1. Automatic Noise Reduction
AI-powered algorithms can isolate and remove background hiss, hum, crackle, and other undesirable artifacts more accurately than traditional noise-reduction tools, resulting in cleaner, more professional masters.
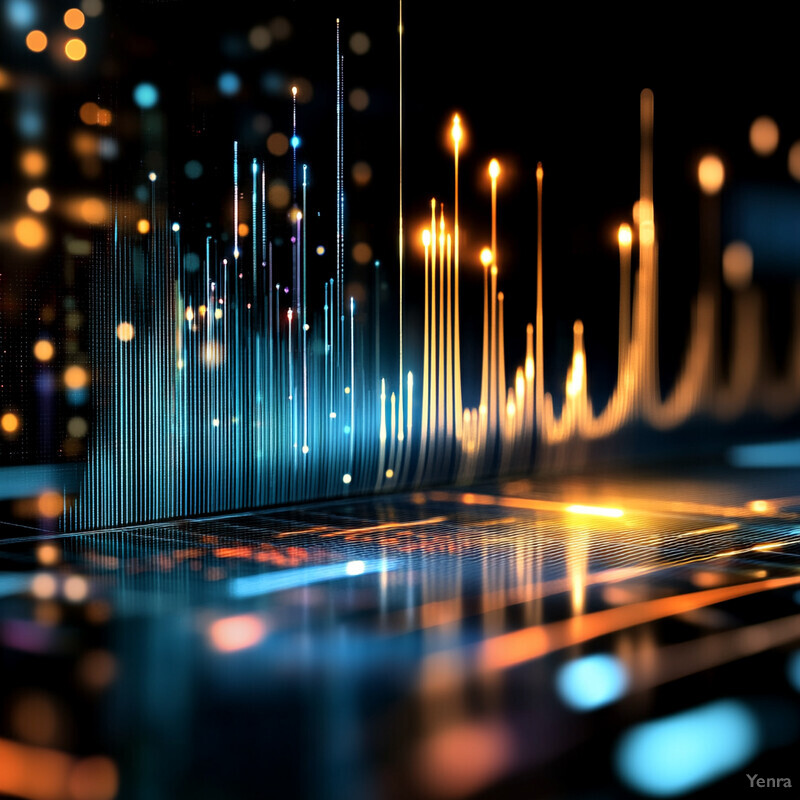
AI-powered noise reduction leverages machine learning models that have been trained on vast libraries of clean and noisy audio samples. Using these references, the system can detect patterns in unwanted sounds—such as tape hiss, vinyl crackle, AC hum, or ambient room noise—and selectively remove them without degrading the original musical content. Unlike traditional noise gates or static filters, AI can adaptively target problem frequencies and transient noises, applying just enough processing to clean up the signal while preserving the integrity and nuance of the performance. This results in final masters that are cleaner, clearer, and more professional, especially beneficial for archival restorations or older recordings where traditional tools might struggle.
2. Smart EQ and Dynamic Processing
Advanced machine learning models can analyze the frequency spectrum of a track and apply adaptive equalization, compression, and limiting tailored to the specific characteristics of the audio, thereby improving tonal balance and loudness consistency with minimal human intervention.
