1. Enhanced Fetal Fraction Estimation
AI-driven algorithms improve the accuracy of measuring the fetal fraction (the percentage of fetal DNA in maternal blood) during non-invasive prenatal testing. Traditional methods for fetal fraction estimation can be influenced by maternal factors and technical variability, sometimes yielding inconclusive results. Machine learning models can learn complex patterns from large genomic datasets, enabling more precise fetal fraction calculations even at lower fetal DNA levels. By flagging borderline cases and guiding re-testing when needed, AI helps ensure tests have sufficient fetal DNA to be reliable. Overall, enhancing fetal fraction estimation with AI reduces test failures and increases the sensitivity and specificity of NIPT results.
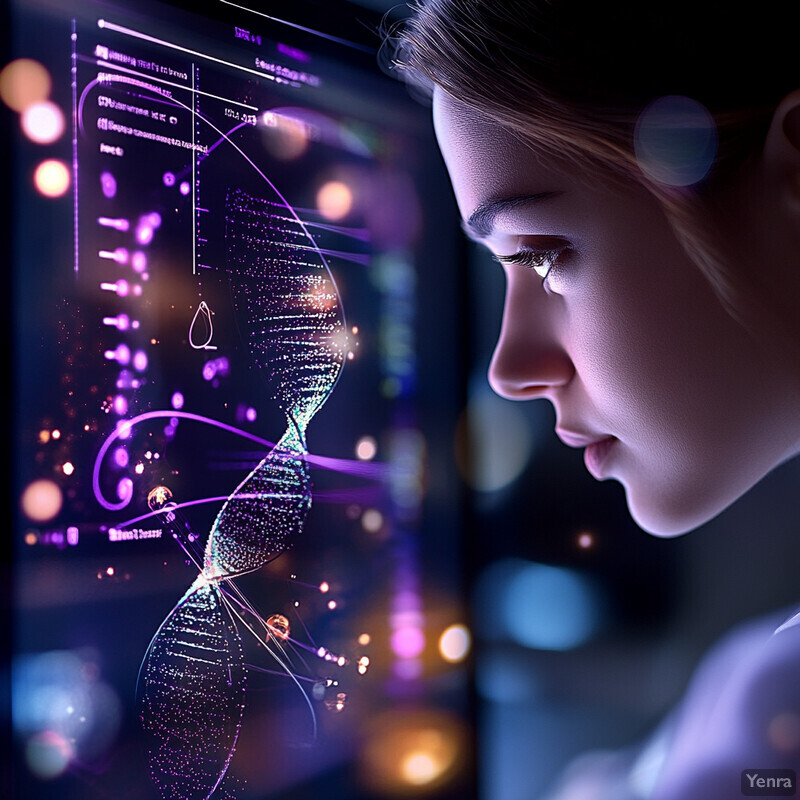
Emerging research shows that AI-based methods can markedly improve fetal fraction analysis. For instance, a deep learning approach (“PREFACE”) outperformed traditional regression models, achieving a Pearson correlation up to ~0.94 between predicted and actual fetal DNA fraction. Studies also highlight variability in fetal fraction reporting across different laboratories globally, underlining the need for standardized, intelligent estimation methods. By training on tens of thousands of pregnancies, one elastic net model reached greater than 0.90 correlation in fetal fraction prediction on validation data. This robustness allows AI systems to reliably detect low fetal fractions (e.g. ~3%–4%), which are typically considered borderline for test quality. In practice, implementing AI-driven fetal fraction estimators can reduce “no-call” rates and ensure that NIPT results are based on sufficient fetal DNA, bolstering confidence in the test’s accuracy.
2. Sophisticated Variant Calling
AI enables more sophisticated variant calling in NIPT, detecting genetic abnormalities beyond the most common trisomies. Traditional bioinformatics pipelines can struggle to find subtle DNA variations in the low-level fetal DNA mixed with maternal DNA. Machine learning models – including deep neural networks and advanced statistical filters – help tease out true fetal genetic signals from sequencing noise. These AI-driven callers can identify smaller copy-number variants, microdeletions, or single-gene mutations that older methods might miss. By improving the signal-to-noise ratio, AI-based variant calling expands NIPT’s scope to a wider range of genetic conditions with greater accuracy.
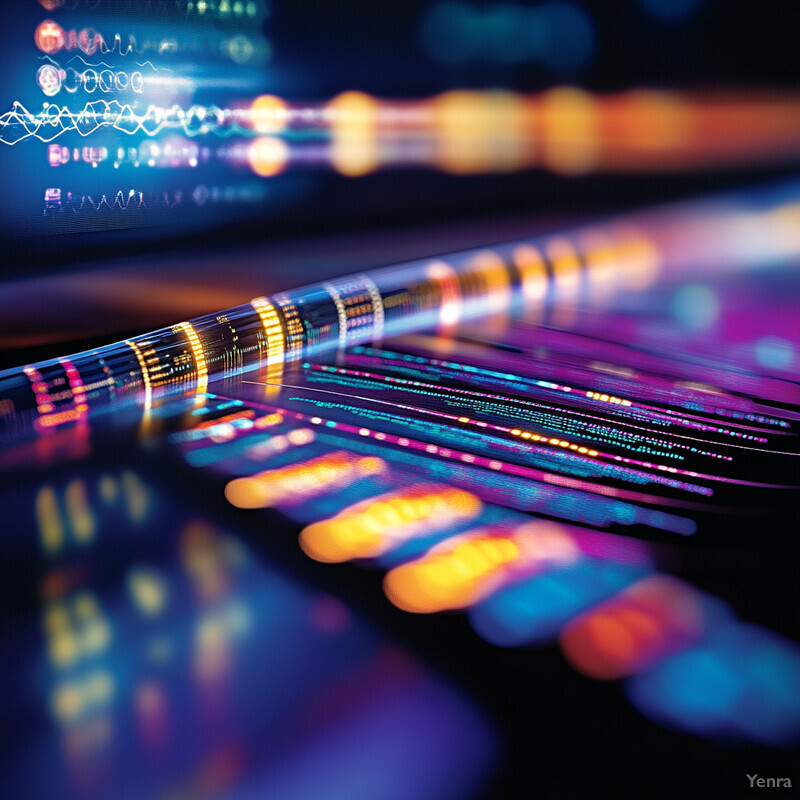
Recent studies demonstrate AI’s power to enhance variant detection in prenatal testing. In 2023, researchers noninvasively screened the entire fetal exome by combining deep sequencing with machine-learning pipelines, successfully identifying de novo single-gene mutations in fetuses. One team used a machine learning model to integrate fetal fraction and read-depth features, aiding detection of copy-number variants (CNVs) that were previously challenging to discern. In a 2024 report, a self-learning neural network was able to predict fetal single-nucleotide variants (SNVs) causing monogenic diseases with up to ~95% genotype accuracy in validation tests. Similarly, back-to-back reports in NEJM (November 2023) showed that high-resolution NIPT using deep exome sequencing and AI could reliably pinpoint a broad range of fetal genetic disorders beyond aneuploidies. These advances illustrate how AI-refined variant calling is transforming NIPT into a more comprehensive genomic screening tool, catching nuanced mutations while maintaining low false-positive rates.
3. Multi-Modal Data Integration
AI facilitates the integration of multiple data modalities in prenatal screening, combining genetic information with other clinical and imaging data. By analyzing cell-free DNA results alongside maternal health records, ultrasound measurements, and serum biomarkers, machine learning models can generate a more holistic risk assessment for fetal conditions. This multi-modal approach provides a comprehensive picture – for example, correlating subtle ultrasound findings with genomic signals to improve screening accuracy. Integrating diverse data sources can personalize risk predictions to the individual patient’s profile. In essence, AI-driven multi-modal integration promises to enhance NIPT by contextualizing genetic findings within broader clinical data, leading to more informed and tailored prenatal care.
