1. Automated Image Segmentation
AI-driven algorithms now automatically segment brain structures in MRI and electron microscopy images, drastically reducing the manual labor of delineating complex anatomy. These tools identify regions like gray matter, white matter, or even individual cells, far faster than human experts. Automating segmentation improves consistency and objectivity, eliminating variability between human raters. Researchers can obtain high-quality, labeled brain images in a fraction of the time, freeing them to focus on interpreting results instead of painstakingly outlining structures. Overall, AI segmentation pipelines have made large-scale brain mapping studies more feasible by handling the initial heavy lifting of image annotation.
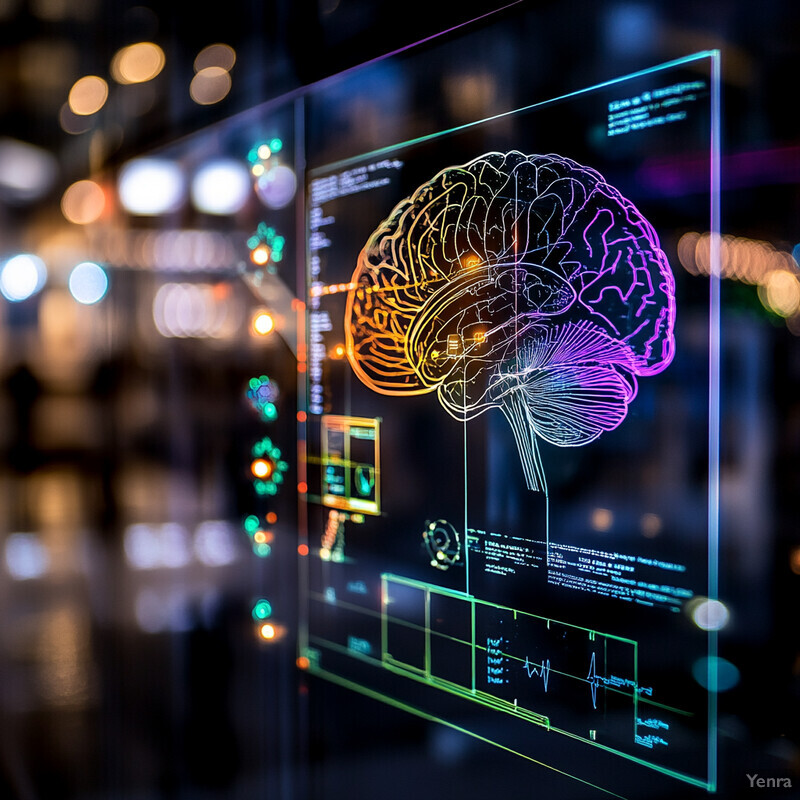
Deep learning methods achieve near-human or superior accuracy in segmenting brain images while greatly accelerating the process. For example, a deep learning U-Net approach significantly improved the speed of MRI tumor segmentation and the accuracy of distinguishing abnormal from normal tissue compared to prior techniques. In connectomics, 3D electron microscopy volumes can now be segmented by convolutional neural networks with exceptional accuracy, reconstructing neurons and synapses at scale—although some manual proofreading remains needed for perfection. A 2023 study reported that automated segmentation yielded highly accurate neuron reconstructions from millimeter-scale EM data, containing rich morphological detail, but required minimal human intervention for error correction. Such advances demonstrate that AI can perform segmentation tasks in hours that once took experts weeks, without loss of quality.
2. High-Resolution Connectome Reconstruction
AI enables the reconstruction of neural circuits at unprecedented resolution by integrating massive imaging datasets. Deep learning models can trace neurons and synapses through thousands of electron microscopy slices, assembling high-resolution “wiring diagrams” of the brain’s connectome. This has allowed neuroscientists to map complete neural networks, even entire insect brains, at synaptic detail. The resulting connectomes reveal how neurons are wired together, which was previously infeasible to chart fully by hand. By automating pattern recognition in terabyte-scale image stacks, AI dramatically speeds up connectome projects and captures fine details like individual synaptic contacts. These advances help researchers understand brain circuitry and information flow with unparalleled completeness.
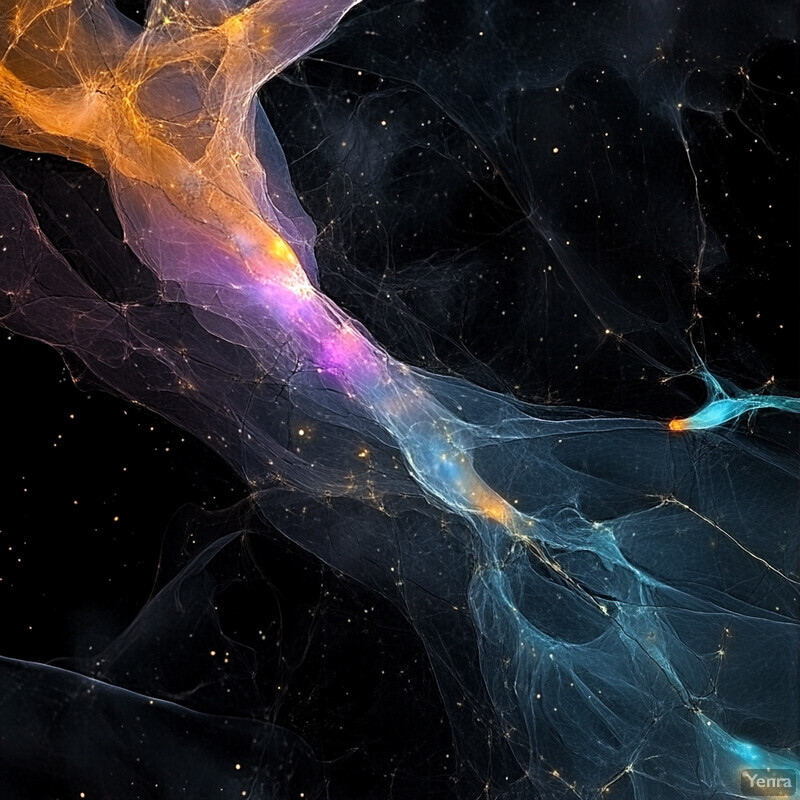
In 2024, an international team used AI-assisted methods to reconstruct the first complete synapse-level connectome of an adult fruit fly brain, mapping roughly 140,000 neurons and 50 million synapses. This feat, published in Nature, was made possible by machine-learning pipelines that traced neuronal processes through petabytes of EM data, something impossible without automation. The resulting fly brain map – the most complex whole-brain connectome to date – demonstrates how AI can handle extraordinary imaging scale and complexity. Similarly, AI tools have been vital in large projects like the Human Connectome, accelerating the integration of multimodal imaging and enabling comparisons across hundreds of brains. By leveraging deep learning, researchers have reconstructed neural wiring with high accuracy and completeness, achieving a milestone in neuroscience.
3. Accelerated Image Processing
AI methods (often using GPU acceleration and specialized neural network architectures) can process neuroscience images dramatically faster than traditional pipelines. High-throughput AI algorithms shorten the time needed to align, filter, and analyze large brain imaging datasets from weeks or months to days or hours. This speed-up makes it practical to conduct population-level brain mapping studies that compare hundreds or thousands of brains. By efficiently handling terabyte-scale imaging data, AI-powered processing enables researchers to extract meaningful patterns (like connectivity matrices or cell counts) at a scale that would overwhelm conventional software. Faster processing also means quicker turnaround between data acquisition and scientific insight, accelerating the pace of discovery in brain mapping.
