1. Enhanced Signal Processing
AI-driven signal processing techniques are used to clean raw data from non-invasive glucose sensors. By learning the patterns of noise (from motion, ambient light, etc.), machine learning models can filter out spurious signal components. These enhanced filters produce smoother, cleaner data streams from optical or electrical sensors. Improved data quality leads to more reliable glucose estimates and fewer false readings. Recent studies show that integrating AI into signal processing significantly boosts sensor accuracy.
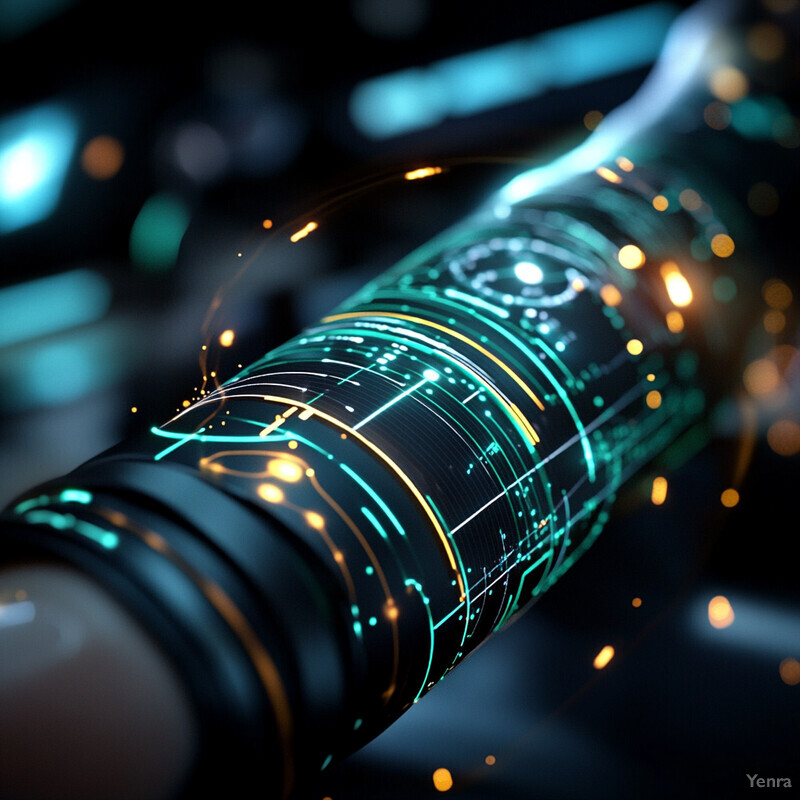
In non-invasive monitoring, motion and light artifacts can corrupt sensor outputs. Zohuri (2025) reports that machine learning models can “filter out noise from movement, ambient light fluctuations, and physiological variations” in PPG and NIRS signals. Similarly, Zeynali et al. (2025) applied a third-order Butterworth bandpass filter to photoplethysmography (PPG) data, effectively eliminating undesired noise (0.5–8 Hz passband) and “ensur[ing] data quality and reliability”. These methods demonstrate that AI-enhanced filters and preprocessing significantly reduce artifacts in non-invasive glucose signals, yielding more stable glucose estimates.
2. Feature Extraction in Spectroscopy
Advanced AI methods automatically extract meaningful features from complex spectroscopic data. Non-invasive glucose sensors often use infrared or Raman spectroscopy, which generate high-dimensional signals. Deep learning models (e.g. CNNs/RNNs) can identify subtle spectral patterns associated with glucose, eliminating the need for manual feature selection. By focusing on wavelengths or spectral shapes that correlate with glucose, AI enhances model sensitivity. These techniques have enabled spectroscopic systems to achieve clinically useful accuracy.
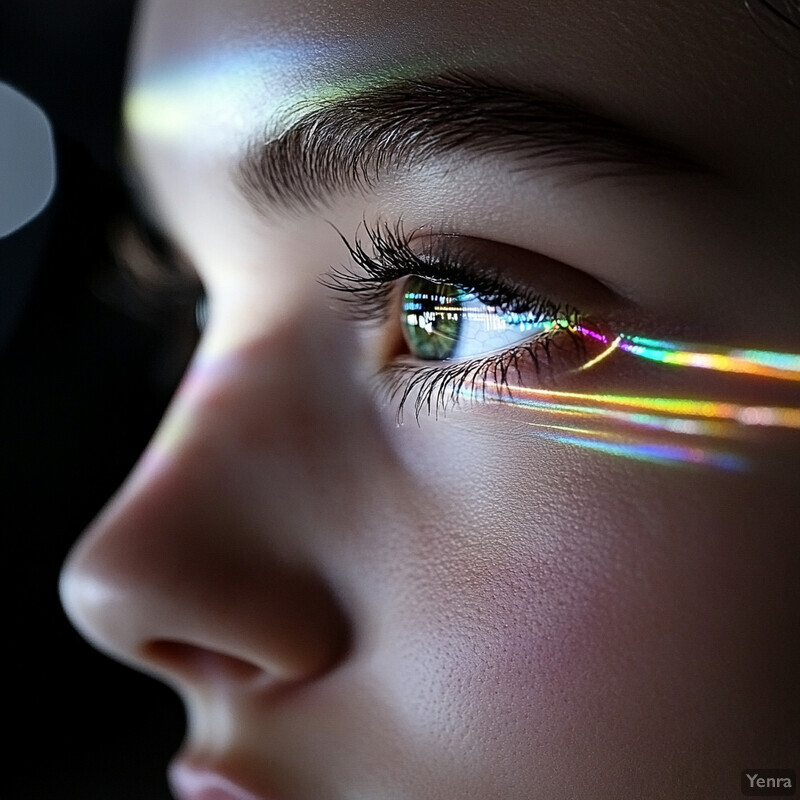
Deep learning has improved spectral analysis in glucose sensing. Zohuri (2025) notes that convolutional and recurrent neural networks “enhance spectral analysis by detecting subtle changes in glucose-induced optical absorption and scattering patterns”. In practice, this translates to accurate glucose estimation: for example, a Raman spectroscopy device using AI-based calibration achieved a 12.8% mean absolute relative difference (MARD) and placed 100% of readings in Clarke Error Grid zones A and B after brief calibration. These results show that AI-driven feature extraction from NIR/MIR/Raman spectra can isolate glucose signals effectively, leading to reliable non-invasive measurements.
3. Multimodal Sensor Fusion
Combining multiple sensor modalities captures complementary glucose-related signals. For instance, optical and electrical sensors, or PPG along with bioimpedance, measure different aspects of physiology. AI algorithms merge these data streams to form a unified estimate, reducing errors caused by any single sensor. Multimodal fusion accounts for individual differences (e.g. skin tone, tissue properties) and environmental factors. Studies show that fused-sensor systems consistently outperform single-sensor approaches in non-invasive glucose estimation.
