1. Automated Optimization of Lens Systems
AI-driven optimization algorithms can rapidly explore vast design spaces to determine optimal lens configurations, reducing the time and human effort traditionally required for manual trial-and-error methods.
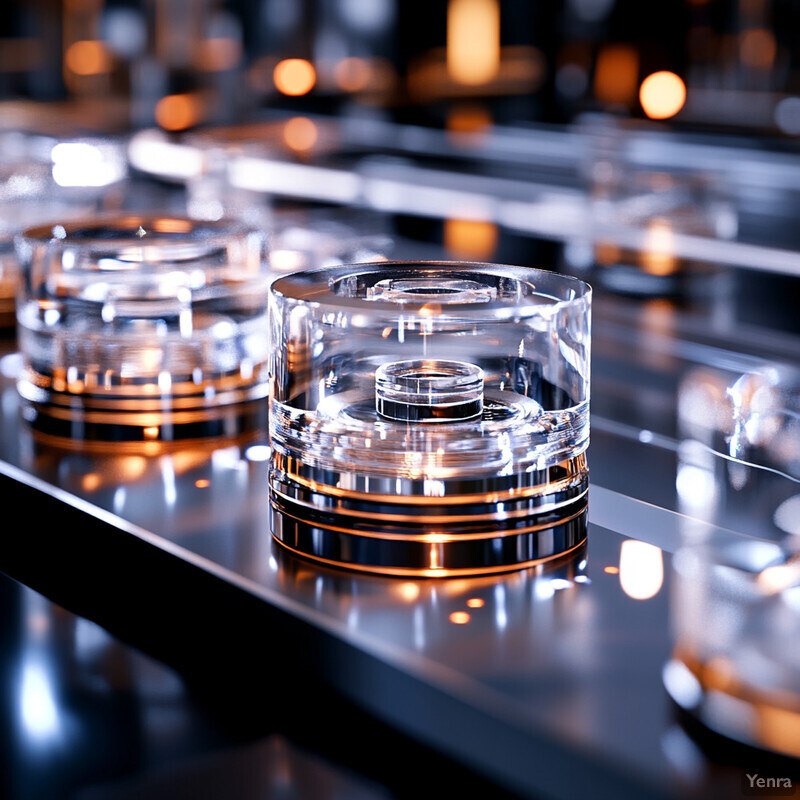
AI-driven algorithms are revolutionizing lens design by significantly reducing the trial-and-error process traditionally required. Instead of relying on experienced engineers to painstakingly tweak parameters, machine learning models can explore an expansive multi-dimensional design space at high speed. These algorithms utilize techniques like genetic optimization and gradient-based methods to refine parameters such as lens curvature, spacing, and aperture size until they converge on an optimal configuration. As a result, engineers can achieve superior image quality, reduced aberrations, and minimized optical distortions much faster than before. This automation not only cuts down development cycles but also frees experts to focus on higher-level conceptual tasks, ultimately improving both performance and time-to-market for advanced optical systems.
2. Inverse Design for Novel Optical Components
Machine learning models can invert the design process, starting from desired optical properties and working backward to determine the shapes, materials, and configurations needed to achieve them.
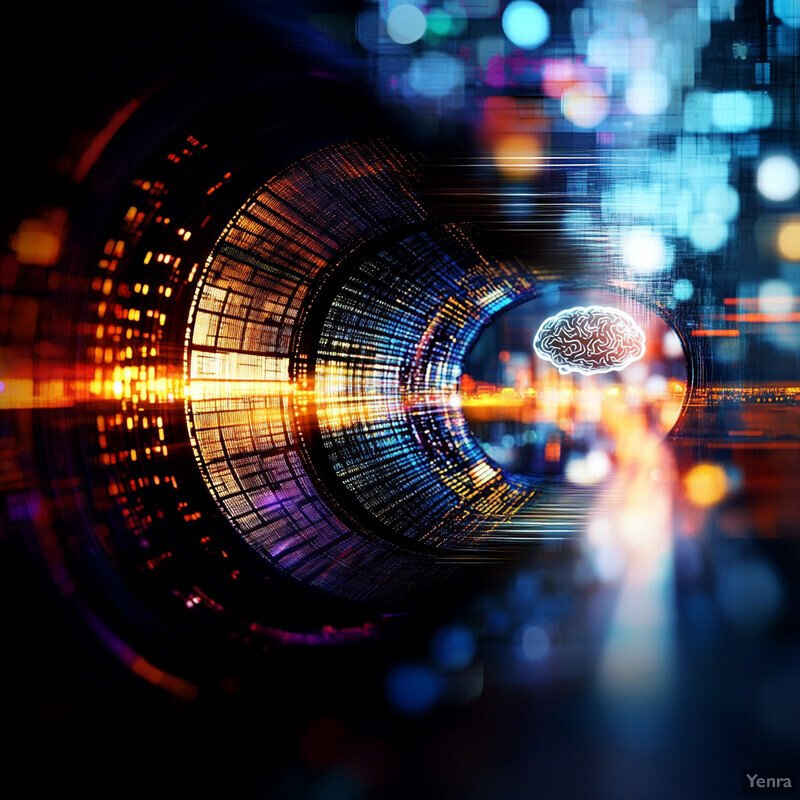
Inverse design methods leverage AI to start from a desired optical function—such as a specific focal length, polarization state, or spectral response—and work backward to uncover the geometric and material configurations that realize it. Instead of starting with a known shape and guessing how it will affect the light, machine learning models produce new device layouts that might not be intuitive to human designers. This approach can uncover unconventional freeform surfaces, intricate metasurfaces, or layered structures that deliver unprecedented optical performance. AI-driven inverse design can thus accelerate innovation, enabling the creation of optical elements that push the boundaries of what’s currently achievable. Ultimately, this process paves the way for more compact, efficient, and highly specialized devices that meet emerging demands in fields like augmented reality, quantum optics, and biomedical imaging.
3. Surrogate Modeling of Complex Systems
AI can create high-fidelity surrogate models that approximate time-consuming physical simulations, enabling designers to predict optical performance in real time.