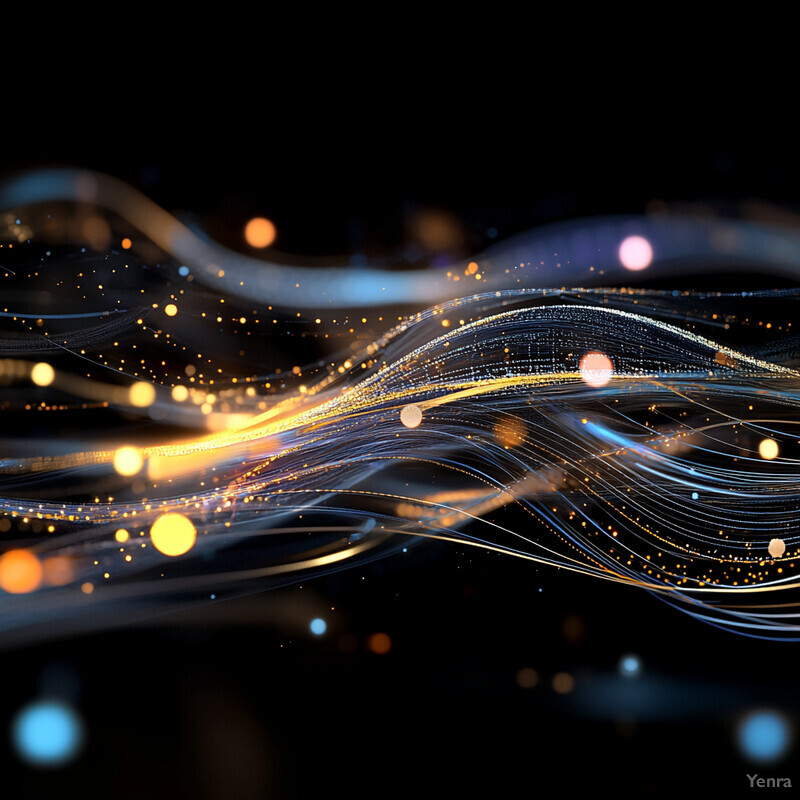
A 2025 study introduced DeepPrep, a deep learning pipeline that preprocesses MRI scans ten times faster than the standard workflow while maintaining robust accuracy. Tested on over 55,000 brain images, DeepPrep’s neural-network modules (for tasks like skull stripping, surface reconstruction, and normalization) reduced processing time per scan from hours to minutes. This massive acceleration meets the scalability needs of projects like UK Biobank, which involves tens of thousands of MRIs. Likewise, in electron microscopy, AI-guided imaging systems such as SmartEM have cut data acquisition time from weeks to days by directing microscopes intelligently. Overall, by leveraging GPUs and optimized architectures, AI pipelines compress formerly intractable computations into manageable timeframes, enabling timely analysis of big neuroimaging data.
4. Improved Noise Reduction
AI-based denoising algorithms enhance signal quality in many brain imaging modalities—such as EEG, MEG, and fMRI—by more effectively separating true neural signals from noise. Machine learning models trained on large datasets can recognize and remove artifacts like head motion, electrical interference, or scanner noise better than traditional filters. By boosting the signal-to-noise ratio, these AI tools allow researchers to detect subtle brain activity patterns or connectivity that would otherwise be obscured. Cleaner data improves the reliability of downstream analyses, whether it’s identifying tiny EEG spikes or faint fMRI connectivity changes. Overall, AI-driven noise reduction yields clearer brain maps and more accurate interpretations of neural data.
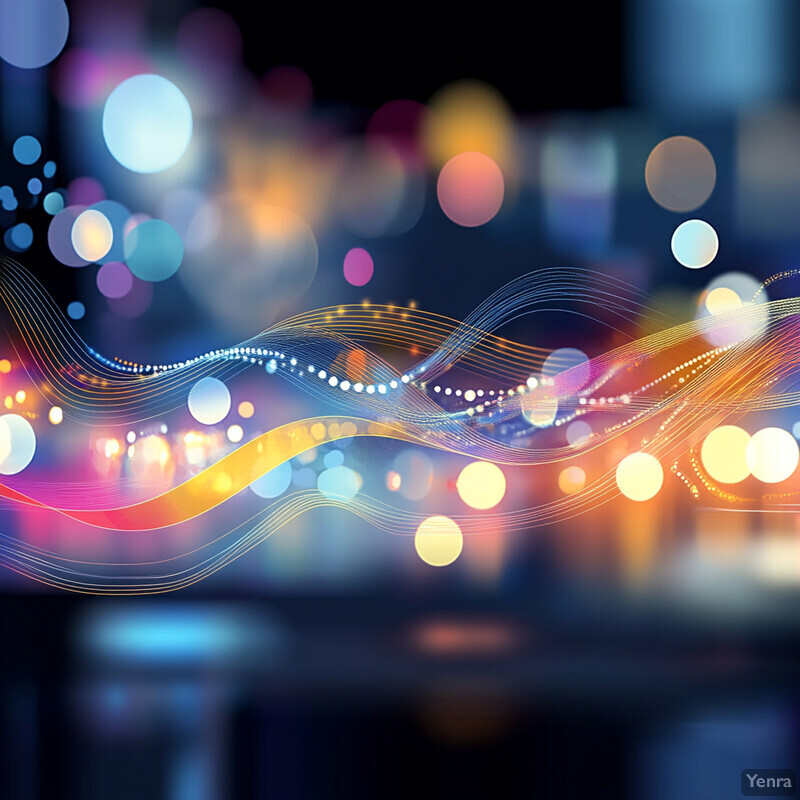
Deep learning approaches now outperform conventional methods in removing artifacts from brain signals. For example, transformer-based neural networks have been used to clean EEG recordings, successfully eliminating muscle and eye-blink artifacts that confound analysis. A 2024 model called ART (Artifact Removal Transformer) demonstrated superior performance to prior algorithms, significantly boosting EEG signal-to-noise ratios and preserving fine neural details after artifact removal. Likewise, for fMRI data, generative AI methods like DeepCor have been applied to denoise functional images: a 2023 preprint showed that DeepCor outperformed a standard method (CompCor) in reducing fMRI noise and even enhanced the differentiation of brain network connections in real datasets. These advances highlight how AI-driven filtering can reveal faint brain signals that previously went undetected.
5. Multi-Modal Integration
AI models can fuse data from multiple brain imaging modalities—such as structural MRI, functional MRI (fMRI), diffusion tensor imaging (DTI), and PET scans—into unified, richer brain maps. By combining the complementary information from each modality (structure, function, connectivity, metabolism, etc.), the integrated maps give a more holistic view of the brain. This integration is challenging due to differences in data scale and format, but AI excels at finding correspondences across modalities. The resulting multi-modal brain maps reveal relationships that might be missed when modalities are analyzed in isolation, such as how a structural pathway supports a functional network. Overall, AI-driven data fusion provides a more complete picture of brain organization and can improve diagnostic or research insights.
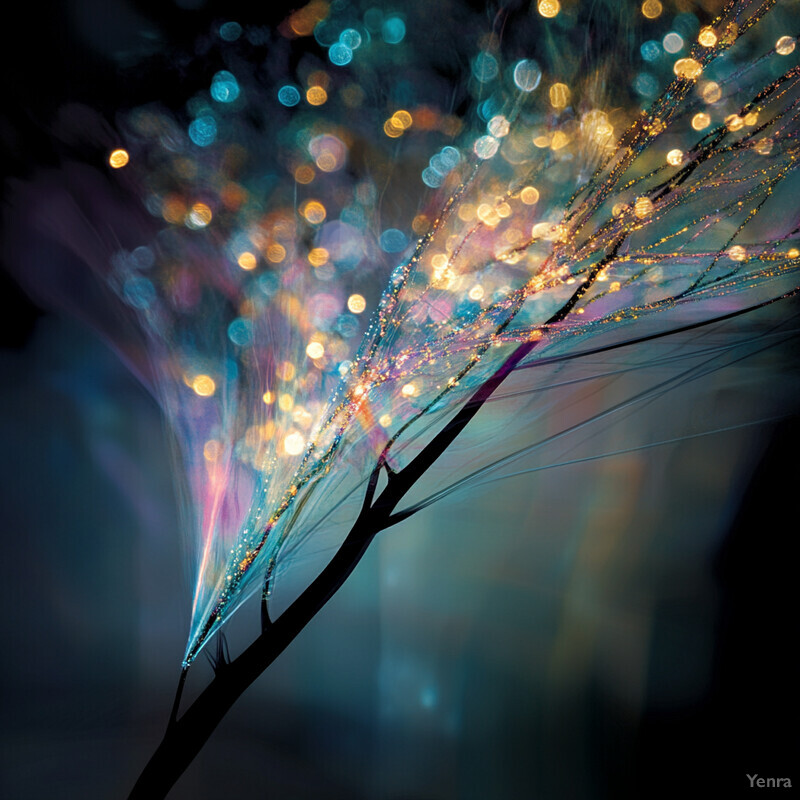
Advanced AI frameworks (often using deep neural networks or graph models) are enabling seamless integration of heterogeneous neuroimaging data. A recent approach combined fMRI (functional connectivity), DTI (structural connectivity), and sMRI (anatomical features) within a graph neural network, yielding a comprehensive model of brain connectivity and anatomy. In tests on the Human Connectome Project dataset, this multi-modal AI model achieved improved accuracy in predicting cognitive outcomes, demonstrating the power of fused data. Generally, studies report that multi-modal deep learning improves brain disorder classification and biomarker discovery: for example, integrating MRI and PET has helped identify Alzheimer’s disease patterns that were not evident in single-modality analysis. These successes underscore that AI can handle the complexity of multi-modal brain mapping, leveraging structural, functional, and metabolic cues together for deeper insights.
