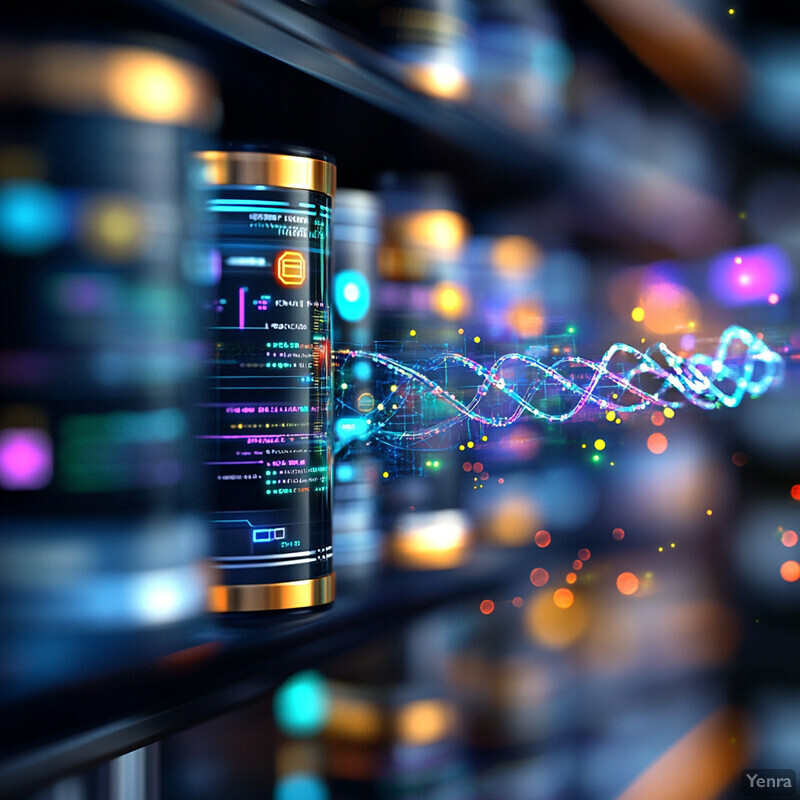
O’Connor & McVeigh (2025) highlighted an AI model (CDRscan) that screened existing drugs and predicted 37 candidates with potential anti-cancer effects – including 14 drugs already used in oncology and 23 from other fields. Such a deep-learning approach effectively prioritized known molecules for new cancer indications. Other AI frameworks, including graph neural networks and similarity-based methods, have proposed repurposing candidates by matching drug-target networks to cancer cell vulnerabilities (reviewed in Qiu et al., 2024). These cases demonstrate how AI systematically uncovers off-label uses of existing drugs, creating a pool of promising compounds for further clinical testing.
4. Optimizing Combination Therapies
AI approaches help identify effective drug combinations by predicting synergistic interactions and avoiding antagonism. Machine learning models can evaluate thousands of possible drug pairs to find those that work better together. These methods use genomic profiles, pathway models, and prior combination data to propose new regimens. In practice, AI has suggested novel combinations that were validated experimentally. By simulating therapy outcomes in silico, AI can recommend potent multi-drug strategies tailored to specific tumors. This streamlines the design of combination treatments, which is otherwise time-consuming and trial-and-error.
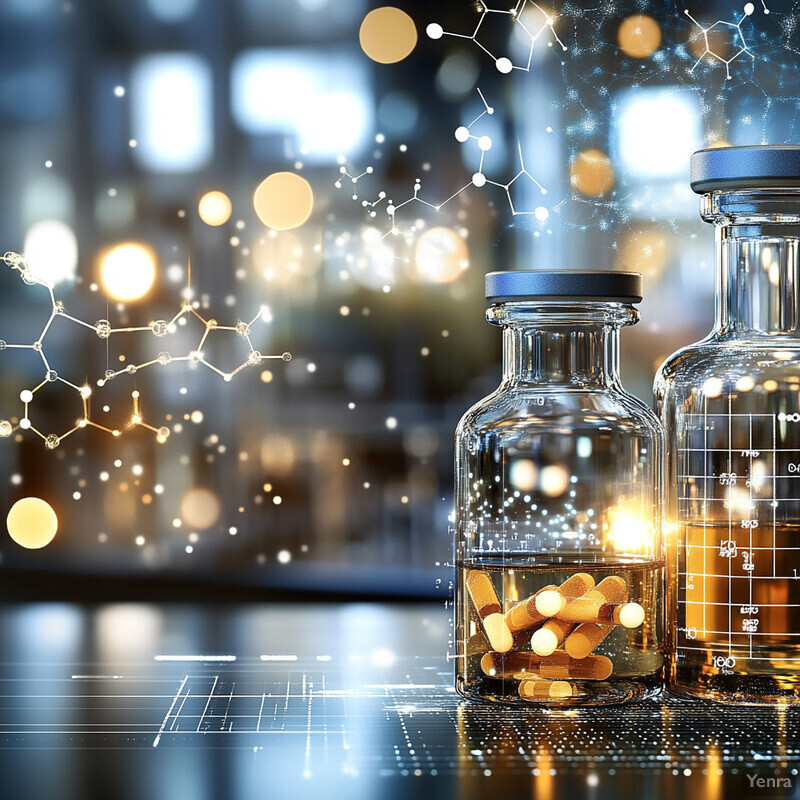
Yin et al. (2024) developed a graph neural network to predict the synergy of FDA-approved drug combinations and reported a classification AUC of ~0.91 for correctly identifying synergistic versus antagonistic pairs. In another study (Wang et al., 2025), the AI tool iDOMO used omics data from triple-negative breast cancer to propose drug pairs; it predicted trifluridine (a chemotherapy) combined with monobenzone (a pigment inhibitor) as highly synergistic, which was subsequently confirmed by in vitro experiments. These examples illustrate that AI models can efficiently prioritize effective drug pairs, achieving high predictive accuracy and suggesting combinations validated in laboratory studies.
