1. Automated Hive Health Monitoring
AI-driven sensors continuously measure hive temperature, humidity, CO₂ levels, sound patterns, and vibration to gauge colony well-being. Advanced algorithms detect anomalies and alert beekeepers to early signs of stress, disease, or environmental problems.
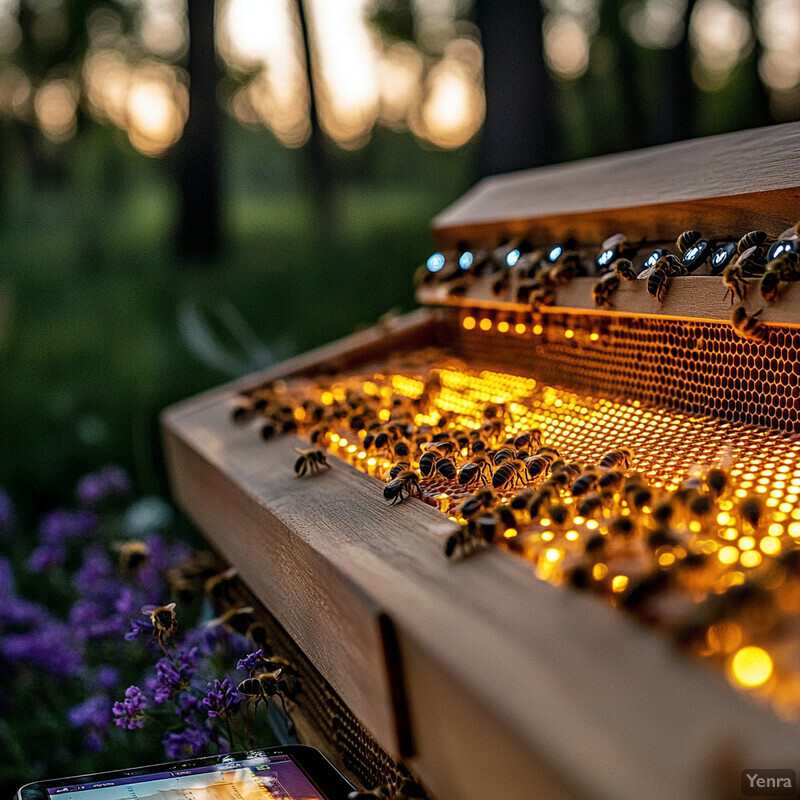
AI-enabled sensors, such as smart microchips and infrared cameras, can continuously record a hive’s internal conditions, including temperature, humidity, carbon dioxide levels, and even subtle vibration frequencies. Algorithms trained on historical data and known optimal ranges compare real-time metrics against established baselines to detect anomalies. For instance, a sudden drop in internal temperature might indicate problems with brood maintenance, or an uptick in CO2 levels could hint at ventilation issues. These systems can quickly flag early warning signs and send real-time alerts to beekeepers’ smartphones or computers, enabling immediate intervention. Such proactive, data-driven management reduces the risk of disease outbreaks, prevents colony collapse, and ensures that bees remain in a stable and productive environment.
2. Varroa Mite Detection and Counting
Computer vision models analyze high-resolution images of bees and comb frames to identify and count parasitic Varroa mites. This enables more timely and targeted interventions, reducing chemical treatments and preserving bee health.
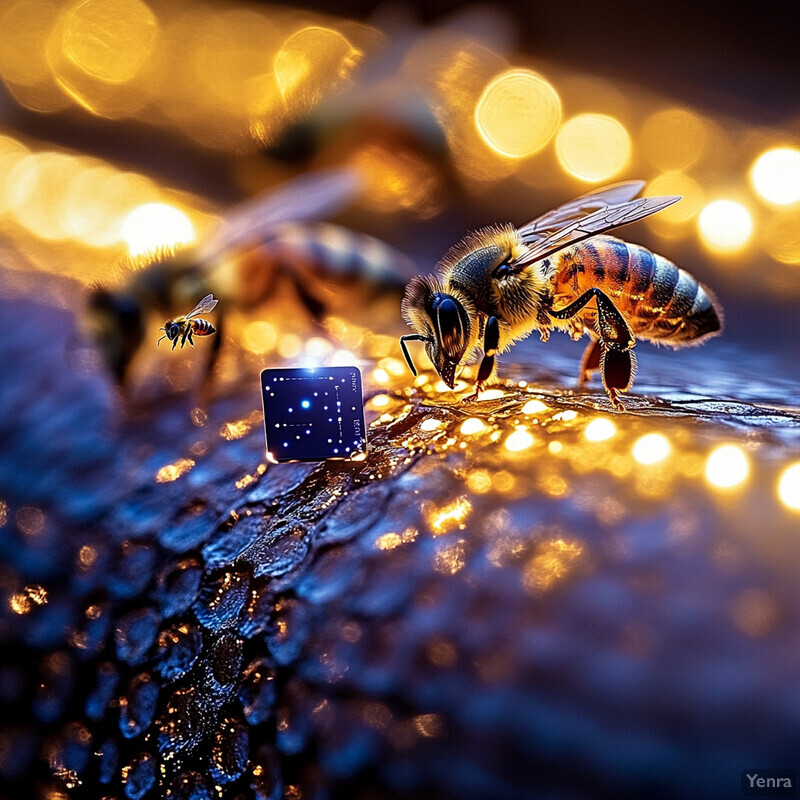
Varroa mites pose one of the most significant threats to modern beekeeping, as they weaken colonies by feeding on bees’ bodily fluids and spreading deadly viruses. Using high-resolution cameras and AI-based image recognition, beekeepers can quickly scan brood frames and detect the tiny, reddish-brown parasites. By automating mite counts, these systems reduce human error, save time, and facilitate more precise, targeted treatments. Rather than relying on broad chemical interventions, beekeepers can apply localized remedies or employ non-chemical approaches when mite infestations reach a predetermined threshold. Over time, this precision management approach leads to healthier bees, lower chemical residue in the hive, and more sustainable apiculture practices.
3. Real-Time Disease Diagnosis
Machine learning models trained on large datasets of bee health markers can detect early indicators of diseases like American foulbrood or Nosema. By recognizing subtle changes in bee appearance or behavior, AI can prompt swift action to contain outbreaks.