6. Automated Brain Parcellation
AI algorithms can automatically partition the brain into distinct regions or “parcels” (whether anatomical or functional) with greater accuracy and consistency than traditional atlas-based methods. In the past, researchers relied on standardized atlases or manual delineation to parcellate brains, which often failed to capture individual variations. Deep learning-based parcellation learns subtle imaging features that indicate region boundaries, resulting in finer and more personalized brain maps. These AI-derived parcellations can adapt to each person’s unique anatomy or functional organization, improving the resolution of brain mapping. Automated parcellation not only saves immense time but also yields reproducible region definitions that can be crucial for comparing subjects or tracking changes over time.
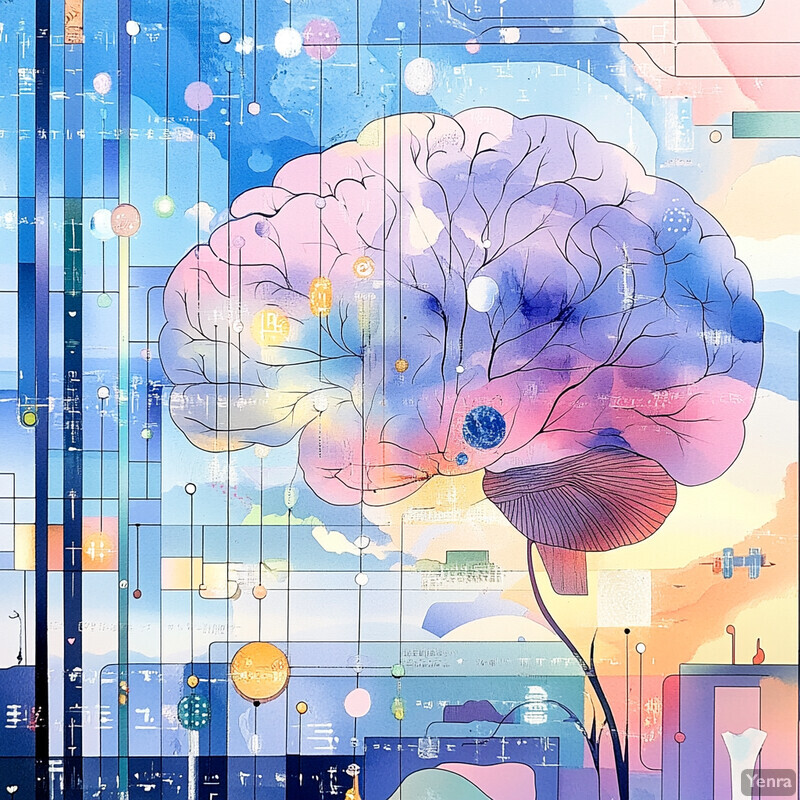
Modern deep learning methods have dramatically improved brain parcellation. For instance, OpenMAP-T1 is a 2024 AI pipeline that segments a whole brain MRI into 280 anatomical regions in under 90 seconds per scan, whereas older multi-atlas approaches took hours. OpenMAP-T1’s convolutional networks achieved this speedup without sacrificing accuracy, proving robust across diverse MRI datasets and even handling cases (e.g. head motion or pathology) that confound atlas-based tools. Likewise, researchers have developed individualized parcellation techniques: Li et al. (2023) and Ma et al. (2024) showed that neural networks can learn subject-specific parcels from training data, successfully producing personalized brain region maps for each new individual. These AI-driven approaches outperform one-size-fits-all atlases by accounting for fine-grained anatomical differences, thus providing a higher-resolution and more faithful segmentation of brain structures.
7. Predictive Modeling of Brain Functions
AI is helping neuroscientists predict how neural circuits will behave under certain conditions, effectively translating static brain maps into dynamic functional predictions. By training on data linking brain structure to activity, machine learning models can forecast neural responses to stimuli or perturbations. This allows researchers to infer functionality from a wiring diagram: for example, predicting which brain regions will activate during a task based on connectivity. Such predictive modeling can also simulate how circuit changes (like a cut connection or neuron loss) might affect behavior or cognition. Ultimately, AI-driven predictive models bridge the gap between anatomy and function, providing a testing ground for hypotheses about how brain structure gives rise to neural dynamics.
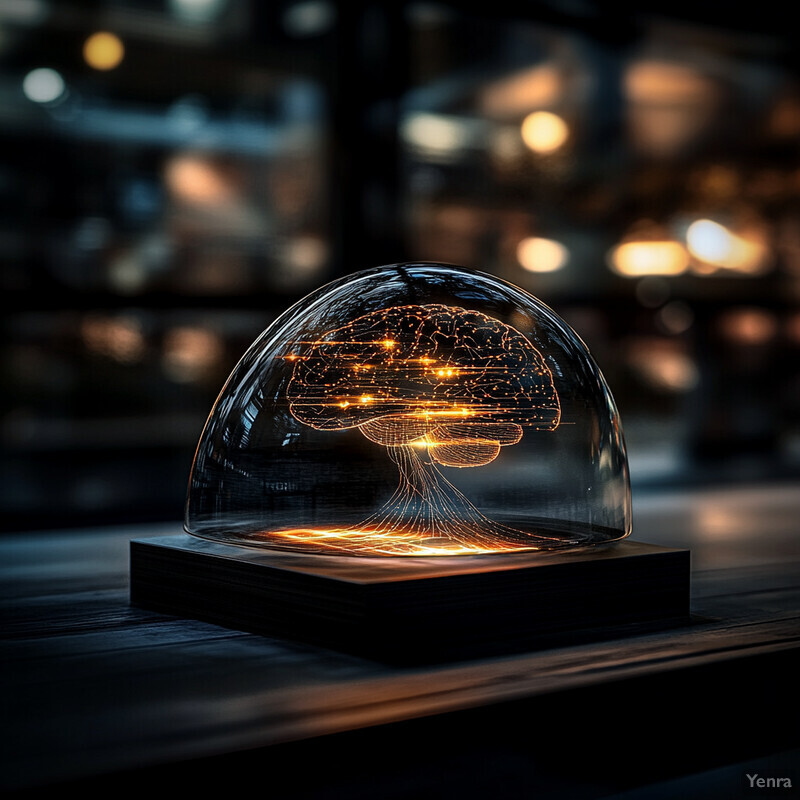
A striking example comes from connectomics: in 2024, scientists showed that combining a detailed fly brain connectome with machine learning enabled accurate prediction of neural activity in that circuit. Using an AI “world model” constrained by the fly’s wiring diagram, they could simulate the fly’s visual system responses with high fidelity. This Nature study demonstrated that connectivity data alone, when paired with learning algorithms, can yield surprisingly precise functional predictions. In human neuroimaging, deep networks have been trained to predict task-evoked fMRI activation from resting-state connectivity patterns. These models significantly outperform traditional statistical approaches, capturing complex nonlinear brain dynamics (e.g., a transformer-based model predicted individualized task fMRI maps from resting scans). Such advances illustrate how AI can turn static brain maps into predictive engines, offering a powerful tool to explore and validate theories of brain function.
8. Advanced Feature Extraction
AI methods, especially deep learning, can automatically extract complex features from brain imaging data that human observers might overlook. These features could be subtle morphological details (like dendritic spine shapes, cortical micro-fold patterns) or latent connectomic motifs (patterns of network connectivity). By learning directly from raw data, deep networks often identify high-dimensional or faint “signatures” that correlate with biological or clinical variables. These extracted features can serve as new biomarkers—for example, a hidden shape pattern in hippocampal neurons associated with epilepsy—or be used as inputs to other models. In essence, AI augments human insight by revealing intricate structures and relationships embedded in brain data, thus deepening our understanding of brain organization.
