1. Enhanced Ultrasound Image Analysis
AI algorithms can analyze fetal ultrasound images with greater precision, improving the detection of subtle abnormalities and helping clinicians spot indicators of genetic or structural anomalies far earlier than traditional methods.
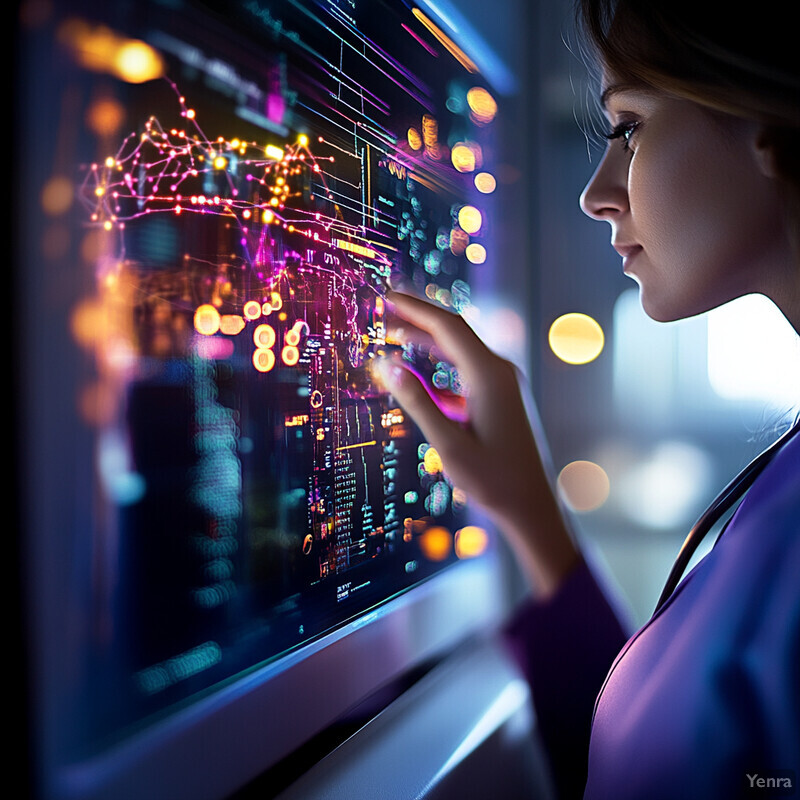
AI-powered image analysis tools are revolutionizing the interpretation of prenatal ultrasound images. Traditional ultrasounds rely heavily on a sonographer’s skill and experience, which can introduce variability in interpretation. By using deep learning algorithms trained on extensive libraries of fetal images, AI can identify anatomical structures with high precision, detect subtle variations that may indicate developmental issues, and differentiate between normal and abnormal growth patterns. This enhanced accuracy ensures that potential problems—such as congenital heart defects, neural tube defects, or craniofacial anomalies—can be detected earlier in the pregnancy, improving the likelihood of timely interventions and more favorable outcomes for both mother and child.
2. Automated Prenatal Measurements
Machine learning models can automatically measure critical fetal parameters—such as crown-rump length and head circumference—from ultrasound images, increasing consistency and reducing human error in assessments.
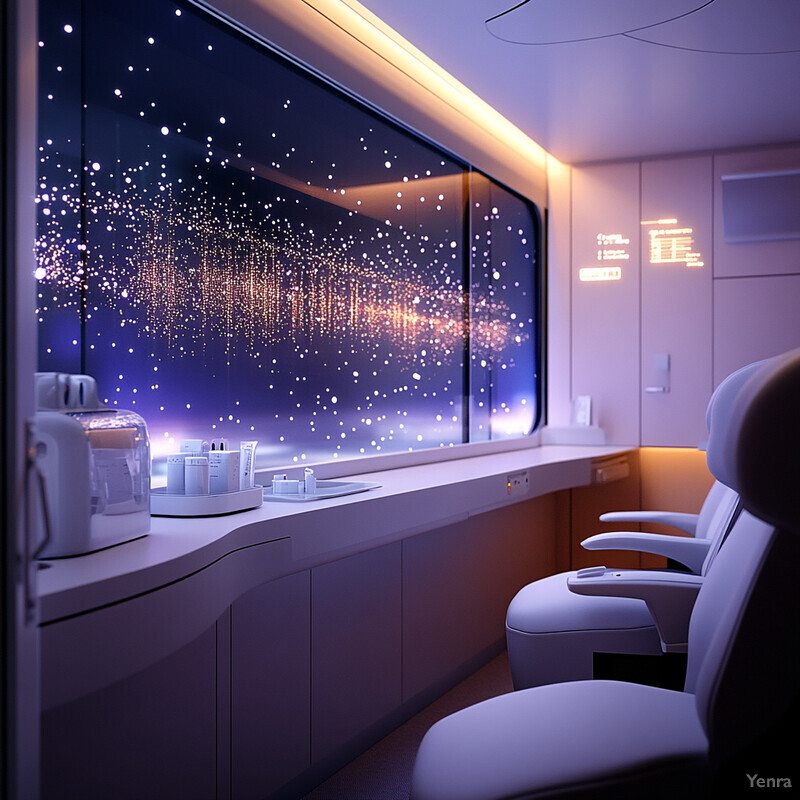
In prenatal care, consistent and accurate measurements of fetal parameters such as head circumference, biparietal diameter, femur length, and abdominal circumference are crucial for assessing growth and development. AI-driven tools can automatically identify fetal landmarks and perform these measurements without relying on manual tracing by clinicians, thus reducing human error. By standardizing these processes, AI ensures reproducible results and more reliable growth charts. This automation not only saves time for healthcare providers but also supports more personalized care plans by providing objective, data-driven insights into fetal health and development.
3. Advanced Cell-Free DNA Analysis
AI-driven pattern recognition and statistical modeling can improve the interpretation of cell-free fetal DNA in the maternal bloodstream, making non-invasive prenatal testing (NIPT) more accurate for detecting chromosomal abnormalities like Down syndrome.