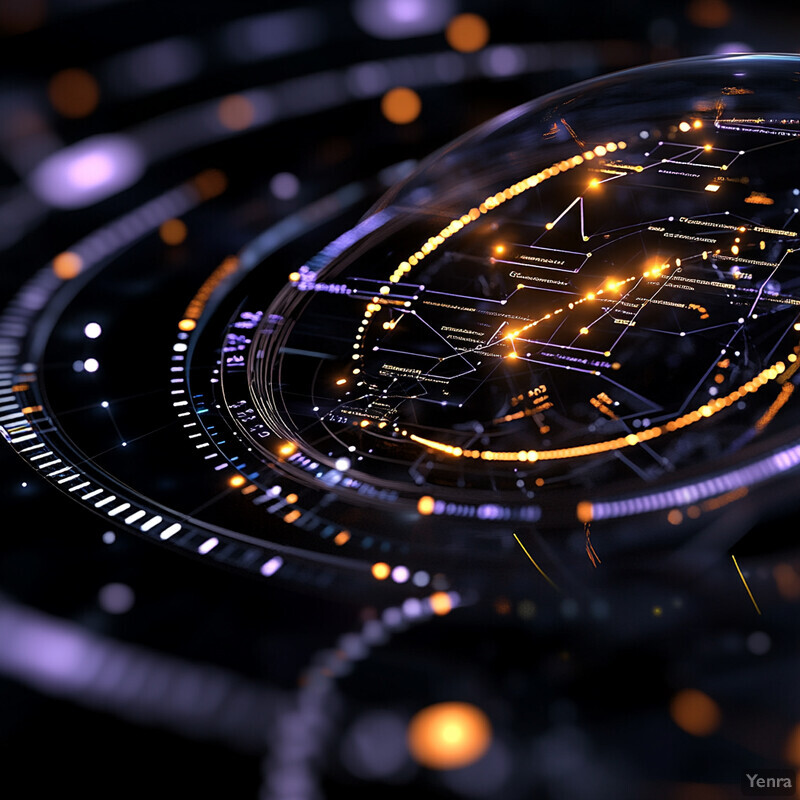
Research shows that combining NIPT with other prenatal data can significantly improve diagnostic performance. A 2025 review reported that machine learning models integrating maternal serum markers, ultrasound nuchal translucency, and cell-free DNA data achieved detection rates above 85% for Down syndrome while keeping false positives low. In practice, this means AI can merge ultrasound image features and blood biomarkers with genomic screening to catch cases that might slip by using one modality alone. Studies also suggest that incorporating maternal factors and sonographic “soft markers” alongside NIPT increases sensitivity and specificity for aneuploidy detection. One 2024 study developed an ML-based Down syndrome screening pipeline that learned from population data and various biomarkers, reporting improved accuracy across diverse patient groups. Such findings underscore AI’s role in multi-modal prenatal testing: by learning from the full spectrum of available data (genetic, biochemical, and imaging), AI systems can deliver more robust and equitable screening results for all populations.
4. Reduction in False Positives/Negatives
AI is helping reduce false positives and false negatives in NIPT by better distinguishing true fetal abnormalities from benign noise. Machine learning models can recognize patterns that indicate whether a DNA aberration is real or an artifact (for example, caused by a maternal variant or technical error). This refined analysis decreases the likelihood of “false alarms” (false positives) that can cause unnecessary anxiety and follow-up invasive tests. At the same time, AI improves detection sensitivity, catching subtle genomic signals and thereby lowering the chance of false negatives where a condition is initially missed. By minimizing both types of errors, AI-driven NIPT provides more reliable results and increases clinical trust in the screening.
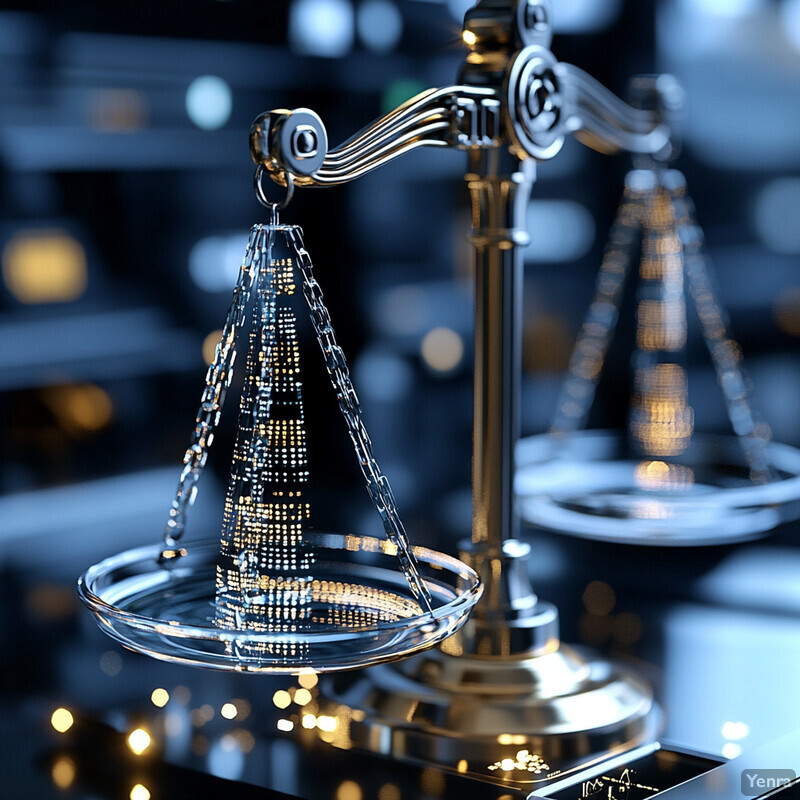
Evidence suggests that advanced algorithms can make NIPT results more accurate. AI-based analyses of large datasets have demonstrated high sensitivity for aneuploidy while maintaining very low false-positive rates. For example, modern NIPT platforms using deep learning report sensitivities ~99% for trisomy 21 with false-positive rates under 0.1%, a performance level unattainable by older screening methods alone. Integrating multiple features (genetic and clinical) via machine learning further helps filter out noise: one 2025 review notes that such models raise detection rates for Down syndrome above 85% without spiking the false-positive rate. Additionally, AI can learn to identify incidental maternal DNA findings (like benign copy-number variations in the mother) that might otherwise trigger false-positive calls. By accounting for these confounding factors, AI-driven NIPT pipelines decrease the incidence of both false positives and false negatives, ensuring that high-risk calls truly reflect fetal conditions and normal results are indeed reassuring.
5. Population-Specific Risk Modeling
AI enables the customization of NIPT risk algorithms to specific populations, improving accuracy across diverse ethnic and regional groups. Genetic variation and background DNA differences can affect test performance from one population to another. Machine learning models can be trained on local population data – including common genetic polymorphisms – to calibrate risk predictions appropriately. This means the NIPT algorithm “learns” the genomic baseline of a given population, reducing biases or errors that might occur if using one-size-fits-all parameters. With continuous learning from population-specific data, AI-driven NIPT provides more equitable screening, offering consistent reliability whether the patient’s ancestry is, for example, European, Asian, African, or otherwise.
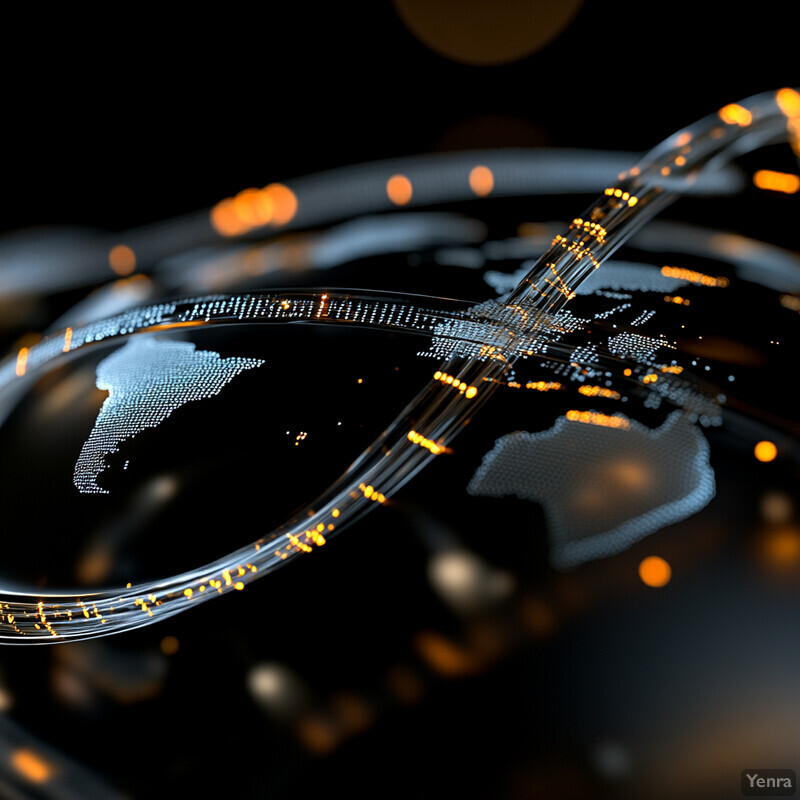
Recent advancements highlight how incorporating population genomics improves NIPT accuracy. In 2024, researchers introduced an AI framework called NIPT-PG that uses an incremental “pan-genome” model, integrating variant and polymorphism data from the tested population. By aligning sequencing reads to a graph of population-specific genetic loci, this approach overcame reference genome biases and achieved more accurate z-scores for aneuploidy detection. In trials on 745 real-world cases, the population-trained NIPT-PG algorithm outperformed the standard method, demonstrating improved detection of trisomies while using the same sequencing depth. Additionally, a global survey in 2023 found significant variability in fetal fraction estimates reported by different NIPT labs, underscoring the importance of regional calibration. Together, these findings show that AI models which learn from local genomic data can adapt NIPT to diverse populations – ensuring that predictive risk calculations remain accurate and valid for all patient groups, not just the ones represented in the original test development.