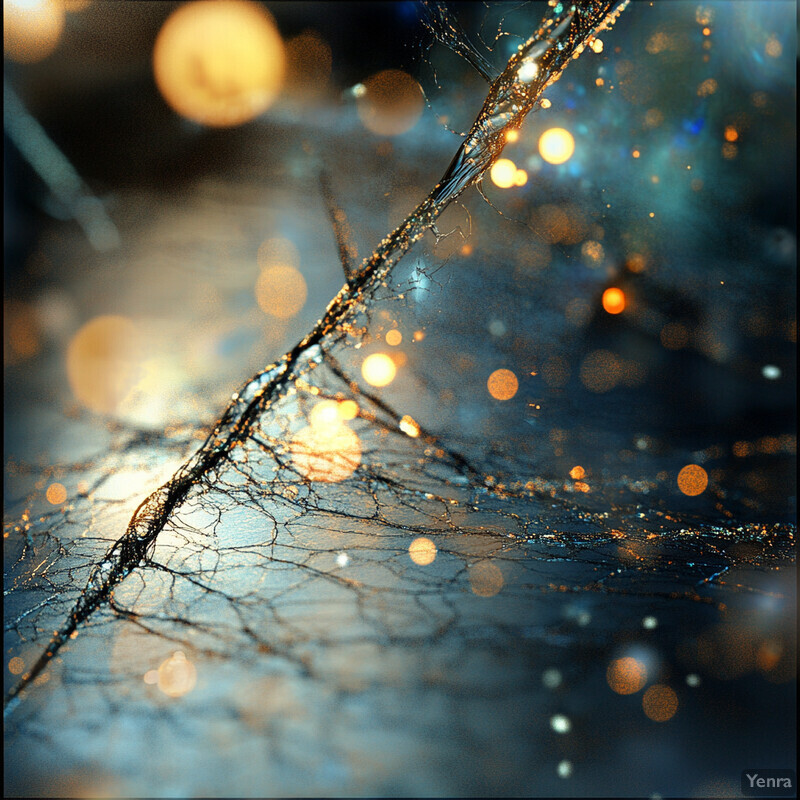
In connectomics, AI-driven analysis is extracting rich morphological and network features from neural circuit reconstructions. A 2023 pipeline (NEURD) converted the complex 3D meshes of neurons from a millimeter-scale EM dataset into graph representations, then automatically quantified features like axon diameters, branching patterns, and even the density of dendritic spines on each neuron. This allowed researchers to catalog fine microcircuit properties (e.g. distributions of spine sizes and neuron types) across the whole dataset in a standardized way. In neuroimaging, unsupervised deep learning models have identified latent dimensions in fMRI data that align with cognitive states or disease traits which were not apparent via traditional analysis. For instance, an unsupervised deep model found a novel pattern of brain connectivity that correlated with schizophrenia symptom severity, suggesting a new phenotype for further study. These examples illustrate AI’s power to unveil hidden structure in brain data, generating new variables and hypotheses for neuroscience.
9. Early Biomarker Detection
AI models can detect extremely subtle patterns in brain scans that serve as early biomarkers of neurological and psychiatric conditions (e.g., Alzheimer’s, Parkinson’s, schizophrenia) – often before overt symptoms arise. By training on large datasets of patients and healthy controls, AI learns to recognize minute changes in brain structure or connectivity that human radiologists might deem within normal variation. These can include slight atrophy in specific subregions, faint hypometabolism on PET, or mild disruptions in network connectivity. Identifying such changes early on enables earlier and potentially more effective interventions. In clinical research, AI-discovered biomarkers help stratify patients by risk or disease stage. Thus, AI significantly enhances the sensitivity of brain mapping for prodromal disease changes, offering hope for pre-symptomatic diagnosis and tracking of disease progression.
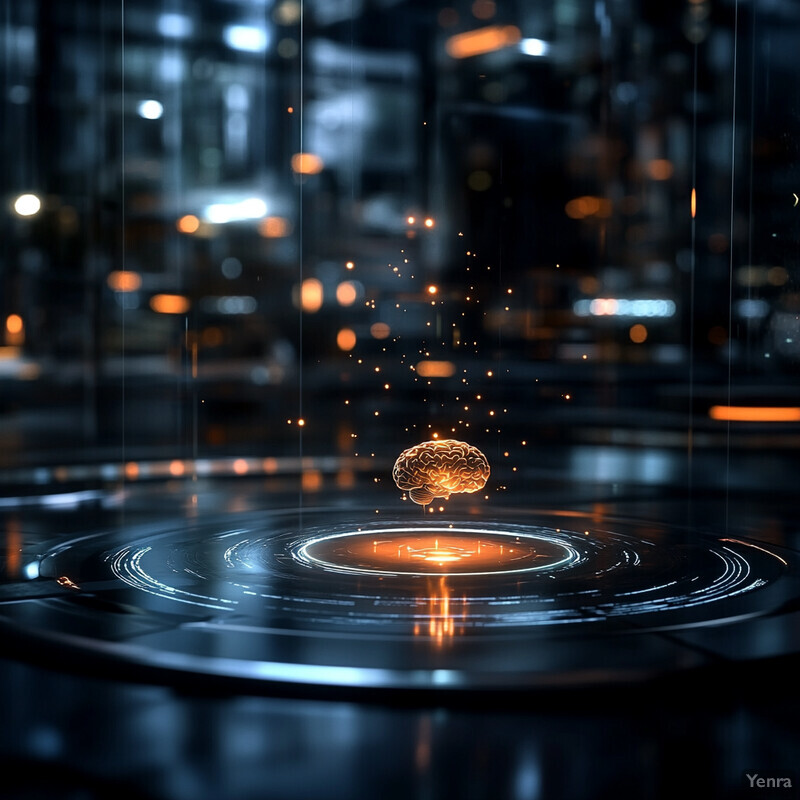
Machine learning approaches have shown remarkable success in early detection of neurological disease signatures. For example, an AI model that analyzes MRI brain scans was able to diagnose Alzheimer’s disease with over 90% accuracy, even identifying individuals in very early (mild cognitive impairment) stages that clinicians might miss. This 2023 system, reported in Nature News, uses patterns of subtle tissue loss and ventricular expansion as predictive biomarkers of Alzheimer’s. Another study applied deep learning to brain age – the discrepancy between a person’s chronological age and the apparent age of their brain on MRI – as an early marker of dementia. In early 2023, researchers showed that individuals whose brains appeared “older” than their actual age (as gauged by a CNN model trained on thousands of MRIs) were more likely to develop cognitive decline later. These examples illustrate how AI can flag the faint brain changes that herald disease, often providing a window of opportunity for early intervention.
10. Adaptive Registration and Alignment
AI has improved the registration (alignment) of brain images across individuals, time points, and modalities by making the process more robust and adaptive. Traditional image registration often struggled with anatomical differences or distortions; AI-driven registration uses learning-based approaches to handle these challenges. Deep learning models can learn how to warp one brain image to match another with minimal error, accounting for normal anatomical variability or even pathology. This ensures that corresponding brain regions line up more accurately in group analyses or longitudinal studies. Better registration means that statistical comparisons (e.g., looking for changes over time or differences between patient and control groups) are more valid since one is comparing like-with-like anatomically. In summary, AI yields more precise and reliable brain image alignment, which underpins essentially all quantitative brain mapping studies.
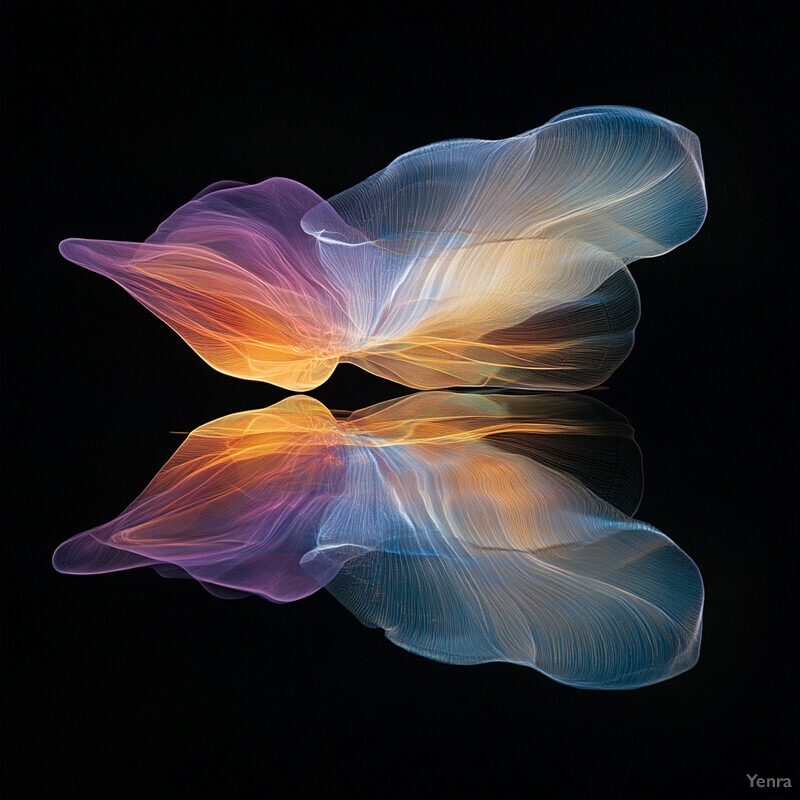
Deep learning–based image registration has demonstrated superior accuracy and speed relative to classic algorithms. In one study, an unsupervised AI model for CT–MRI brain registration improved cross-modal alignment by up to 12% (measured via mutual information similarity) compared to standard methods. The AI-registered images showed visibly better overlap of structures between MRI and CT, addressing issues like tissue deformation and scanner-induced distortion that rigid alignment missed. Another example is VoxelMorph, a deep learning registration framework, which has been shown to produce accurate deformable alignments in seconds, whereas conventional iterative solvers took minutes and often got stuck in suboptimal solutions. More recently, researchers introduced BrainMorph, a foundation model trained on 100,000 MRIs, which can robustly register brains even in the presence of tumors or lesions, far beyond the capacity of any single atlas approach. These advancements illustrate how AI-driven registration handles variability and artifacts gracefully, ensuring that brain images can be compared on a truly equal footing.