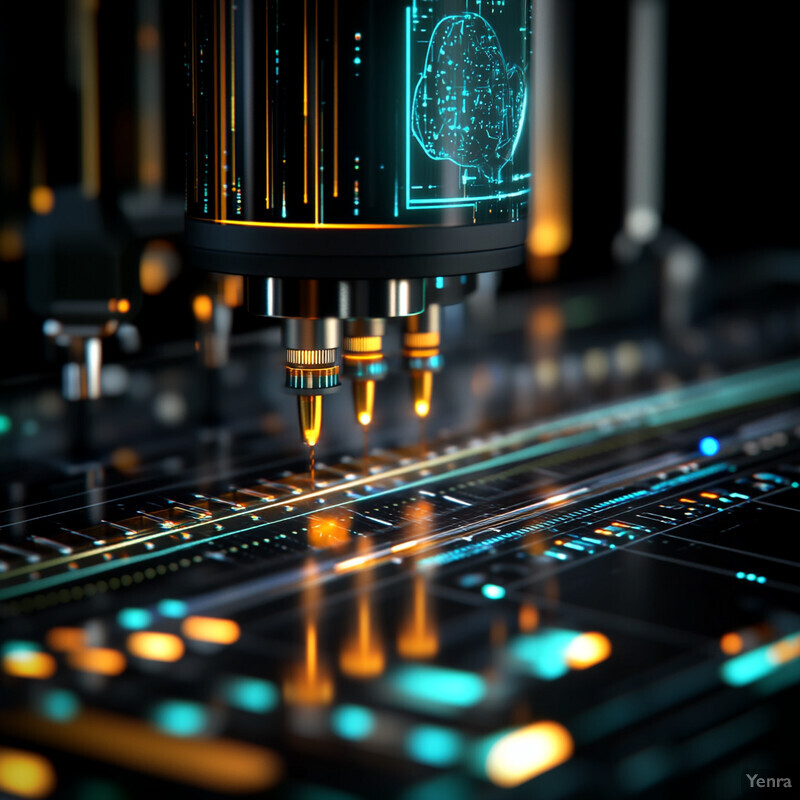
Rather than relying on fixed presets or manual experimentation, AI-driven equalization and dynamic range control technologies analyze the entire spectral and dynamic content of a piece. By understanding where certain frequencies or dynamics may be masking others, the AI can apply subtle EQ boosts, cuts, compression, or expansion to bring out the best qualities in the music. The system can identify if vocals sound muddy or if certain instruments are too piercing and then adjust the tone and dynamics accordingly. This targeted approach, guided by deep learning, ensures that the balance remains faithful to the artist’s vision while enhancing clarity, depth, and presence with minimal guesswork.
3. AI-Driven Source Separation
Neural networks trained on large audio datasets can separate vocals, drums, bass, and other instruments from mixed tracks, facilitating more granular remastering choices such as adjusting a single instrument’s level, tone, or effect in isolation.
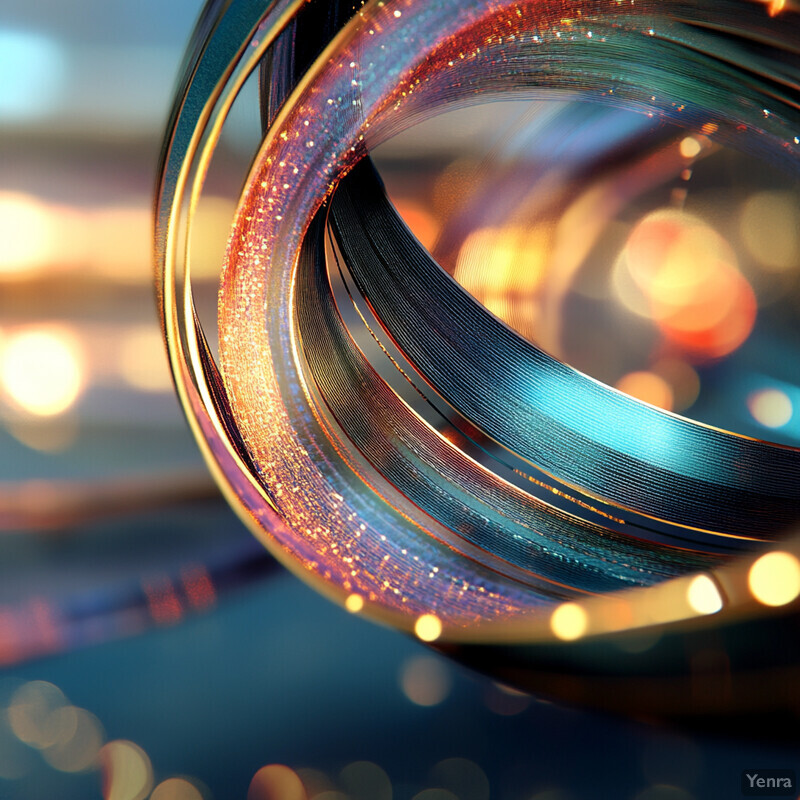
Modern AI models, particularly those using advanced neural network architectures, can “unmix” completed tracks, separating a full mix into its constituent elements—vocals, guitar, bass, drums, and more. This capability allows mastering engineers to revisit older recordings or poorly balanced mixes and make instrument-specific refinements long after the original multitrack files were lost or unavailable. By giving access to individual stems, AI-assisted tools enable nuanced adjustments to timing, tonal balance, and effects on specific parts. The end result is a more precise and controlled mastering process, where subtle tweaks can be made to single elements without compromising the rest of the mix.