6. Automated Quality Control
AI can automate quality control (QC) in NIPT laboratories by continuously monitoring sample and sequencing metrics. Intelligent systems are capable of flagging samples with potential issues – such as inadequate DNA concentration, high maternal blood cell contamination, or low read quality – earlier and more reliably than manual checks. Machine learning algorithms can learn the normal ranges and patterns of run data, detecting anomalies in real time (for example, if a sequencing run’s quality scores dip below acceptable thresholds). By catching these problems immediately, AI-driven QC reduces the need for repeat testing and ensures that only high-quality results are reported. This automation streamlines lab workflows, maintains testing standards, and provides detailed logs for auditing and regulatory compliance.
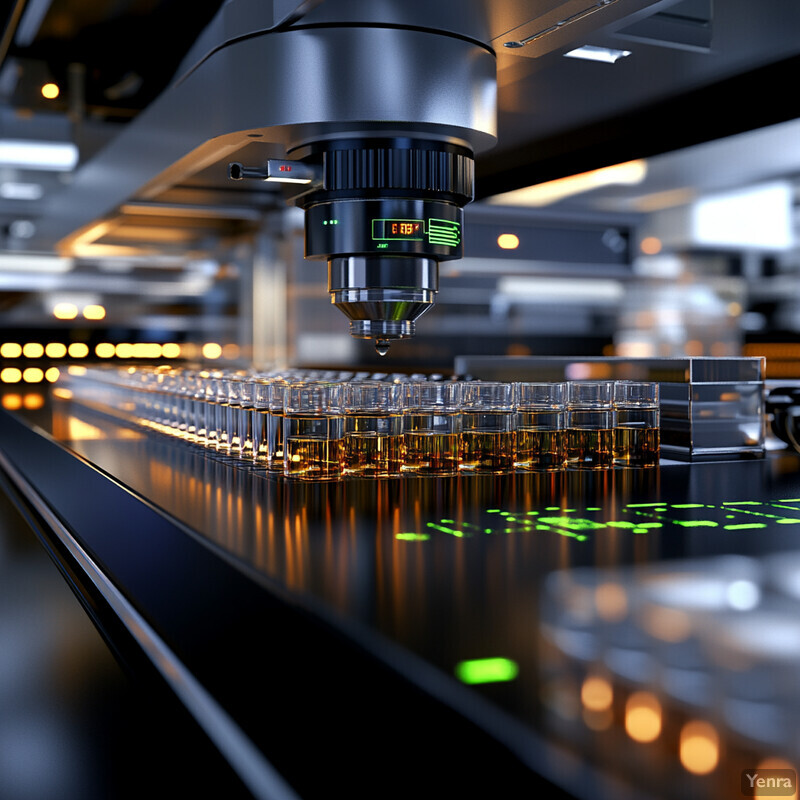
Implementing automated QC has tangible benefits in NIPT processing. A 2024 national study detailed stringent QC measures for NIPT, including minimum DNA concentrations (≥8 ng/µL) and sequencing quality criteria (e.g. ≥10 million reads per sample with ≥85% of bases at Q30 or higher). AI systems can track such metrics across each run and sample, instantly alerting operators if any parameter (like read depth or fragment size profile) falls outside the acceptable range. For instance, one report noted that samples with fetal fraction below ~4% are typically QC failures; an AI can preemptively identify these borderline cases and recommend a redraw before analysis proceeds. Automated pipelines also record each step’s parameters, creating an audit trail by default. In practice, labs using AI-driven QC have seen fewer failed or unreportable NIPT results, as issues like sample mix-ups or instrument errors are caught early. This leads to more efficient operations and consistent adherence to quality standards without solely relying on human oversight.
7. Rapid Turnaround Times
AI-powered NIPT pipelines can significantly speed up the time it takes to go from blood sample to test result. By automating data analysis and interpretation, machine learning systems reduce the bottlenecks that traditionally required manual review. For example, AI algorithms can process sequencing data and generate reports in a matter of hours, whereas older workflows might have taken days. Additionally, intelligent systems triage samples and optimize lab processes (such as multiplexing and scheduling) to maximize efficiency. The result is a shorter turnaround time for NIPT – often shrinking from about one week to just a few days – which in turn helps expectant parents and clinicians make timely decisions with crucial genetic information.
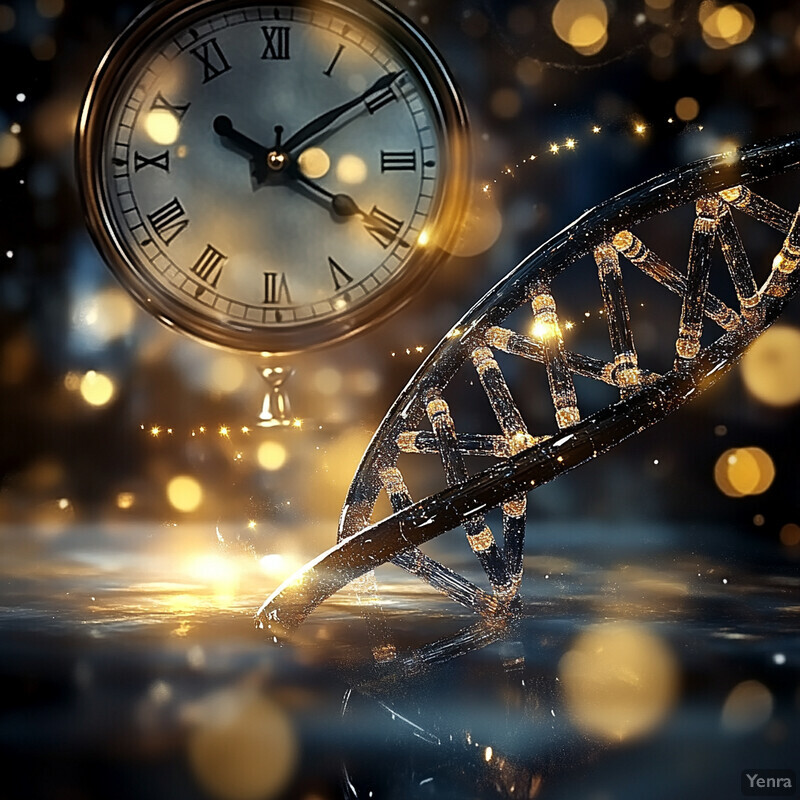
Although turnaround time depends on many factors, AI-driven improvements are beginning to accelerate the NIPT process. Researchers have noted the potential for “rapid” AI analysis in prenatal genomics, emphasizing that advanced algorithms can swiftly detect fetal anomalies once sequencing data is available translational-medicine.biomedcentral.com . In practice, some modern NIPT services (leveraging automation and AI) now report results in ~3–5 days, compared to the 7–10 days typical of earlier workflows. The acceleration comes from machine learning models streamlining steps like read alignment, aneuploidy calling, and report generation, which were partly manual or slower with legacy software. For instance, an AI-based pipeline can automatically interpret complex patterns without needing a human analyst to double-check every read, thereby saving considerable time. As laboratories continue to integrate AI, many expect prenatal screening to approach near-real-time analysis – enabling clinicians to receive NIPT results faster than ever and improving the prenatal decision-making timeline.