11. Quantitative Analysis of Microcircuitry
AI tools can automatically trace and quantify the fine details of neural microcircuits—such as individual neurons and their synaptic connections—in high-resolution brain images. Traditionally, mapping a local circuit (like a cortical column or a patch of hippocampus) required painstaking manual tracing of each axon and dendrite. Now, computer vision algorithms identify and count synapses, measure neuronal morphologies (e.g., dendrite lengths, branch counts), and detect network motifs (like recurrent loops) in these microscopy datasets. This yields rich quantitative descriptors of microcircuit architecture: for example, average synapse density per neuron, or distribution of inhibitory versus excitatory synapse counts. Such quantitative maps allow scientists to rigorously compare circuits (e.g., healthy vs diseased tissue) and to test theories of how circuit structure relates to function.
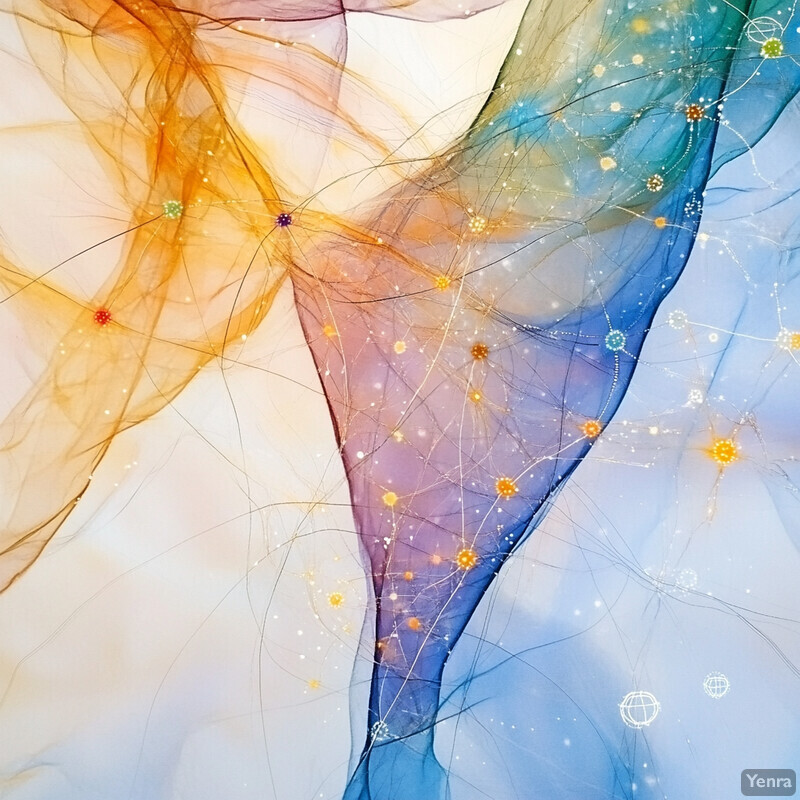
Recent AI-powered connectomic studies have quantified microcircuit properties on an unprecedented scale. In 2021, researchers reconstructed a ~1 mm³ chunk of human cortex (~57,000 neurons, hundreds of millions of synapses) and, using automated analysis, catalogued metrics like synaptic densities and connectivity degree distributions for that entire circuit. This petascale connectome dataset (H01) was only analyzable thanks to machine vision algorithms that could count synapses and classify cell types across the volume. Similarly, the MICrONS project (2021) mapped a large mouse visual cortex microcircuit and employed AI to quantify over 500 million synaptic connections, revealing network motifs such as common input patterns onto certain neuron classes. In another example, a deep learning pipeline automatically traced individual retinal neurons and measured each cell’s complete wiring diagram, enabling researchers to correlate specific wiring motifs with known functional circuit roles. These advances underscore that AI is turning qualitative circuit diagrams into quantitative datasets, advancing our understanding of neural network architecture.
12. Automated Quality Control
AI-based quality control (QC) systems automatically evaluate brain imaging data for common problems—such as motion artifacts, scanner distortions, or segmentation errors—ensuring that only high-quality data are used in analyses. In large neuroimaging studies, manual QC (visually inspecting each scan) is time-consuming and subjective. AI alleviates this by learning what a “good” vs “bad” image looks like from examples. It can flag scans with excessive motion blur, bad slices, or technical issues in real time or post-acquisition. By filtering out low-quality data or even prompting re-acquisition of a scan, AI QC improves the reliability and reproducibility of brain mapping studies. It also standardizes QC criteria, reducing variability in what different technicians might consider acceptable data.
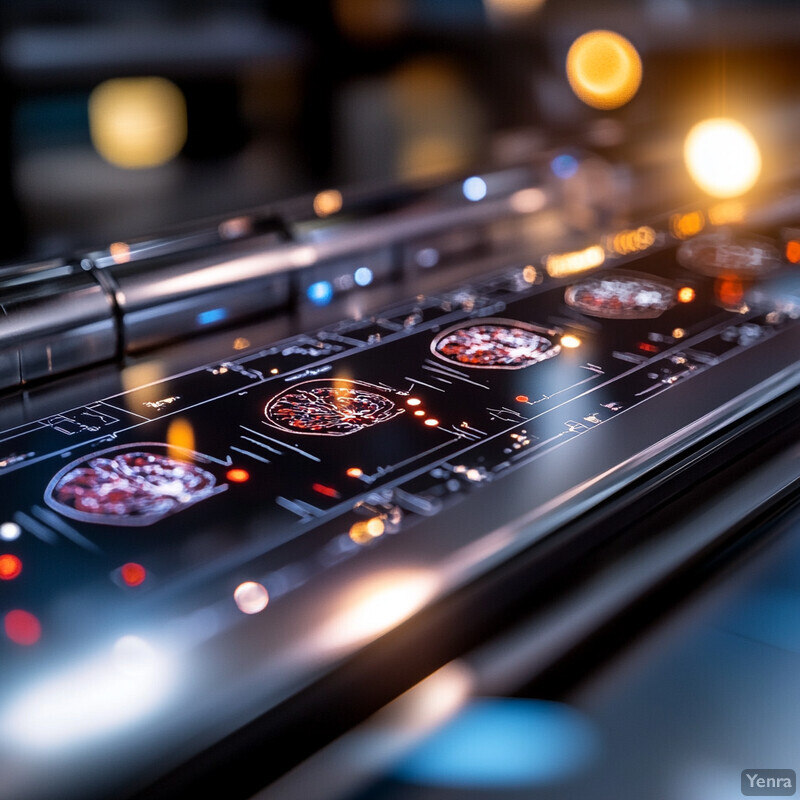
Novel deep learning models have been developed to detect artifacts in MRI and other neuroimages with high accuracy. For instance, a 2023 system by Pizarro and colleagues employed a 3D convolutional neural network with uncertainty estimation to identify MRI scans corrupted by motion or other artifacts. Their approach achieved human-expert level performance in screening a large, imbalanced dataset of brain MRIs, automating a task that previously required laborious visual review. In another study, researchers created a lightweight deep learning tool that sorted over 22,000 MRI volumes and reliably detected those with artifacts (like ringing or ghosting), vastly speeding up data cleaning in a multi-site study. These AI QC methods are now being integrated into neuroimaging workflows – for example, the UK Biobank imaging pipeline uses machine-learning based metrics to exclude scans with head motion or poor contrast. Overall, using AI for quality control has proven effective in maintaining high data quality standards at scale.