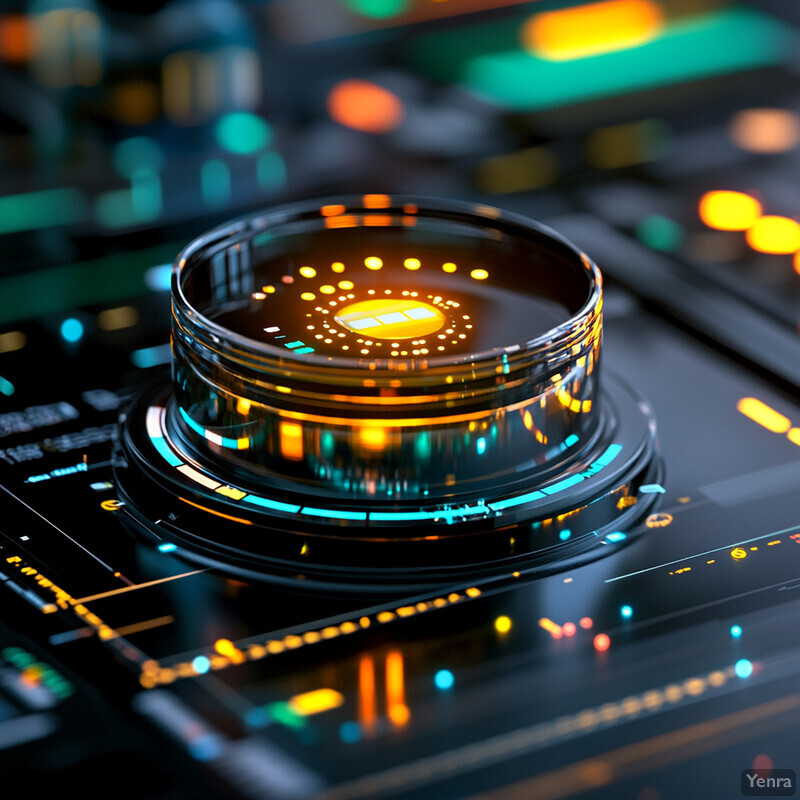
Integrating diverse signals improves accuracy. A review by Sunstrum et al. (2023) found “higher accuracy… when using NIR spectroscopy alongside SpO2 and heartrate in a compact fingertip sensor”. Similarly, combining radio/microwave (RF/mmWave) measurements with NIR light “significantly increase[s] accuracy and sensitivity” in wearable prototypes. Another example: Yen et al. (2020) reported that fusing dual-wavelength PPG with bio-impedance data via a neural network enhanced estimation accuracy. These results indicate that AI-driven sensor fusion, leveraging multiple wavelength and modality inputs, yields more robust glucose monitoring.
4. Machine Learning for Continuous Estimation
AI models can continuously predict glucose levels from streaming sensor data. By training regression or deep learning algorithms on wearable inputs (optical signals, physiological metrics, etc.), these systems output real-time glucose estimates. This replaces or supplements invasive CGM by leveraging contextual data. Continuous models often use multivariate inputs (e.g. circadian rhythms, activity levels) to maintain accuracy over time. Recent demonstrations show that well-trained models on wearable data achieve accuracy approaching that of standard glucose monitors.
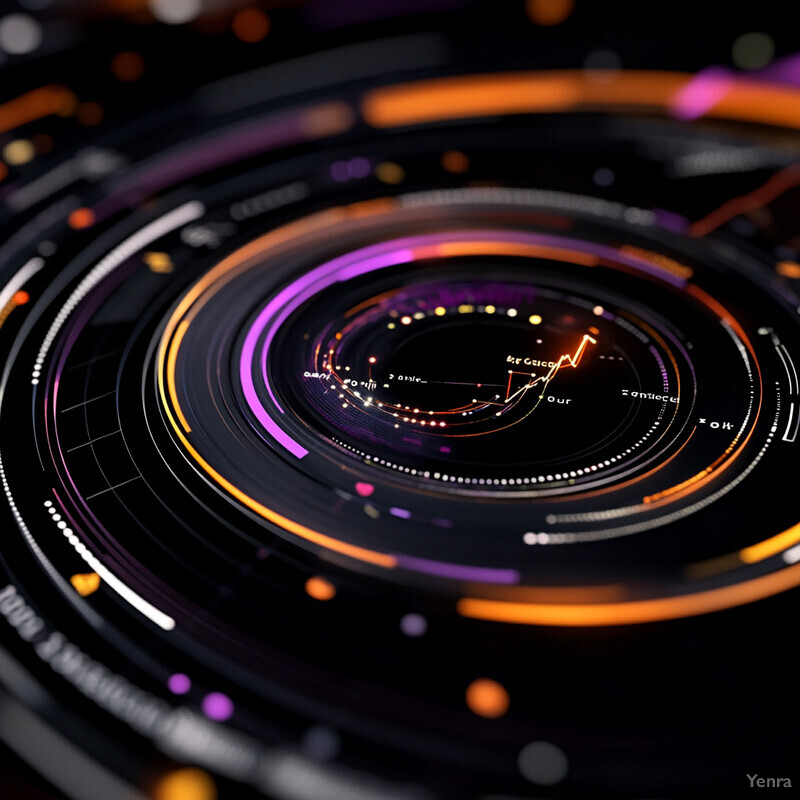
In a real-world study, Liang et al. (2025) built continuous glucose prediction models using only passively collected wearable data. Their XGBoost model achieved an R2=0.73, root-mean-square error 11.9 mg/dL, and a MARD of 7.1%, with 99.4% of predictions in Clarke zones A or B. This indicates that the model’s outputs closely matched actual glucose. The features used included physiological and behavioral data. These results underscore that machine learning can produce reliable continuous glucose estimates from non-invasive sensor streams.
5. Personalized Calibration Models
AI enables models to be tailored to individual physiology, reducing systematic error. Rather than using a one-size-fits-all approach, machine learning models adjust their parameters or inputs based on each user’s characteristics (age, skin properties, baseline metabolism). Personalized calibration can occur at model-training time or continuously during use. By accounting for personal factors, AI models improve long-term stability and accuracy of non-invasive readings for each person.
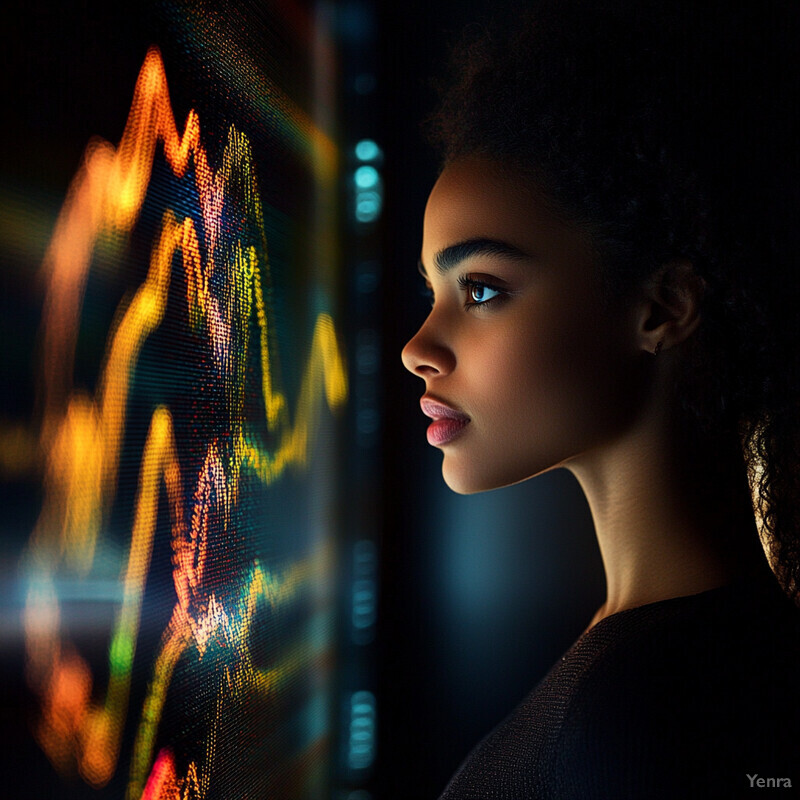
Zohuri (2025) notes that AI-driven predictive modeling “calibrat[es] devices to a user’s specific physiological characteristics, reducing errors and improving accuracy”. In practice, Liang et al. (2025) found that including features like biological sex, circadian information, and electrodermal activity significantly enhanced model performance. Their AI model effectively leveraged these personal predictors to adjust glucose estimates. Together, these findings show that incorporating individual calibration via AI leads to more accurate non-invasive glucose monitoring.