4. Adaptive Room Emulation
AI models can identify the ideal acoustic environment or reverb settings for a given piece of music, applying room simulations that enhance the track’s spatial depth and ambiance without human trial-and-error.
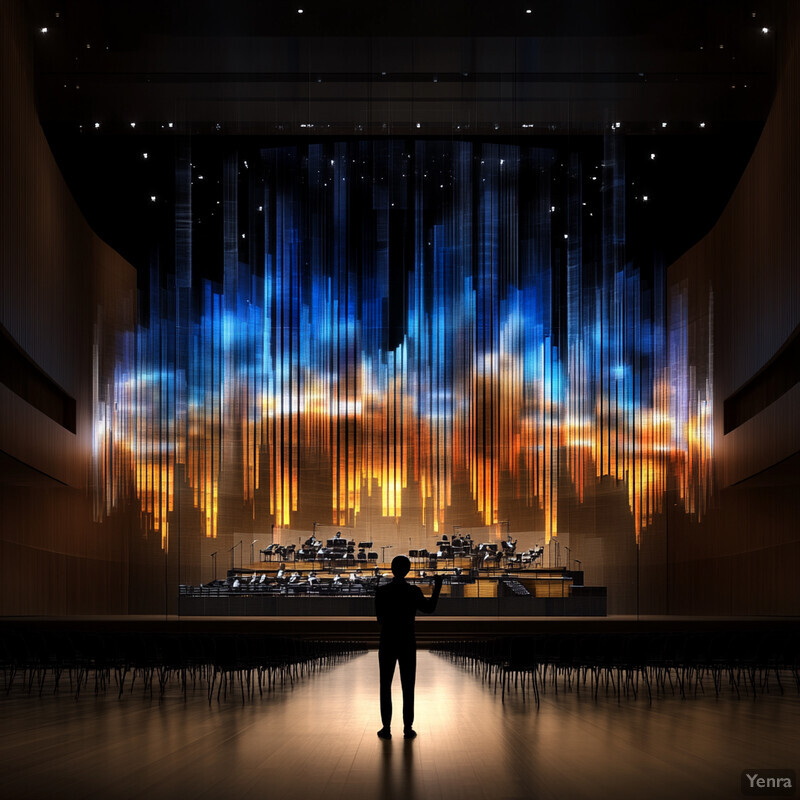
For decades, mastering engineers have worked meticulously to choose the right reverb or acoustic environment for a track. AI-based room emulation tools take this a step further by “listening” to the music and identifying what type of acoustic space would best enhance it—be that a warm studio booth, a grand concert hall, or a vibrant club setting. The system matches the musical style, instrumentation, and mood with sophisticated reverb profiles, automatically adjusting parameters such as decay time, early reflections, and diffusion. This intelligent matching removes much of the trial-and-error process, allowing engineers and producers to quickly arrive at the perfect spatial signature for their music.
5. Harmonic Enhancement
Machine learning algorithms can detect harmonic structures within a track and subtly reinforce or correct them, improving clarity, warmth, and musicality in ways that a mastering engineer might take hours to accomplish manually.