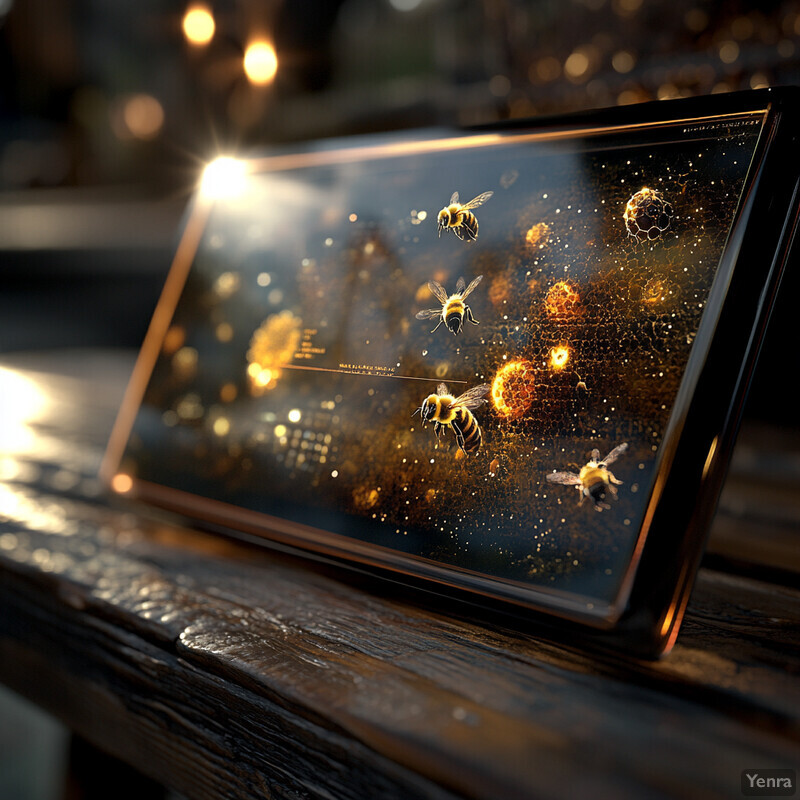
Bee diseases like American foulbrood, Nosema, or chalkbrood often present subtle early symptoms that can be easily missed by the naked eye. Advanced machine learning models, trained on thousands of images and data points from diseased and healthy hives, can learn to distinguish early-stage infections from normal variations. These AI diagnostic tools analyze images, sounds, and behavioral patterns to detect anomalies early on. By providing immediate, accurate detection, they empower beekeepers to isolate affected hives, apply targeted treatments, and prevent the disease from spreading. This swift, data-driven response not only reduces colony losses but also improves the overall health and resilience of the beekeeping operation.
4. Foraging Behavior Analysis
Advanced telemetry and computer vision allow AI to track individual bees’ flight patterns, identifying optimal pollen and nectar sources. The resulting insights help beekeepers choose better hive locations and improve forage availability.
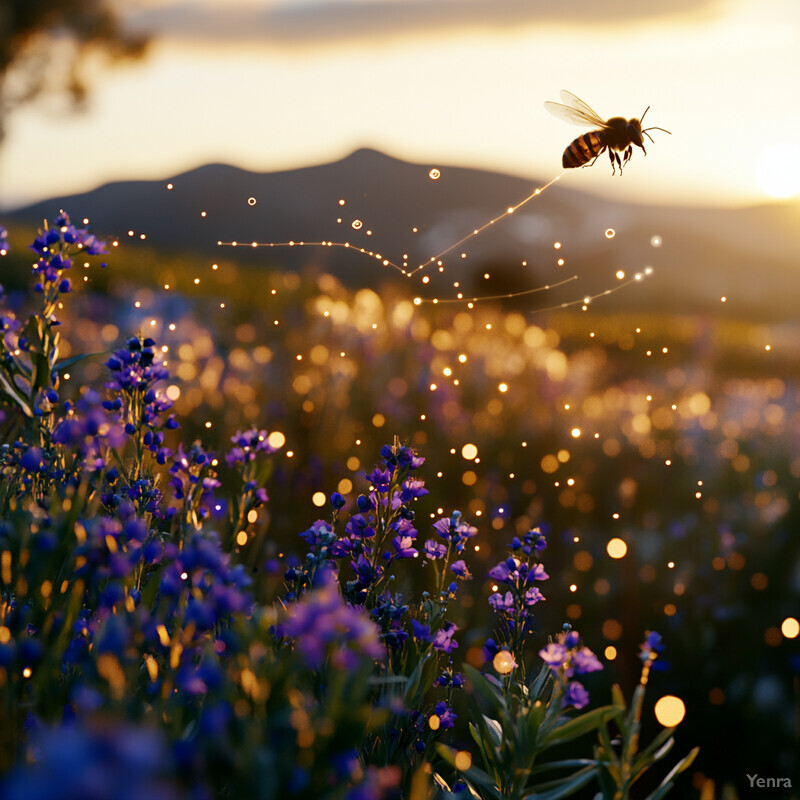
Understanding where bees forage, how far they travel, and which flowers they prefer is crucial for improving both pollination services and hive productivity. With AI-powered tracking—employing RFID tags, computer vision, or GPS micro-transmitters—beekeepers can map out bees’ flight patterns and feeding preferences in great detail. Machine learning models synthesize these data into actionable insights, such as identifying areas of abundant nectar flow, highlighting regions with diverse pollen sources, or pinpointing potential forage gaps. By leveraging these insights, beekeepers can strategically position hives, plant supportive flora, and collaborate with nearby farmers to ensure high-quality forage throughout the bees’ active season, ultimately enhancing colony strength and honey yields.
5. Colony Collapse Prediction
Predictive analytics assess a wide array of factors—weather patterns, hive acoustics, bee population changes, pesticide exposure, and nectar availability—to forecast the likelihood of colony collapse. Early warnings help beekeepers take preventative measures.
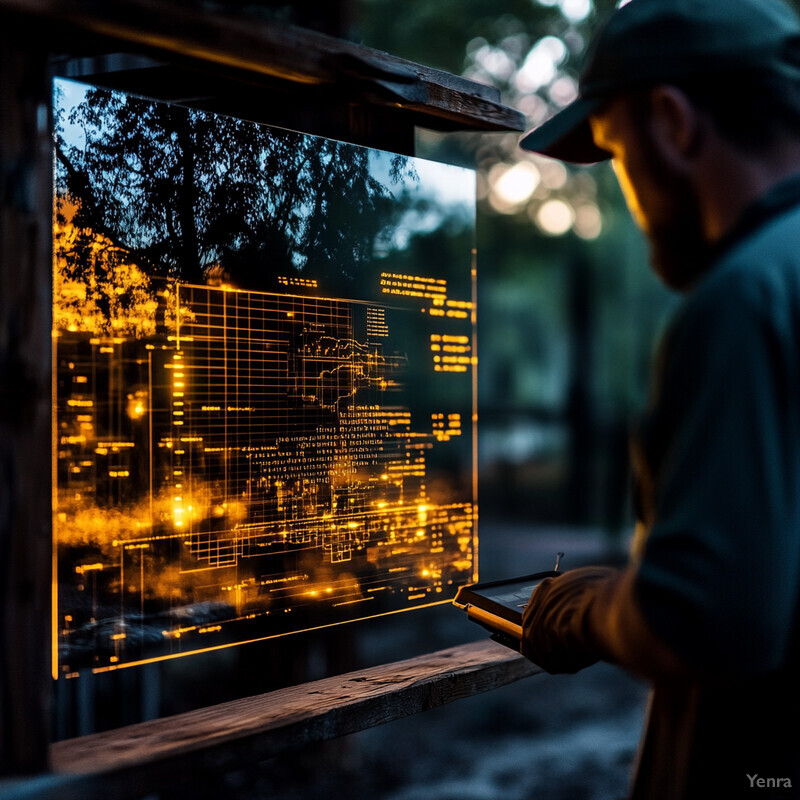
Colony Collapse Disorder (CCD) is a complex phenomenon influenced by numerous factors, including pests, pathogens, pesticides, nutritional deficiencies, and climate stressors. AI-based predictive analytics integrate multi-modal data—such as hive acoustics, population dynamics, environmental metrics, and historical hive performance—to forecast the likelihood of collapse. By examining patterns that would be too subtle or complex for humans to recognize, these algorithms provide early warnings that help beekeepers implement timely protective measures. This might include adjusting hive location, improving dietary supplements, increasing disease surveillance, or reducing exposure to agricultural chemicals. Proactive interventions guided by these predictive models can significantly lower the incidence of CCD and safeguard beekeeping operations.