8. Feature Extraction from Sequencing Data
AI is adept at extracting subtle features from raw sequencing data that can enhance NIPT’s diagnostic power. Traditional NIPT mainly looks at read counts per chromosome, but advanced algorithms can delve deeper into the data – examining patterns like fragment lengths, fragment end motifs, GC-content biases, and other sequence context. Deep learning models, in particular, can automatically learn which combination of these features best distinguishes aneuploid or abnormal samples from normal ones. By leveraging these high-dimensional features, AI expands the range of conditions detectable by NIPT (including epigenetic or fragmentomic signals of disease) and improves the test’s overall accuracy. In short, AI unlocks information embedded in sequencing data that humans or simple models might overlook.
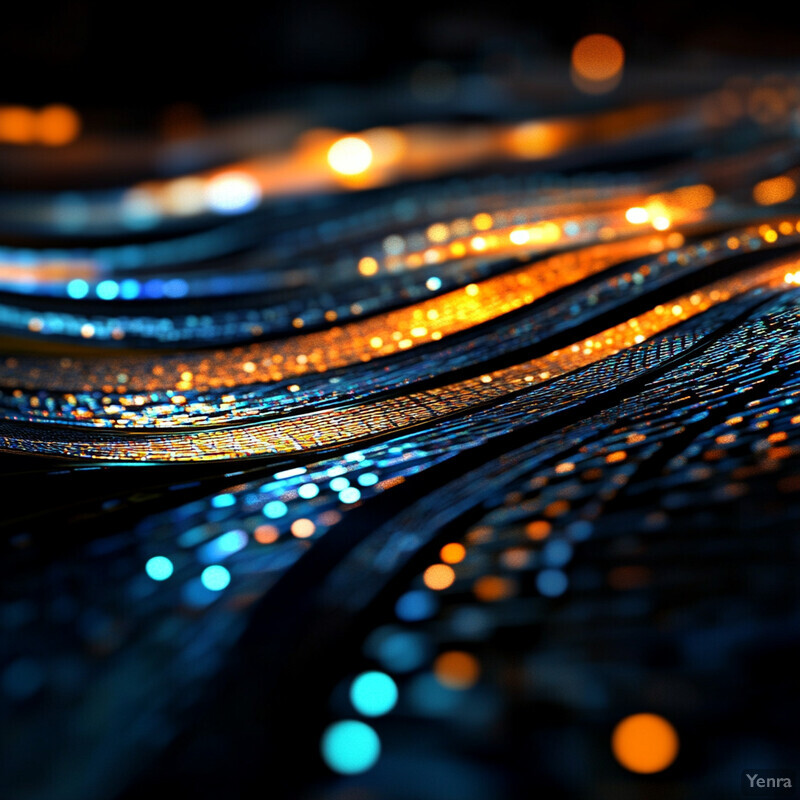
Studies have shown that machine learning can pull rich information from cfDNA sequencing beyond basic read counts. One 2024 NIPT study engineered 67 distinct features from cfDNA and parental genomes – ranging from haplotype-based metrics to coverage patterns – and used them as input to a neural network, which ultimately selected 56 of those features for optimal predictive performance translational-medicine.biomedcentral.com . Deep learning models also exploit fragmentomic patterns: researchers have observed that cfDNA fragment end sequences are not random but reflect nucleosomal footprints. AI algorithms can learn these end-motif frequencies and fragment size distributions to differentiate fetal-derived fragments from maternal background. In practice, an AI-enhanced pipeline might notice a subtle coverage dip in a specific genomic window or a slight shift in fragment length profile – clues that a conventional workflow might treat as noise. Indeed, an early application of such features was the SeqFF algorithm (now improved via deep learning), which uses dozens of coverage features across the genome to accurately infer fetal fraction. By incorporating comprehensive feature sets, AI-driven NIPT can detect nuances like microdeletions or single-gene disorders, and even glean epigenetic signatures, all from the same sequencing run.
9. Ultrasound Image Analysis Integration
AI enables integration of ultrasound imaging with NIPT to provide a more robust prenatal assessment. By analyzing fetal ultrasound images using computer vision techniques, AI can detect anatomical markers or subtle anomalies that correlate with genetic conditions. When combined with cell-free DNA results, these AI-analyzed ultrasound findings can improve screening accuracy. For instance, an AI might quantify nuchal translucency or identify facial markers of Down syndrome on an ultrasound and then incorporate that data into the overall risk calculation alongside NIPT. This synergy means that even if an ultrasound is borderline and NIPT is low-risk (or vice versa), the AI can reconcile the information for a clearer interpretation. Ultimately, adding AI-driven ultrasound analysis to NIPT brings together the strengths of imaging and genomics for more reliable and comprehensive prenatal screening.
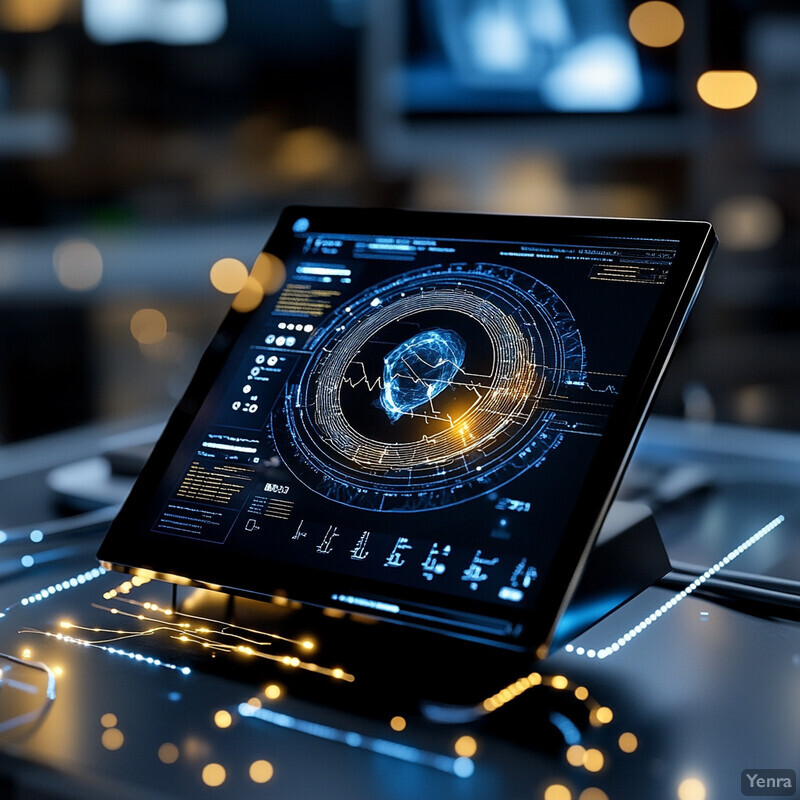
Early studies demonstrate the promise of AI in prenatal ultrasound analysis. A 2023 study applied a Swin-transformer deep learning model to second-trimester ultrasound images for Down syndrome detection, achieving high accuracy in flagging abnormal findings from the images alone. Another project in 2024 developed a deep learning architecture to assess first-trimester fetal ultrasound scans, successfully recognizing phenotypic traits indicative of Down syndrome that might be missed by the human eye. These AI-extracted imaging features – when used in conjunction with NIPT genetic data – enhance predictive performance. For example, machine learning models that incorporate ultrasound “soft markers” (like mild ventriculomegaly or certain facial measurements) alongside cfDNA screening have demonstrated improved sensitivity for aneuploidies compared to using NIPT alone. Clinically, this means an AI might analyze a routine 20-week scan, detect a subtle heart or profile anomaly, and alert that the negative NIPT result might warrant a second look. Such integrated approaches are still being refined, but they foreshadow a future where AI seamlessly merges imaging and genomic data to boost the overall detection rate of prenatal conditions.
10. Enhanced Predictive Analytics for Complex Disorders
AI is expanding NIPT beyond chromosomal anomalies to predict a broader spectrum of genetic disorders and pregnancy complications. Advanced predictive models can analyze subtle changes in cfDNA data to infer risk for conditions like single-gene (monogenic) diseases, rare microdeletion syndromes, or complex disorders that involve multiple genes. This goes hand-in-hand with integrating other biomarkers: AI might combine genetic signals with maternal factors to forecast issues like preeclampsia or preterm birth. By recognizing patterns associated with these complex outcomes, AI transforms NIPT from a test that screens mainly for a few trisomies into a platform that can flag many potential health concerns earlier in pregnancy. This enhanced predictive capability gives clinicians and families a head start in managing or further diagnosing a wider array of conditions.