5. Radiomics and Imaging Biomarkers
Radiomics uses AI to convert medical images (CT, MRI, PET) into quantitative biomarkers for cancer. AI algorithms extract complex texture, shape, and intensity features that correlate with tumor biology. These image-derived signatures can predict gene mutations, protein expression, or therapy response noninvasively. For example, radiomic models have been built to estimate PD-L1 levels or tumor mutational burden from routine scans. Integrating such imaging biomarkers with clinical data enables better patient stratification without additional invasive tests. As a result, radiomics with AI can expand precision oncology by using widely available scans to provide molecular-level insights.
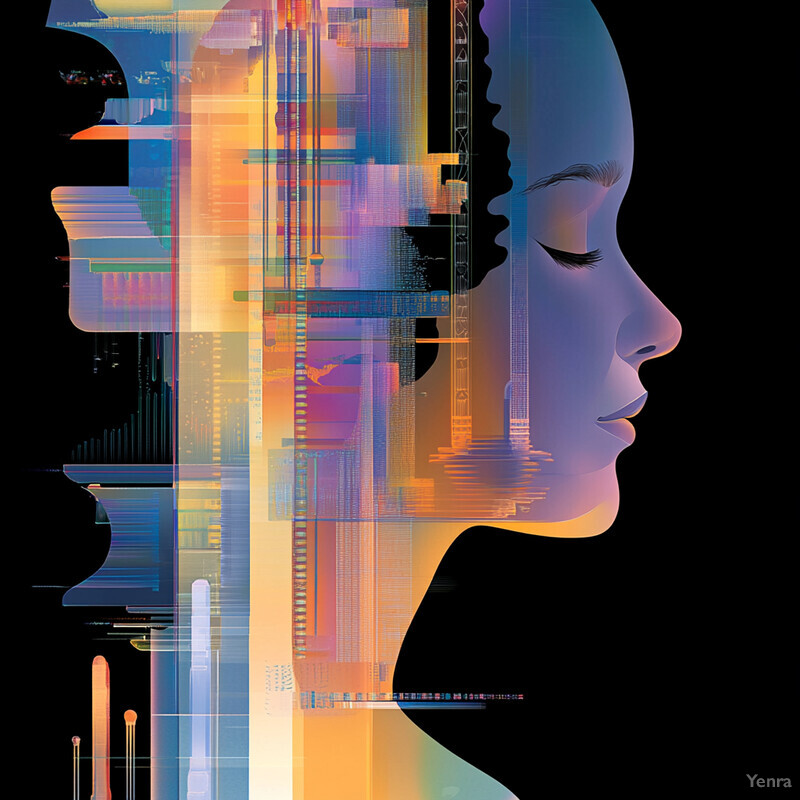
A meta-analysis by Ligero et al. (2024) found that CT-based radiomic models could predict PD-L1 expression in non-small cell lung cancer, achieving a pooled AUC of about 0.86. This indicates strong performance for noninvasive prediction of this key immunotherapy biomarker. Other studies have similarly reported that AI-derived imaging features correlate with tumor mutation burden, microsatellite status, and immune infiltration metrics (reviewed in [107]). These results show that AI-identified radiomic signatures can serve as effective imaging biomarkers, often reaching high accuracy (e.g. AUC >0.8) in predicting molecular tumor properties from standard clinical images.
6. Prognostic and Predictive Modeling
AI models integrate multimodal data (imaging, genomics, pathology, and clinical features) to forecast patient outcomes. These tools provide individualized prognoses (survival, recurrence risk) that often outperform traditional staging or biomarkers. For instance, deep learning algorithms applied to tumor images and patient data can predict overall survival or disease-free survival. In some cases, AI models have correctly stratified patients as effectively as or better than expert clinicians. By offering precise risk assessments and predictive scores, these models guide treatment intensity and follow-up strategies. Overall, AI-driven prognostic models help clinicians personalize care by highlighting which patients may need more aggressive treatment or surveillance.
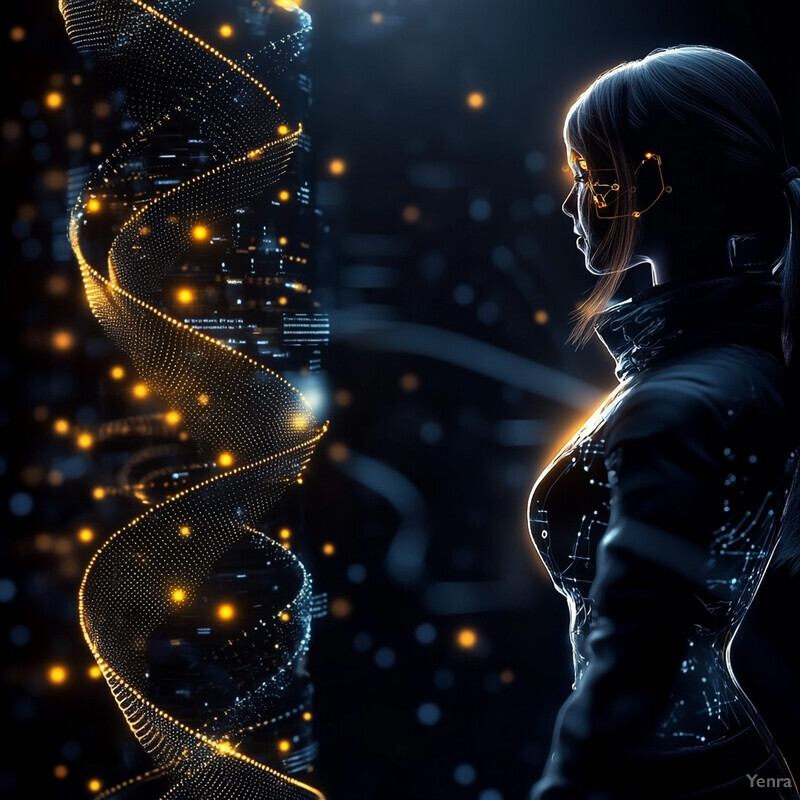
A multimodal AI model (MUSK) from Stanford used clinical and molecular data to predict cancer-specific survival. It correctly predicted outcomes 75% of the time across all cancer types, compared to 64% accuracy for standard clinical prediction. This shows a marked improvement in prognosis accuracy. Similarly, other ML models have integrated patient records and pathology features to achieve high C-indices (e.g. ~0.70–0.85) in predicting survival and recurrence in cancers like lung and gastrointestinal malignancies. Such quantitative gains demonstrate AI’s utility in refining prognostic assessments beyond conventional methods.