6. Predictive Modeling of Glucose Trends
AI methods can forecast glucose fluctuations without needing constant invasive measurements. Temporal models (e.g. LSTM networks) learn long-term patterns linking lifestyle factors to glucose changes. These models use historical data and contextual inputs to predict near-term trends. By learning from many users or universal datasets, they can also generalize to new individuals. Such predictive analytics allow anticipating glucose rises or falls, improving proactive management.
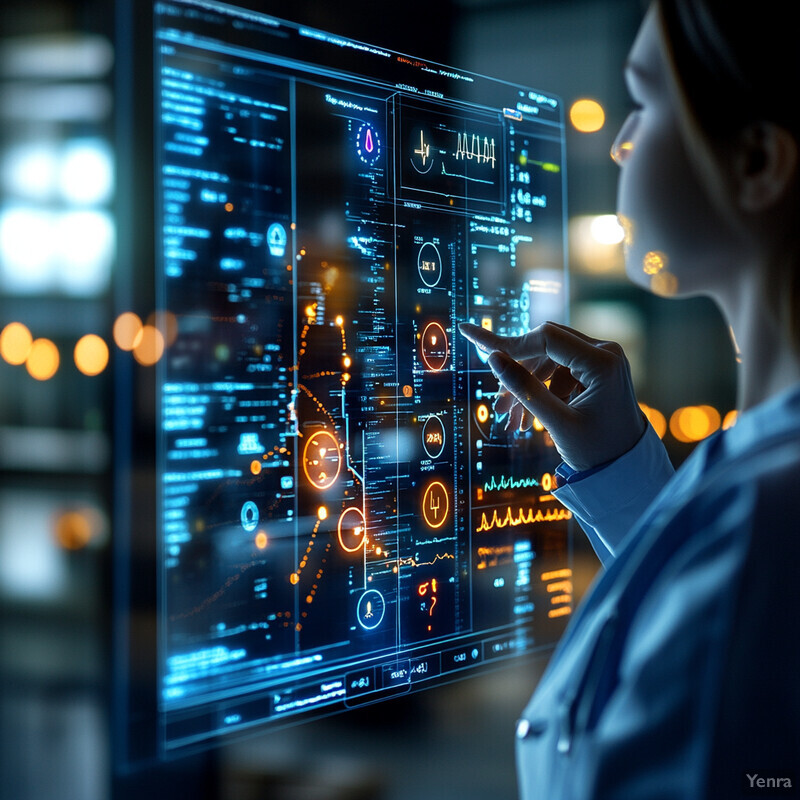
Recurrent neural networks like LSTMs are effective at capturing glucose dynamics. Lim et al. (2024) describe that “LSTMs can learn long-term dependencies… making them capable of capturing the complex relationships between lifestyle factors and glucose fluctuations over time”. This ability allows the model to forecast current and future glucose levels. Indeed, their framework achieved strong predictive accuracy (e.g. low RMSE) for continuous glucose without relying on real-time blood input. These results confirm that AI-driven time-series models can predict glucose trends from contextual data.
7. Context-Aware Analysis
AI models incorporate contextual data (like diet, exercise, stress) alongside sensor inputs. By considering factors such as meal timing, physical activity, and circadian rhythms, models better interpret the glucose signals. For example, time-of-day patterns or concurrent sensor readings (EDA, motion) provide context that impacts glucose. Context-aware analysis allows the system to distinguish glucose-related changes from unrelated fluctuations, improving overall accuracy.
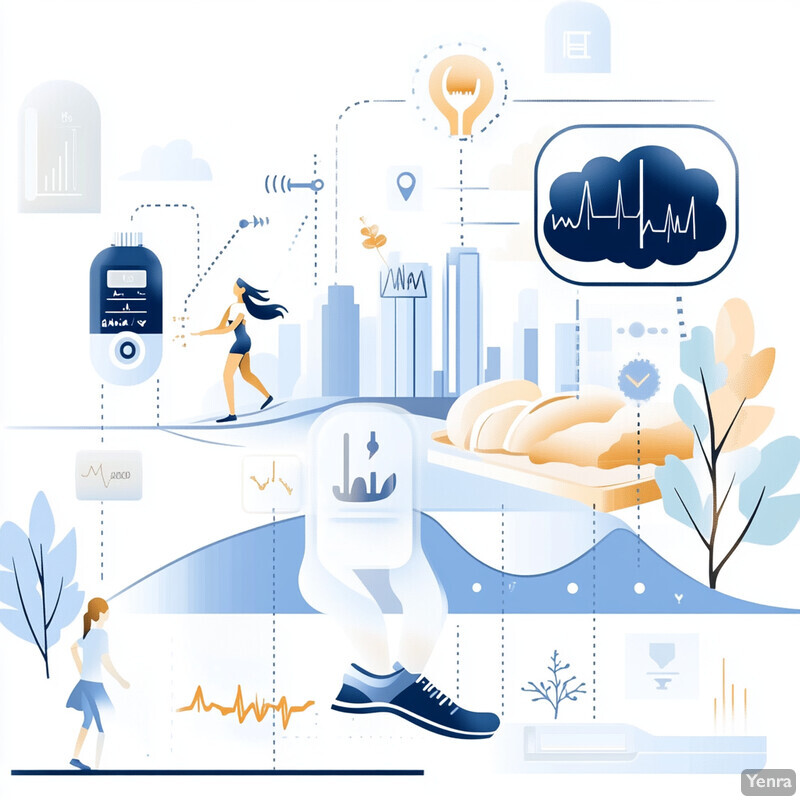
Contextual features have been shown to be strong predictors. Liang et al. found that “circadian rhythm, behavioral features, and tonic features of electrodermal activity (EDA) emerged as key predictors of glucose levels” in their model. This implies that including time-of-day and stress-related signals helped the algorithm. Similarly, Lim et al. emphasize using “life-log data such as food intake and physical activities” to predict glucose. By leveraging these diverse data streams, AI models can capture how context influences glycemia. As a result, predictions become more reliable in variable daily conditions.
8. Transfer Learning for Small Datasets
Transfer learning allows leveraging pre-trained models to bootstrap new ones, which is vital when patient data are scarce. An AI model can be trained on a large general dataset (or on data from many users) and then fine-tuned to a new individual using limited data. This significantly reduces training time and data needs. By sharing learned representations, transfer learning helps maintain accuracy even for novel users or devices with small datasets.