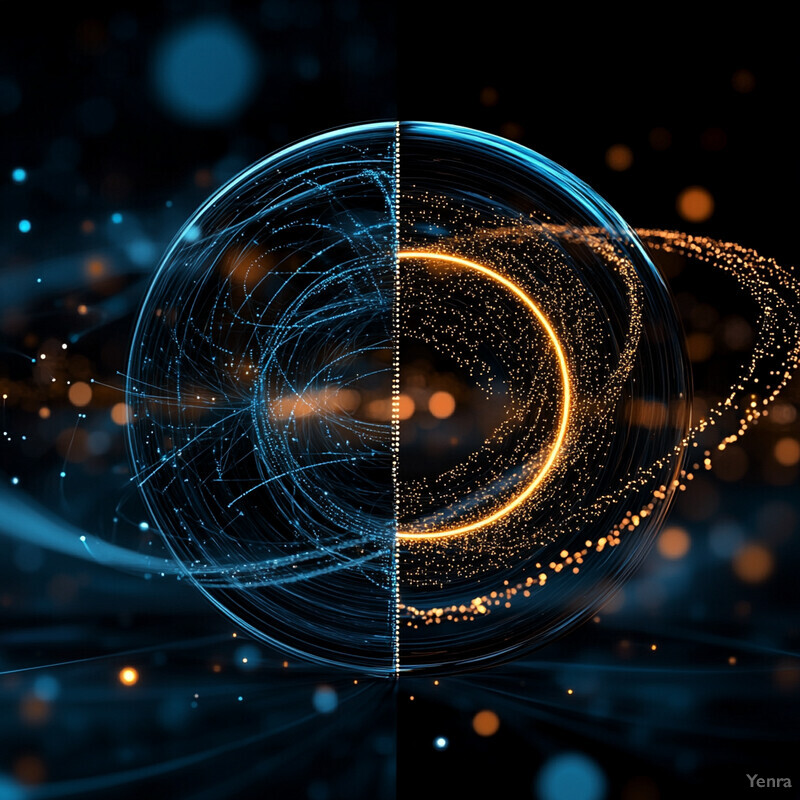
Optical simulations, such as rigorous electromagnetic field modeling or ray tracing through complex lens assemblies, can be computationally expensive and time-consuming. AI methods, especially deep learning, can create surrogate models that closely approximate these simulations with dramatically reduced computational overhead. By training on a representative dataset of known configurations and their corresponding outputs, these surrogate models can predict system performance almost instantaneously. As a result, engineers can rapidly iterate through multiple design options without running a full simulation each time. This acceleration in the design loop helps identify optimal solutions sooner and allows designers to explore more complex variations that would have been impractical to evaluate previously.
4. Adaptive Optical System Control
Using reinforcement learning and adaptive algorithms, optical systems can adjust elements like deformable mirrors and tunable lenses on the fly to maintain optimal focus and compensate for environmental fluctuations.
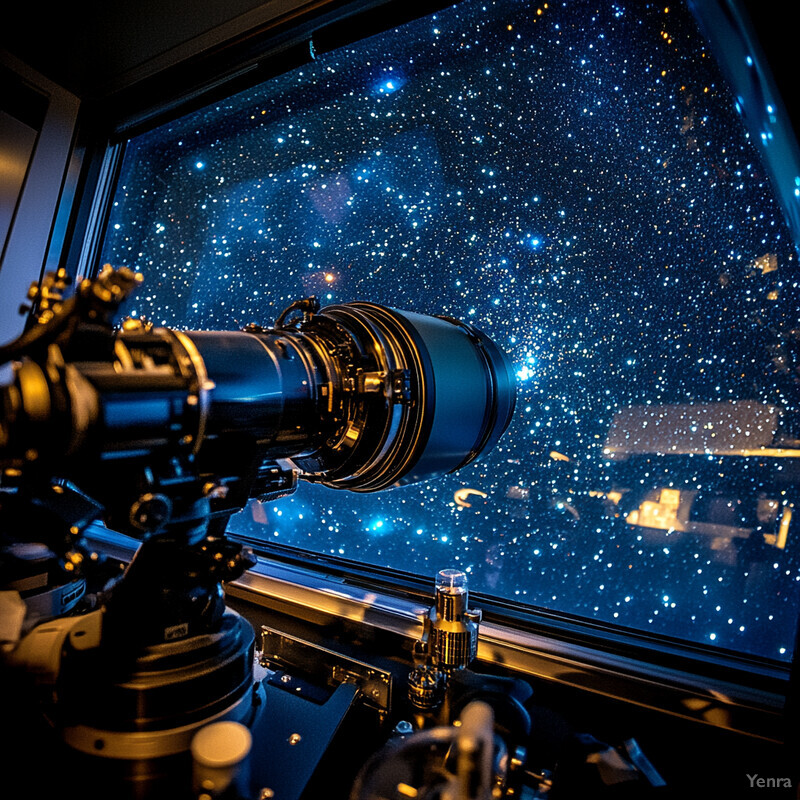
Adaptive optics has traditionally relied on feedback control loops that adjust elements like deformable mirrors or liquid crystal modulators to correct wavefront distortions. AI takes this a step further by implementing reinforcement learning or advanced control strategies that dynamically respond to changing environmental conditions. Rather than following a set of predefined rules, the system continuously learns the best control actions to maintain desired performance. This can be particularly valuable in environments prone to fluctuations—such as atmospheric turbulence in astronomical telescopes or vibration in industrial laser systems—ensuring stable and high-quality outputs. AI-driven adaptivity reduces the need for human intervention and improves the reliability and precision of optical systems used in research, imaging, and communication applications.
5. Machine Learning-Assisted Material Selection
By analyzing large datasets of material properties, AI can help identify or design new optical materials with ideal refractive indices, dispersion characteristics, and durability.
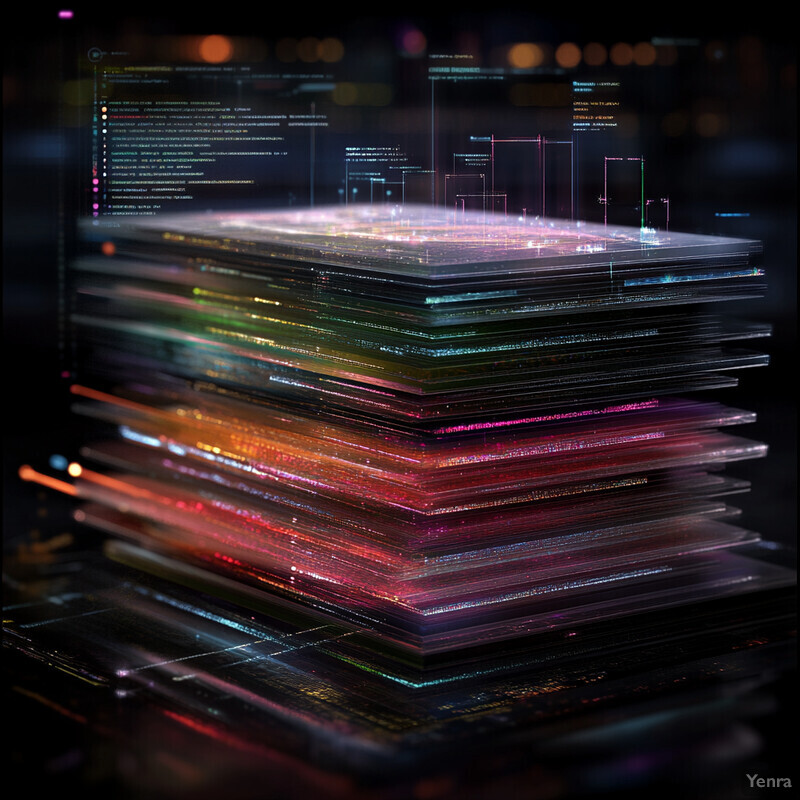
Selecting the right optical material is crucial for achieving desired properties like the correct refractive index, minimal dispersion, and high transmittance. With AI, designers can now sift through vast libraries of known and novel materials, correlating their chemical composition with optical characteristics. By training on experimental and simulation data, machine learning models can predict the performance of materials under different wavelengths, temperatures, and manufacturing conditions. These insights help identify not only optimal materials but also opportunities to synthesize new ones with tailored optical properties. The outcome is an accelerated path from concept to component, enabling more efficient, resilient, and cutting-edge optical devices.