6. Optimized Nutrition Management
Machine learning systems analyze bee growth rates, brood patterns, and environmental conditions to recommend optimal feeding regimens, ensuring that hives receive the precise nutrients needed at each developmental stage.
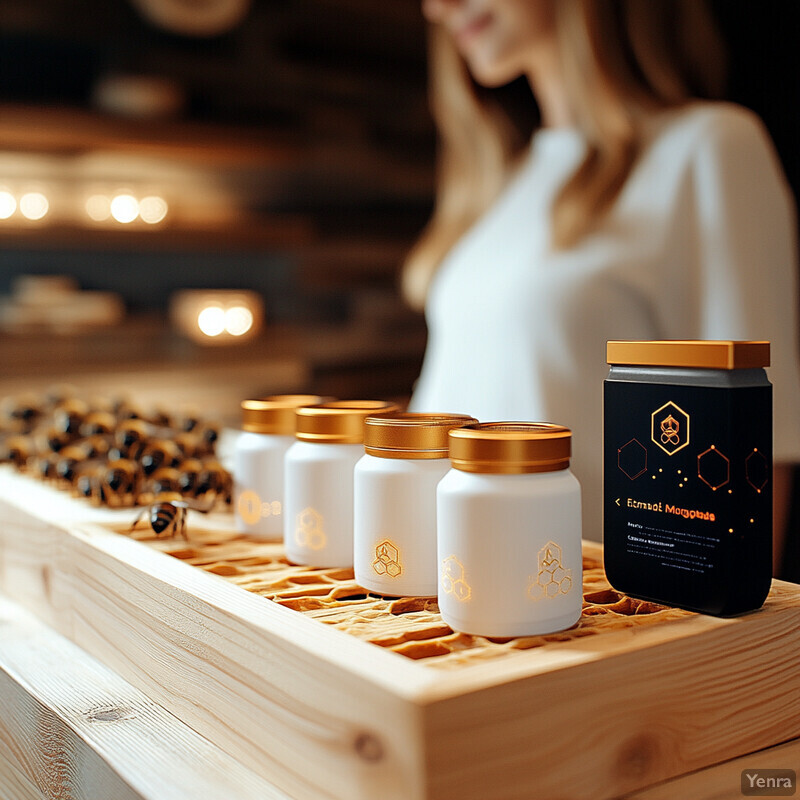
Just as athletes have tailored diets, bees benefit from precise nutritional support at different developmental stages. AI-driven models tap into data on brood pattern, forager return rates, local floral diversity, and weather forecasts to tailor feeding recommendations. For example, if the natural pollen supply is forecasted to dip in the coming weeks, the system might suggest supplemental protein feeds. These nuanced diet plans can help maintain robust brood development, ensure adequate honey storage, and bolster immune systems against disease. By continually fine-tuning the nutritional profile offered to bees, AI ensures colonies remain vigorous and productive even under changing environmental conditions.
7. Behavioral Insights Through Audio Analysis
By using AI to decode hive sounds, it is possible to detect stress signals, swarming intentions, or queenlessness. Speech recognition-like algorithms identify subtle acoustic patterns that indicate a colony’s internal state.
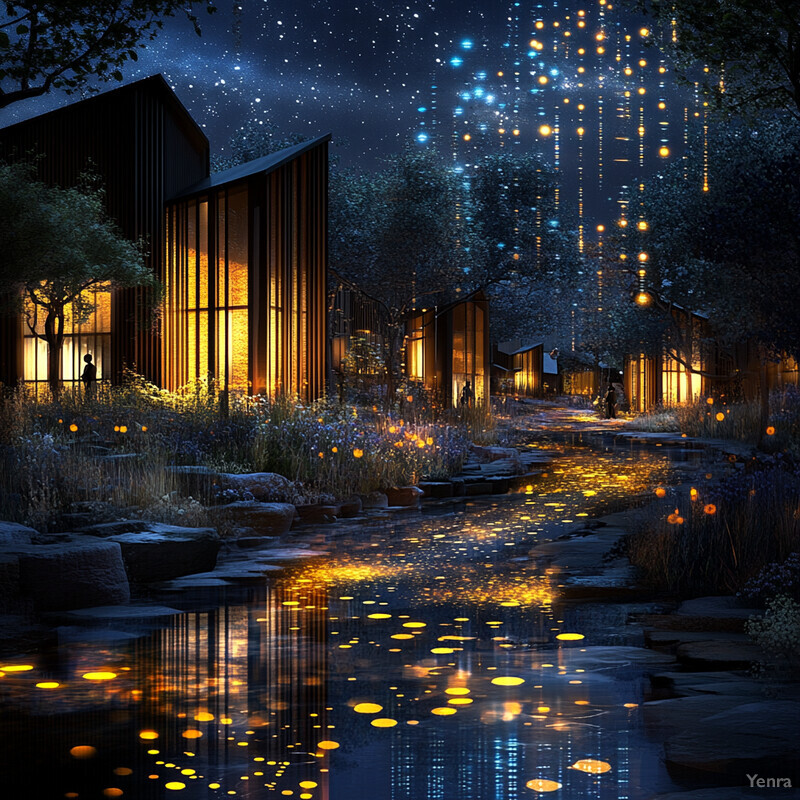
Bees communicate through buzzing patterns and vibrations that convey critical information about their internal state. Machine learning techniques, akin to speech recognition software, can analyze audio data to detect signals of swarming, queen loss, or stress. The subtle changes in pitch, pattern, or volume can alert beekeepers to issues well before they become visually apparent. By logging these changes over time, AI audio systems can identify trends, predict seasonal behaviors, and differentiate between normal fluctuations and warning signs. This innovative use of sound diagnostics transforms passive observations into precise, actionable insights, allowing beekeepers to respond promptly to a colony’s needs.
8. Smart Hive Entrances
AI-enabled “smart entrances” integrate computer vision to count bees entering and exiting the hive and monitor pollen loads. This data helps determine colony productivity and identify potential issues like robbing or predator attacks.