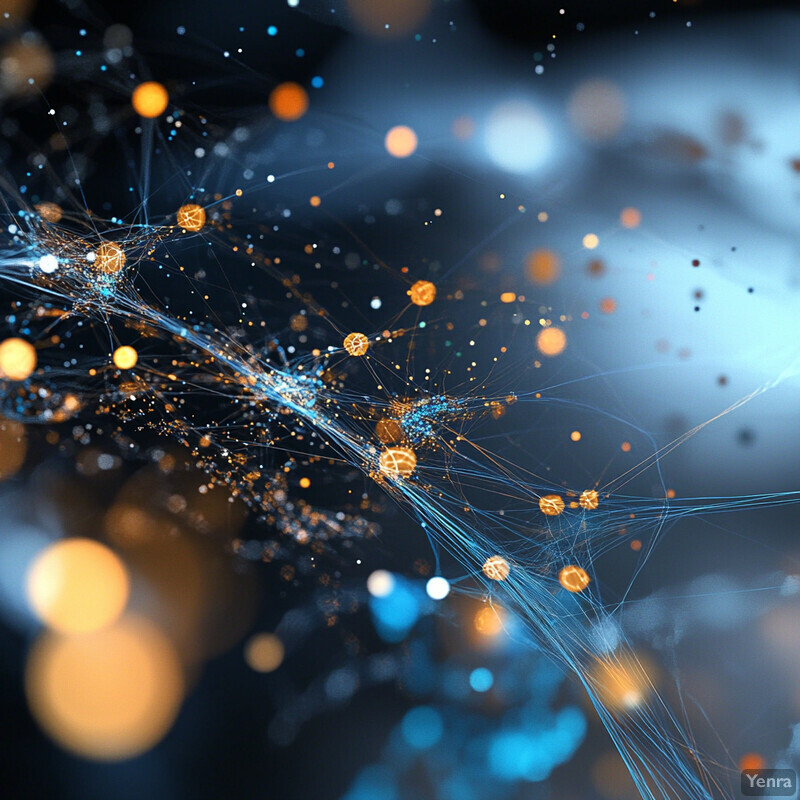
Lim et al. implemented a transfer learning approach for glucose monitoring. They trained a ‘universal model’ on aggregated data and then fine-tuned it on each subject’s specific data. This two-stage training “achieved significant improvements in glucose prediction accuracy across multiple evaluation metrics”. The personalized model outperformed models trained from scratch on small individual datasets. This demonstrates that initializing AI models with pre-trained weights (transfer learning) enables high performance without needing large subject-specific data.
9. Reducing Motion Artifacts
Specialized filtering and AI techniques reduce motion-induced errors in non-invasive readings. When a user moves, optical sensors like PPG can be disturbed. AI models can be trained to recognize and subtract these artifacts. By combining hardware filtering with model-based noise cancellation, systems achieve more stable readings even during everyday activities. Effective artifact reduction means users need not remain completely still for accurate measurement.
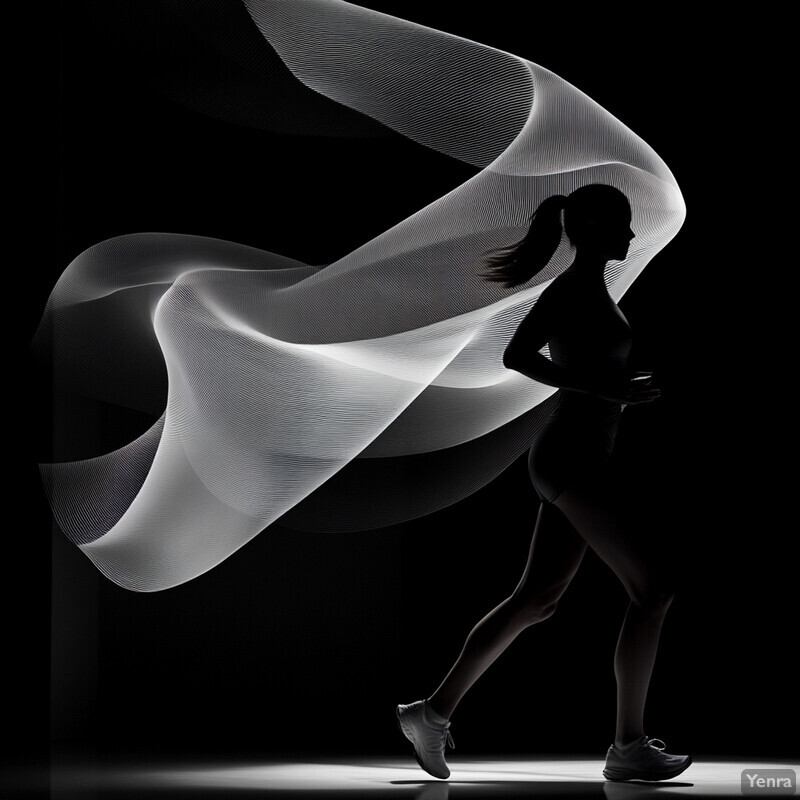
As noted earlier, ML-based filtering effectively suppresses motion noise. In practice, Zeynali et al. used a Butterworth bandpass filter on the PPG signal, explicitly to “eliminate undesired noise and artifacts”. The cleaned signal retained the relevant physiological frequencies for glucose estimation. This preprocessing step, combined with deep learning, significantly improved the signal-to-noise ratio. Thus, state-of-the-art algorithms and filters together mitigate the impact of motion on non-invasive glucose data.
10. Improved Sensor Design Insights
AI-driven analysis informs sensor design by identifying optimal parameters (e.g. wavelengths, materials, geometry). Modeling and optimization tools allow rapid exploration of design alternatives. This leads to sensors that are more sensitive and specific to glucose. For example, choosing specific microwave frequencies or optical bands can be guided by simulations that incorporate AI to predict performance. These insights help engineers build better hardware for non-invasive monitoring.
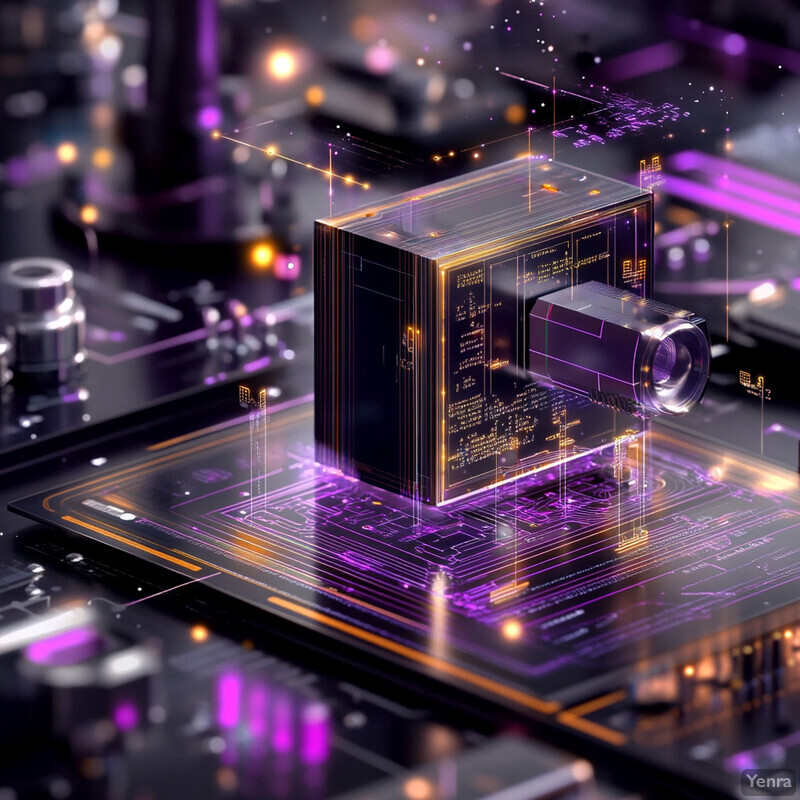
In one study, Farouk et al. used AI-enabled design to create a novel dual-band microwave sensor. Their filter included three split-ring resonators tuned to 2.45 GHz and 5.2 GHz. This design is “improved sensitivity, compact [and] high-quality factor” for glucose sensing. The dual-band approach targets frequencies where glucose changes have distinct dielectric signatures, providing multiple redundant data points. Such AI-guided designs (validated by simulation and experiments) demonstrate how analytical tools accelerate the creation of high-performance NIGM sensors.