6. Automated Tolerance Analysis
AI can quickly perform tolerance analyses, determining how imperfections in components or slight deviations from design specs affect overall performance.
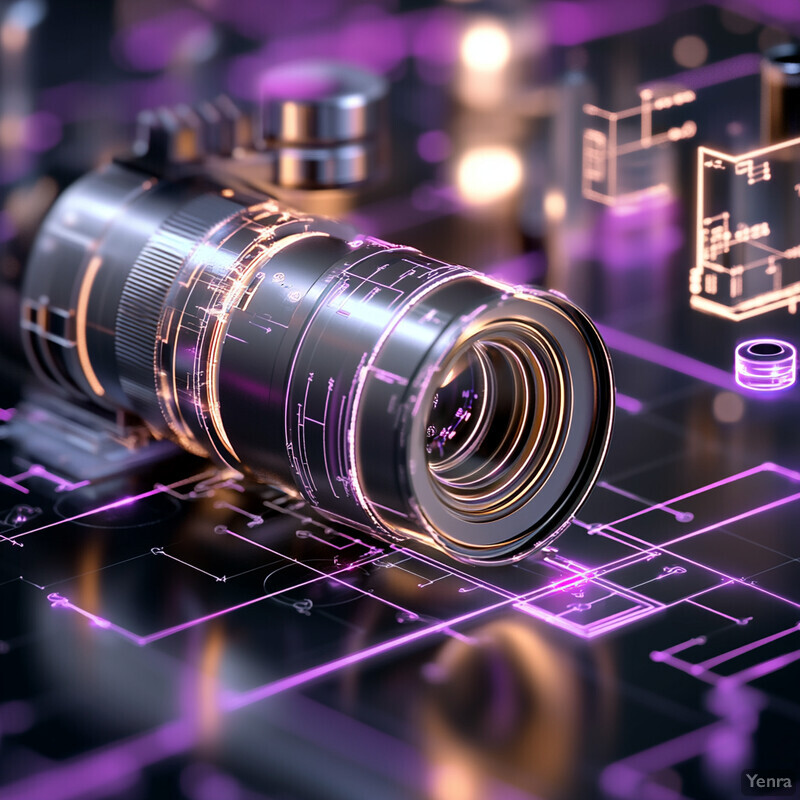
Tolerance analysis determines how sensitive an optical design is to small variations in manufacturing or assembly. Traditionally, engineers run numerous simulations adjusting parameters within specified tolerances to see how performance changes. AI-driven approaches can streamline this by using predictive models that quickly map tolerance ranges to performance outcomes. Engineers can thus identify critical tolerances early and guide manufacturing processes to prioritize the most impactful specifications. This results in more robust and practical designs, as well as reduced waste and rework. Over time, AI-based tolerance analysis contributes to higher product reliability and overall cost savings.
7. Metasurface and Metamaterial Design
For cutting-edge devices like meta-lenses, AI can handle the vast parameter space of nano-structures, identifying configurations that achieve desired phase shifts, polarization control, or diffraction properties.
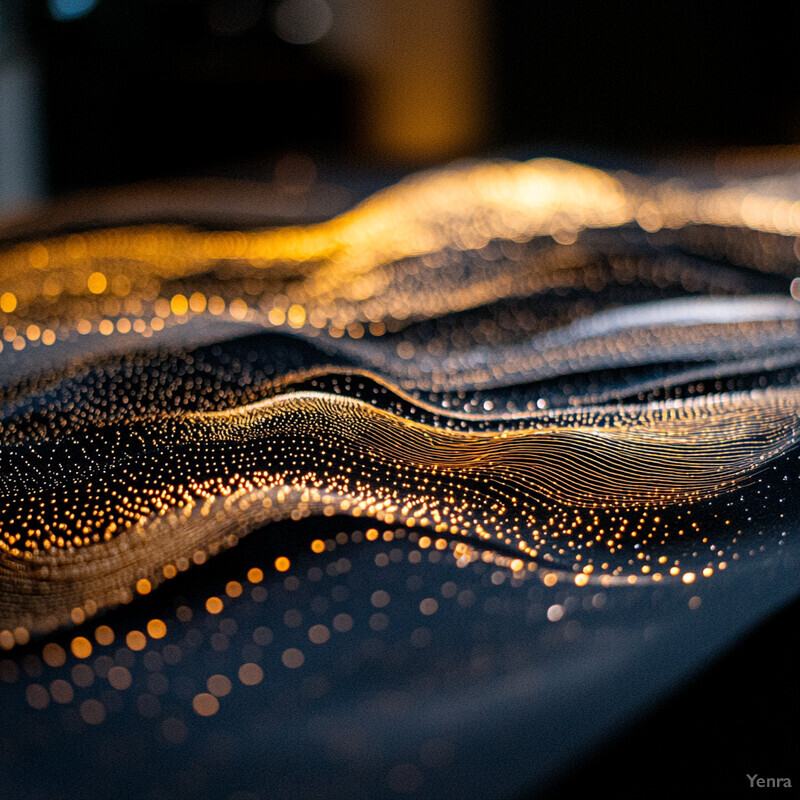
Metasurfaces—ultra-thin structured layers that manipulate light in novel ways—promise breakthroughs in lens miniaturization, beam shaping, and polarization control. Designing these intricate nanostructures involves navigating a vast parameter space, including shape, size, periodicity, and material composition. AI excels at exploring such high-dimensional spaces, using optimization methods and neural networks to identify configurations that produce desired phase shifts or resonances. By training on simulations and even experimental data, AI can rapidly home in on optimal metasurface patterns that would be hard to conceive manually. This accelerates the development of innovative optical components like flat lenses, holographic elements, and efficient couplers for integrated photonics.
8. Lens Coating and Filter Optimization
Deep learning models can suggest optimal multilayer coatings and spectral filters, providing higher optical efficiency, reduced glare, and improved contrast under varying lighting conditions.