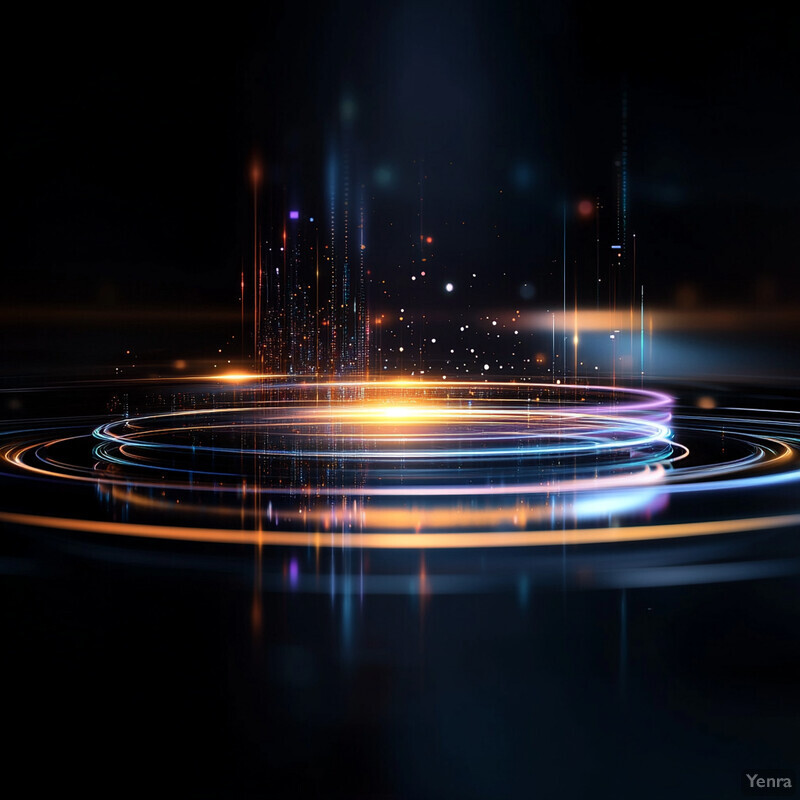
Harmonic content is central to the musical character of a track, influencing its warmth, clarity, and emotional impact. AI-driven harmonic enhancement tools analyze the underlying harmonic structure, identifying where certain frequencies or harmonic series may be underrepresented. Using advanced signal processing, they can add subtle harmonic overtones or reinforce existing ones, lending fullness to thin recordings or enhancing the richness of an already beautiful piece. Because the algorithm “hears” the music as a whole, it knows how to delicately shape and sculpt harmonic content in ways that are musically beneficial without sounding artificial or over-processed.
6. Intelligent Loudness Control
AI systems can automatically optimize overall track loudness to modern streaming standards or tailor it to specific distribution platforms (Spotify, Apple Music, YouTube), ensuring consistent perceived loudness without over-compression.
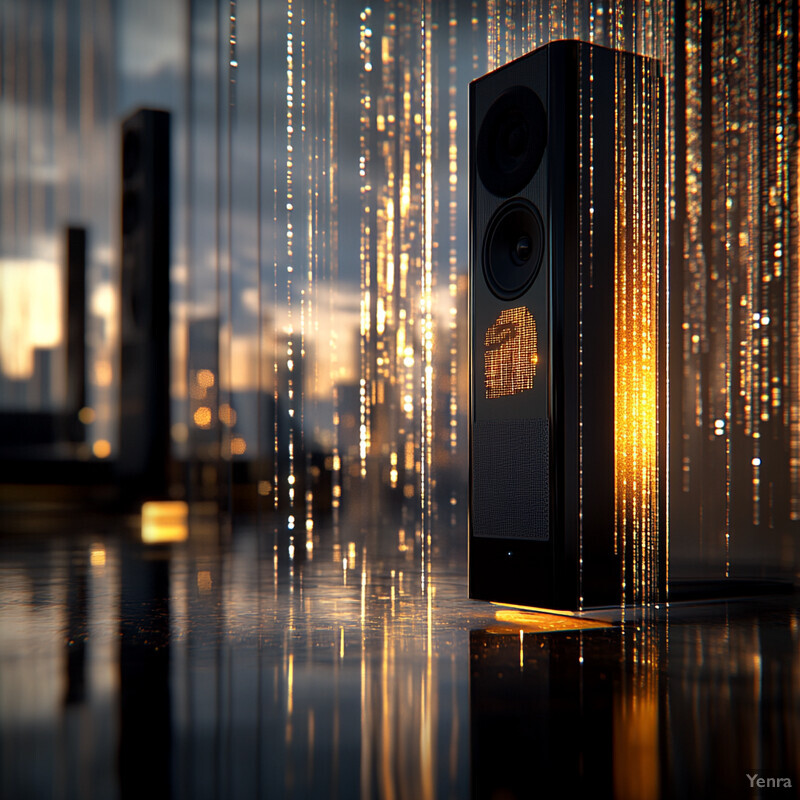
In a world where music is consumed across a variety of platforms—from streaming services to radio broadcasts—adhering to loudness normalization standards is crucial. AI systems can automatically measure and conform a track’s loudness to platform-specific guidelines, ensuring consistency in playback volume. Instead of applying a one-size-fits-all approach, these intelligent tools consider the track’s dynamics, genre, and instrumental makeup. They carefully adjust the gain and compression so that the final output is loud enough to be competitive while preserving transient detail and musicality. This dynamic and context-sensitive approach eliminates the risk of overly crushed masters and ensures that the music remains dynamic and engaging.