11. Adaptive Algorithms for Physiological Variability
AI algorithms can adapt in real time to variations in a user’s physiology. For example, changes in hydration, blood flow, or skin condition over the day can affect readings. Adaptive methods monitor these shifts and update the model parameters accordingly. By continuously learning from incoming data, the model maintains accuracy despite physiological changes. This adaptability is crucial for long-term stability of non-invasive monitors.
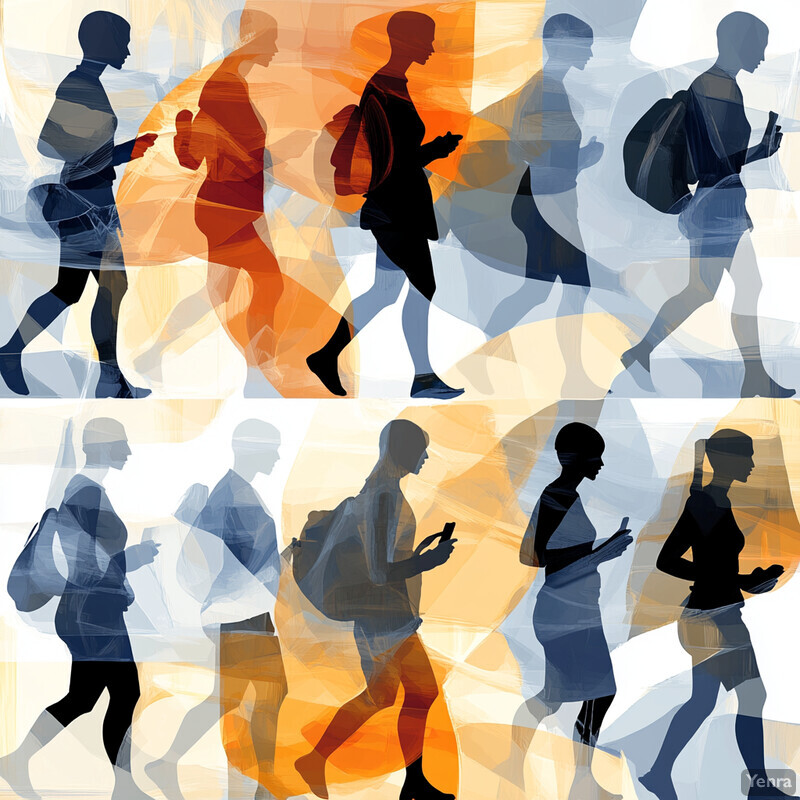
Personalized calibration helps address variability. Zohuri (2025) emphasizes that AI models “reduce errors” by adjusting to the user’s physiology. Practically, this means continually updating the model. Lim et al. (2024) implemented such adaptation: they pretrained a universal model and then fine-tuned it on each user’s data. This personalization “achieved significant improvements” even under unseen conditions. By fine-tuning with new user-specific data, the algorithms remain tuned to individual variability.
12. Feedback Loop for Sensor Performance
AI systems incorporate feedback from ongoing use to refine their models. When new calibration or user data become available, the model is retrained or adjusted. This feedback loop ensures that sensor predictions remain accurate over time. Anomalies can be detected and corrected using fresh data, preventing degradation of performance. Overall, automated feedback-driven learning helps maintain long-term device reliability.
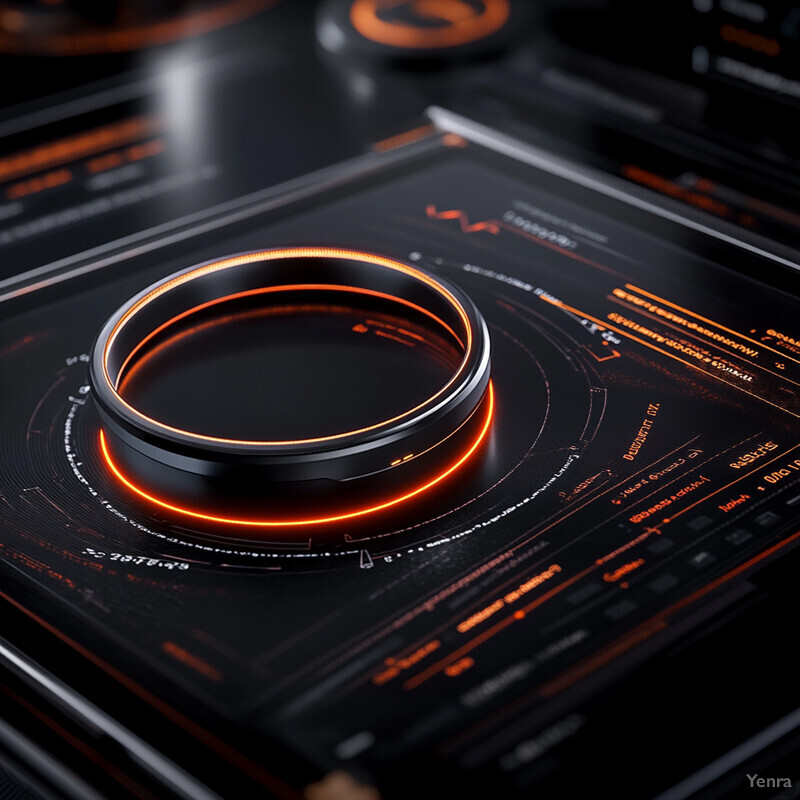
In practice, models are iteratively updated. Pors et al. (2025) reported that refining their pre-trained calibration model with additional patient data “led to improved measurement accuracy, less variability between subjects, and a further reduction in calibration requirement”. In other words, as more data were collected, the AI model automatically updated its parameters to correct any drift. This demonstrates how a feedback loop of new data and model refinement can sustain high sensor performance without manual intervention.
13. Integration With Smartphones and Wearables
AI enables seamless connectivity between glucose sensors and consumer devices. Smartwatches, fitness bands, and mobile apps can receive and process data from non-invasive monitors. On-device machine learning provides real-time analysis and alerts. This integration improves user engagement: for example, smartphones can display trends, send notifications for high/low glucose, or suggest actions. Overall, AI enhances the user interface and accessibility of monitoring.