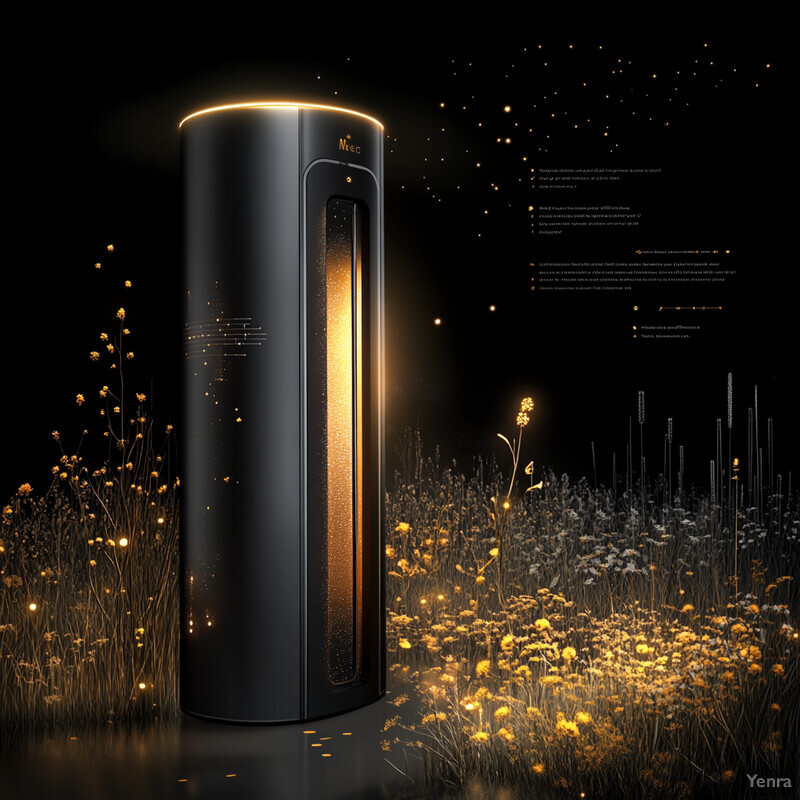
A hive’s entrance is a bustling gateway where thousands of bees come and go each day. AI-enabled cameras and sensors mounted at the entrance count bees, measure the loads of pollen they carry, and even estimate the colony’s productivity. These systems can detect anomalies, such as unusual forager losses, sudden drops in returning pollen, or signs of robbing by other bees. Early detection of entrance-level problems allows beekeepers to step in quickly, reinforcing the hive structure, adjusting feeding plans, or relocating the colony if necessary. The entrance thus becomes a critical data collection point, offering a real-time window into hive health and efficiency.
9. Adaptive Hive Climate Control
Intelligent climate control systems, guided by AI, regulate temperature, humidity, and ventilation inside the hive. They adapt dynamically based on colony size, brood composition, and external weather, ensuring ideal hive conditions year-round.
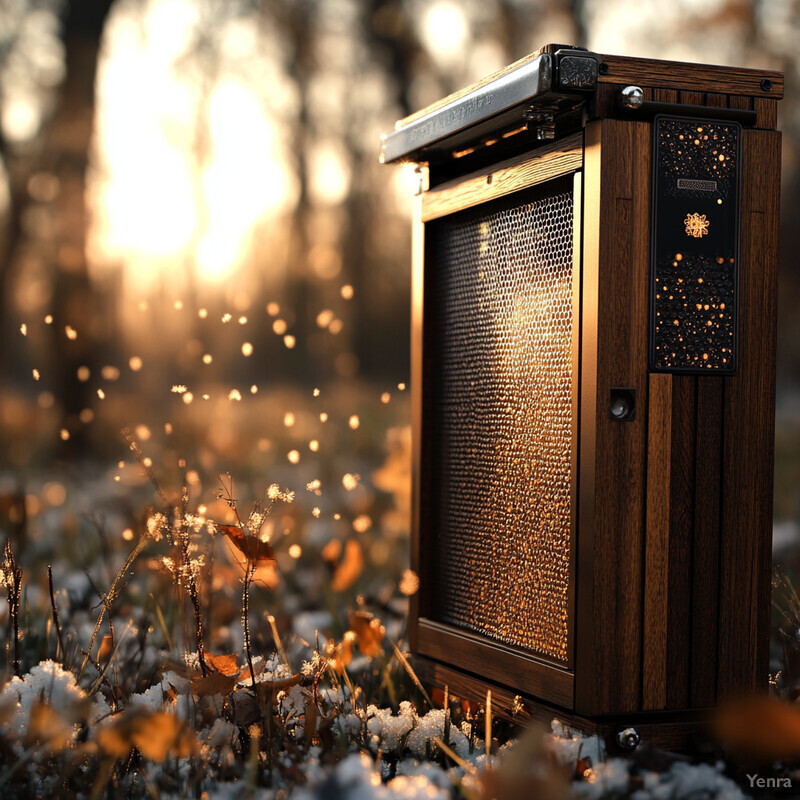
Maintaining an optimal internal climate is vital for brood development and overall colony health. AI-driven climate control systems use sensor networks to monitor temperature, humidity, and ventilation within the hive. When external weather conditions shift—such as a sudden cold snap or a heatwave—the system automatically adjusts ventilation or insulation. Over time, machine learning algorithms refine these adjustments by considering colony size, brood stage, and local climate patterns. This precision results in stable brood rearing conditions, reduced stress on adult bees, and efficient energy use. Hives equipped with adaptive climate control can better withstand environmental fluctuations, enhancing survival and productivity rates.
10. Precision Pollination Forecasting
By combining AI-driven predictive models with field-level data, beekeepers and farmers can anticipate pollination readiness, ensuring that bees are deployed at peak bloom for maximum crop yield.
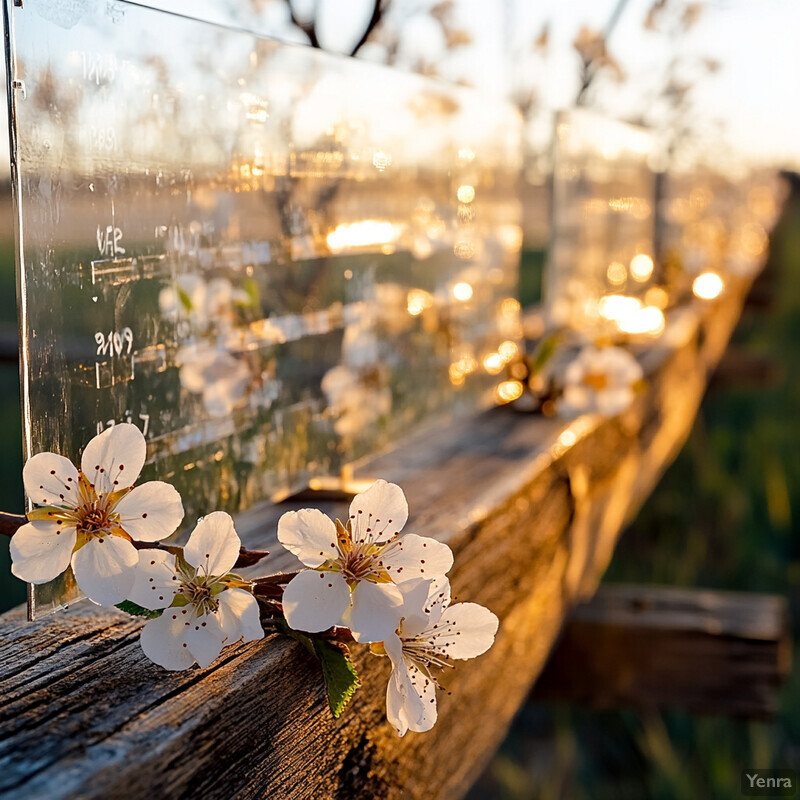
Agricultural success often depends on the timely presence of pollinators. By combining weather forecasts, bloom timelines, hive activity data, and historical records, AI models predict when and where bees will best complement flowering crops. These insights enable beekeepers and farmers to coordinate hive placement and timing for maximum pollination effectiveness. Farmers can schedule irrigation or pesticide applications around bee activity, while beekeepers ensure their colonies are at peak foraging strength when the blossoms appear. This synergy increases crop yields, optimizes resource use, and supports a mutually beneficial relationship between pollinators and the agricultural ecosystem.