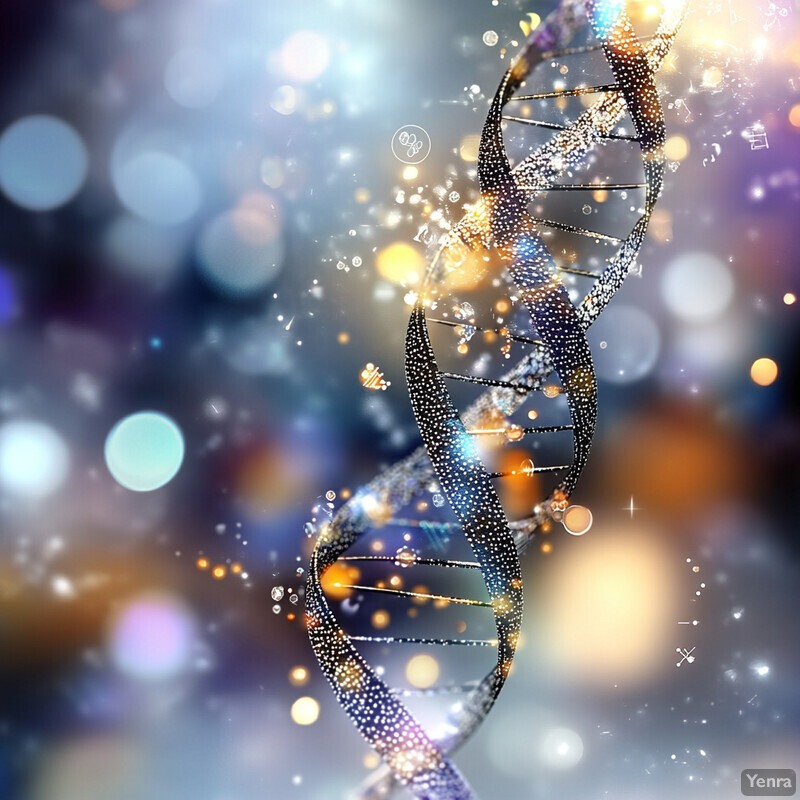
Non-Invasive Prenatal Testing (NIPT) leverages the presence of cell-free fetal DNA in the maternal bloodstream to detect chromosomal abnormalities like trisomies (e.g., Down syndrome, Edwards syndrome). AI enhances this process by applying sophisticated algorithms that improve the interpretation of the raw genomic data. Through pattern recognition, machine learning models can differentiate between maternal and fetal genetic signatures, handle issues like low fetal fraction, and more accurately stratify the risk of specific genetic conditions. This leads to increased sensitivity and specificity, offering expectant parents and clinicians greater confidence in the results of these non-invasive tests.
4. Risk Stratification for Genetic Disorders
By integrating maternal age, family history, and blood test results, AI can generate personalized risk profiles for various genetic conditions, guiding clinicians in deciding which additional tests (if any) are needed.
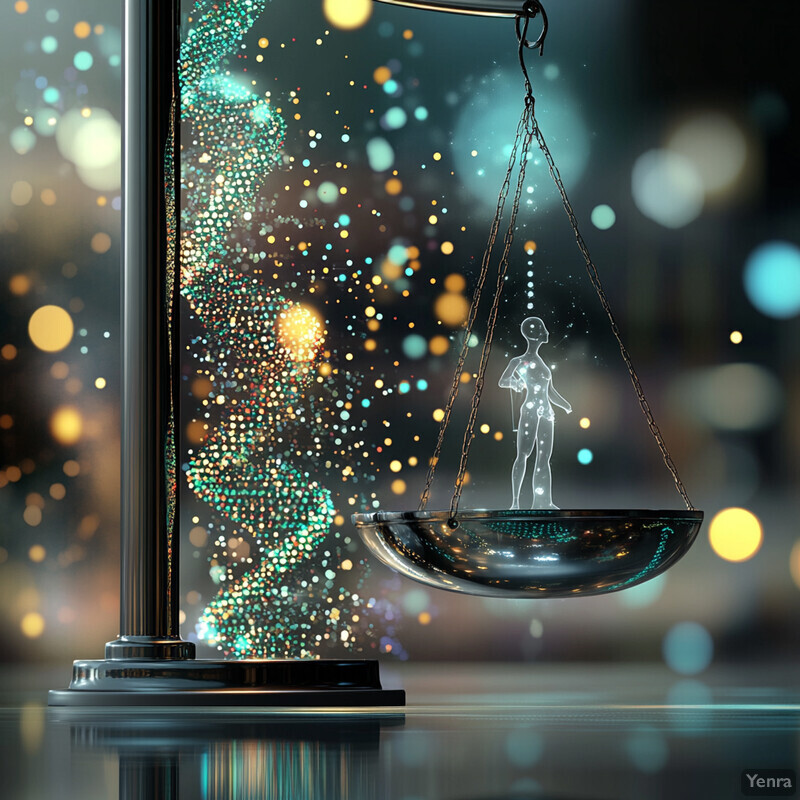
Determining the likelihood of a fetus having certain genetic disorders involves synthesizing multiple data points—from maternal age and ethnicity to family history, blood biomarkers, and imaging findings. AI models excel at integrating these heterogeneous datasets and identifying patterns that might be subtle or invisible to the human eye. By quantifying risk in a personalized manner, AI supports informed decision-making about whether to pursue additional testing or interventions. This individualized approach allows clinicians to prioritize resources efficiently, reduces unnecessary invasive testing, and provides a more nuanced understanding of a fetus’s genetic risk profile.
5. Improved Sensitivity and Specificity
Machine learning techniques can refine cutoff points and scoring algorithms, reducing the rates of false positives and false negatives in prenatal screening tests.
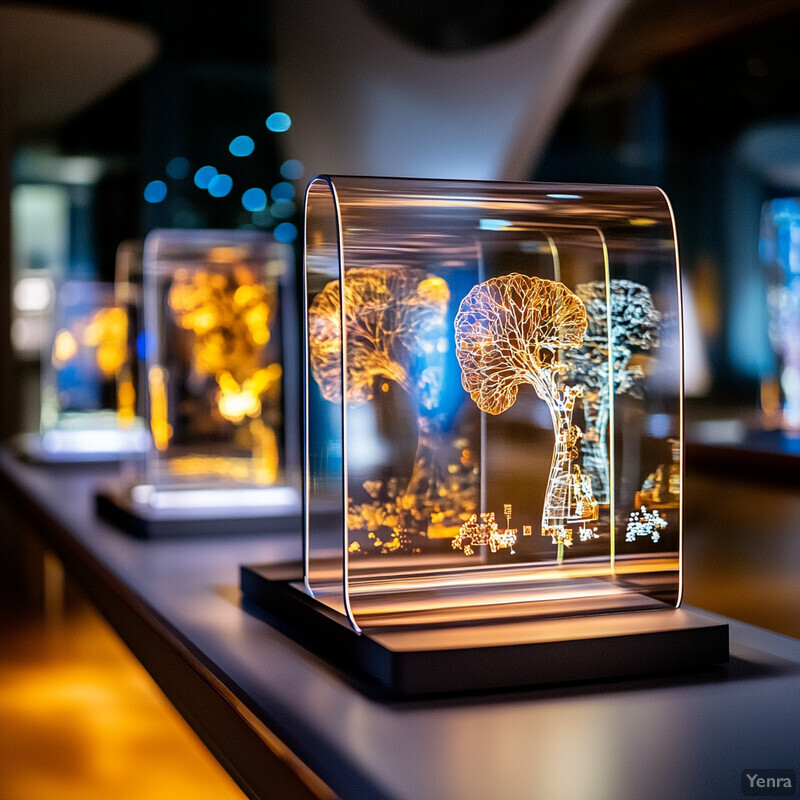
One of the main challenges in prenatal screening is balancing the reduction of false positives (which lead to undue stress and potentially unnecessary invasive tests) with maintaining high sensitivity (to ensure no significant conditions are missed). AI algorithms can fine-tune the cutoff values and diagnostic thresholds used in prenatal screenings by learning from historical patient outcomes. Over time, these models adjust their predictive criteria to maintain a careful equilibrium—improving the accuracy and reliability of screening tests. The resulting gains in sensitivity and specificity ensure that more families receive accurate information, reducing anxiety and enhancing trust in prenatal care protocols.