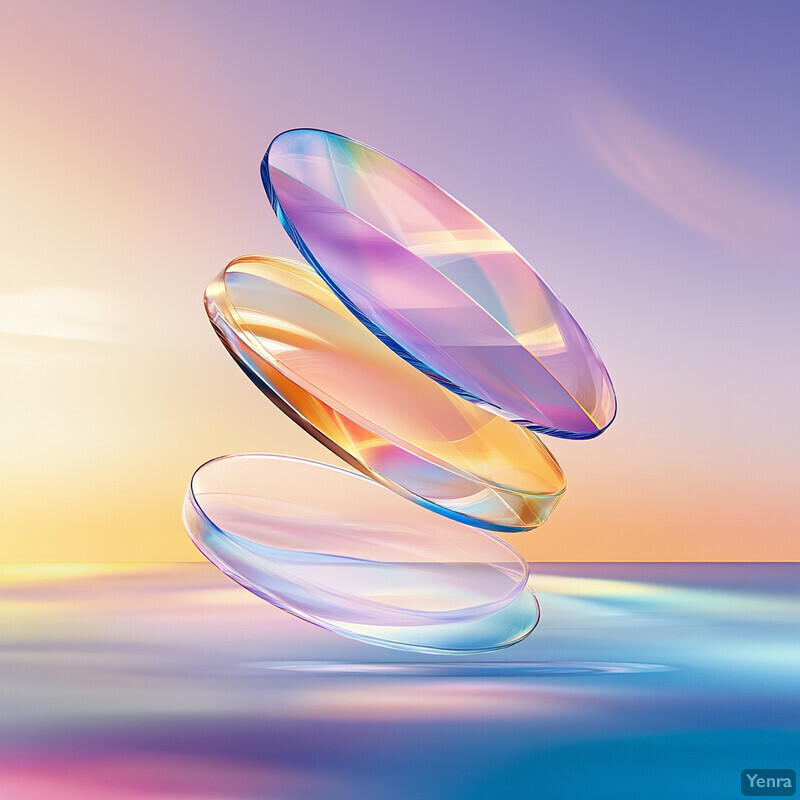
Coatings and filters are essential for controlling reflection, transmission, and absorption at specific wavelengths. Designing multi-layer coatings or thin-film filters that meet stringent optical requirements can be complex. AI-driven optimization can consider numerous variables—layer thicknesses, material indices, deposition order—and predict how these changes affect spectral performance. By systematically narrowing down the solution space, AI helps engineers identify combinations that yield improved efficiency, reduced glare, or sharper spectral cut-offs. This streamlines the iterative process of filter development and ensures more consistent, high-quality optical components in fields like photography, lidar, and spectrometry.
9. Predictive Maintenance and Quality Control
AI can analyze sensor and test data during manufacturing and assembly to predict defects or alignments issues, enhancing quality control and reducing production scrap rates.
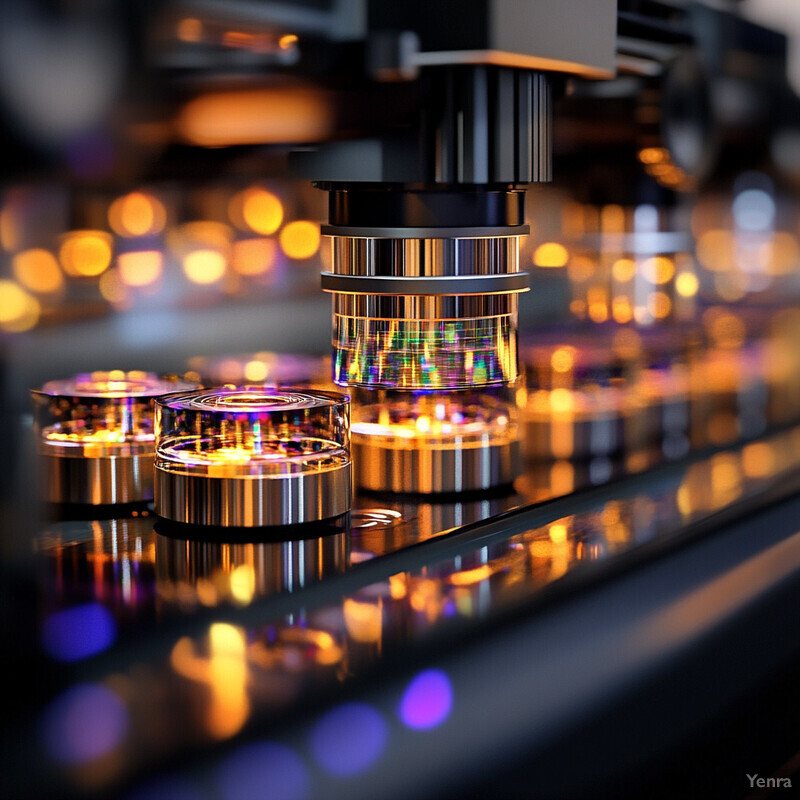
In manufacturing and assembling optical systems, even minor component misalignments or surface defects can degrade performance. AI-based inspection systems can analyze data from sensors, cameras, and testing equipment to detect subtle anomalies during production. By comparing measured parameters to a learned baseline, these models can predict when a component might fail or need recalibration before it happens. This predictive maintenance reduces downtime, minimizes material waste, and ensures that only top-quality systems leave the production line. Such improvements are critical in high-volume manufacturing or sensitive applications, ensuring reliability, safety, and adherence to performance standards.
10. Enhanced Beam Shaping and Wavefront Engineering
By learning from simulation and experimental data, AI can design freeform optics and wavefront-shaping elements that yield precisely controlled beam profiles.
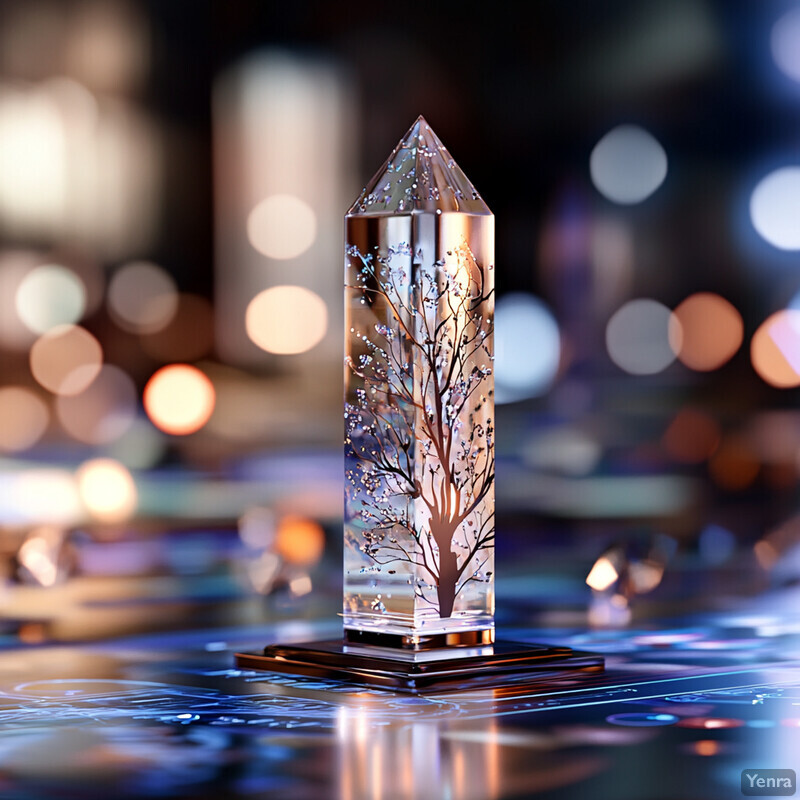
Controlling the shape of laser beams or other light fields is central to many applications—from high-resolution microscopy to advanced laser machining. AI can assist by learning from simulation and experimental data, helping to design optical elements (like holographic plates or diffractive gratings) that produce desired beam profiles. Unlike traditional approaches that rely on analytical solutions or repetitive experimentation, AI-driven techniques can rapidly evaluate numerous configurations. This leads to custom-tailored solutions, such as beams with topological charge for optical tweezers or shaped pulses for precision material processing. Ultimately, AI enhances flexibility, efficiency, and accuracy in beam shaping tasks.