11. Pesticide Exposure Monitoring
AI systems analyze sensor data, bee mortality patterns, and local pesticide usage logs to estimate a colony’s exposure risk. This information helps in making informed decisions on hive placement and timing to minimize chemical hazards.
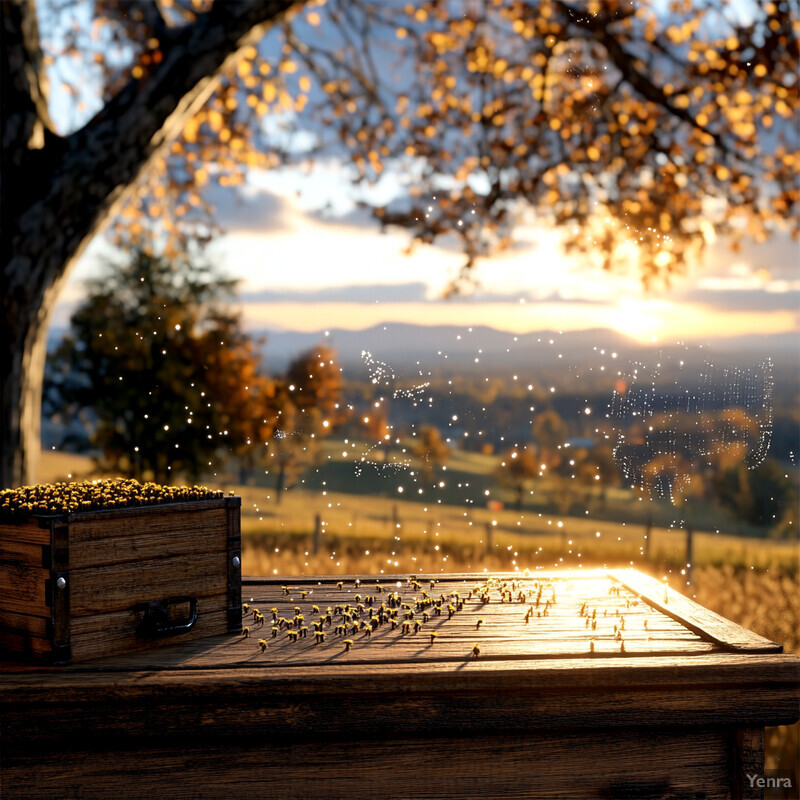
Exposure to certain agricultural chemicals can weaken or kill honeybees. AI systems pull data from sensors placed in or near the hive, along with publicly available pesticide usage logs, to assess the colony’s risk of exposure. By analyzing correlations between bee health metrics—such as mortality rates or reduced foraging—and the timing of nearby pesticide applications, machine learning algorithms can identify higher-risk periods. Beekeepers can then respond by temporarily covering hives, relocating them, or working with farmers to choose safer application windows. Through continuous risk assessment, these AI-driven solutions help protect bee colonies from harmful chemical impacts, improving long-term colony survival.
12. Informed Queen Cultivation Programs
Deep learning models analyze genetic data, colony performance metrics, and environmental adaptation to guide selective breeding of queens. This accelerates the development of disease-resistant, more productive bee strains.
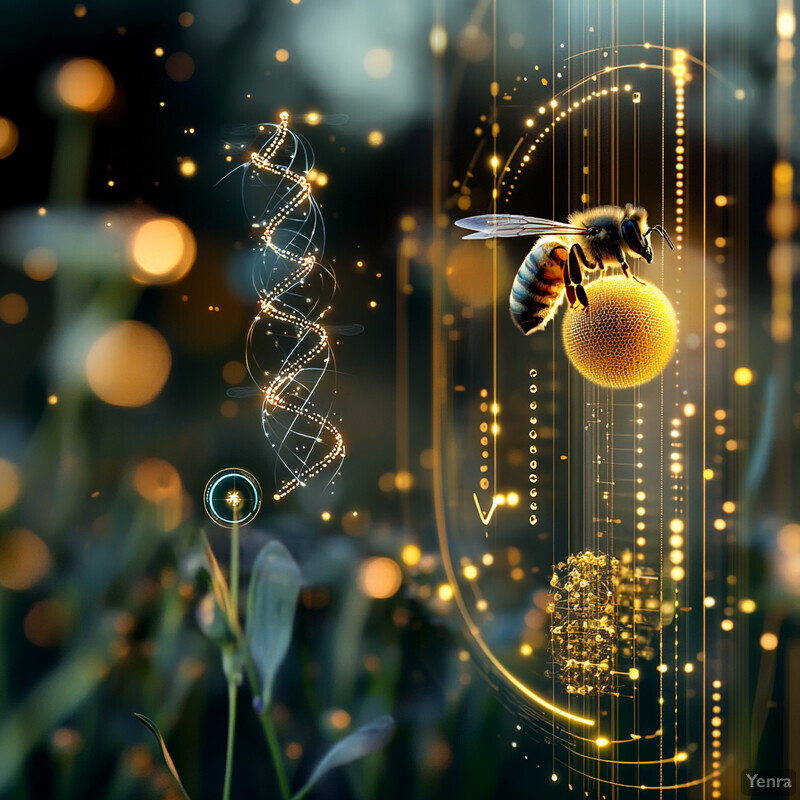
The genetic quality of a queen bee greatly influences colony health, productivity, temperament, and disease resistance. AI models integrate performance data—such as honey yields, brood patterns, and foraging success—from numerous colonies alongside genetic information and local environmental conditions. By identifying which genetic traits correlate with robust health and productivity under certain circumstances, these models guide selective breeding strategies. Over time, this accelerates the development of queens with desirable traits, such as improved disease resistance or adaptability to local climates. The result is a stronger genetic foundation for future colonies, improving sustainability and resilience across the beekeeping industry.
13. Dynamic Hive Population Estimates
Vision-based counting and modeling assess the population of worker bees, drones, and brood cells. By providing consistent, automated counts, AI helps beekeepers track population health and expansion over time.