7. Real-Time Treatment Adaptation
AI can support dynamic treatment adjustments in “real time” based on patient responses. For example, adaptive radiotherapy uses AI to continuously update radiation plans as tumors shrink or move, ensuring precision targeting during each session. In drug therapy, reinforcement-learning models have been explored to adjust dosing schedules based on biomarker feedback. These systems aim to optimize therapy on the fly – escalating dose if tumor markers rise or de-escalating if toxicity appears. Such AI-guided adaptation enables more personalized treatment, potentially improving efficacy and reducing side effects by tailoring interventions to how the patient’s disease actually evolves.
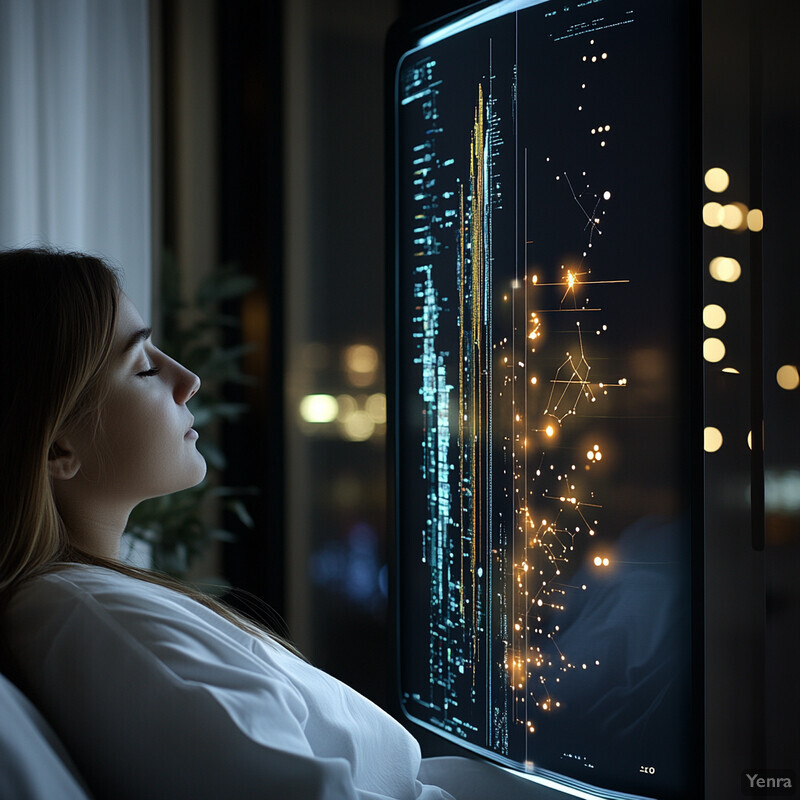
In one study, a deep reinforcement learning (DRL) controller was trained in simulation to manage chemotherapy dosing. Compared to fixed schedules, the DRL model significantly reduced total treatment time and cumulative drug dose while achieving faster tumor cell kill. This suggests AI can adapt drug administration to patient-specific tumor dynamics. Similar approaches in radiotherapy have been shown in preliminary studies to adjust plans based on daily imaging, although large clinical results are still emerging. These examples illustrate AI’s potential to improve treatment efficiency by continuously learning and adapting to patient data.
8. High-Throughput Genomic Data Analysis
AI excels at analyzing vast genomic datasets to identify disease patterns and markers. Techniques like deep learning and ensemble models handle high-dimensional data (e.g. whole-transcriptome or single-cell profiles) to discover signatures of response or resistance. AI-driven analysis can reveal novel gene combinations predictive of phenotype that classical methods miss. For instance, ML models built on single-cell RNA-seq have been used to predict which tumors will respond to specific therapies. By integrating genomics with other data types (proteomics, metabolomics), AI provides a comprehensive view of tumor biology at high throughput. This enables faster biomarker discovery and insights from large-scale studies in cancer genomics.
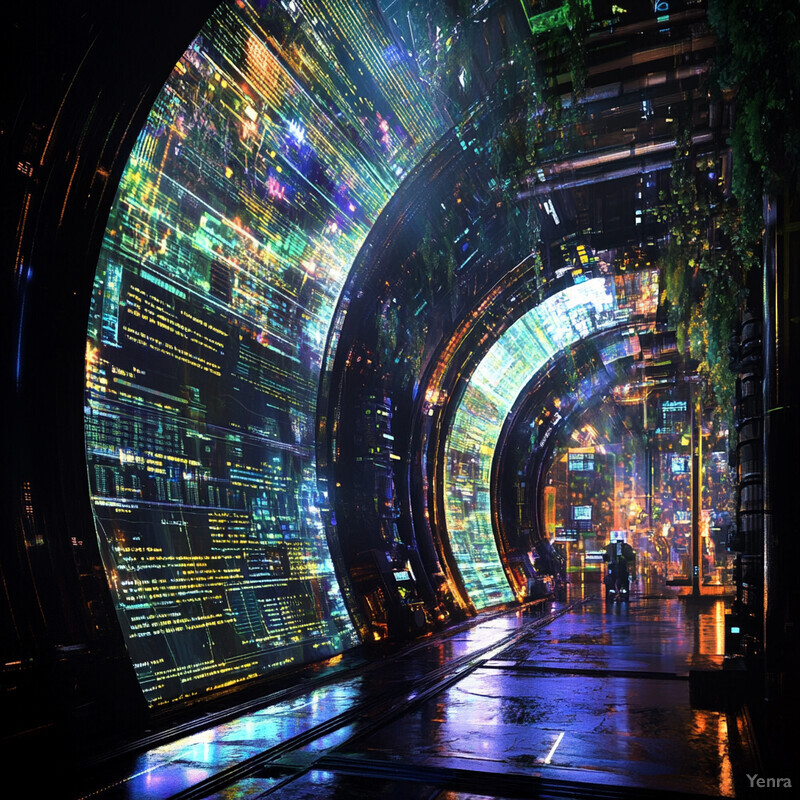
Sun et al. (2025) developed an AI framework (PRECISE) analyzing ~16,000 single-cell profiles from patients. Using XGBoost on scRNA data, the model predicted immunotherapy response with an AUC of 0.84. This indicates strong performance in predicting ICI benefit from raw single-cell data. Other high-throughput applications include unsupervised clustering of large cell atlases to identify rare resistant clones. These cases demonstrate that AI-based analysis of large genomic datasets can yield accurate predictive signatures and uncover subtle biological structures in the data.