13. Human-in-the-Loop Systems
Human-in-the-loop AI systems create a collaboration between machine and expert, where AI algorithms provide suggestions (for example, segmenting a structure or labeling a neuron) and human neuroscientists refine or correct those suggestions. This iterative feedback loop leverages the speed of AI and the judgment of human experts. The AI can rapidly propose annotations for large datasets, while the human validates and fixes any mistakes. Over time, the AI can even learn from the human corrections to improve its future performance (continuous learning). Such systems dramatically accelerate workflows like image annotation or connectome proofreading while maintaining high accuracy. They also give human experts control to ensure that the final output makes sense scientifically, increasing trust in AI-assisted results.
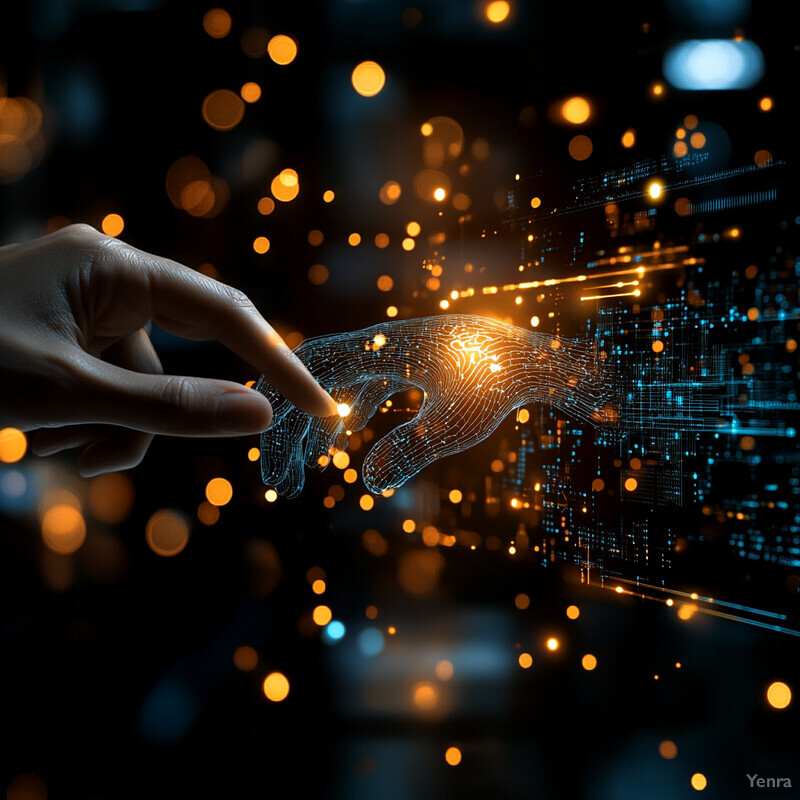
One recent approach in interactive segmentation had experts and AI working together to annotate medical images much faster than manual efforts alone. In this 2023 study, an AI would pre-segment brain images and an expert would then adjust the AI’s output; importantly, the AI model was continuously fine-tuned on these expert revisions, leading to progressively more accurate predictions. The authors showed that after a few rounds of this human-in-the-loop training, the AI’s segmentation quality approached that of fully manual expert delineations, cutting overall annotation time substantially. In practical connectomics, similar systems are used: for example, an AI might trace most of a neuron’s branches in EM data, and a human proofreader only corrects occasional errors (like a mis-joined branch), achieving near-perfect reconstructions in a fraction of the time it would take a human from scratch. These interactive AI tools have been deployed in large projects (like proofreading the fly brain connectome), where they sped up annotation by an order of magnitude while keeping error rates low. The result is that extremely large brain maps can be curated feasibly, with humans guiding AI to the correct outcome.
14. Graph Analytics for Connectomics
AI techniques rooted in graph theory (like graph neural networks) are being applied to analyze the brain’s wiring diagrams (connectomes) as complex networks. By representing brain regions or neurons as nodes and connections as edges, these methods can uncover important topological features: for example, identifying hub nodes that are highly connected, clusters or communities of interlinked regions, and how network structure relates to function or disease. Graph-based AI can handle the huge size of brain networks and find patterns not obvious by visual inspection. This yields insights into how the brain’s network organization (small-world properties, modularity, centrality measures) underpins cognitive processes or how it is altered in disorders. In short, AI-driven graph analytics turns raw connectivity data into deeper understanding of brain network architecture and its influence on behavior.
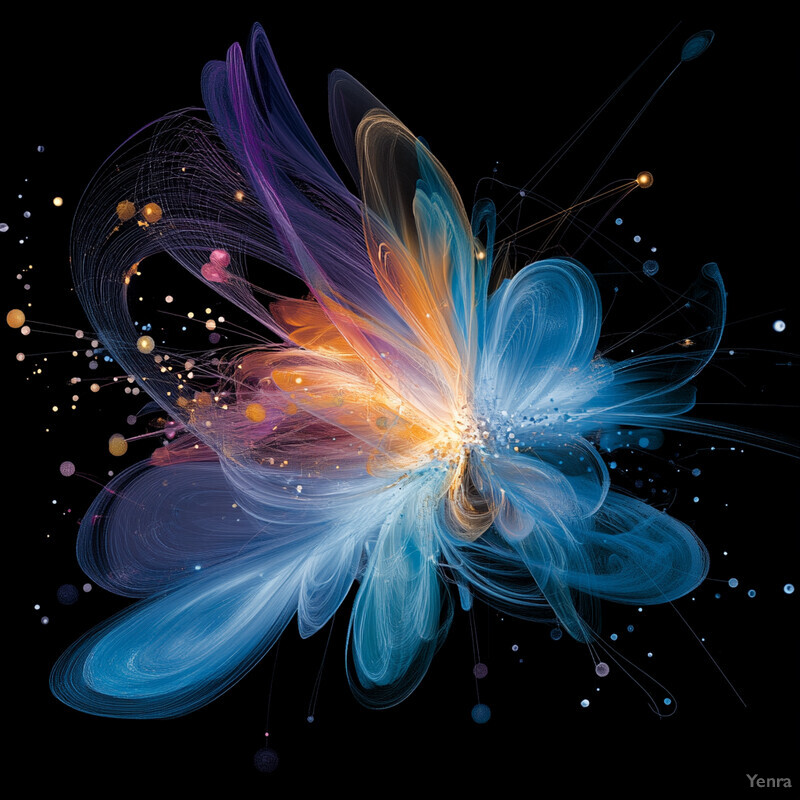
Graph neural networks (GNNs) have shown exceptional ability in modeling and interpreting connectome data. In one benchmark (BrainGB, 2022), various GNN models outperformed traditional network analysis in predicting individual traits (like intelligence or disease status) from brain connectivity patterns. These GNNs automatically learned which nodes (brain regions) and connections were most informative – effectively highlighting network hubs and subnetworks linked to the outcome. Another study introduced an interpretable GNN that identified consistent “network signatures” of brain disorders across patients. For example, in schizophrenia, the model discovered a subnetwork of fronto-temporal connections whose disruption was strongly predictive of the diagnosis, suggesting a network-level biomarker. Moreover, new graph attention techniques allow AI to assign weights to connections, so researchers can see which specific circuit anomalies drive differences. Overall, applying AI to brain graphs is revealing multi-scale organizational principles – from tightly-knit communities of neurons to crucial long-range links – and how these relate to cognitive function and dysfunction.
15. Reduction of Manual Labeling Effort
AI is reducing the need for large manually labeled datasets through techniques like active learning and semi-supervised learning. In brain mapping, obtaining annotations (for example, labeled brain regions, or identified cells in microscopy) is labor-intensive. Active learning algorithms intelligently select the most informative examples for human labeling, so that each expert annotation yields maximal benefit for the model. Semi-supervised methods allow models to learn from both limited labeled data and abundant unlabeled data (e.g., many MRI scans without annotations), extracting meaningful patterns without exhaustive labels. By doing more with less labeled data, these approaches greatly alleviate the bottleneck of manual annotation. This accelerates development of new AI models for brain mapping, especially in scenarios where labeled data are scarce (such as rare disorders or novel imaging techniques).