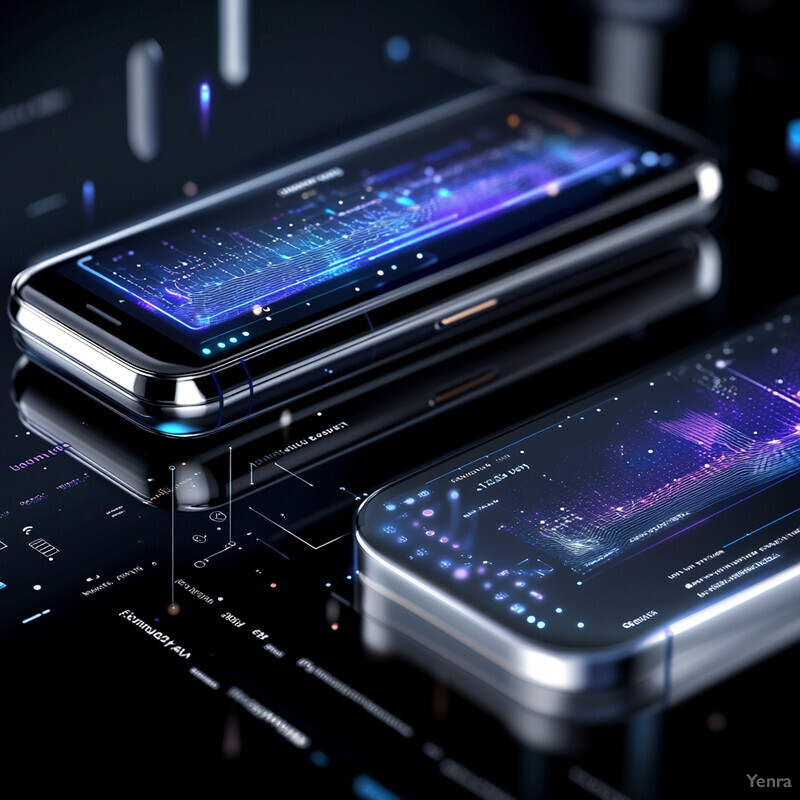
According to Zohuri (2025), non-invasive glucose devices can be integrated with IoT wearables to provide real-time feedback. Specifically, “AI enables seamless integration… with smartwatches, fitness trackers, and mobile health apps,” allowing systems to “alert users to significant glucose fluctuations”. This shows that built-in AI algorithms can continuously analyze sensor data and push actionable alerts through connected devices. Such integration streamlines the user experience, making non-invasive monitoring practical in daily life.
14. Reducing the Burden of Fingerstick Calibration
AI methods greatly reduce how often invasive calibration is needed. By learning from prior datasets, models can start accurate predictions with minimal user calibrations. This lightens the need for repeated finger-pricks. Advanced algorithms effectively internalize calibration curves, so devices can maintain accuracy without frequent recalibration. Consequently, patients enjoy more convenience and less discomfort.
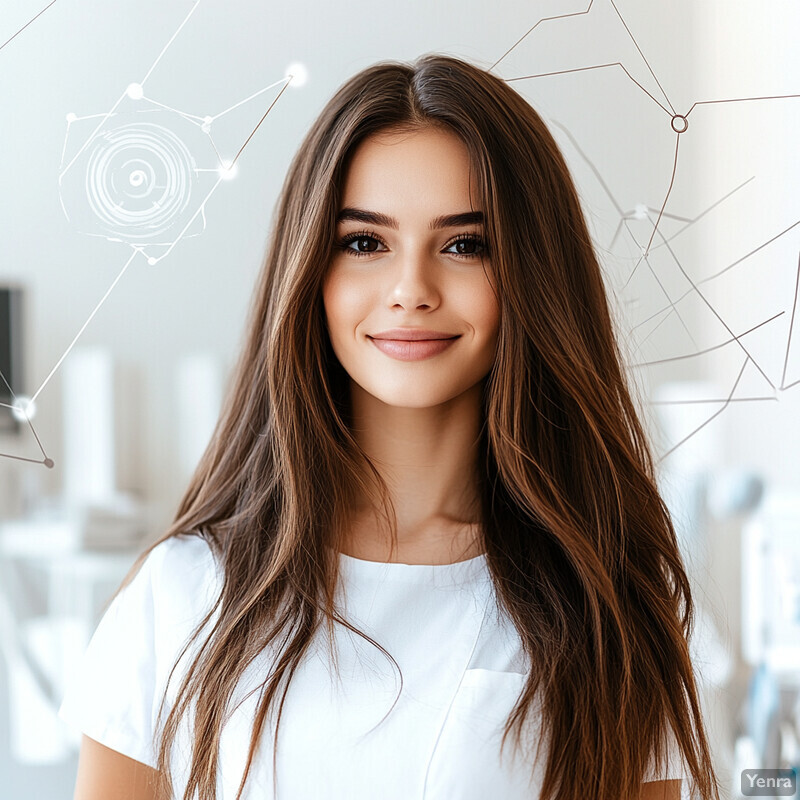
Pors et al. (2025) demonstrated this effect. They used a pre-trained AI model that required only a 4-hour calibration phase of 10 fingerstick measurements. After this brief calibration, the Raman device tracked glucose with a 12.8% MARD and 100% of readings in safe zones. This “practical calibration scheme” shows that with AI assistance, non-invasive monitors can achieve high accuracy from just a few calibration points, approaching a factory-calibrated system.
15. Early Detection of Measurement Drift
AI continuously checks for signs of sensor drift and compensates automatically. If the device’s accuracy begins to degrade (due to wear, contamination, etc.), the model can signal a recalibration or adjust itself. Early detection prevents systematic errors from accumulating. Essentially, the AI monitors its own predictions over time, learning any bias changes and correcting them before they affect the user.
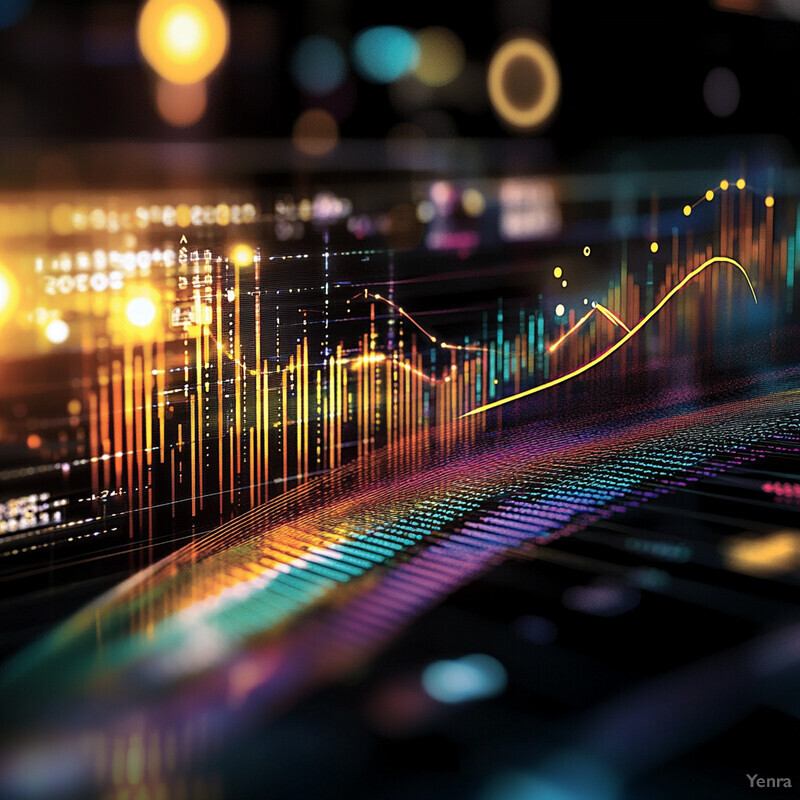
Pors et al. noted that their AI-based calibration could be refined over time to handle drift. They reported that “the pre-trained calibration model can be refined, leading to improved measurement accuracy, less variability between subjects, and a further reduction in calibration requirement”. This means the algorithm detected deviations and updated itself to compensate. Such iterative refinement indicates that AI systems can detect and correct drift early, keeping the glucose readings reliable.