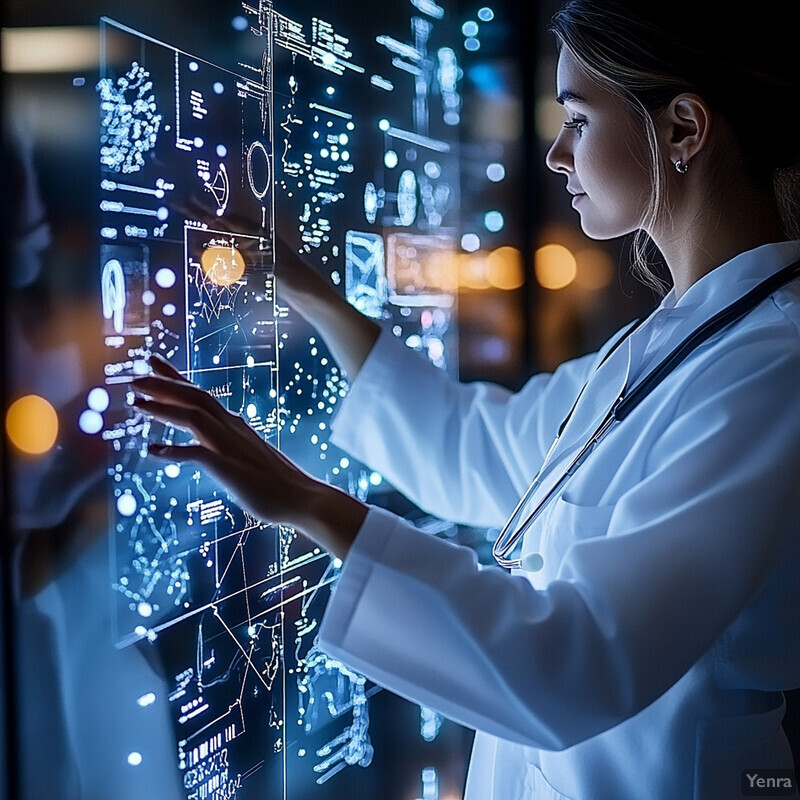
Cutting-edge studies illustrate AI’s role in broadening NIPT’s scope. In 2023, two separate teams reported the successful non-invasive detection of fetal single-gene disorders by sequencing the fetal exome and using machine learning analysis translational-medicine.biomedcentral.com translational-medicine.biomedcentral.com . These proof-of-concept cases identified monogenic mutations (for example, causing metabolic diseases) from maternal plasma – something not possible with standard NIPT protocols. Likewise, a 2024 neural network model was shown to predict fetal genotypes for inherited disorders with up to ~95% accuracy, even when fetal DNA fractions were low translational-medicine.biomedcentral.com . Beyond genetics, AI is linking NIPT data to complex outcomes: researchers have developed classifiers (using cfDNA fragment patterns and other inputs) that predict pregnancy complications like preeclampsia and preterm birth months before clinical onset. For instance, a large 2025 study introduced an SVM-based “PTerm” model that uses whole-genome cfDNA promoter profiles to forecast spontaneous preterm birth, achieving an AUC ~0.85 in independent validation. These advances underscore that AI-enhanced NIPT can serve as an early warning system for a variety of conditions – from rare genetic syndromes to obstetric complications – far beyond the traditional aneuploidy focus.
11. Adaptive Learning Over Time
AI models for NIPT have the ability to continuously improve over time as they are exposed to more data. In practice, this means that as thousands more pregnant individuals undergo NIPT, the machine learning algorithms can update themselves to reflect new patterns, rare variants, or shifts in population demographics. This adaptive learning ensures that the predictive performance of NIPT doesn’t stagnate – it actually gets better and more generalizable. Over time, the AI can incorporate emerging research (such as newly discovered genetic markers or refined understanding of cfDNA biology) into its predictions. This continuous refinement helps the NIPT platform stay current with the latest knowledge and maintain high accuracy in diverse clinical settings and populations.
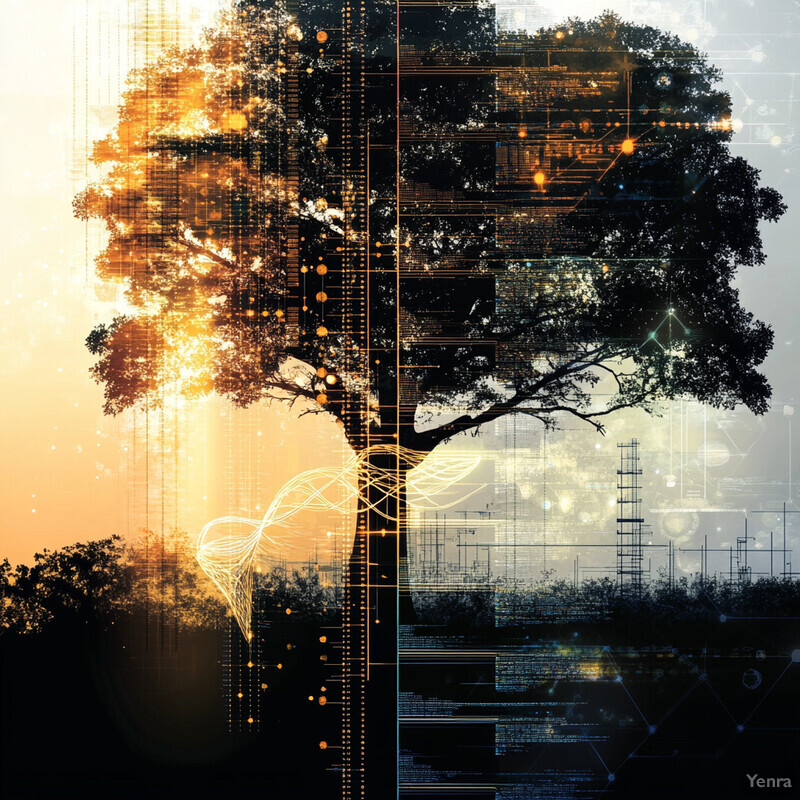
Experts emphasize the importance of training data growth for model improvement. As one review notes, an “ever-expanding body of sequencing data” is enabling the exploration of highly adaptable AI frameworks for cfDNA diagnostics. In other words, the more data these models see, the better they become at making robust predictions. Studies follow this principle: for example, a fetal fraction predictor trained on over 25,000 samples achieved excellent accuracy on new cases, a result of sheer training scale. Similarly, the NIPT-PG pan-genomic algorithm can be incrementally updated with population-specific variants as more genomes are analyzed, continually refining its z-score calculations for aneuploidy. Developers are also leveraging federated learning approaches (learning from distributed datasets) to update models without pooling sensitive data, allowing them to adapt using multi-center experience without compromising privacy. All these trends indicate that NIPT algorithms aren’t static products – they are evolving systems. Through ongoing validation and retraining with incoming data, AI-driven NIPT will keep improving its accuracy and utility over time, essentially “learning” from every test performed.
12. Epigenetic Marker Analysis
AI is enhancing NIPT by incorporating epigenetic markers – such as DNA methylation patterns – into analysis. Epigenetic information can help distinguish between similar genetic variants or identify which tissue DNA is coming from. By analyzing methylation signatures in cell-free DNA, AI algorithms can, for instance, differentiate fetal DNA from maternal DNA more precisely or detect conditions that have unique methylation profiles (like certain imprinting disorders or pregnancy complications). This added layer of information provides deeper insight into fetal health beyond the DNA sequence alone. Integrating epigenetic analysis through AI can improve NIPT’s accuracy and also broaden its applications (like predicting preeclampsia or other placental problems) since many of those involve epigenetic changes.