11. Computational Imaging and End-to-End System Design
Integrating AI into the image formation pipeline allows the joint optimization of optics and post-processing algorithms, leading to high-quality images with simpler lens designs.
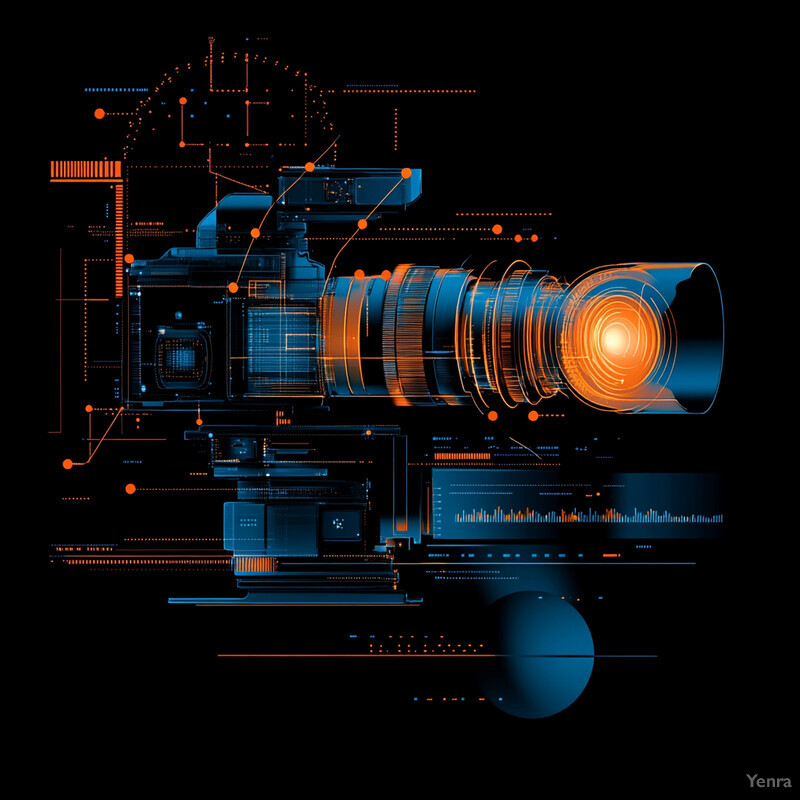
Optical design often focuses solely on hardware, but computational imaging integrates optics with digital post-processing algorithms. AI allows for joint optimization of these elements—designing lenses that, when combined with AI-driven image reconstruction, produce superior results with simpler optics. For example, a camera might use a less complex lens if the downstream AI algorithm can correct its aberrations. This co-design approach reduces hardware costs, lightens system weight, and enhances performance under challenging conditions. It’s transforming fields like consumer photography, medical imaging, and remote sensing by enabling super-resolution, extended depth of field, and improved low-light performance without overly complex hardware.
12. Photonics Circuit Layout and Integration
AI-guided design of integrated photonic circuits helps compress complex optical functions into smaller footprints and improve signal integrity.
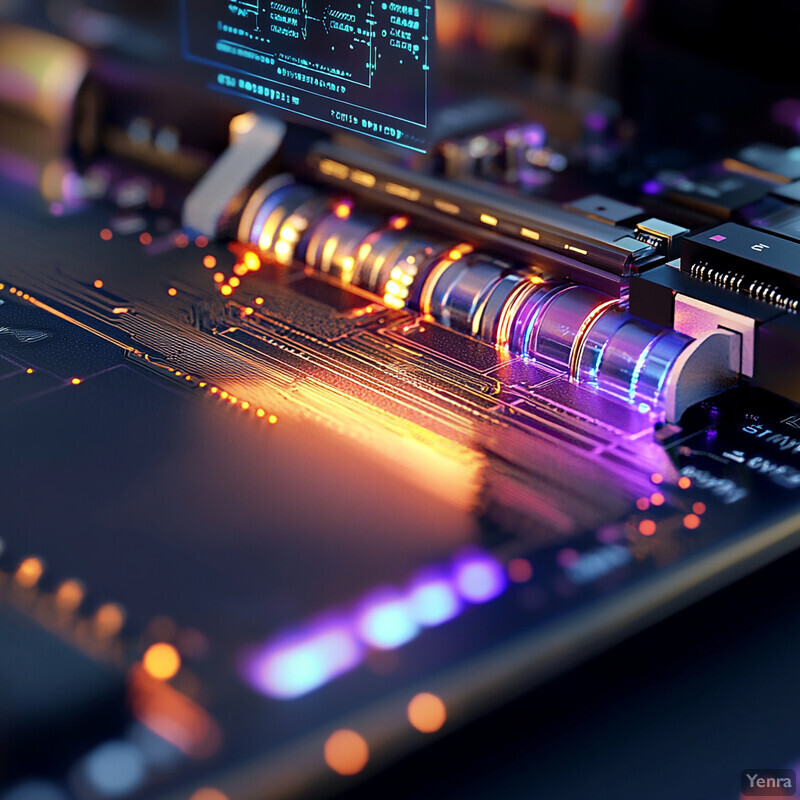
As photonic integrated circuits (PICs) grow more complex, finding optimal layouts for waveguides, couplers, and resonators becomes increasingly challenging. AI-based design tools can handle these intricate constraints, negotiating trade-offs between footprint, loss, bandwidth, and fabrication limits. Machine learning models can suggest compact and efficient on-chip optical routing architectures that minimize crosstalk and improve signal fidelity. This aids the development of advanced photonic chips for data centers, quantum computing, and biosensing. By streamlining PIC layout and integration, AI helps bring optical computing and high-speed optical networks closer to widespread commercial reality.
13. Nonlinear and Diffractive Optical Element Design
AI methods can handle complex nonlinear interactions and diffraction-limited conditions, guiding designers toward elements that break classical limitations or push performance boundaries.