9. Automated Clinical Trial Matching
AI-driven tools now scan electronic medical records (EMRs) and trial databases to match patients to appropriate clinical trials. Natural language processing and structured data extraction automate the review of complex eligibility criteria. These systems significantly speed up the matching process without losing accuracy. In practice, they can screen many more patients in a fraction of the time it takes a human reviewer, increasing enrollment rates and reducing bias. By broadening trial access, AI matching helps patients find targeted therapy trials that they otherwise might miss, improving the feasibility of precision trials.
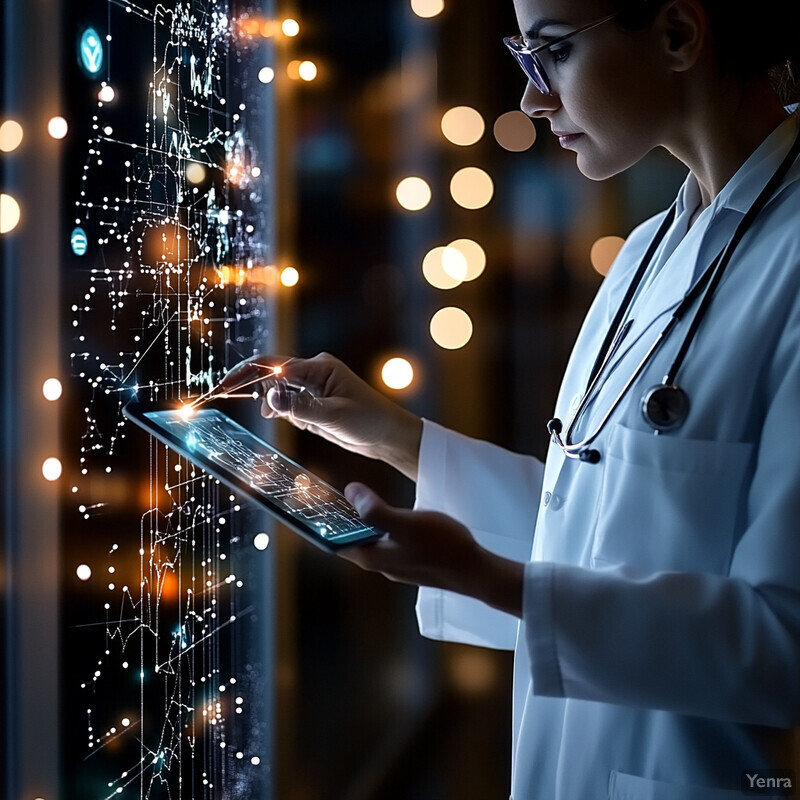
ConcertAI reported that an AI-enabled EMR screening tool identified trial-eligible patients 3× faster than manual review at ASCO 2024. In a real-world study, human reviewers screened 58 patients in 40 hours (41.4 min/patient), whereas the AI method screened 120 patients in 25 hours (12.5 min/patient), with no loss of accuracy or bias. This large time saving illustrates how AI automates the matching of patients to trial criteria, enabling clinical research teams to evaluate far more candidates quickly.
10. Intelligent Electronic Health Records (EHR) Utilization
AI-powered analytics are increasingly applied to EHR data to extract insights and improve decision-making. NLP algorithms can transform free-text notes into structured information (e.g. diagnoses, lab results), enabling large-scale outcome research. AI can also continuously mine EHR trends to flag adverse events or clinical deterioration. Moreover, federated learning allows models to train on EHR data across centers without sharing raw records, enhancing generalizability. These intelligent EHR tools help leverage real-world data in oncology, guiding patient selection, monitoring safety signals, and supplementing clinical trials with population-level evidence.
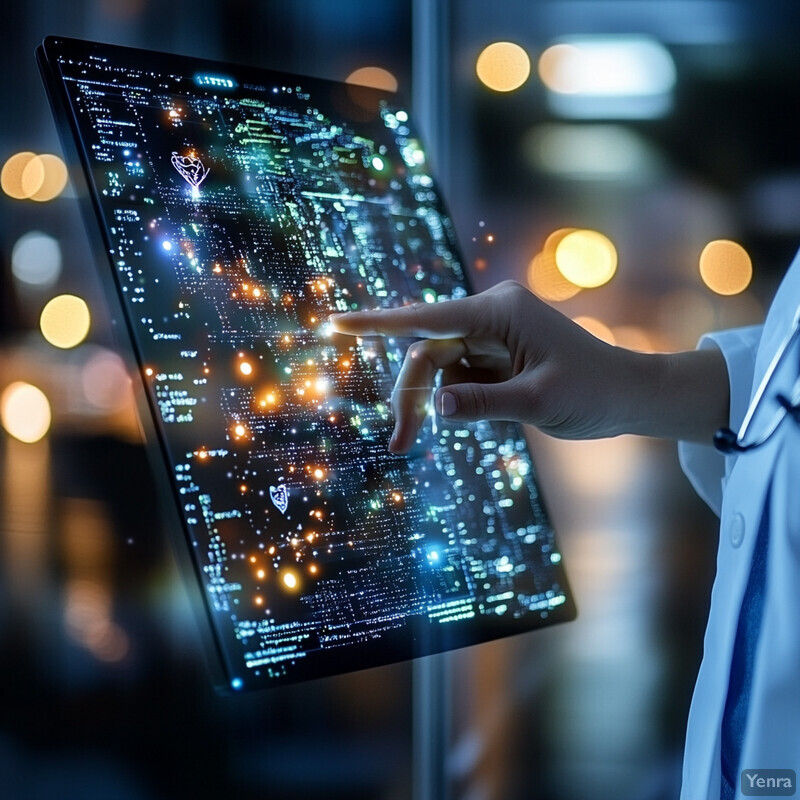
Kehl et al. (2024) developed a shareable machine learning model to extract cancer outcomes (like progression) from radiology reports across institutions. Their “DFCI-prognosis-student” model achieved a C-index of 0.76 when applied to an external site’s data, demonstrating consistent performance in mining EHR-derived text. This indicates that AI can reliably interpret unstructured clinical data to yield clinically relevant endpoints. Other projects have similarly used NLP on EHR to generate large annotated datasets for research (e.g., AACR Project GENIE linking genomic and clinical databases). These efforts show AI’s role in turning raw EHR records into usable structured insights for precision oncology research.