7. Spectral Shaping and Restoration
Advanced AI spectral editing tools can identify problematic resonances, phase issues, or digital clipping and correct them, improving the tonal integrity and smoothness of the recording.
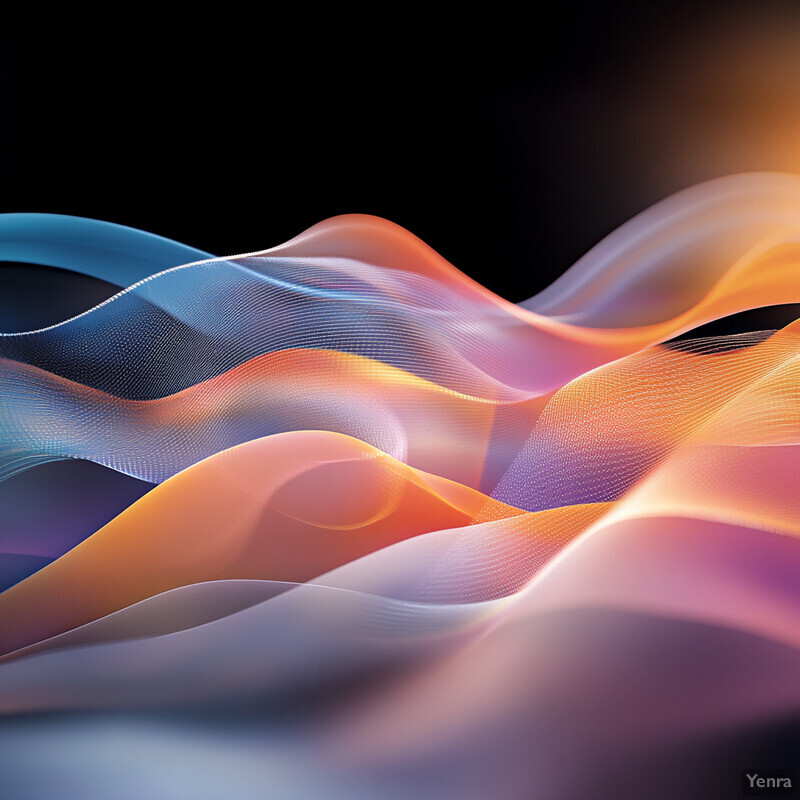
Spectral editing involves analyzing the frequency content of a track in great detail. AI-based spectral shaping tools can identify problematic frequencies, resonances, phasing issues, and digital artifacts that might be missed by the human ear or standard plug-ins. With precision reminiscent of a surgeon’s scalpel, the AI “repairs” these flaws at the spectral level, smoothing out harsh tones, restoring clarity to distorted sections, and aligning phase relationships. This produces a more transparent, lively, and pure sound. The process is far more efficient and accurate than manual spectral editing, saving significant time and improving results, particularly in older recordings or complex mixes.
8. Style Transfer from Reference Masters
Neural style transfer techniques enable AI to analyze the mastering profile of a reference track—its EQ curve, compression characteristics, stereo image—and apply a similar “finishing touch” to a new piece of audio automatically.