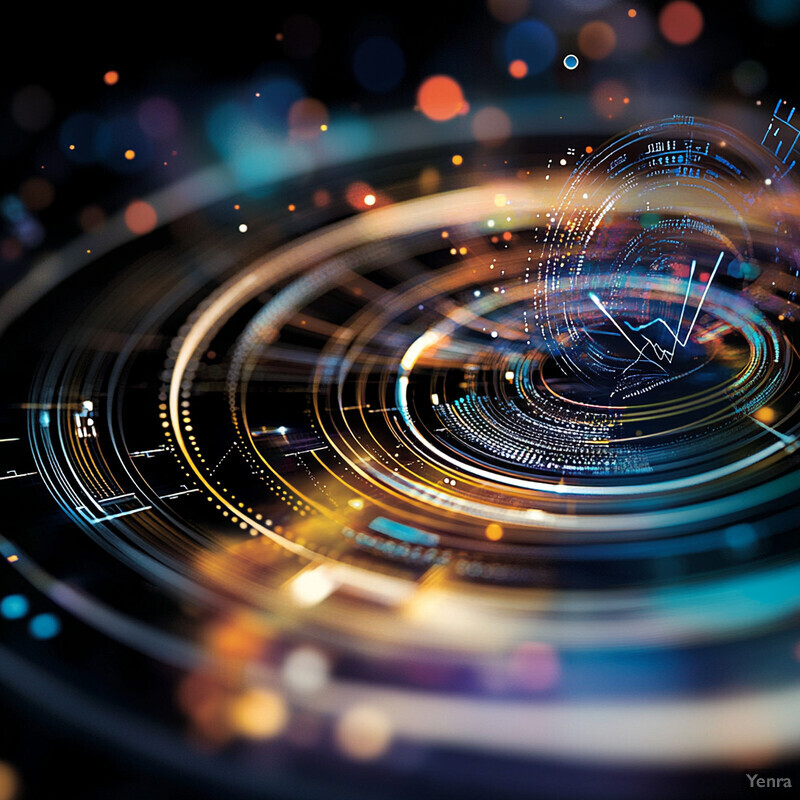
Nonlinear and diffractive optical elements open doors to controlling light in more exotic ways—frequency conversion, supercontinuum generation, and high-resolution diffraction patterns. However, these effects are often difficult to model or predict. AI-based optimization, trained on both simulations and experiments, can efficiently navigate the immense design space. It can identify non-intuitive parameter combinations that maximize efficiency or generate tailored diffraction patterns. Whether developing new frequency-doubling crystals for lasers or advanced diffraction gratings for spectrometers, AI’s capacity to handle complexity leads to superior, application-specific solutions.
14. Accelerated Monte Carlo Simulations
Instead of relying solely on brute-force Monte Carlo analyses, AI-based variance reduction techniques can produce accurate statistical performance estimates more efficiently.
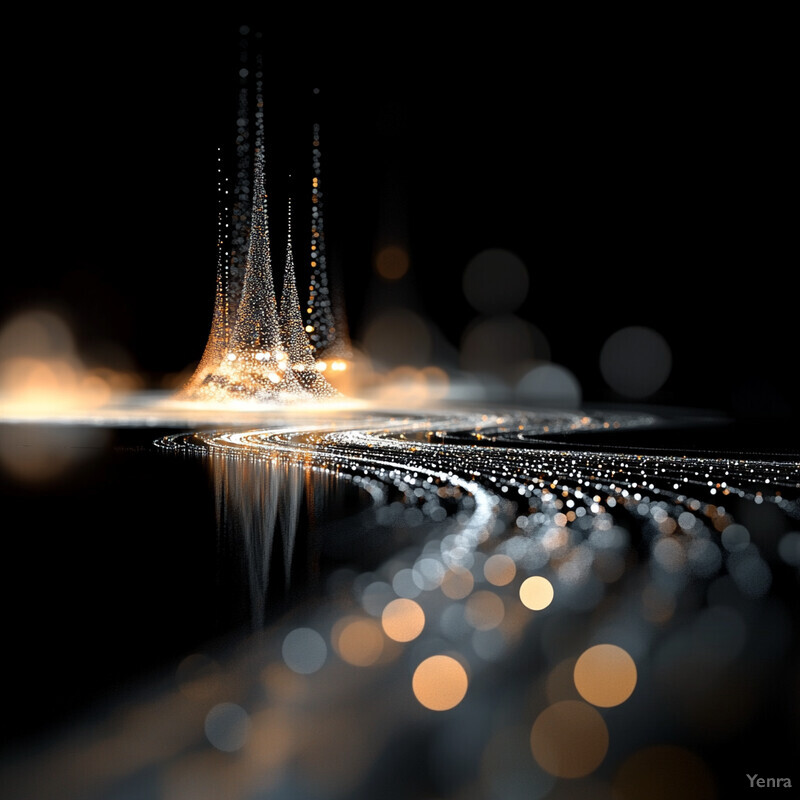
Monte Carlo simulations are widely used in optical design to statistically evaluate performance under real-world variability, including scattering and noise. These simulations can be computationally intensive. AI techniques can serve as variance reduction tools or surrogate models, enabling much faster approximation of the same statistical results. By replacing some portion of brute-force computations with learned patterns, engineers can quickly estimate performance distributions. This speedup allows for more robust risk assessments, thorough design-space exploration, and frequent iteration—improving confidence in the final design before it goes into production.
15. Real-Time Wavefront Sensing and Correction
Advanced machine learning algorithms can infer wavefront distortions from sensor data and rapidly compute correction settings for adaptive optical systems in real time.
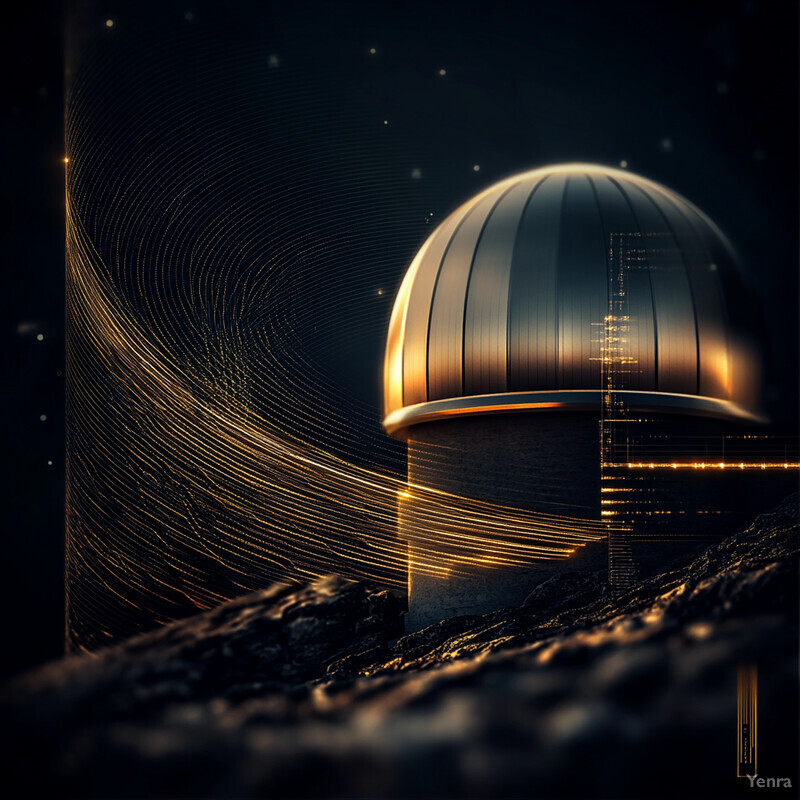
Wavefront distortions degrade image quality in telescopes, microscopes, and other high-precision systems. Traditional wavefront correction often relies on iterative processes that may not keep up with rapid changes in the environment. Machine learning models trained to infer wavefront errors from limited sensor data can instantly recommend correction settings. This enables real-time adjustments with minimal latency, improving the dynamic range and stability of adaptive optical systems. In astronomy, for instance, such real-time corrections yield sharper images of distant galaxies. In biomedical imaging, it enhances the clarity of cells deep within tissue samples, advancing research and diagnostics.