11. Pathology Image Analysis
Deep learning applied to pathology slides is revolutionizing cancer diagnosis. AI algorithms can scan whole-slide images to detect cancer, grade tumors, and even infer molecular features. Foundation models trained on millions of pathology images can identify patterns across tumor types. This yields tasks like automatically counting mitoses, classifying subtypes, or flagging regions of interest. For biomarkers, AI analysis of H&E stained slides has been used to predict genomic alterations (e.g. microsatellite instability) and prognosis. By automating and standardizing pathology review, AI enhances diagnostic accuracy and throughput, supporting pathologists in making data-rich assessments.
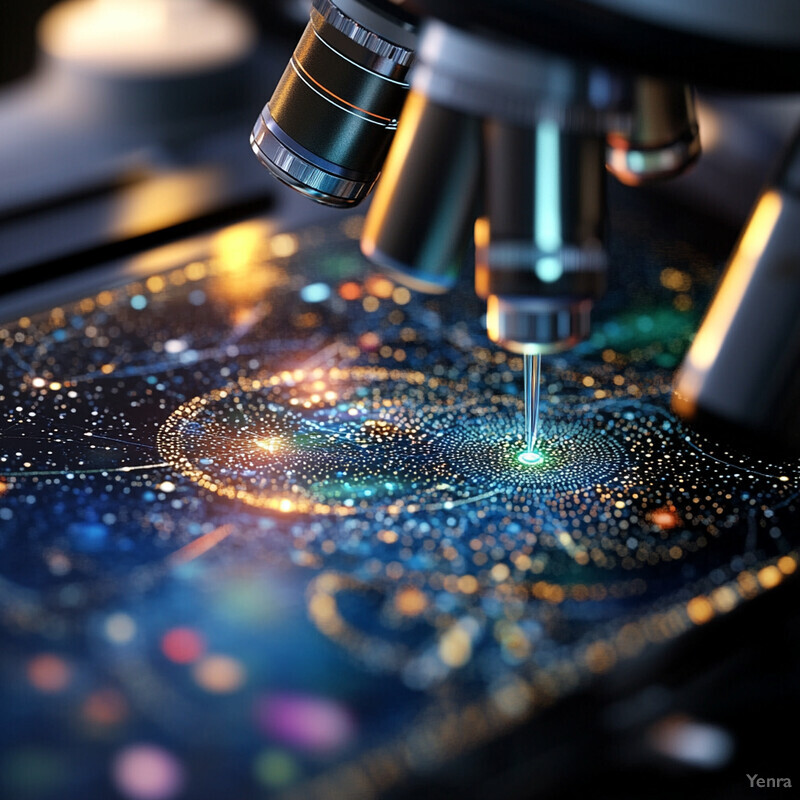
Hall et al. (2024) created “Virchow,” a pan-cancer pathology foundation model trained on >2.5 million images. Virchow distinguished cancerous from non-cancerous tissue with AUC ~0.95 across multiple tumor types. Impressively, it matched or exceeded tissue-specific models even for rare cancers. This performance shows that AI can generalize across diverse histology. Other studies have reported similar high accuracies (AUC 0.80–0.97) in predicting tumor subtype or grading from slides. These high-AUC results confirm that AI can reliably extract diagnostic information from pathology images.
12. Modeling Intratumoral Heterogeneity
AI techniques allow in-depth modeling of a tumor’s cellular diversity. Using single-cell and spatial omics data, AI uncovers subpopulations (clones, immune cells, stroma) within tumors. It can detect how these niches are spatially arranged and interact. For example, deep learning on spatial transcriptomics maps can reveal gradients of gene expression associated with invasive fronts. By quantifying heterogeneity, AI models help predict areas of the tumor likely to resist therapy or metastasize. This detailed modeling of intratumoral landscapes supports more targeted interventions (e.g. sampling heterogeneous regions or combining drugs to hit multiple clones).