16. Robust Quality Control
AI implements checks to ensure data integrity before making glucose predictions. Algorithms reject or flag poor-quality readings (e.g. due to sensor misplacement or excessive noise). Preprocessing steps like artifact detection, filtering, and signal interpolation are used. By enforcing quality control, the system avoids making clinical decisions on unreliable data. This leads to safer, more trustworthy monitoring.
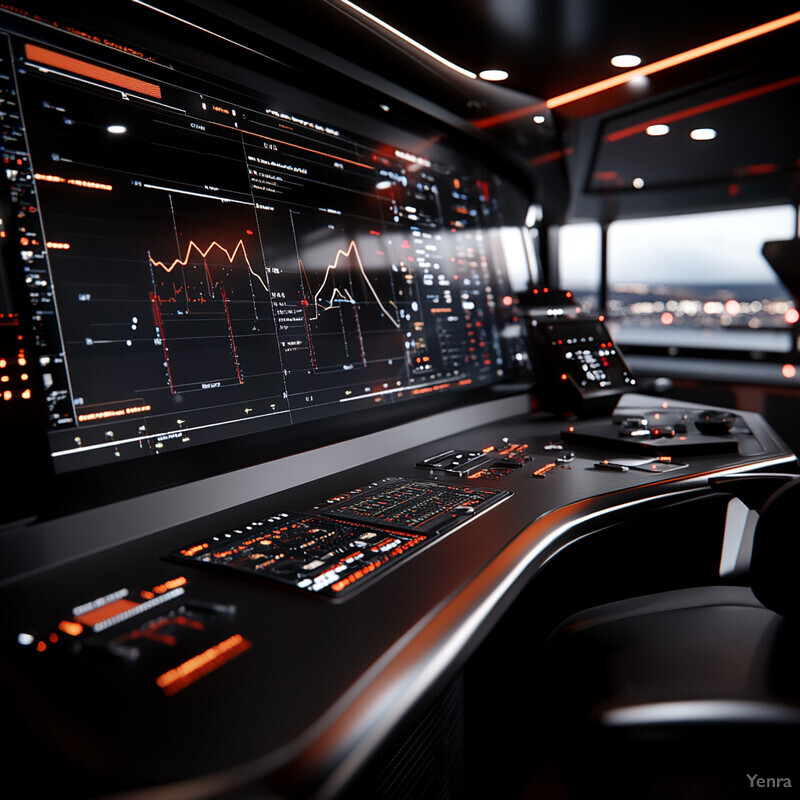
Rigorous data cleaning is critical. In one example, Zeynali et al. applied a Butterworth filter to their PPG data and used a preprocessing toolkit (“Nerukit2”) to remove corrupt segments. They ensured that 8-minute windows with insufficient data were excluded and missing points were filled by interpolation. These measures “ensur[e] data quality and reliability,” as noted in their study. Such AI-driven quality control steps prevent noise or gaps from misleading the glucose model.
17. Integration With Clinical Decision Support
AI-driven glucose data can feed into healthcare systems for decision support. Alerts for hypo/hyperglycemia can be sent to clinicians. Data from non-invasive monitors can be incorporated into electronic health records. AI can also contextualize glucose trends with patient history to aid therapy adjustments. By automating analysis, these tools help clinicians make faster, data-informed treatment decisions.
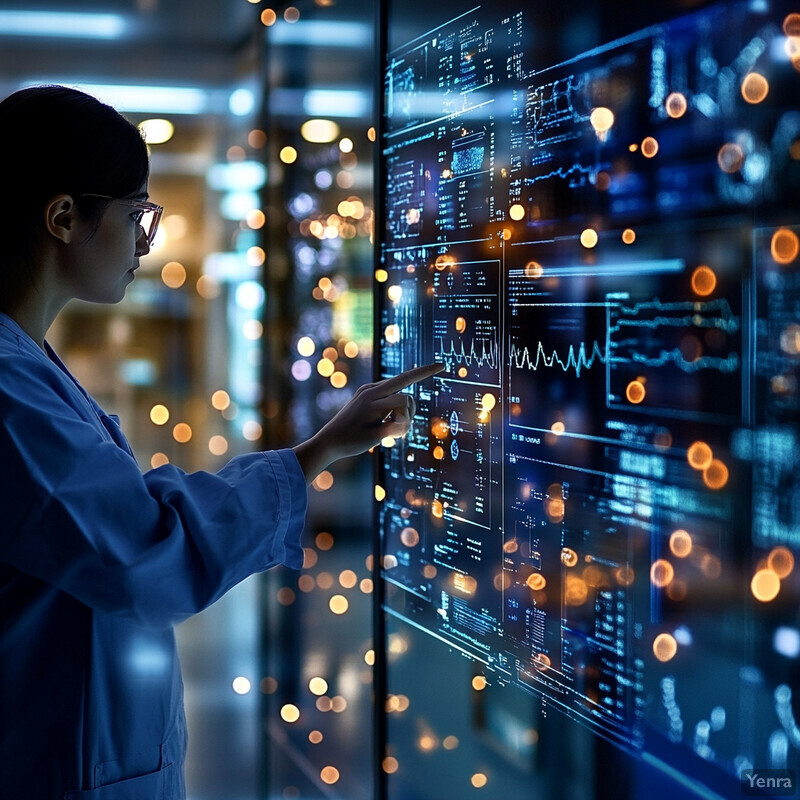
AI-enhanced monitoring is expected to transform diabetes care. Zohuri (2025) notes that “AI-driven advancements in signal analysis [and] predictive modeling… are set to transform diabetes management, making it more accessible, convenient, and effective for millions”. This vision includes real-time analytics that clinicians could use. For instance, integrating continuous non-invasive data with AI could alert a doctor to a patient’s emerging issue before symptoms occur. Thus, AI tools act as a bridge between raw sensor output and actionable clinical insights.
18. Automated Model Updates With New Data
As new measurements are collected, AI models retrain or update without human intervention. This automation ensures the latest data informs the model. Continuous learning pipelines ingest freshly acquired sensor readings to refine predictions. Automation reduces manual re-calibration needs and keeps the model up to date with population-level insights or device changes.