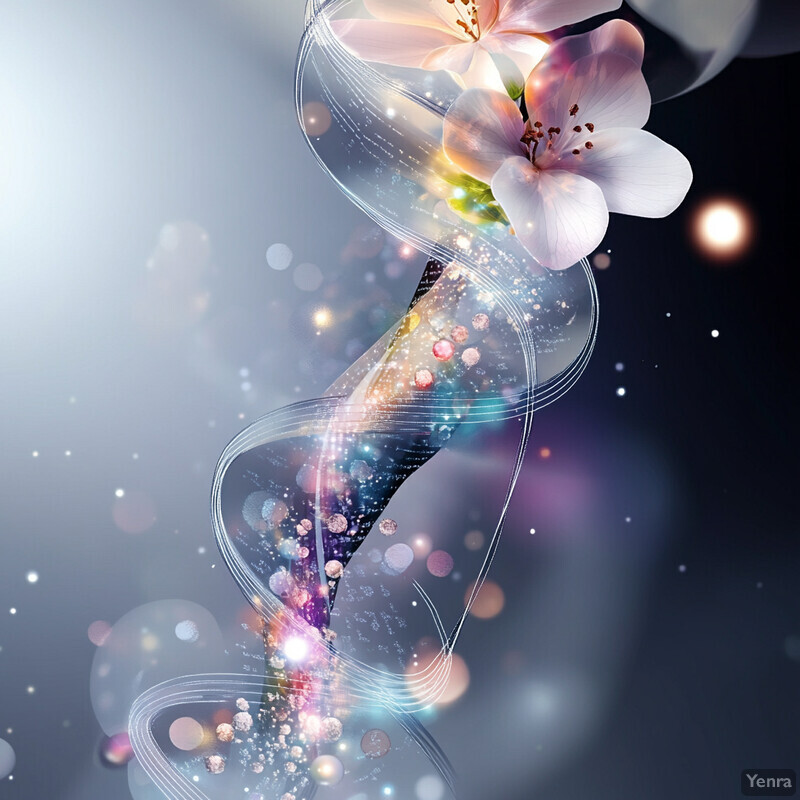
Recent studies underscore the value of epigenetic data in prenatal testing. In 2023, a study of first-trimester pregnancies used cfDNA methylation profiling to successfully predict early-onset preeclampsia risk before symptoms appeared. The researchers’ AI-driven model, which analyzed methylation across the genome, achieved an area under the curve (AUC) of ~0.85 for predicting preterm preeclampsia when combining methylation data with standard maternal risk factors. This demonstrates that methylation markers in the plasma can reveal presymptomatic signs of placental dysfunction. More broadly, AI can leverage known differential methylation patterns – for example, certain genes’ promoters are uniquely methylated in placenta vs. maternal blood – to improve NIPT interpretations. Machine learning models have also been developed to integrate methylomic data for tissue-of-origin analysis, which could help identify if an abnormal cfDNA signal comes from the placenta (fetus) or from a maternal tumor or other source. As these approaches mature, NIPT could double as an epigenetic screening, with AI algorithms flagging atypical methylation signatures associated with specific syndromes or pregnancy complications. It’s a promising convergence of genomics and epigenomics made possible by AI’s ability to handle complex patterns.
13. Advanced Mosaicism Detection
AI is better equipped to detect mosaicism – cases where only a fraction of fetal cells carry a genetic abnormality – during NIPT. Mosaicism can make test interpretation tricky, because the signals of aneuploidy in cfDNA are diluted. Advanced algorithms can recognize the subtle shifts in sequencing data that indicate a mosaic condition (for example, a slight elevation in reads from a chromosome that isn’t high enough to qualify as a full aneuploidy by standard cutoffs). By adjusting confidence thresholds and analyzing patterns across multiple data features, AI can catch partial abnormalities with higher sensitivity. This nuanced detection provides more diagnostic information, distinguishing true low-level mosaic cases from technical noise, and allowing clinicians to follow up appropriately when a mosaicism is suspected.
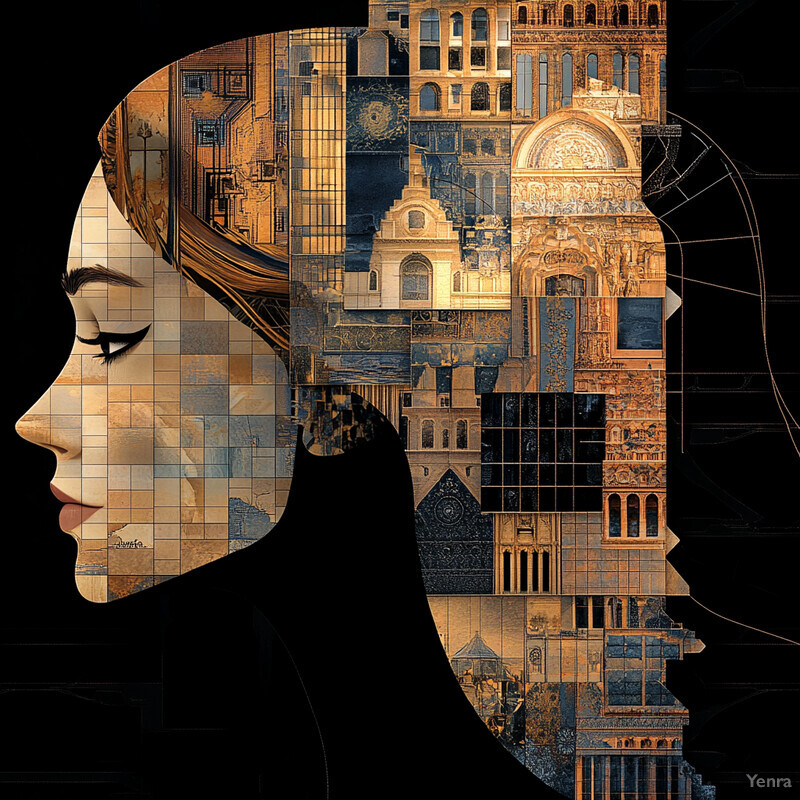
Mosaic genetic cases have been identified through improved NIPT analysis. For instance, one report documented two prenatal cases of trisomy 9 mosaicism that were detected by combining NIPT with multiple genetic tests – the non-invasive test showed an intermediate-risk result, which AI or specialized analysis flagged as possible mosaicism, later confirmed by amniocentesis. AI-driven pipelines are incorporating such capabilities: a 2024 machine-learning NIPT model demonstrated that it could handle fetal mosaicism without loss of accuracy. This model did not require a fixed fetal fraction input and maintained high predictive performance even in simulations of mixed cell populations (e.g., mosaic trisomy 21 in 30% of cells). Additionally, experts recommend using adjusted z-score thresholds for mosaic aneuploidy detection, a strategy that AI algorithms can dynamically implement rather than the static cutoffs in traditional analysis. Although full validation is ongoing, these developments suggest that AI will enable NIPT to report potential mosaic findings – giving a warning of partial aneuploidy that clinicians can investigate further – whereas earlier NIPT methods might have missed or dismissed those weak signals.
14. Reduced Sequencing Depth Requirements
AI’s advanced analytics allow NIPT to achieve high accuracy even with lower sequencing depth, which can reduce costs and make testing more accessible. Traditionally, NIPT requires sequencing millions of DNA fragments to detect aneuploidies with confidence. But with machine learning models extracting maximal information from fewer reads, it’s becoming possible to use shallower sequencing (fewer reads or shorter read lengths) without sacrificing performance. This means labs can potentially use less reagent and time per sample or multiplex more samples on one sequencing run. The ability to get reliable results from low-depth sequencing opens the door to cheaper NIPT, which could be especially beneficial in resource-limited settings or for broad population screening.
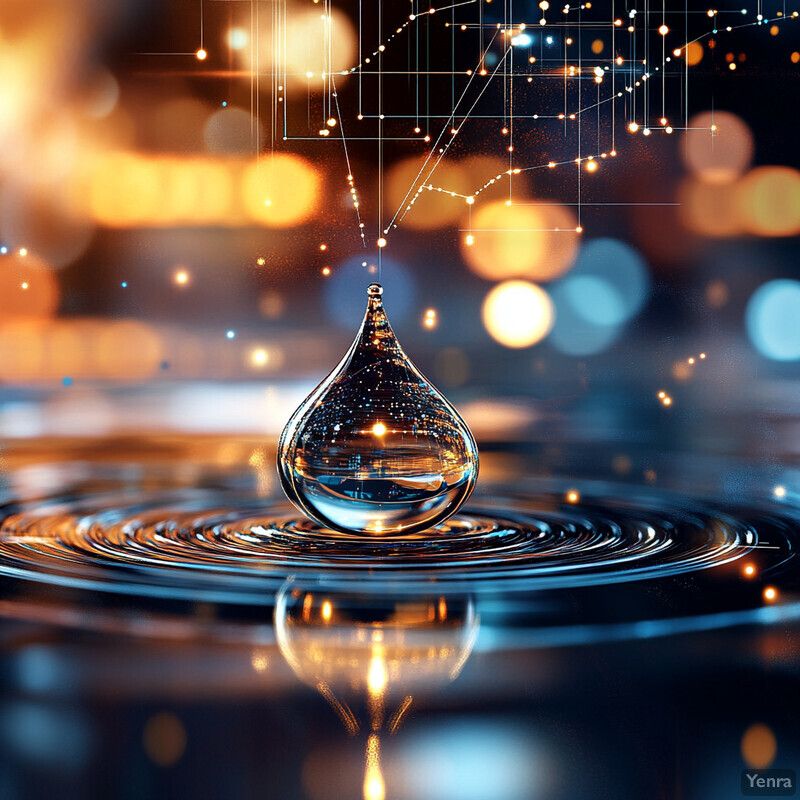
A recent study showcased an AI-optimized NIPT pipeline that delivered excellent accuracy with low sequencing input. The 2024 study proposed using only 40-base single-end reads (significantly shorter and fewer reads than usual) combined with a logistic regression model. Trained on public and clinical data, the AI pipeline achieved AUCs of 0.997–0.999 for trisomies 21, 18, and 13, comparable to standard high-depth methods. Specifically, for Down syndrome (T21) the model’s AUC was 99.85% and it attained positive predictive value (PPV) ~92% at a high sensitivity, outperforming the conventional z-score approach on the same low-coverage data. Importantly, this ML pipeline proved robust on an independent test set of 314 pregnancies from different centers, indicating it generalizes well. The authors noted their method did not even require a separate fetal fraction calculation step and could handle scenarios like twins while still using low-depth sequencing. These results imply that intelligent algorithms can compensate for reduced data quantity. By extracting more signal from fewer reads, AI makes it feasible to maintain NIPT’s high detection rates and low false-positive rates while cutting down sequencing requirements – a development that could lower per-test costs and increase throughput.