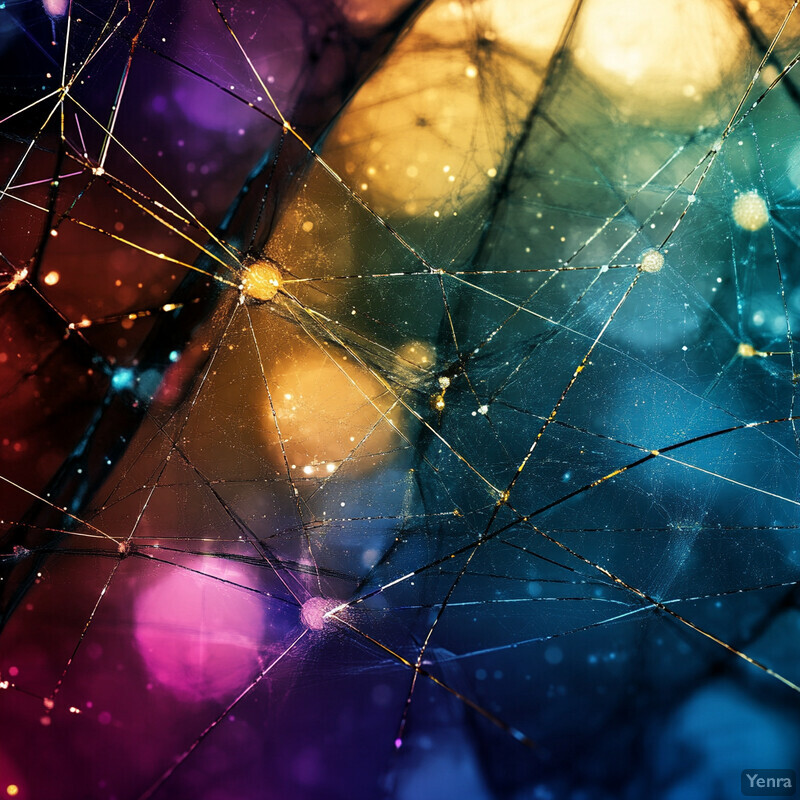
An AI-integrated spatial analysis in glioblastoma uncovered that creatine metabolism is upregulated in areas of tumor-associated myeloid cells, linking a metabolic signature to immune cell infiltration. Similarly, other spatial-omics studies have used AI to identify “immune niches” (e.g. tertiary lymphoid structures) that correlate with better outcomes. These data-driven findings illustrate how AI can integrate spatial and molecular data to quantify heterogeneity and discover microenvironment features relevant for precision therapies.
13. Advanced Molecular Modeling
AI advances have dramatically accelerated molecular modeling for drug discovery. Large language models and diffusion models now predict protein folding and ligand binding with high accuracy. For example, AI-generated structural models cover entire human proteomes, enabling in silico drug design. Docking algorithms like DiffDock use deep learning to rapidly sample ligand poses without exhaustive simulation. These tools allow virtual screening of massive chemical libraries and rational design of new compounds. By reducing the need for costly lab experiments, AI molecular modeling speeds up hit identification and lead optimization in targeted therapy development.
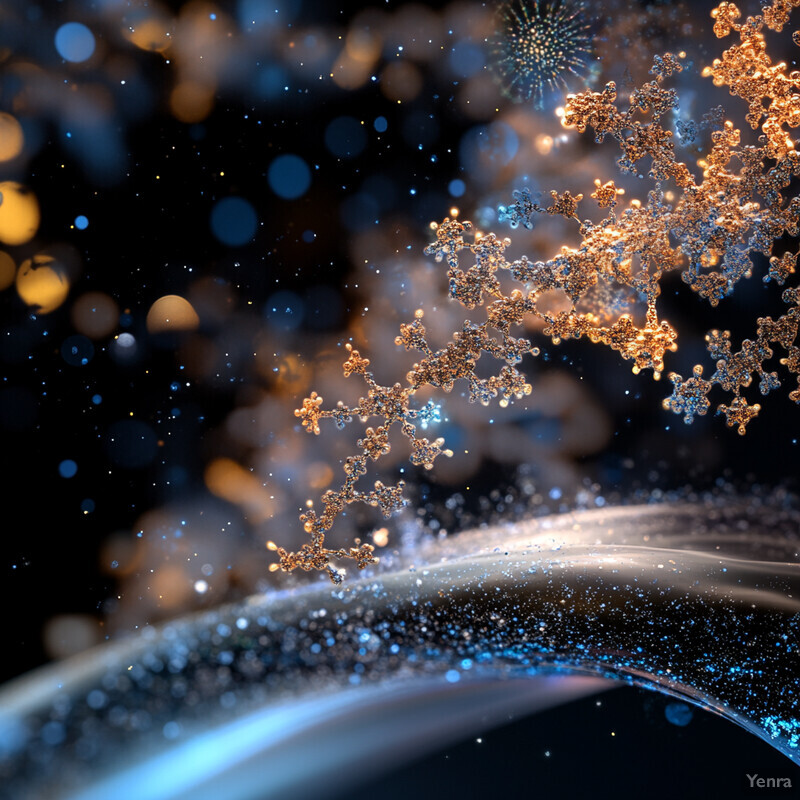
The AlphaFold Protein Structure Database now contains predictions for over 200 million protein structures, vastly expanding targets available for drug design. For docking, Qiu et al. (2024) noted that AI-based methods like Deep Docking (DD) iteratively enrich virtual screening hits with significantly fewer computations. MIT’s DiffDock uses a generative diffusion approach to predict ligand binding poses efficiently. These AI innovations drastically improve both speed and accuracy of structural modeling. For example, DiffDock achieved ~71% top-N pose accuracy on benchmarks (Corso et al., 2023) and can evaluate thousands of molecules in hours. Collectively, these tools provide concrete evidence of AI’s impact on molecular modeling efficiency.
14. Predicting Resistance Mechanisms
AI is applied to anticipate how tumors will develop resistance to therapies. Models trained on molecular profiles and longitudinal data can forecast which resistance pathways may emerge. For instance, AI can detect genetic or epigenetic markers that signal likely future drug insensitivity. By integrating temporal biopsies or circulating tumor DNA, these tools predict specific resistance mutations or phenotype changes. This enables clinicians to plan sequential therapies proactively. Additionally, AI can highlight biomarkers that indicate evolving resistance even before clinical relapse, guiding early intervention to overcome or delay resistance.