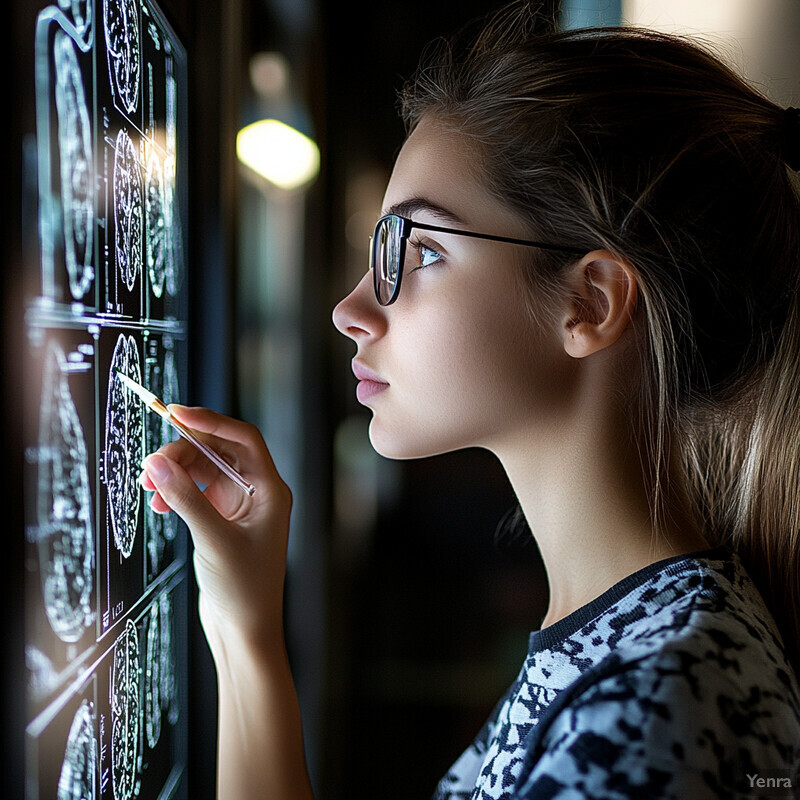
A 2024 study presented a semi-supervised pipeline called SAND that achieved state-of-the-art neuron segmentation in calcium imaging movies with far fewer manual labels than previous methods. SAND combined active learning (to pinpoint which frames truly needed expert annotation) with ensemble predictions on unlabeled frames to train its model, cutting the number of required labeled neurons by an order of magnitude while maintaining accuracy. In another example, for brain MRI segmentation, researchers have shown that querying just the most uncertain regions for annotation (instead of entire images) can reduce labeling effort by ~50% without loss of performance. Similarly, an active learning approach for multimodal brain tumor segmentation asked radiologists to label only the most informative image slices, and the resulting model performed on par with one trained on fully annotated scans, drastically lowering labor costs. These successes demonstrate that with AI guidance, we can curate high-quality brain mapping datasets with a fraction of the manual labels previously thought necessary.
16. Causal Inference in Connectivity
AI techniques, including reinforcement learning and causal inference methods, are being used to distinguish cause-and-effect relationships in brain networks, rather than simple correlations. In complex neural data, two regions might be correlated in activity but AI can help infer directionality (effective connectivity) – i.e., does region A drive region B or vice versa? By applying frameworks like causal discovery algorithms or training agents to manipulate simulated brain networks, researchers attempt to identify which connections are functionally causal. This moves brain mapping from static association maps to models that can predict outcomes of interventions (like stimulating one region to see effects elsewhere). Ultimately, understanding causal circuitry is key to devising targeted therapies (for example, knowing which node to stimulate to influence a whole network) and for fundamental insights into how information flows through the brain.
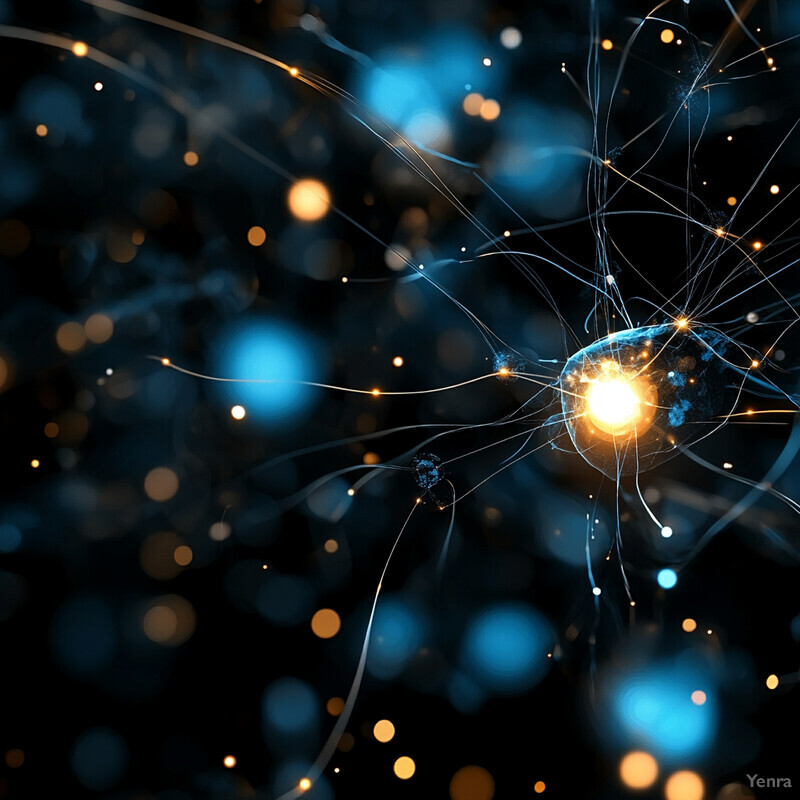
Researchers have started to incorporate causal modeling into brain network analysis. A 2023 study used a deep reinforcement learning approach to evaluate effective connectivity changes in patients with tinnitus. The RL-based model identified specific directed connections in functional MRI data that changed after therapy, suggesting those connections were causally involved in the symptom relief. In another line of work, a causal autoencoder framework (MetaCAE, 2023) was proposed to learn brain network representations that reflect true causal influences rather than mere correlations. It focuses on brain effective connectivity – defined as the causal effect one region has on another – and was shown to better differentiate patients from controls by capturing directionality in brain interactions. These approaches, still in early stages, hint that AI can help decode the brain’s causal wiring: for example, highlighting that region X’s activity reliably triggers changes in region Y, which might explain behaviors or pathologies. As methods mature, we expect a clearer separation of causal circuits from coincidental connections in brain maps.
17. Integration with Genomic Data
AI systems are bridging brain maps with genomic and transcriptomic data, revealing how genetic factors shape the brain’s structure and connectivity. By jointly analyzing imaging and genetic information, AI can uncover correlations between, say, a gene variant and the size or activity of a particular brain region. Integrative models can handle the high dimensionality of both MRI/fMRI data and genome-wide data, finding linkages that traditional analyses miss. This leads to insights such as identifying sets of genes associated with connectivity in neural networks or discovering imaging biomarkers that mediate gene–behavior relationships. Ultimately, AI-driven integration of multi-omics data with neuroimaging paves the way for personalized medicine approaches (for example, using a patient’s genetic profile alongside their brain scan to predict disease risk or treatment response).
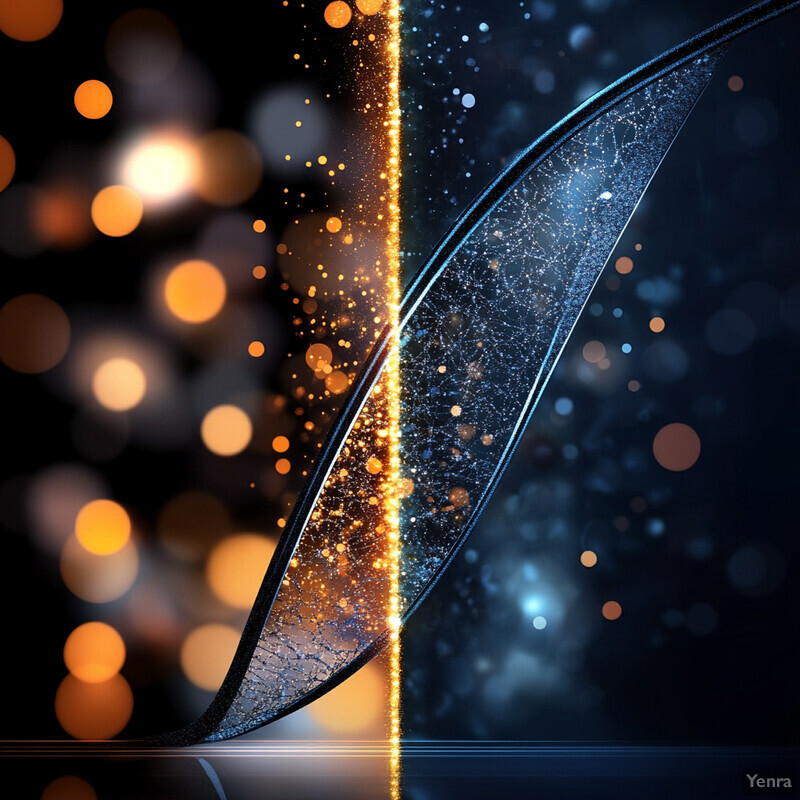
Multimodal studies are already leveraging AI to connect genes and brain maps. One 2023 analysis combined MRI scans and genome-wide data to better characterize schizophrenia: integrating genetic risk scores with brain connectivity patterns improved the performance of machine learning models in distinguishing patients from controls. This suggests that certain genetic variants exert detectable effects on brain network organization, which AI can pick up when both data types are analyzed together. Another example is a recent Nature study where researchers linked gene expression profiles to regional brain volumes; by training a model on both MRI measures and gene expression data, they identified novel genetic loci influencing hippocampal volume that were not found by imaging or genetics alone. These integrated approaches are uncovering gene–brain associations for conditions like Alzheimer’s and autism, e.g., an AI model found that a set of Alzheimer’s-related genes correlates with early metabolic changes in PET scans, suggesting a molecular pathway for observed neurodegeneration. The convergence of genomics and neuroimaging through AI is thus illuminating the molecular underpinnings of brain structure and function.