6. Early Prediction of Pregnancy Complications
Using predictive modeling on maternal biomarkers, patient history, and environmental factors, AI can forecast conditions such as preeclampsia or gestational diabetes before they manifest, allowing for earlier interventions.
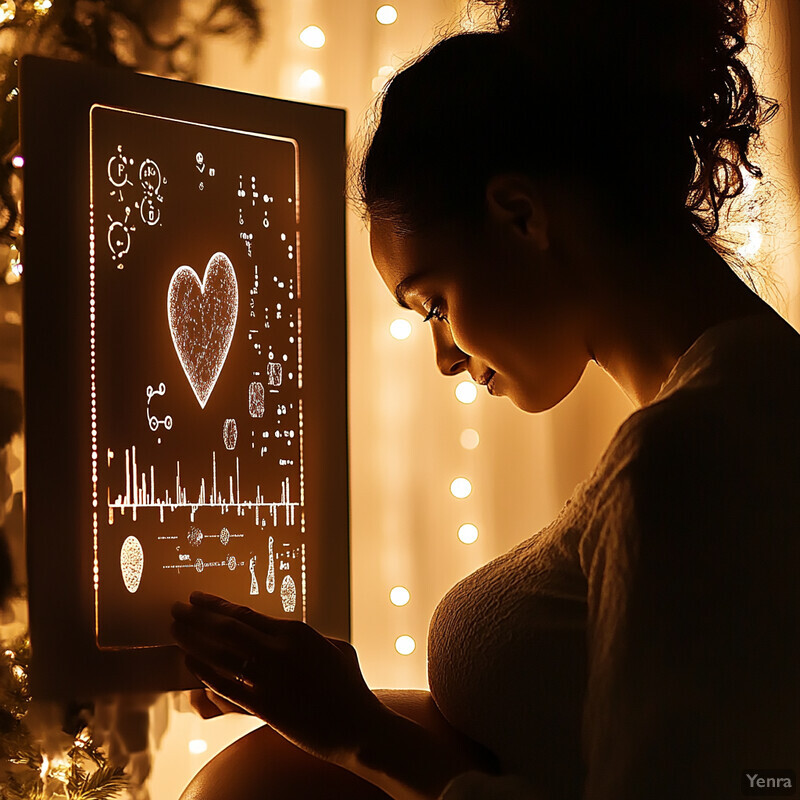
Conditions such as preeclampsia, intrauterine growth restriction (IUGR), and gestational diabetes often emerge in mid-to-late pregnancy, sometimes with limited opportunities for preemptive interventions. AI-driven predictive models can combine maternal medical history, genetic predispositions, environmental factors, and early pregnancy biomarker levels to anticipate these complications far in advance. By flagging high-risk pregnancies early, healthcare providers can initiate preventive measures—such as dietary adjustments, closer monitoring, or prophylactic medications—ultimately improving maternal and fetal outcomes and potentially reducing healthcare costs associated with managing late-stage complications.
7. Integration of Multi-Modal Data
AI can synthesize information from imaging, biochemical markers, and maternal health records, yielding a more holistic assessment of fetal well-being without invasive procedures.
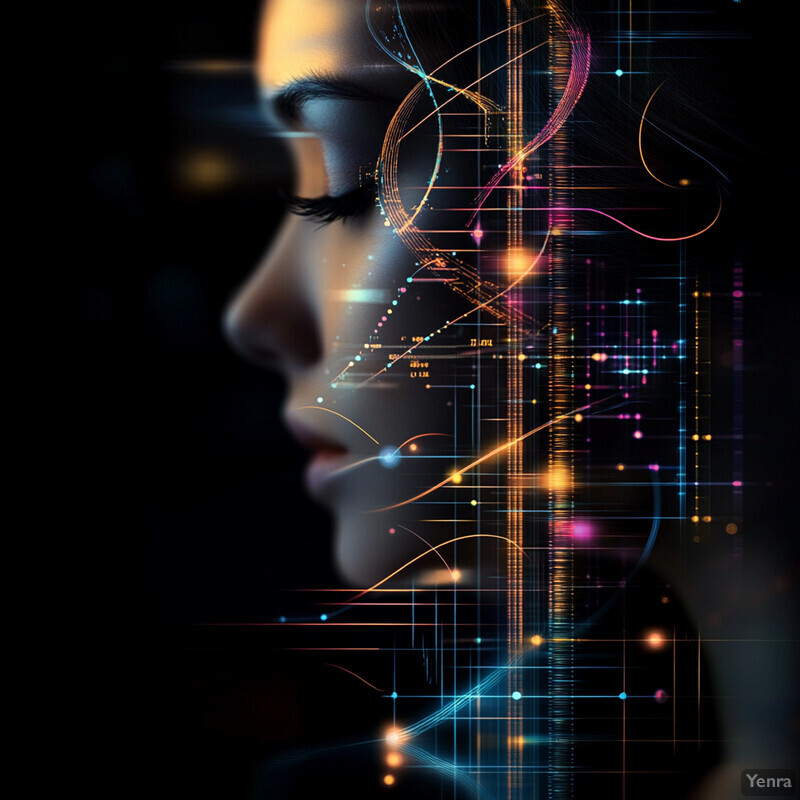
Prenatal assessments often involve a variety of data streams, including ultrasound imaging, biochemical tests, maternal blood pressure readings, and lifestyle factors. AI excels at synthesizing this diverse information into a comprehensive picture of fetal health. Rather than relying on any single data source, advanced models integrate all available metrics to identify patterns and anomalies that might be missed when data sources are considered in isolation. This holistic approach leads to more accurate risk assessments, helping clinicians tailor monitoring schedules, intervention strategies, and patient counseling sessions with unparalleled precision.
8. Genomic Variant Interpretation
With the growing volume of genetic data, AI tools can help interpret uncertain or novel genomic variants detected in non-invasive tests, providing clearer guidance on the significance of these findings.