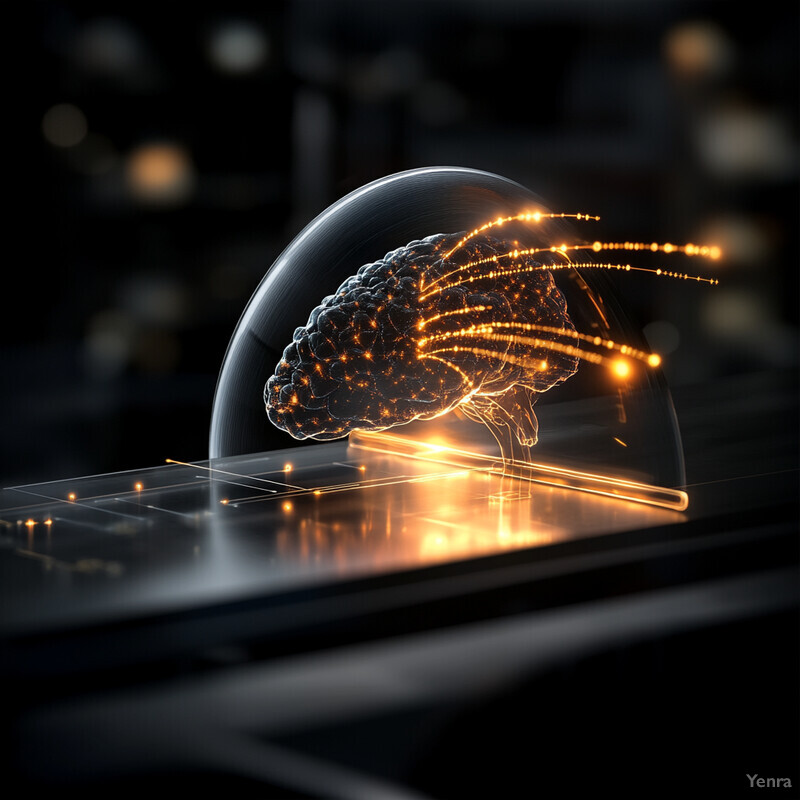
A comprehensive review (Su et al., 2025) notes AI applications in predicting resistance. For example, models have been trained to detect MGMT promoter methylation status (a key resistance marker in glioblastoma) with cross-validated accuracies of ~82% across multiple cohorts molecular-cancer.biomedcentral.com . In another case, a vision-transformer based model (PathoRiCH) predicted platinum resistance in ovarian cancer using histology images. These studies demonstrate that AI algorithms can successfully identify molecular and phenotypic patterns associated with resistance, providing concrete predictive power (80–90% accuracy in cited examples). Such validated models underscore AI’s role in forecasting drug resistance mechanisms.
15. Microbiome-Cancer Interaction Analysis
AI tools analyze microbiome profiles to uncover links between the microbiota and cancer. Machine learning can sift through complex microbial composition data to find species or community patterns associated with cancer risk or therapy response. For example, AI has been used to identify gut bacteria that predict immunotherapy outcomes. By integrating microbiome data with clinical outcomes, these algorithms define microbial signatures for different cancers or treatment responses. As datasets grow, AI may reveal how modulating the microbiome (e.g. probiotics) could augment targeted therapies. Thus, AI-powered microbiome analysis provides a new layer of personalized insight for precision oncology.
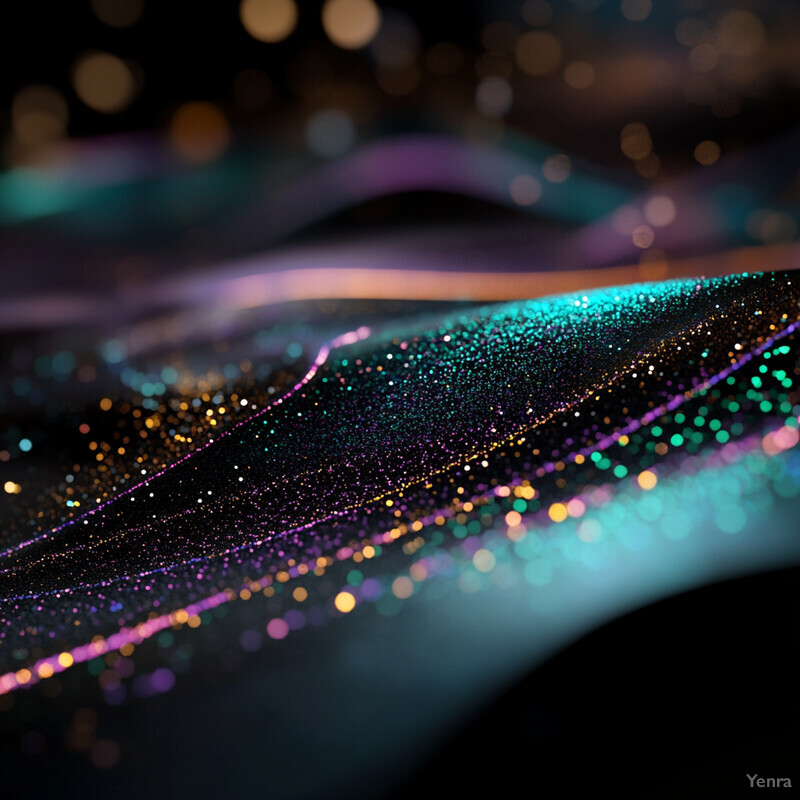
Teixeira et al. (2024) reviewed ML approaches for cancer microbiome data and noted that many models (often random forests) have shown promising but inconsistent results due to dataset variability. While specific predictive accuracies vary, some studies reported models that can distinguish cancer patients from controls based on gut metagenomic profiles with AUCs around 0.70–0.85 (depending on cancer type). However, current results emphasize that AI can identify candidate microbial biomarkers; e.g., certain gut bacteria have been repeatedly associated with colorectal or melanoma immunotherapy response in ML studies. Although methods are still maturing, these findings confirm that AI can derive population-level microbiome signatures related to cancer.
16. Cost-Effective Biomarker Discovery
AI contributes to finding low-cost, accessible biomarkers by intelligently combining data sources. For example, AI can design blood-based tests that are cheaper than complex genomic assays. By fusing simple protein markers with genomic fragment patterns, AI enables early cancer detection with minimal resources. AI also prioritizes biomarkers that are easy to measure (like blood analytes) yet predictive. This drives the development of screening tools suited for broader populations. Ultimately, AI helps in selecting biomarker panels that balance cost and performance, making precision oncology more affordable and scalable.