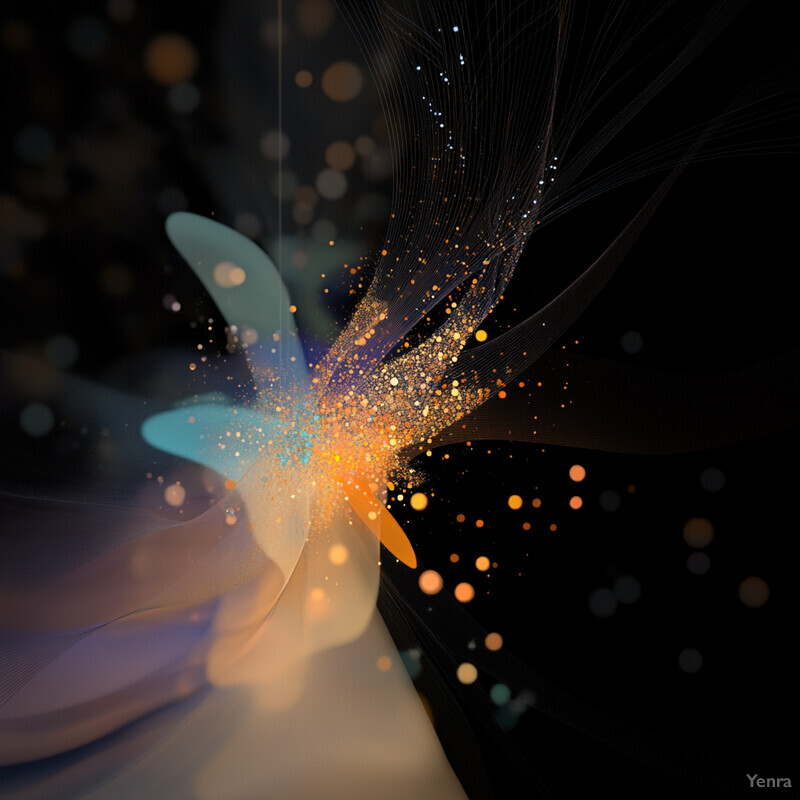
Automated updating has shown clear benefits. Pors et al. (2025) report that by continuously refining the calibration model, they achieved “significant improvements in glucose prediction accuracy across multiple metrics”. The system automatically adjusted its parameters as more patient data became available, improving correlation and reducing error over time. This demonstrates that an auto-update loop — training on new data — enhances model reliability.
19. Improved Usability and User Experience
AI improvements make devices more user-friendly. By reducing calibration and pricks, and by providing clear guidance through apps, the burden on patients decreases. Smart algorithms can summarize complex trends into simple visualizations or alerts. The convenience of using everyday devices (smartphones, watches) with AI support makes monitoring less intrusive. Overall, patients benefit from easier, more intuitive diabetes management.
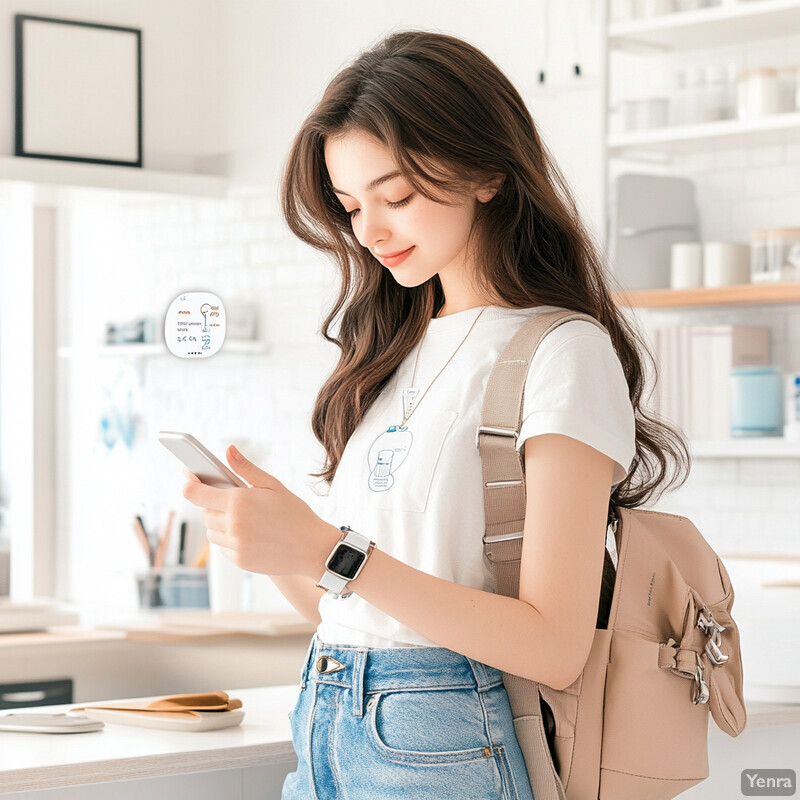
Enhanced usability is a key AI benefit. Zohuri (2025) emphasizes that AI-enabled monitoring will be “accessible, convenient, and effective”. This refers to features like wireless connectivity and automatic analysis that remove manual steps. For example, an AI-enhanced device might calibrate itself and notify the user only when necessary. The cited companies (Abbott, DexCom, etc.) are already pushing toward integrating non-invasive sensors with apps. These developments highlight how AI reduces effort (fewer fingersticks, automated alerts) and improves the patient experience.
20. Accelerated Research and Development Cycle
AI tools speed up R&D by enabling rapid prototyping and simulation. Virtual testing of designs (using AI models or simulations) can identify promising approaches before hardware fabrication. Machine learning helps analyze large experimental datasets quickly. As a result, research cycles shorten. Models can suggest optimal parameters, conduct parameter sweeps virtually, and identify failure modes, accelerating innovation in non-invasive technologies.
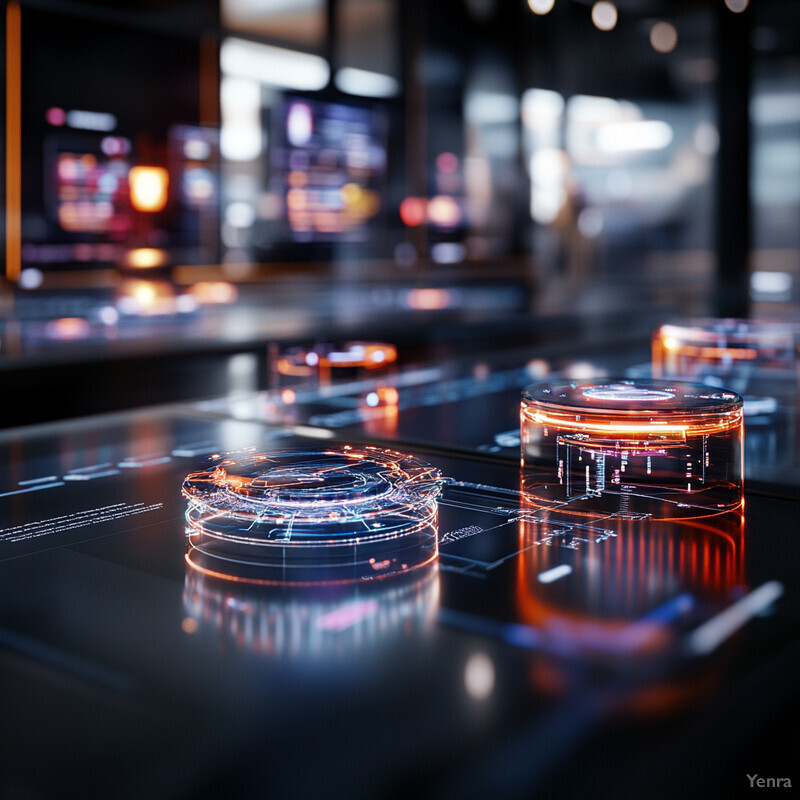
Incorporating AI dramatically shortens development time. Farouk et al. (2025) used simulation and ML to iterate on sensor design quickly, validating a complex microwave filter in software before physical tests. Zohuri (2025) notes that such AI-driven innovation has companies racing to market: “non-invasive glucose monitoring is at an exciting juncture” with AI making advances faster. Together, these examples show that AI accelerates hypothesis testing and prototype evaluation, thus hastening the overall R&D cycle for glucose monitoring devices.