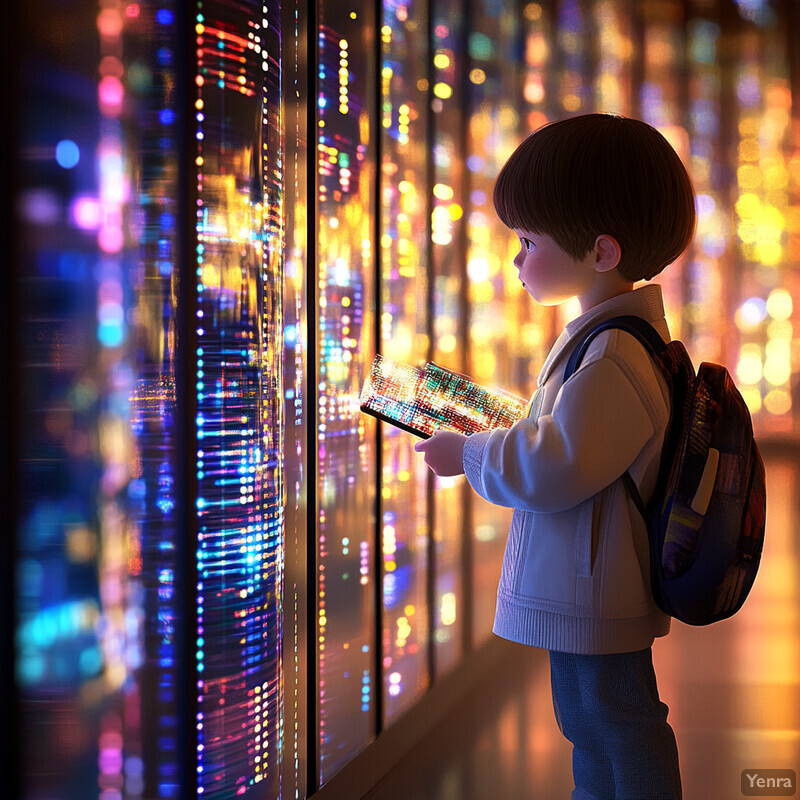
With the ongoing expansion of genetic testing, clinicians increasingly encounter novel or poorly characterized genetic variants. Determining whether these variants are benign or pathogenic can be complex. AI-driven genomic analysis tools can rapidly query large genomic databases, identify similar variants reported in the literature, and predict the functional consequences of genetic changes. Through machine learning, these systems become more adept at classifying variants over time, assisting genetic counselors and physicians in providing clearer, evidence-based guidance to expectant parents about the implications of newly discovered genetic markers.
9. Real-Time Decision Support
AI-powered clinical decision support systems can guide physicians during prenatal screening, suggesting optimal test combinations, highlighting suspicious findings, and recommending follow-up actions on the fly.
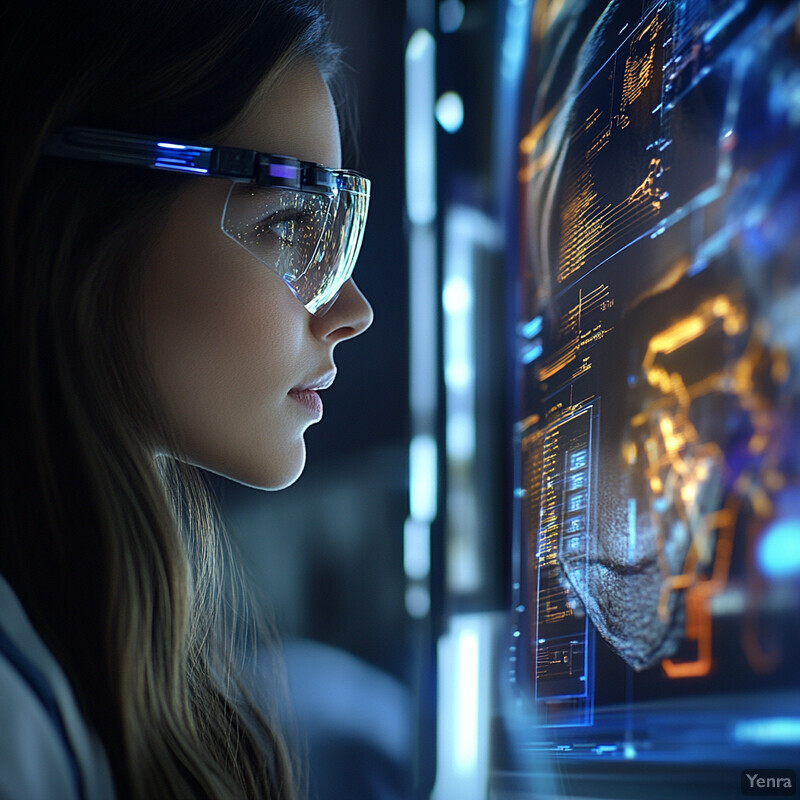
Clinical decision support (CDS) systems powered by AI can guide healthcare providers through the complexity of prenatal screening. As clinicians input patient data, the AI can suggest which tests to order next, highlight subtle abnormalities on ultrasound images, or recommend genetic counseling based on risk profiles. By offering immediate, evidence-based suggestions, these tools free clinicians from cognitive overload, reduce reliance on subjective judgment, and maintain consistent standards of care. The result is a more standardized and streamlined decision-making process that leverages the best available information at every step.
10. Ultrasound Workflow Optimization
Automated segmentation and analysis of fetal ultrasound structures streamline the sonographer’s workflow, freeing up time for more patient counseling and reducing operator dependency.
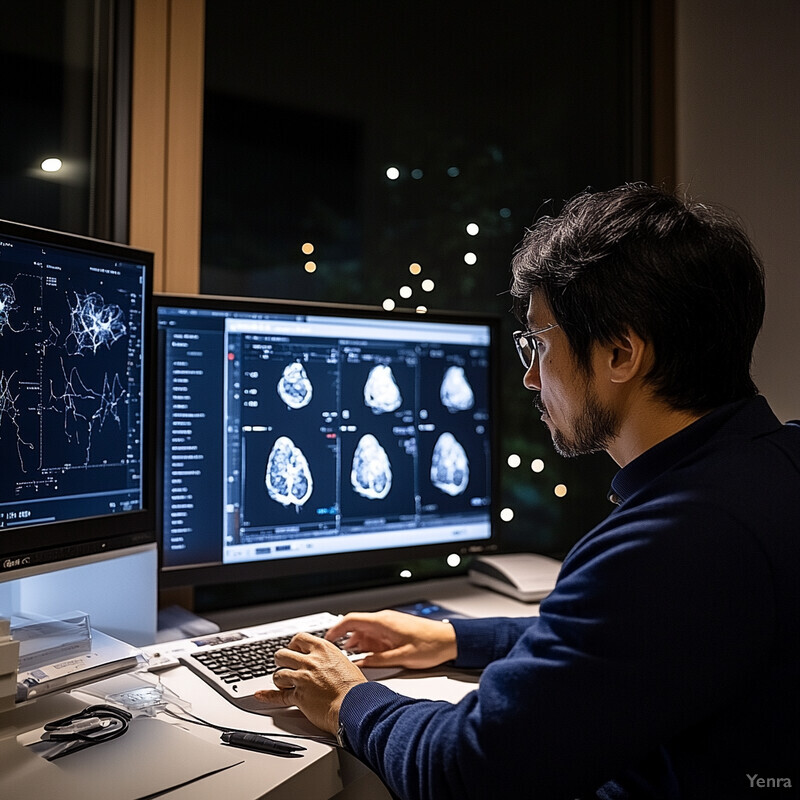
Ultrasound examinations are time-sensitive and operator-dependent. AI-driven workflow solutions can segment fetal structures automatically, label anatomical landmarks, and generate preliminary reports, significantly shortening the time sonographers need to spend on routine tasks. By taking over repetitive components, these systems reduce human fatigue, minimize operator-to-operator variability, and allow clinicians to focus on interpreting the data rather than merely acquiring it. Enhanced efficiency also means that healthcare teams can handle more patients while maintaining a high standard of care, ultimately improving access to quality prenatal imaging services.