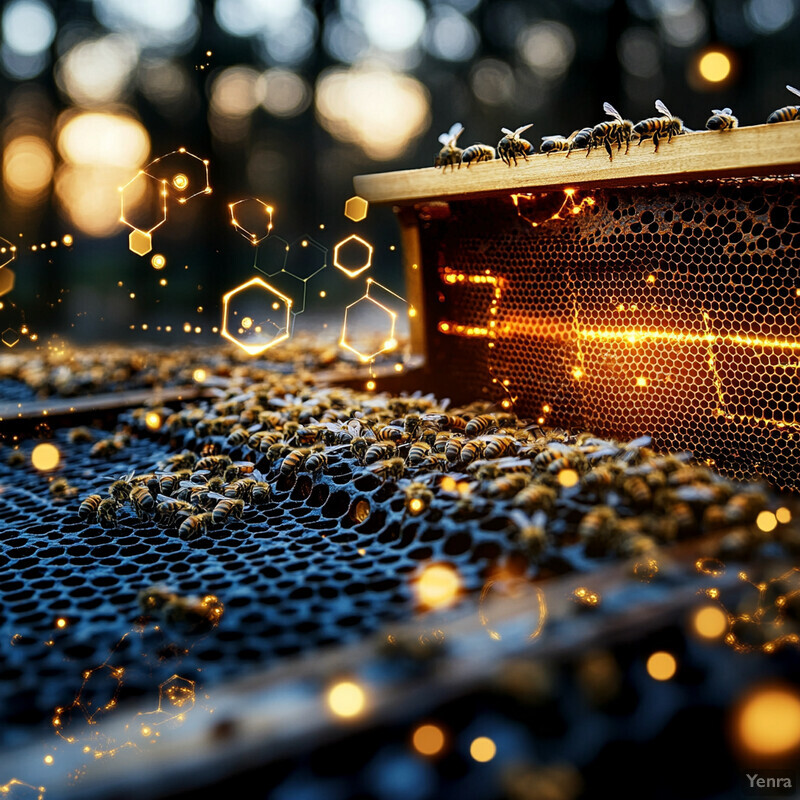
Manually estimating hive populations can be laborious and imprecise. AI-based vision systems can count bees and brood cells from hive inspection images or videos, providing accurate population metrics without disturbing the bees. By tracking population changes over time, these systems help beekeepers understand colony growth rates, forager-to-brood ratios, and seasonal fluctuations. With precise population data, beekeepers can better predict when to add supers for honey storage, when to split hives to prevent swarming, or when to consolidate colonies for winter. This data-driven approach ensures that management decisions align with the colony’s natural rhythms and needs.
14. Stress Event Detection and Alerts
AI integrations with hive sensors can detect stress events such as predator disturbances, pesticide sprays nearby, or extreme weather shifts. Immediate alerts enable quick protective measures like relocating hives or sealing entrances temporarily.
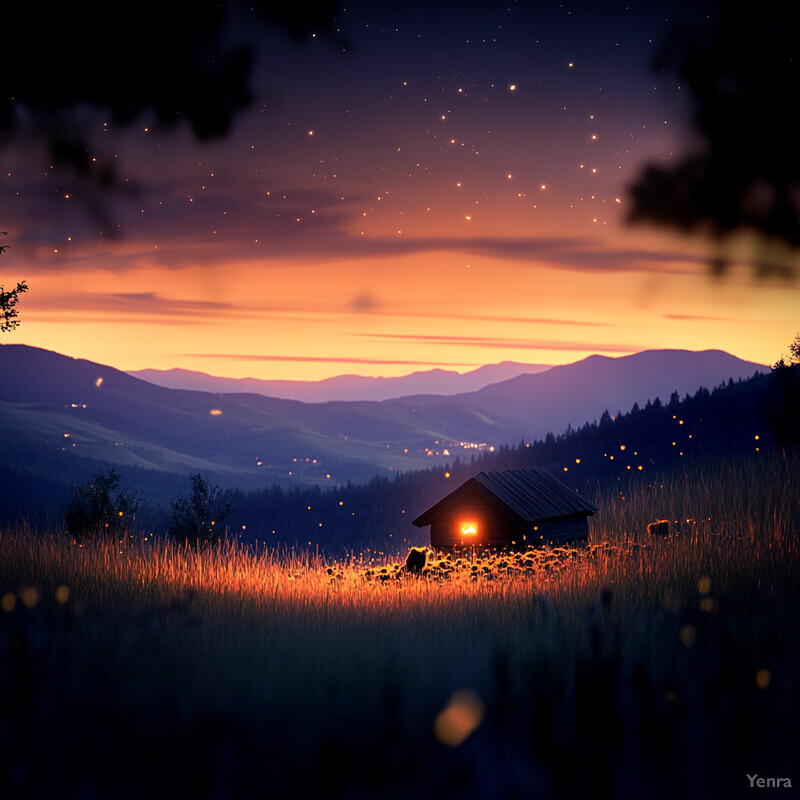
Hives sometimes face acute stress events like sudden attacks by predators (e.g., bears, skunks) or abrupt pesticide drift. Integrating AI with motion detectors, sound sensors, and environmental monitors enables real-time recognition of such disturbances. If, for example, an unexpected spike in hive vibration coincides with unusual noise, the system can send an immediate alert to the beekeeper’s device. Rapid detection allows for prompt on-site responses: relocating the hive, setting up deterrents, or temporarily sealing the entrance. By mitigating stress events before they escalate, beekeepers enhance colony well-being and minimize losses.
15. Predictive Maintenance of Equipment
Machine learning can forecast wear and tear on beekeeping equipment—such as hive components and feeders—based on historical usage, environmental conditions, and colony activity. This leads to proactive maintenance and improved hive longevity.
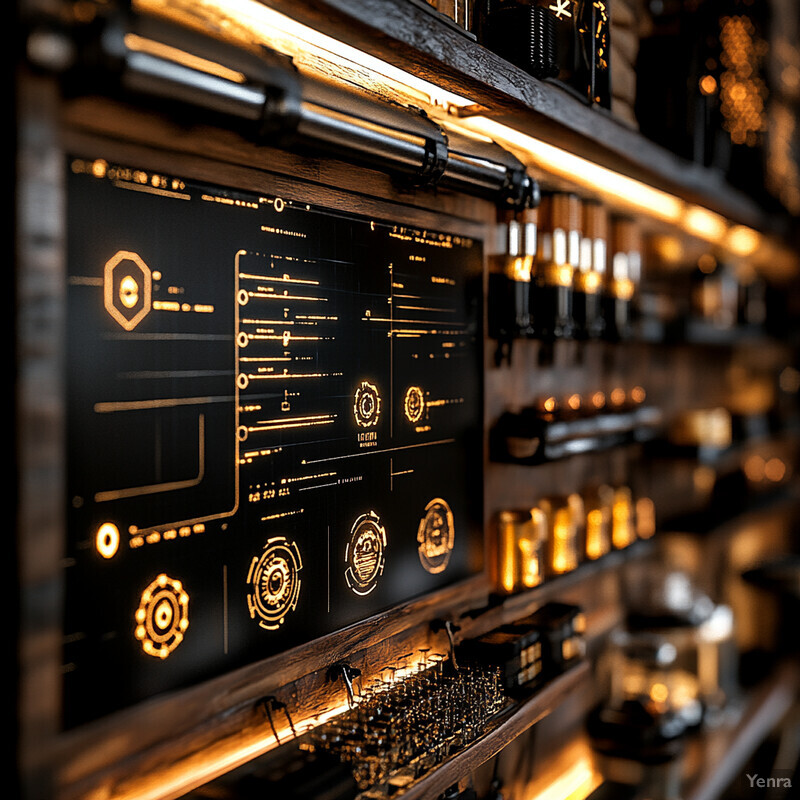
Beekeeping equipment—from hive boxes and frames to feeders and sensors—undergoes wear and tear over time. Machine learning models can analyze data on temperature fluctuations, colony population changes, and historical equipment lifespans to predict when maintenance will be required. By anticipating repairs or part replacements, beekeepers avoid sudden equipment failures that could compromise hive health. Predictive maintenance reduces downtime, extends the lifespan of materials, and ensures hives remain stable and protective environments for bees. A proactive approach to equipment management also lowers costs and streamlines beekeeping operations.