15. Integration with Electronic Health Records (EHRs)
AI allows NIPT to be integrated with patients’ electronic health records to personalize risk assessments. By pulling in data from EHRs – such as maternal age, medical history, lab results, or prior pregnancy outcomes – machine learning models can provide context to the raw genetic findings from NIPT. This holistic analysis can refine the risk estimates; for example, an AI might weigh a slightly elevated NIPT risk differently if the EHR shows the mother has certain risk factors or, conversely, protective factors. Integration with EHRs also enables AI to present results in a clinically meaningful way, highlighting relevant patient-specific factors and recommending tailored next steps. In essence, AI bridges the gap between genomic data and clinical data, moving prenatal testing towards a more comprehensive, “precision medicine” approach.
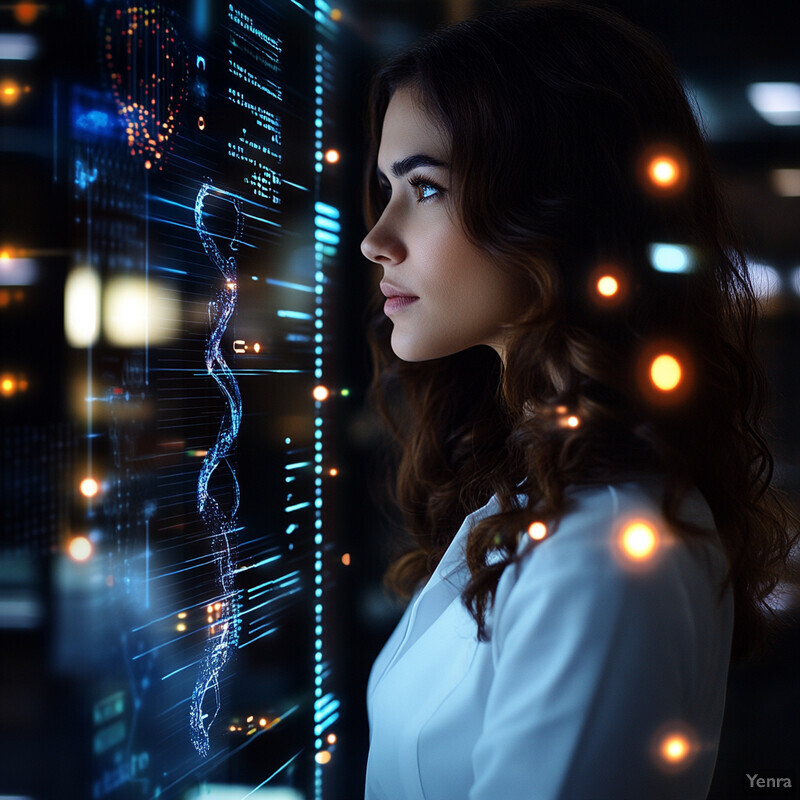
Early efforts illustrate the benefits of combining NIPT results with EHR data. In a 2023 study, incorporating routine maternal risk factors (like blood pressure, BMI, and pregnancy history) with cfDNA methylation data boosted the predictive accuracy for preeclampsia from an AUC of 0.75 (using DNA data alone) to 0.85. This shows that adding clinical context markedly improved outcome prediction. On the technical side, AI frameworks are being developed to merge multi-modal health data: a recent review highlights that multimodal AI can integrate not just genomic features but also clinical records and even patient conversations for more robust diagnostics. In practice, one could imagine an AI that knows a patient’s EHR-documented history of say, a heart defect in a prior baby, and therefore looks more closely at related cfDNA signals or ultrasound markers in the current pregnancy. Some institutions are already exploring such tools – for example, using large language models (LLMs) to summarize patient history and feed it into risk models. While still in development, these integrations point toward a future where NIPT is not an isolated test but part of an AI-driven, comprehensive prenatal profile that leverages all available patient data for the most accurate risk assessment.
16. Federated Learning for Data Privacy
Federated learning is an AI approach that allows NIPT algorithms to train on data from multiple centers or populations without ever pooling or exposing the raw data, thereby protecting patient privacy. In a federated model, each hospital or lab keeps its patient data locally and only shares encrypted model updates (not identifiable genetic information) with a central server. The central model learns from these combined updates. This means an AI can benefit from a much larger effective dataset – improving its accuracy and generalizability – without breaching confidentiality or violating data sharing regulations (like HIPAA or GDPR). Federated learning thus enables collaborative improvement of NIPT AI models on a global scale while ensuring sensitive prenatal genetic data remains secure and private at its source.
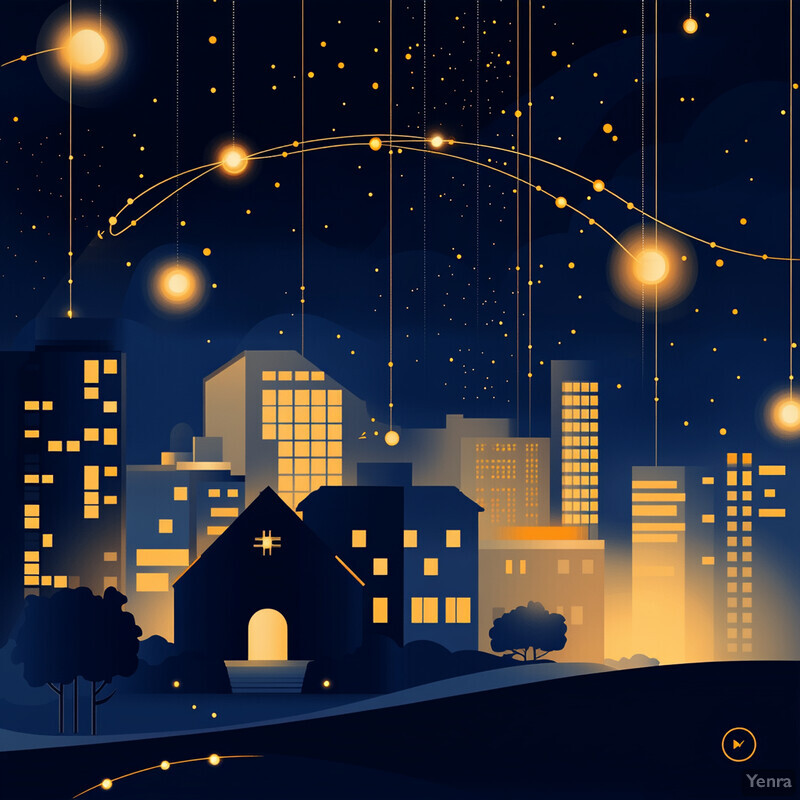
The healthcare industry is actively embracing federated learning to break down data silos. A 2024 review in the genomics domain noted that FL is “driving advancements in personalized healthcare while maintaining stringent privacy protections,” allowing data analysis without direct data transfer. This approach has already been tested on genomic datasets: a 2023 study simulated federated training on genomic data (e.g., UK Biobank) and found that the resulting models performed nearly as well as centrally-trained models, demonstrating the viability of FL for genetics. In context of NIPT, federated learning would let algorithms learn from diverse populations – say, combining expertise from labs in the US, Europe, and Asia – to recognize patterns (including rare variants or outcomes) that a single-institution model might miss. Importantly, each institution’s data stays behind its firewall, addressing legal and ethical concerns. Researchers highlight that FL can yield “stronger and more generalisable results” in multi-center healthcare studies by leveraging greater statistical power without compromising privacy. As NIPT datasets grow worldwide, federated learning provides a framework for collective AI model improvement: every new piece of data can contribute to the whole, all while each patient’s genetic information remains confidential and local.