16. Robustness to Environmental Changes
AI can identify how temperature, vibration, and humidity shifts affect optical performance, then proactively adjust system parameters or suggest design changes.
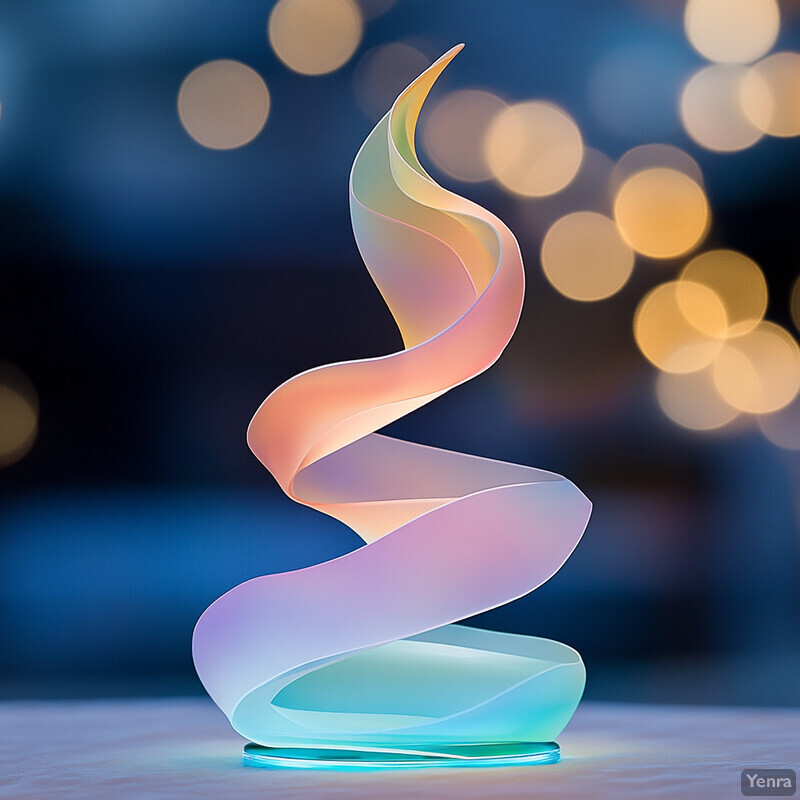
Optical systems often must operate under fluctuating conditions, including temperature variations, vibrations, and humidity changes. AI can help predict how these conditions affect performance and guide the system to compensate accordingly—either through adaptive control of optical elements or by recommending design modifications that inherently tolerate such variations. This proactive approach extends the operational lifetime and improves reliability for sensitive instrumentation, such as surgical endoscopes, lidar sensors in autonomous vehicles, or satellite-based imaging systems. By learning from environmental data, the system effectively becomes more resilient and consistent, even in challenging field conditions.
17. Data-Driven Aberration Correction
Instead of using standard polynomial fittings, neural networks can directly learn and correct intricate aberration patterns, leading to sharper images or beams.
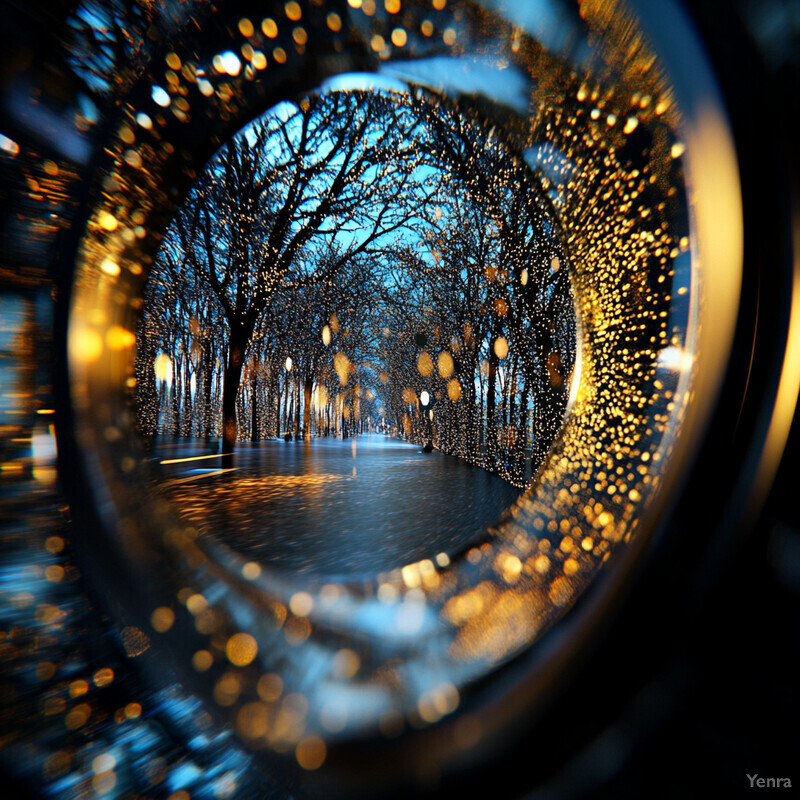
Aberrations, such as spherical or chromatic distortions, have historically been corrected using known mathematical expansions or iterative fine-tuning. AI-based methods now learn directly from raw data—images captured by the optical system—to identify and correct complex aberrations. This data-driven approach can achieve superior correction performance because it is not constrained by classical aberration models. It can handle unusual or application-specific distortion patterns, yielding higher-quality images for applications like microscopy, lithography, or surveillance. As the model continuously refines its understanding, it can provide near real-time correction, reducing the need for elaborate calibration procedures.
18. High-Dimensional Design Space Exploration
AI can handle parameter spaces with dozens or hundreds of variables—apertures, curvatures, distances, materials—efficiently converging on high-performance solutions.