11. Longitudinal Growth Tracking
AI can track fetal growth metrics over time, identifying deviations from normal growth patterns early, helping clinicians monitor the fetus’s health comprehensively without invasive measures.
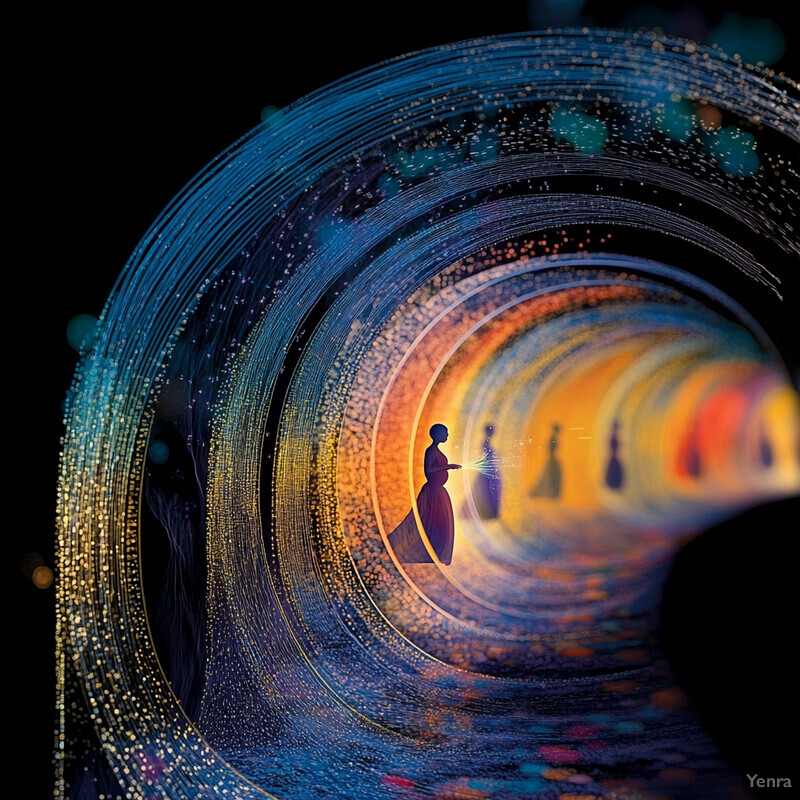
Monitoring fetal growth over multiple prenatal visits is essential for detecting deviations such as growth restriction or macrosomia. AI-based systems can integrate measurements from sequential ultrasounds, creating sophisticated growth curves and alerts when trends deviate from established norms. By automatically flagging abnormal trajectories, these systems help providers intervene earlier—whether that involves nutritional counseling, increased surveillance, or scheduling delivery at an optimal time. Consistent, data-driven growth tracking leads to better-informed clinical decisions and can improve neonatal outcomes by ensuring potential issues are addressed promptly.
12. Quality Assurance in Laboratories
AI-driven quality control systems can continuously evaluate non-invasive test data, ensuring test kits and laboratory processes maintain high standards and produce consistent results.
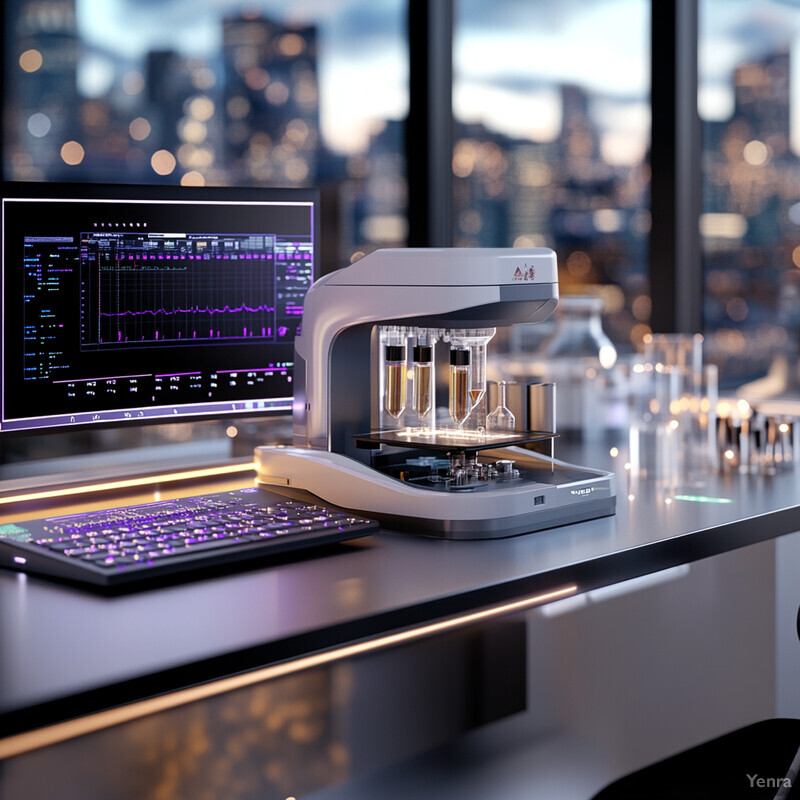
As prenatal testing expands, ensuring that laboratory procedures for non-invasive tests remain accurate and consistent is paramount. AI-based quality control systems can monitor large volumes of test data, identifying patterns that might indicate reagent degradation, calibration drift, or procedural inconsistencies. By spotting problems early, these tools help laboratories maintain regulatory compliance, minimize batch failures, and ensure the reliability of their outputs. This heightened level of quality assurance ultimately translates to more trustworthy test results and greater confidence for both clinicians and patients.
13. Predictive Analytics for NICU Needs
By correlating prenatal data with outcomes, AI can predict the likelihood that a newborn may require specialized neonatal care, aiding in preparedness and resource allocation.