17. Predictive Modeling of Pregnancy Outcomes
Beyond detecting genetic abnormalities, AI is leveraging NIPT data to predict broader pregnancy outcomes. By correlating early cfDNA signals and other biomarkers with later pregnancy events, machine learning models can assess the risk of complications such as preeclampsia, gestational diabetes, preterm birth, or fetal growth restriction. This predictive modeling transforms NIPT into a tool not only for genetic screening but also for forecasting obstetric health. An AI might detect, for instance, a subtle shift in fragment patterns that indicates placental stress, alerting clinicians to a higher risk of preeclampsia well in advance. Such early warnings enable proactive monitoring or preventive measures. In sum, AI is turning NIPT data into a window on pregnancy wellness, helping anticipate and improve outcomes for mother and baby.
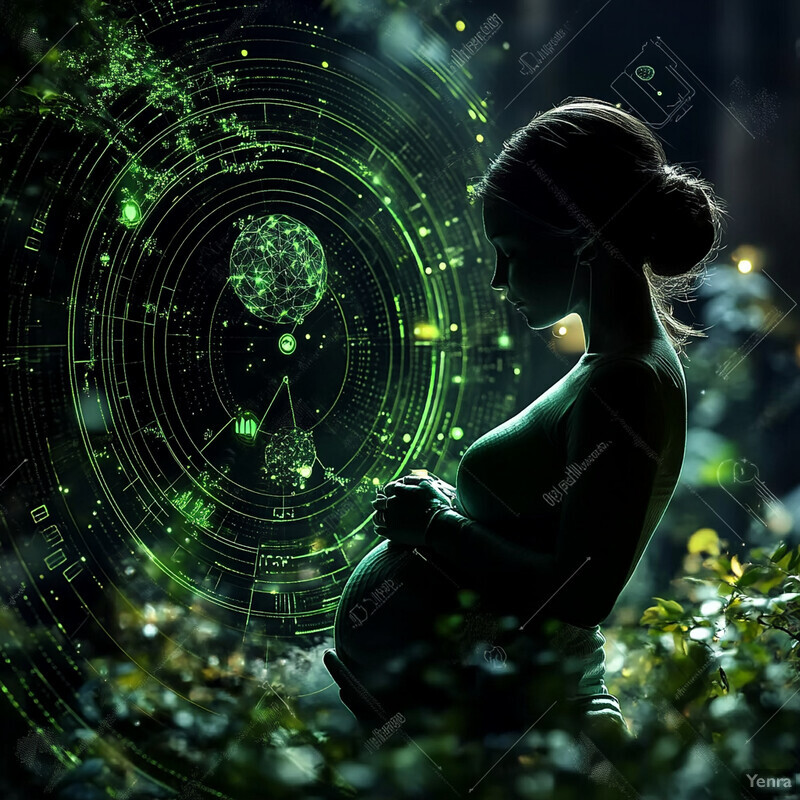
Recent research validates that cfDNA-based models can prognosticate pregnancy complications. A prime example is the PTerm model (2025), which used machine learning on cfDNA sequencing to predict spontaneous preterm birth. Trained on over 2,500 cases, this SVM classifier focused on nucleosome positioning signals in cfDNA and achieved an AUC of ~0.85 in independent validation, correctly identifying a large proportion of women who went on to deliver preterm. Similarly, another study profiled cfDNA methylation in 498 first-trimester pregnancies and developed a model that presymptomatically predicted early-onset preeclampsia; at 80% specificity, it could predict ~72% of severe preeclampsia cases, months before clinical diagnosis. These advances were possible because AI can find patterns in cfDNA – reflecting placental DNA release, hypoxia-related methylation changes, etc. – that correlate with later disease. Importantly, these predictive models did not require additional invasive tests: they repurposed the same blood draw used for NIPT. As such, an AI-enhanced NIPT report might one day include not just “low risk for aneuploidy,” but also a personalized risk score for preterm birth or other outcomes, giving obstetric providers invaluable foresight to tailor prenatal care.
18. Robust Decision Support Tools
AI is powering a new generation of decision support tools for prenatal testing, aimed at helping both clinicians and patients understand NIPT results. These tools often feature intuitive interfaces (like apps or software dashboards) that translate complex genetic probabilities into clear visuals or language. For clinicians, AI can provide evidence-based recommendations – for example, suggesting when an abnormal NIPT result warrants an immediate diagnostic test versus when it might be a borderline finding to monitor. For patients, AI-driven chatbots or virtual counselors are being developed to answer common questions about NIPT and genetic risks, helping to reduce anxiety and improve informed decision-making. Overall, these decision support systems act as a bridge between raw data and actionable next steps, making the NIPT process more user-friendly and supportive.
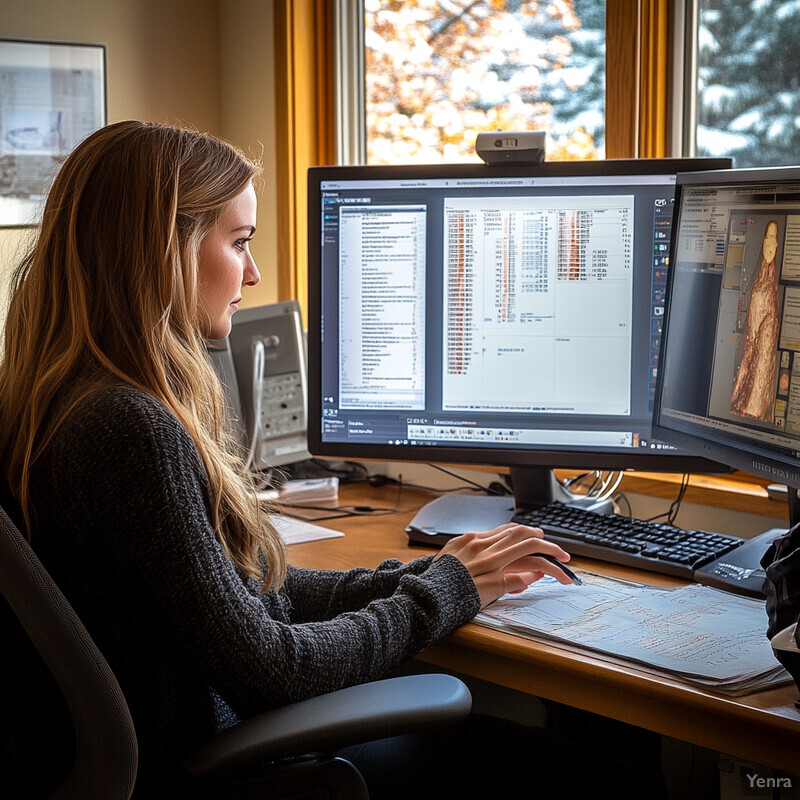
Studies show that AI-based tools can effectively assist in genetic counseling and patient education. In 2024, researchers tested a generative AI (ChatGPT) on common genetic counseling questions and found it provided correct answers about 83% of the time. This suggests AI could serve as a reliable informational resource for patients asking about NIPT and related topics. Moreover, a multicenter randomized trial published in 2025 evaluated a chatbot designed for pre-test education on prenatal aneuploidy screening. The results were striking: patients who used the AI chatbot before seeing their provider had significantly greater knowledge gains about their testing options (+4.1 correct answers improvement vs +1.9 in the control group). Both patients and providers reported high satisfaction with the chatbot approach, indicating that it augmented (not replaced) the counseling experience effectively. These findings demonstrate that well-designed AI tools can demystify NIPT results and next steps. For example, an AI-driven platform might display a graphical risk chart for a detected microdeletion and then, in plain language, explain what that means and suggest discussion points for the doctor and patient. By providing clear explanations and guidance, AI decision support tools enhance informed consent and ensure that expectant parents feel supported and empowered throughout the NIPT process.