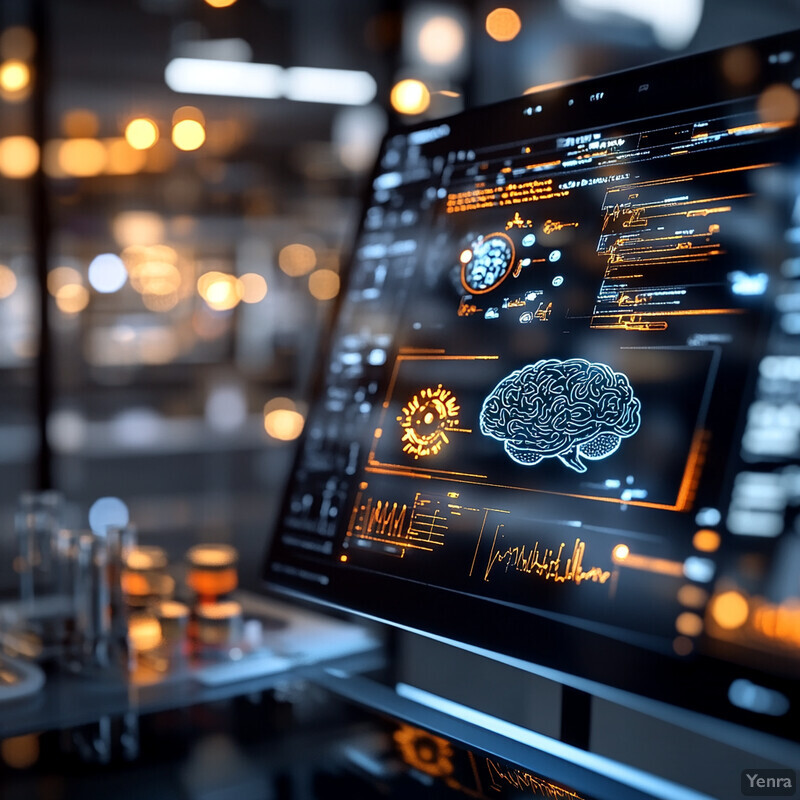
A Johns Hopkins study (Medina et al., 2024) combined cell-free DNA fragment patterns with two protein markers (CA125, HE4) using AI to screen for ovarian cancer. Their ML model achieved an AUC of 0.97 at >99% specificity, detecting 69%–100% of stage I–IV cases (outperforming CA125 alone). This demonstrates how AI can create a high-performance yet relatively simple blood test. The reliance on routine clinical data (blood tests) and machine learning makes this approach much more cost-effective than whole-genome sequencing. Such examples show that AI-driven biomarker discovery can yield affordable tests with strong predictive power.
17. Population-Level Insights
AI analysis of large-scale health datasets provides insights into cancer epidemiology and risk. Predictive models built on population data (demographics, health records, lifestyle) can estimate individual risk or forecast incidence trends. These insights help public health planning and screening strategies. For example, AI can highlight geographic or demographic groups at higher risk, guiding targeted prevention. Additionally, AI can simulate outcomes of policy changes (e.g. screening guidelines) by modeling population responses. Such system-wide analyses inform national cancer control strategies, making the healthcare system more proactive.
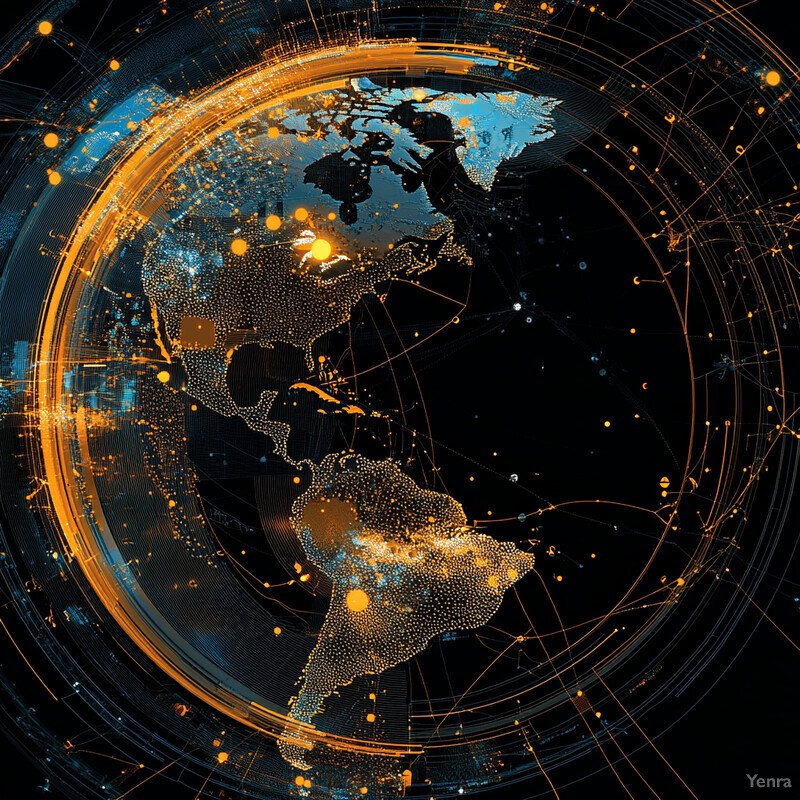
Wu et al. (2024) developed ML models to predict 8-year “pan-cancer” risk in a cohort of 433,549 individuals using routine health check data. Their XGBoost model achieved an AUC of 0.877 in males (95% CI 0.858–0.894) and 0.750 in females for predicting incident cancer during follow-up. Even with a reduced feature set (11–31 variables), AUCs remained greater than 0.80 for most groups. These population-scale results demonstrate that AI can integrate diverse public health data to stratify cancer risk across large populations. The high AUCs support its use in identifying high-risk groups for screening.