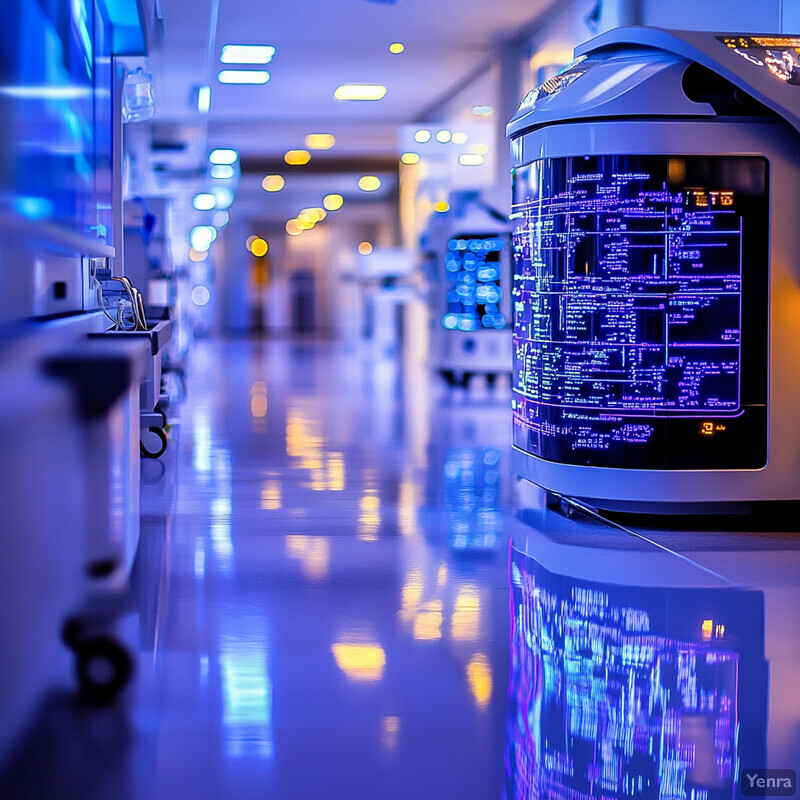
Even before birth, AI models can estimate the likelihood that a newborn will require specialized neonatal intensive care. By analyzing prenatal imaging, maternal health records, and known risk factors, predictive analytics can forecast complications such as respiratory distress or neonatal jaundice. Hospitals can then allocate NICU resources more effectively, ensuring that staff and equipment are ready when needed. This proactive approach not only improves the quality of neonatal care but can also optimize healthcare spending, reduce emergency scenarios, and provide parents with greater peace of mind.
14. Enhanced Detection of Rare Conditions
AI can detect subtle genomic or biochemical signatures associated with rare genetic syndromes, improving the chances of early and non-invasive identification.
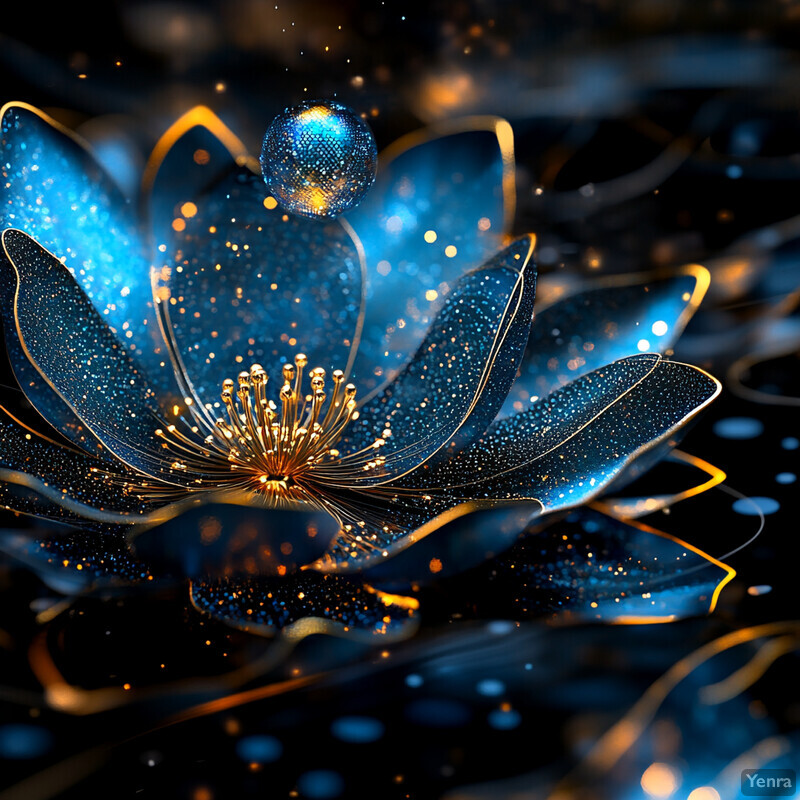
Rare genetic disorders can be challenging to detect through standard screening protocols due to their low prevalence and subtle indications. AI systems trained on large datasets can identify uncommon but significant genetic or biochemical signatures in prenatal test results. By improving the detection rate of rare conditions, these tools allow for earlier diagnosis and better-informed management. Expectant parents benefit by having more time to consider care options, participate in genetic counseling, and prepare emotionally and practically for a child with special healthcare needs.
15. Image-Based Biomarker Discovery
Deep learning can identify previously unknown image-based markers that correlate with certain fetal conditions, enhancing non-invasive diagnostic capabilities.
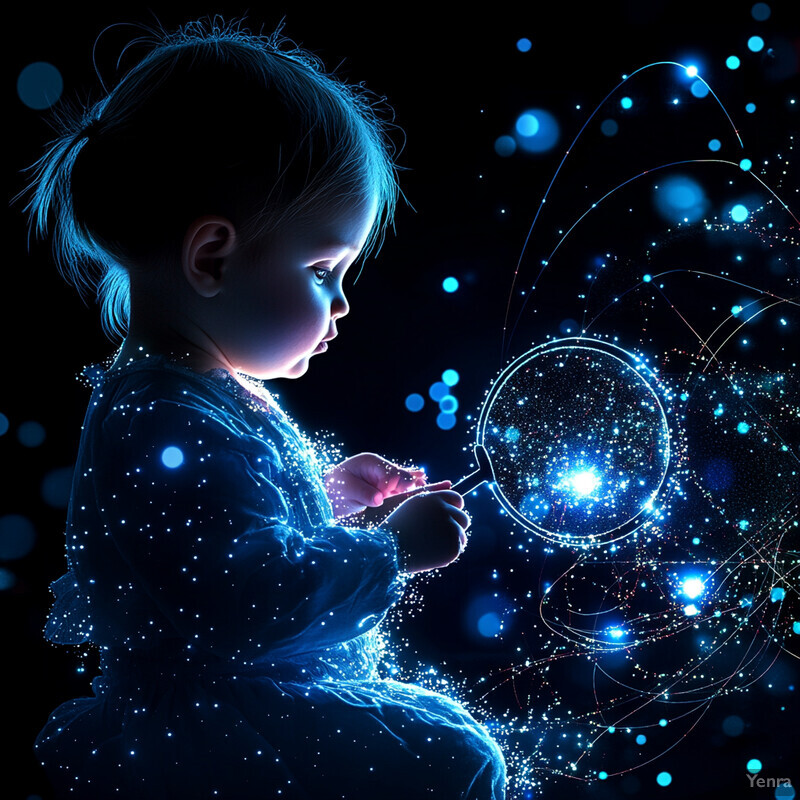
Beyond established imaging criteria, deep learning models can uncover previously unknown image-based biomarkers correlated with fetal health. These might include subtle textural patterns in ultrasound images, specific changes in blood flow detectable by Doppler analysis, or minute differences in skeletal development. By recognizing these patterns, AI expands the diagnostic toolkit available to clinicians. Over time, the discovery of new biomarkers can lead to earlier detection of anomalies, improved risk assessments, and more targeted follow-ups that minimize invasive procedures.