16. Hive Theft and Tampering Detection
Computer vision and anomaly detection algorithms can recognize when hives are being moved or opened unexpectedly. This supports theft prevention and provides early warnings if someone tampers with the colony.
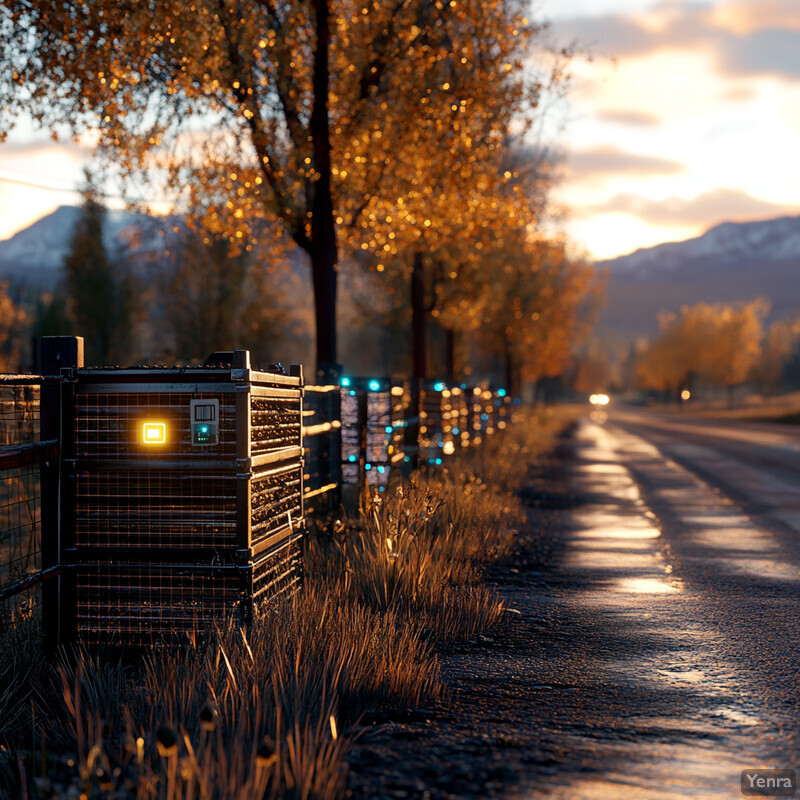
Hive theft is a growing concern, particularly in regions where pollination services are in high demand. AI-equipped security systems, using camera feeds and motion sensors, can distinguish between normal beekeeper activities and suspicious movements. If a hive is moved unexpectedly, the system sends instant alerts and can even trigger tracking devices. This not only deters potential thieves but also aids in the rapid recovery of stolen hives. Ensuring hive security through AI-based monitoring saves beekeepers significant financial losses and protects the colonies from stress and displacement.
17. Global Best Practice Recommendations
AI platforms aggregate and analyze hive performance data from many regions. They identify best practices suited to different climates, flora, and disease pressures, providing locally adapted, data-driven management suggestions.
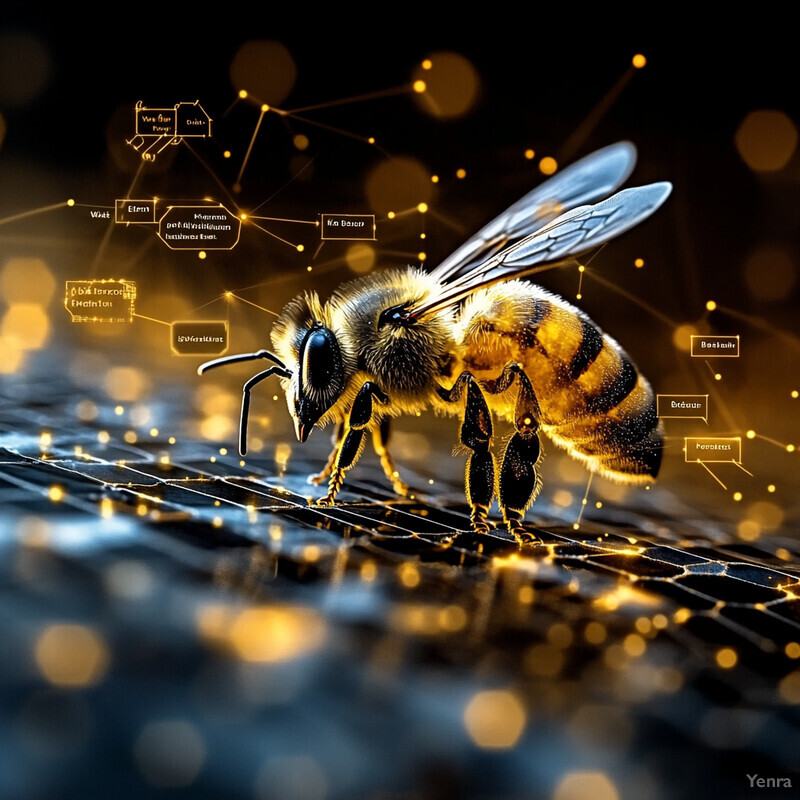
By aggregating performance data from a wide variety of environments, AI-driven platforms can identify what works best under specific conditions. For example, a management technique that succeeds in a humid coastal climate may not yield the same results in a dry inland region. AI analysis helps refine best practices, offering localized recommendations for feeding schedules, disease treatments, breeding approaches, and hive placement strategies. As this knowledge base grows, beekeepers worldwide benefit from evidence-based insights, accelerating improvements in beekeeping techniques and outcomes regardless of location or scale.
18. Climate Change Impact Modeling
Advanced simulations predict how changing weather patterns and temperatures may impact bee foraging, reproduction, and survival rates. This empowers beekeepers to adjust management strategies in advance of environmental shifts.