18. Personalized Brain Mapping
AI enables the creation of personalized brain maps that account for an individual’s unique anatomy and functional patterns, moving beyond one-size-fits-all atlases. By learning from large populations and then fine-tuning to a single person’s data, AI can generate individualized connectomes or parcellations. This is crucial in precision medicine: each person’s brain differs in subtle ways (folding patterns, connectivity strengths), and personalized maps capture those differences. Clinically, this means diagnoses or treatments (like targeting a stimulation electrode) can be tailored to an individual’s specific brain wiring rather than a generic template. In research, individualized maps improve the sensitivity to person-specific brain–behavior relationships. AI is thus facilitating a shift from population averages to maps as unique as a fingerprint for every brain.
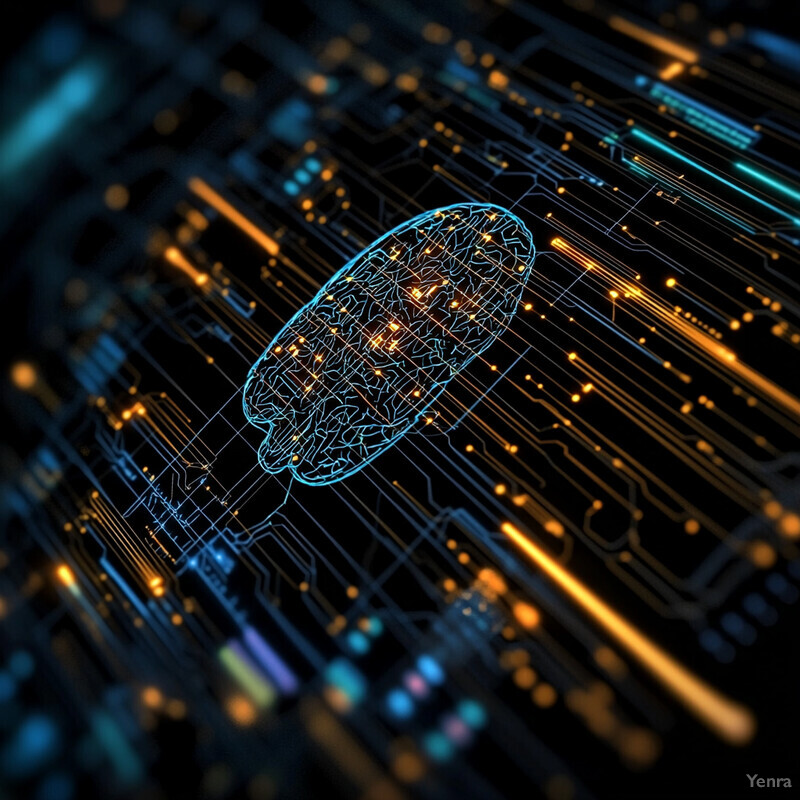
Recent work in functional neuroimaging demonstrates the power of personalized mapping. A 2024 study by Ma et al. used a deep learning model to individualize functional brain parcellations: the AI adjusted atlas boundaries for each subject based on their own fMRI connectivity, resulting in parcels that were significantly more predictive of that subject’s cognitive profile than standard atlas regions. In another example, researchers applying personalized connectome analysis to epilepsy patients found that AI-derived individual brain networks could pinpoint seizure-generating regions better than group-derived networks, informing more effective surgical plans (case series, 2023). Moreover, large initiatives like the “brain charting” project have used machine learning on tens of thousands of scans to establish normative ranges and then identify outliers at the individual level – for instance, detecting when a person’s hippocampal volume is abnormally low relative to peers, which might indicate early Alzheimer’s changes. These advances underscore that AI is making personalized brain mapping feasible and useful in both research and clinical domains, bringing us closer to precision neuroscience.
19. Virtual and Augmented Reality for Visualization
AI-enhanced visualization techniques are improving the clarity and interactivity of 3D brain maps, often through virtual reality (VR) or augmented reality (AR) environments. These immersive tools allow neuroscientists (and even clinicians or students) to “walk through” neural circuits or view layered brain scans in 3D, greatly aiding intuition and understanding. AI plays a role by processing complex datasets into smooth, explorable 3D models and by highlighting features of interest in real time (for example, an AI might label regions or flag connections while one navigates a VR brain). This makes the experience much more informative. By literally adding a new dimension to brain mapping, VR/AR helps users grasp spatial relationships in the brain’s wiring or anatomy that are hard to appreciate on flat screens. It also facilitates collaboration and communication of results, as multiple users can share an immersive view of the data.
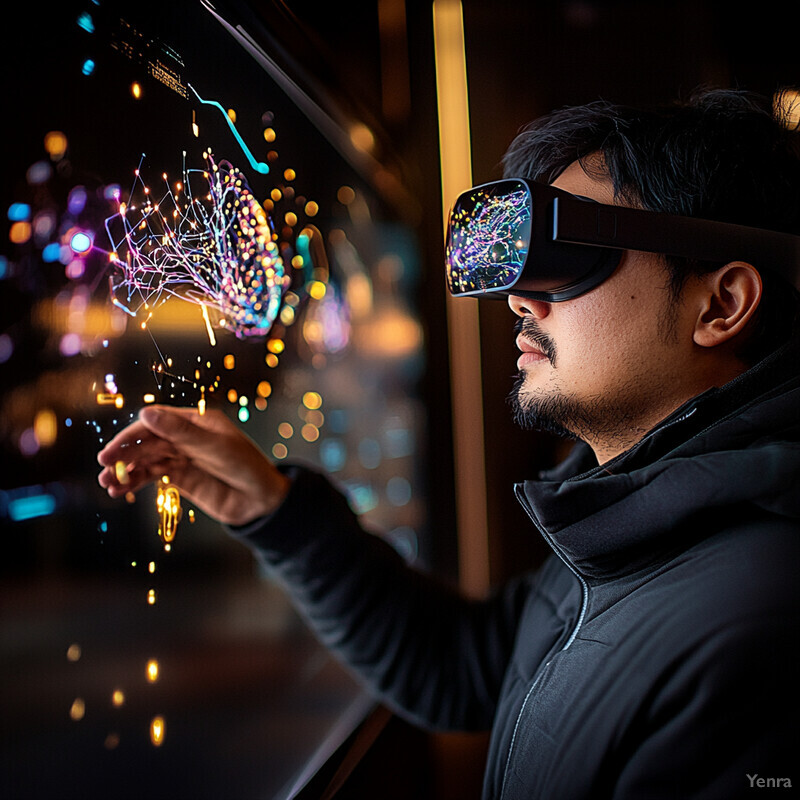
A recent system called VRNConnect exemplifies how immersive technology is applied to connectome data. Presented in 2024 (IEEE VR conference), VRNConnect lets users put on a VR headset and interact with a 3D graph of the brain’s connectivity – one can use hand gestures to select nodes (brain regions), display network metrics, and even see pathways light up when exploring connections. The tool’s AI components ensure the massive connectome graph is rendered smoothly and can emphasize important sub-networks dynamically. Another project, NeuroCave, provides a web-based VR experience of functional brain networks, where machine learning filters can be applied in real time to emphasize, say, the strongest connections or a particular module in the network. AR is also being used in the operating room – for instance, surgeons can wear AR glasses that overlay patient-specific brain tractography (processed by AI for clarity) onto the real surgical field, improving navigation through critical fiber pathways. These innovations indicate that immersive visualization, boosted by AI, is making complex brain data more accessible and actionable than everap-lab.ca .