16. Machine-Learned Diagnostic Protocols
AI systems can learn from historical datasets, refining testing protocols and suggesting personalized screening pathways that minimize unnecessary invasive procedures while maximizing diagnostic yield.
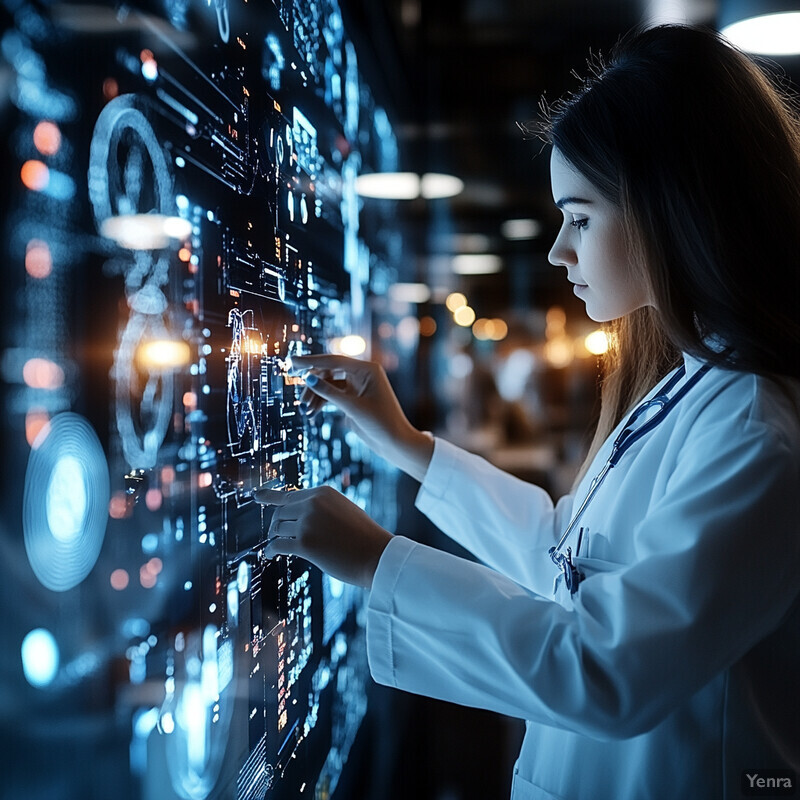
Clinical guidelines for prenatal screening evolve over time as new research emerges. AI systems can accelerate this evolution by analyzing retrospective datasets, outcomes, and patient cohorts to determine which testing protocols yield the best balance of accuracy, cost-effectiveness, and patient comfort. As these models continuously learn from real-world results, they can recommend dynamic, patient-specific testing pathways. This adaptive approach ensures that prenatal care keeps pace with scientific advancements and that patients benefit from the most up-to-date, evidence-based screening strategies available.
17. Population-Level Insight
Aggregating data from large populations allows AI to identify patterns and trends in prenatal health, aiding public health agencies and improving screening guidelines for diverse populations.
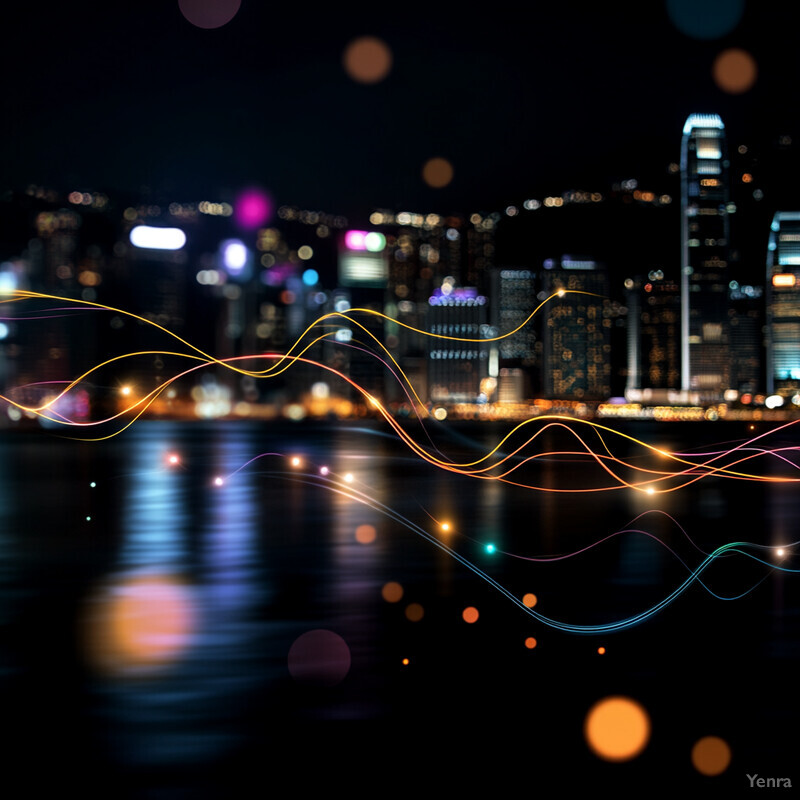
Aggregated, anonymized prenatal data analyzed by AI can generate valuable public health insights. By examining large-scale trends in fetal health, such as correlations between maternal conditions and birth outcomes, AI can inform policy decisions, screening guidelines, and resource allocation for entire populations. Public health agencies can use these findings to refine screening protocols, ensure equitable access to care, and target interventions where they are most needed. Over time, population-level insights contribute to healthier pregnancies and improved neonatal outcomes across different communities.
18. Patient-Centric Educational Tools
AI-driven chatbots and interactive decision aids can help expectant parents understand their non-invasive test results, providing personalized explanations and reducing anxiety.