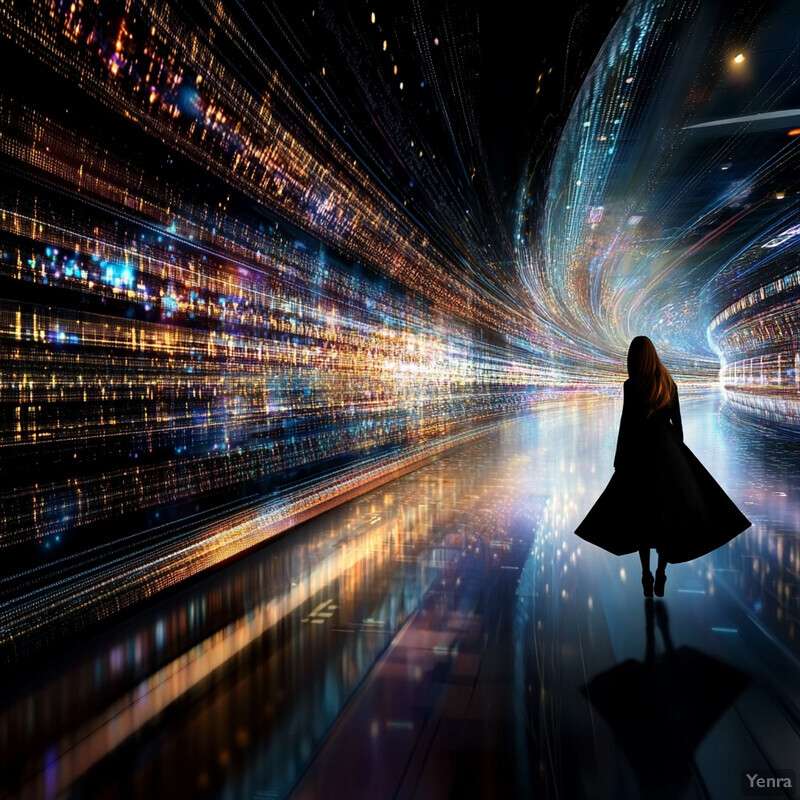
As optical systems become more intricate—with multiple lenses, aspherical surfaces, coatings, diffractive elements, and nonlinear materials—designers face an enormous parameter space. AI excels at searching these high-dimensional spaces efficiently, identifying combinations that yield optimal performance metrics, such as resolution, field-of-view, or efficiency. The process involves iterative optimization, active learning, or Bayesian methods to make informed decisions about where to sample next. By making sense of high-dimensional complexity, AI empowers designers to discover entirely new configurations that were previously overlooked, leading to breakthroughs in compactness, performance, and cost-effectiveness.
19. Generative Models for Rapid Prototyping
Generative adversarial networks can propose entirely new classes of optical layouts, stimulating innovation and non-intuitive solutions.
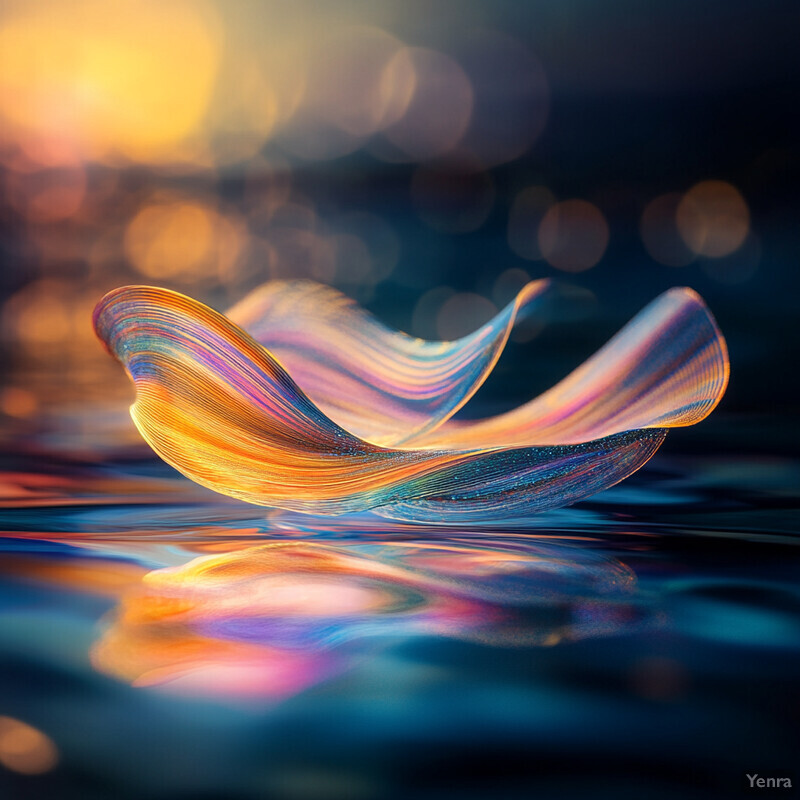
Generative Adversarial Networks (GANs) and other generative models can create candidate optical designs from scratch. By learning the statistical properties of successful designs, these models can propose entirely new configurations that human experts might never consider. This can jump-start creativity and innovation, providing a starting point for further refinement or validation. Rapid prototyping with AI-generated concepts helps explore more options in less time, accelerating the cycle of invention. The end result could be novel freeform optics, custom waveguides, or unique filter stacks that distinguish cutting-edge products from conventional solutions.
20. Holistic End-to-End System Co-Design
By simultaneously considering optical elements, mechanical housings, illumination sources, and sensors, AI can optimize the entire optical system as a single unit.
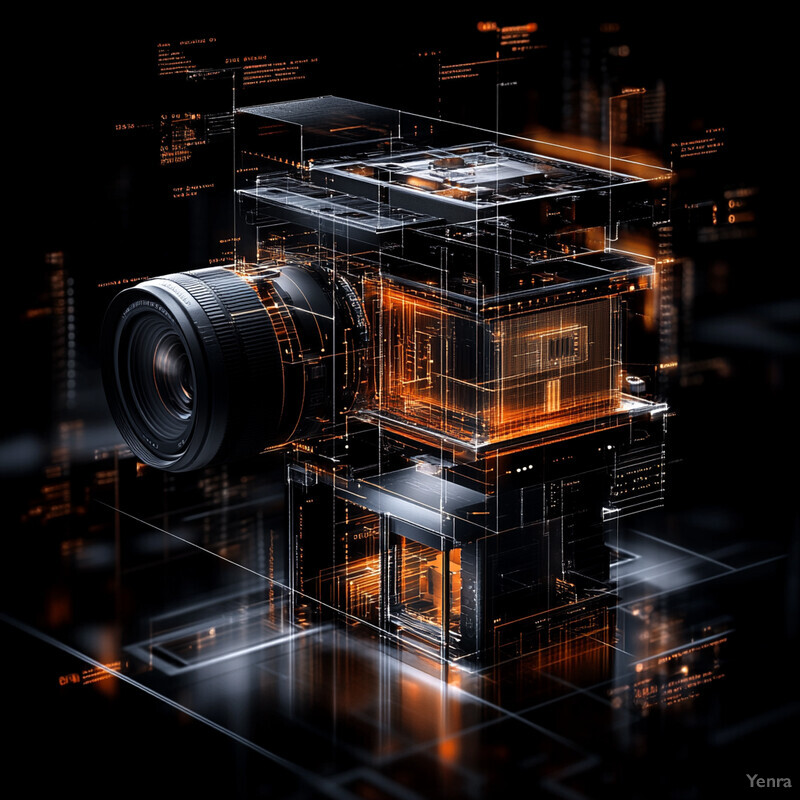
Traditionally, optical design focuses on individual components—lenses, mirrors, coatings—without fully integrating mechanical, electronic, and algorithmic elements. AI enables an end-to-end design approach, simultaneously optimizing the full stack that includes illumination sources, sensor arrays, optical elements, mechanical housings, and the post-processing algorithms. By considering all aspects collectively, the system can find global optima, achieving unprecedented performance. For example, a camera might balance lens complexity with powerful computational algorithms to reduce cost and size while improving image quality. This holistic approach delivers synergy among all subsystems, resulting in more efficient, compact, and high-performance optical solutions poised to excel in a variety of advanced applications.