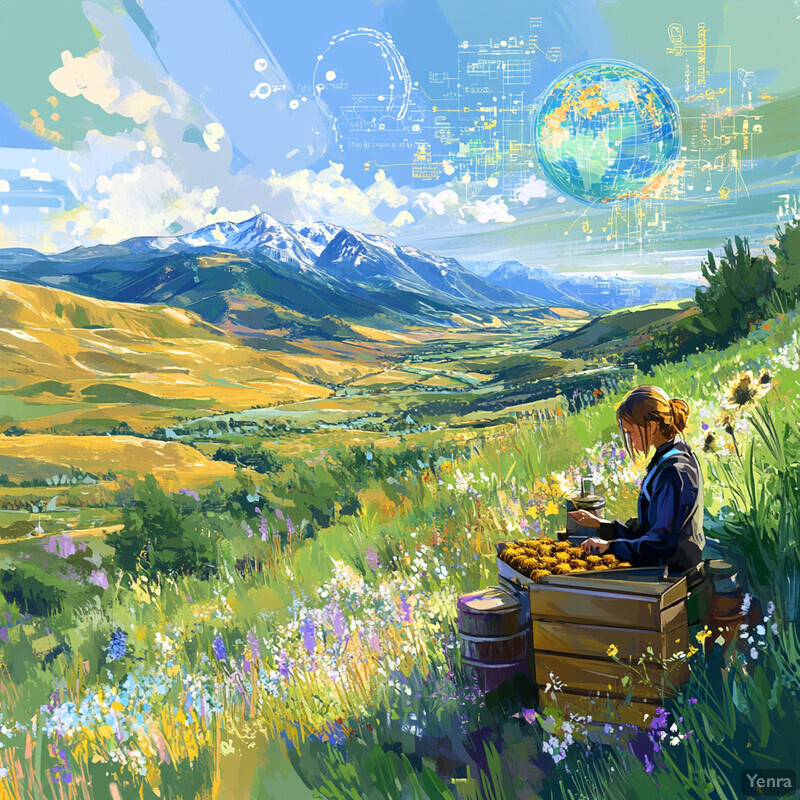
As global temperatures shift and weather patterns become more erratic, bees face new challenges in timing their forage, surviving colder winters, or withstanding hotter summers. AI models simulate how different climate scenarios might affect floral bloom times, forage availability, and hive health. Armed with these forecasts, beekeepers can plan adaptive strategies—such as diversifying pollen sources, providing additional shade, or adjusting feeding times—to help their colonies thrive amid changing conditions. By anticipating climate-driven challenges, beekeepers remain resilient, maintaining steady honey production and stable colony numbers even under environmental stress.
19. Efficient Hive Migration Decisions
Beekeepers relying on AI-driven analytics can decide when and where to move hives seasonally for improved forage, reduced disease risk, and better overwintering conditions, optimizing both productivity and bee welfare.
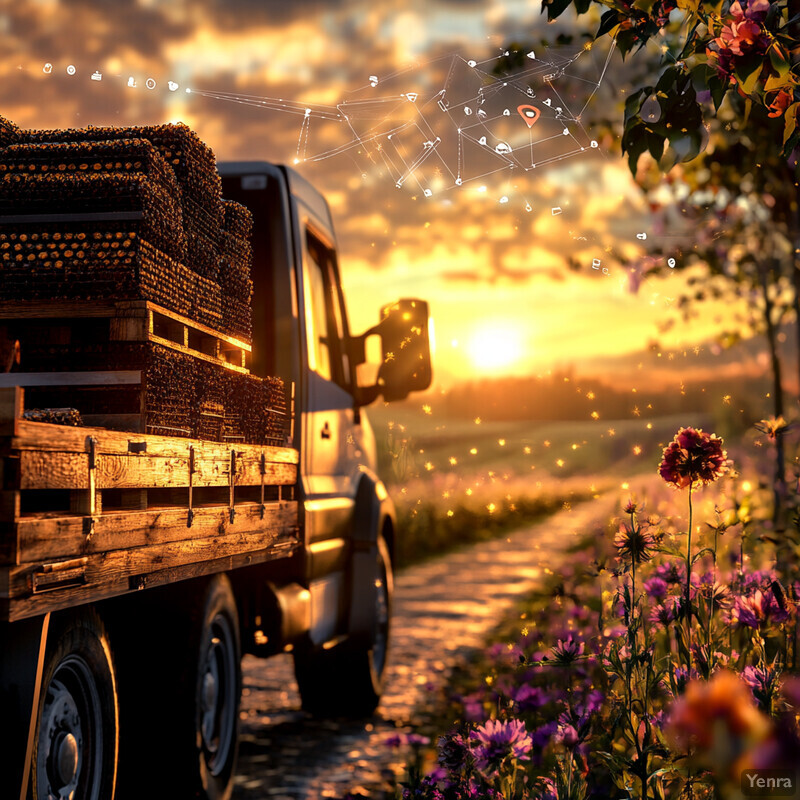
Migratory beekeeping moves hives seasonally to follow nectar flows or meet pollination demands for various crops. Deciding when and where to migrate is complex, considering weather, bloom cycles, local pests, and transportation logistics. AI algorithms compile these variables into predictive models that offer optimal migration schedules. They might suggest relocating a portion of the apiary a week earlier to catch an early apple bloom or waiting an extra few days in one spot to capitalize on a late-season clover flow. This data-driven approach to migration reduces trial-and-error guesswork, optimizes resource use, and ensures that colonies remain productive throughout the year.
20. Integration with Smart Farming Systems
Precision agriculture platforms that incorporate bee-related AI insights enable integrated farm management. Farmers and beekeepers can coordinate planting, spraying, irrigation, and hive placement to maximize pollination and ensure sustainable agricultural ecosystems.
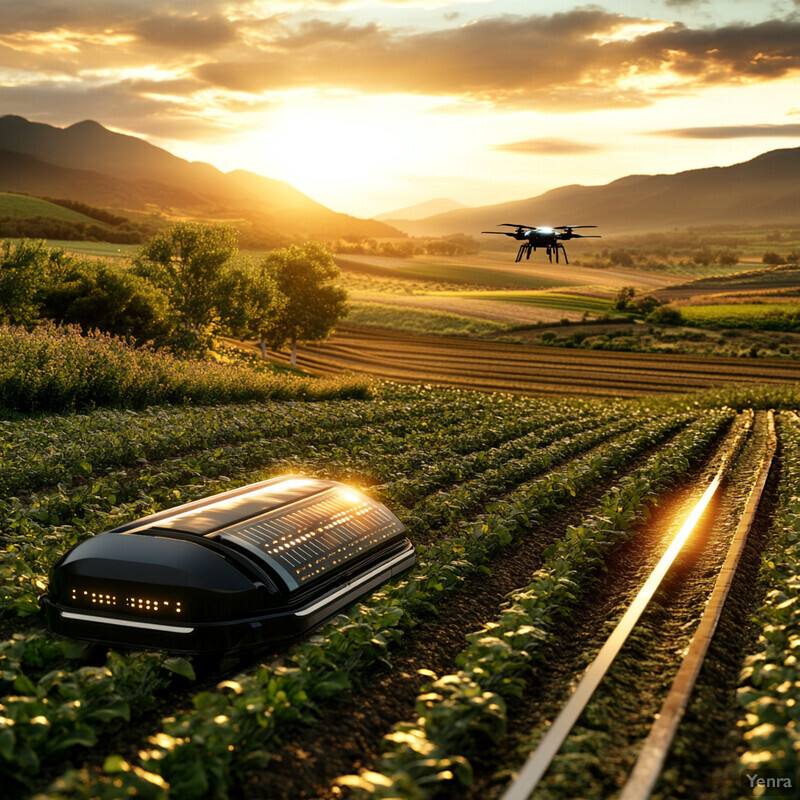
Modern agriculture increasingly relies on precision farming techniques, using AI to manage irrigation, fertilizer application, and pest control. By integrating beekeeping data into these platforms, farmers and beekeepers achieve a synchronized ecosystem. For example, if AI predicts a high pollination window for a crop, farmers can adjust their planting and watering schedules accordingly, while beekeepers ensure their colonies are at peak strength. Conversely, if the system warns of a forthcoming pesticide spray, beekeepers can shield their hives at the right time. This two-way information flow creates more sustainable, productive, and pollinator-friendly farmlands, driving synergy between crop production and healthy bee populations.