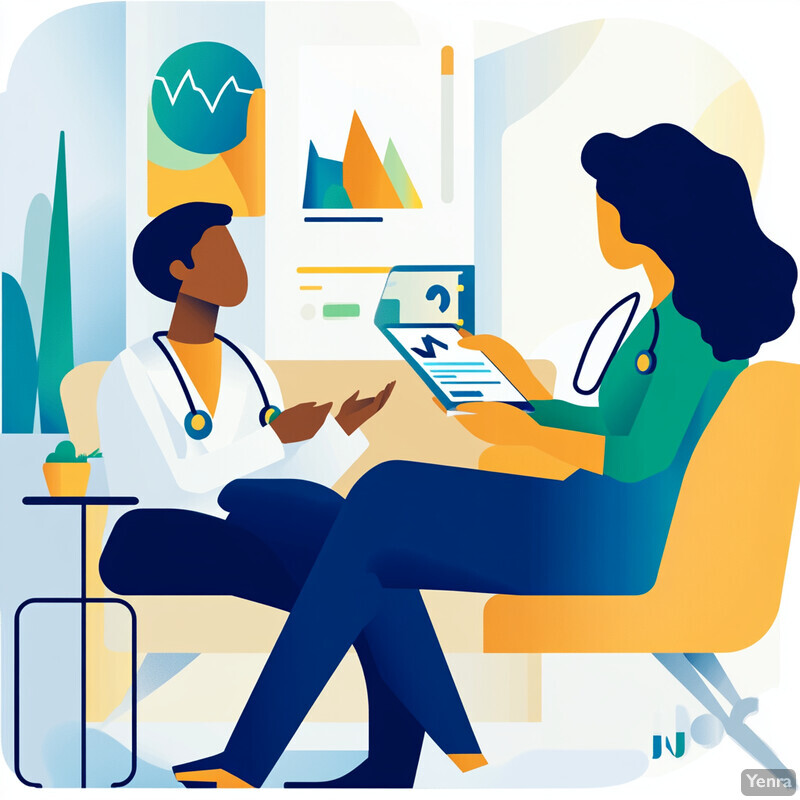
For expectant parents, understanding complex prenatal test results can be overwhelming. AI-powered chatbots and educational platforms can translate technical, data-heavy findings into clear, accessible language. These interactive tools provide personalized explanations, highlight the significance of certain biomarkers, and offer next steps, empowering parents to ask informed questions during consultations. By reducing confusion and anxiety, patient-centric AI tools promote shared decision-making and foster a stronger collaborative relationship between families and their healthcare providers.
19. Reduced Need for Invasive Testing
By increasing the accuracy and confidence in non-invasive assessments, AI reduces the necessity for confirmatory invasive tests like amniocentesis, lowering risks to both mother and fetus.
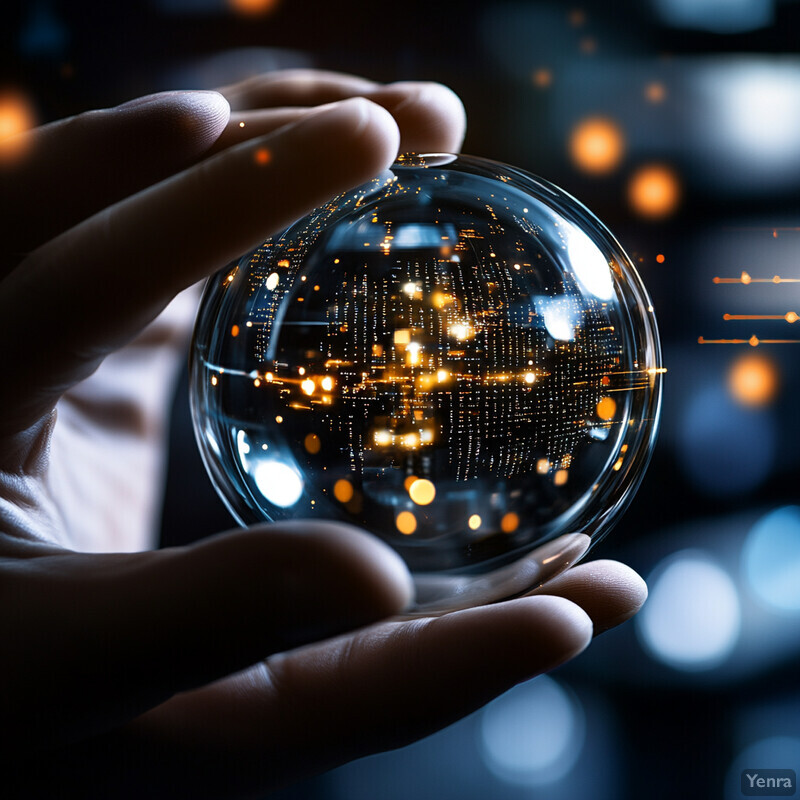
As AI improves the sensitivity, specificity, and predictive value of non-invasive tests, fewer patients will need confirmatory invasive procedures like amniocentesis or chorionic villus sampling. These procedures carry risks such as miscarriage, so minimizing their necessity reduces potential harm. In turn, the healthcare system can focus on providing non-invasive tests widely, increasing accessibility and comfort for patients. Ultimately, AI-driven improvements in non-invasive testing contribute to a safer, more patient-friendly prenatal care experience.
20. Continuous Improvement Through Feedback Loops
AI models can update and refine themselves as they receive new data, continually improving the accuracy, reliability, and utility of non-invasive prenatal health assessments over time.
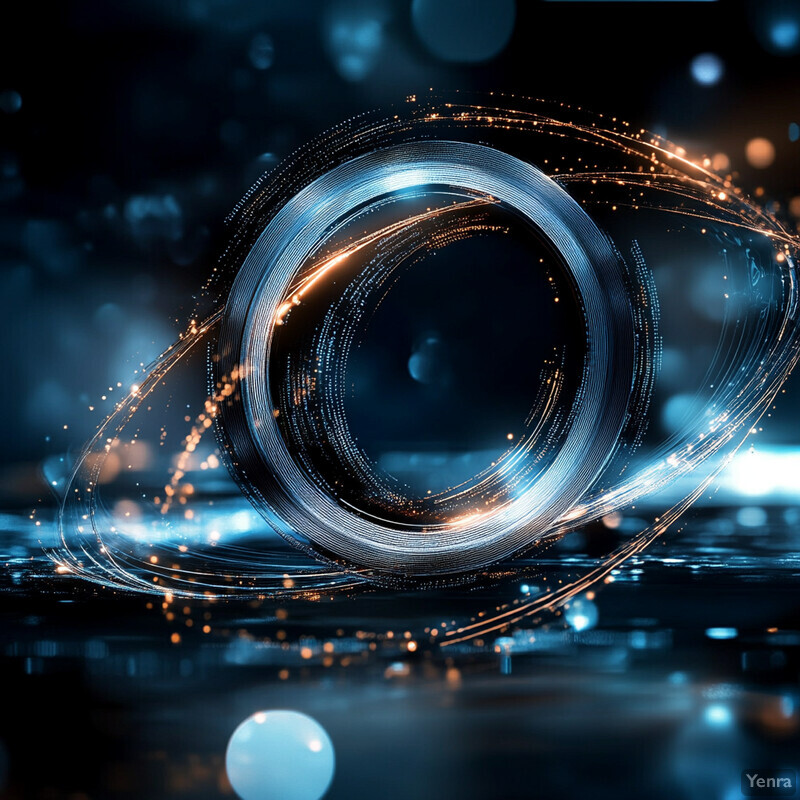
AI models do not remain static. They continuously learn from new data, patient outcomes, and advances in research. As more prenatal tests are conducted and integrated with patient follow-ups, AI algorithms refine their predictions, improve their diagnostic criteria, and adapt to emerging conditions or detection methods. This iterative process ensures that prenatal assessment methods remain on the cutting edge of accuracy and reliability. Over time, the system’s performance improves, benefiting future patients and bolstering the overall quality of prenatal healthcare worldwide.