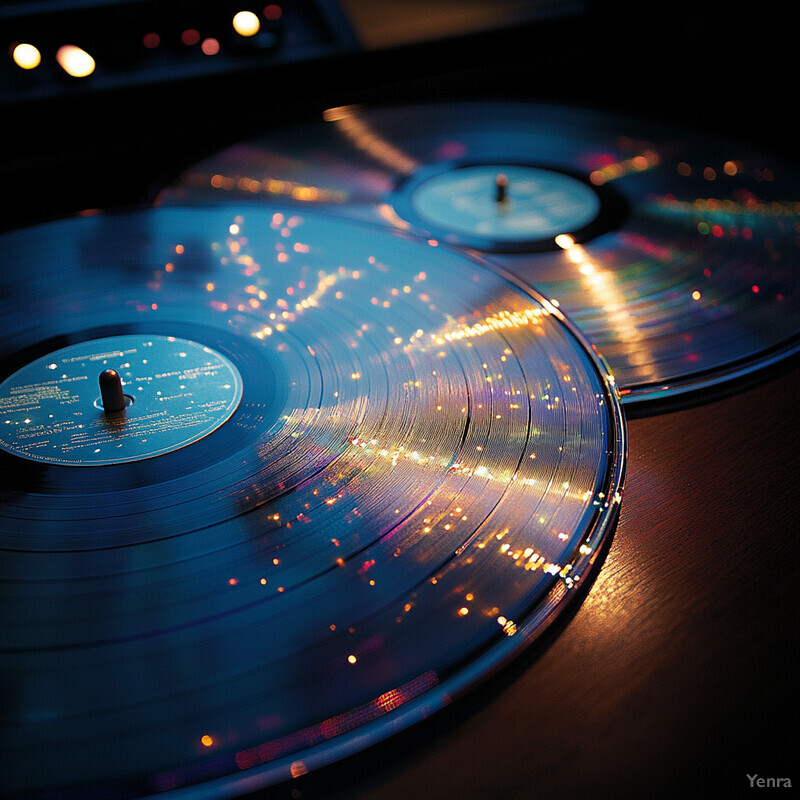
Style transfer, a concept borrowed from image processing, has found its way into audio mastering through deep learning. Engineers can feed the AI both a target track and a reference master that exemplifies the desired sound signature. The AI analyzes the characteristics of the reference—its EQ curve, stereo imaging, dynamic shaping, and overall sonic fingerprint—and then applies those characteristics to the target track. This approach allows for quick and consistent replication of a mastering style, useful for maintaining brand identity for a label, matching an established artist’s sound, or achieving stylistic coherence across multiple tracks in a playlist or album.
9. Batch Processing and Uniformity
AI can handle large collections of tracks simultaneously, ensuring consistent mastering quality and sonic signature across entire albums or catalogs quickly and efficiently.
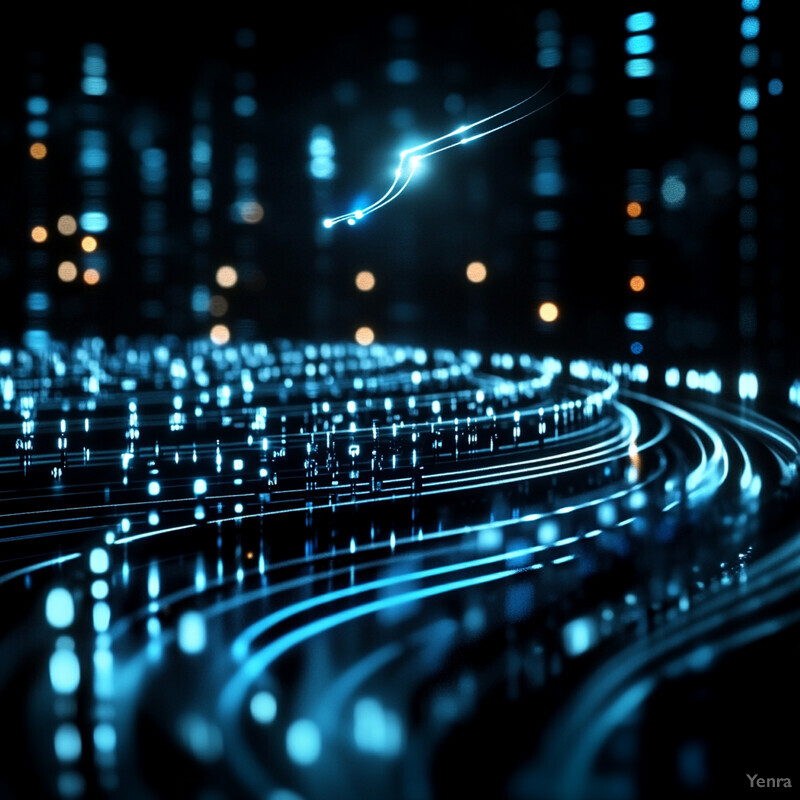
When dealing with large catalogs of music, such as entire discographies or streaming libraries, maintaining a consistent sonic character across tracks is challenging. AI-driven batch processing systems can automate this task by analyzing each track’s tonal and dynamic profiles and then applying standardized mastering parameters to achieve a uniform aesthetic. This ensures that every song in a playlist, compilation, or album series fits together coherently. The system can simultaneously handle a high volume of tracks, saving enormous amounts of time and reducing the drudgery of repetitive tasks. By ensuring every piece meets a certain quality standard, these tools elevate overall listener experience.
10. Adaptive Limiting and Clipping Control
Machine learning systems can detect transient details and apply the right amount of limiting, preventing unwanted distortion while maintaining energy and punch in the music.
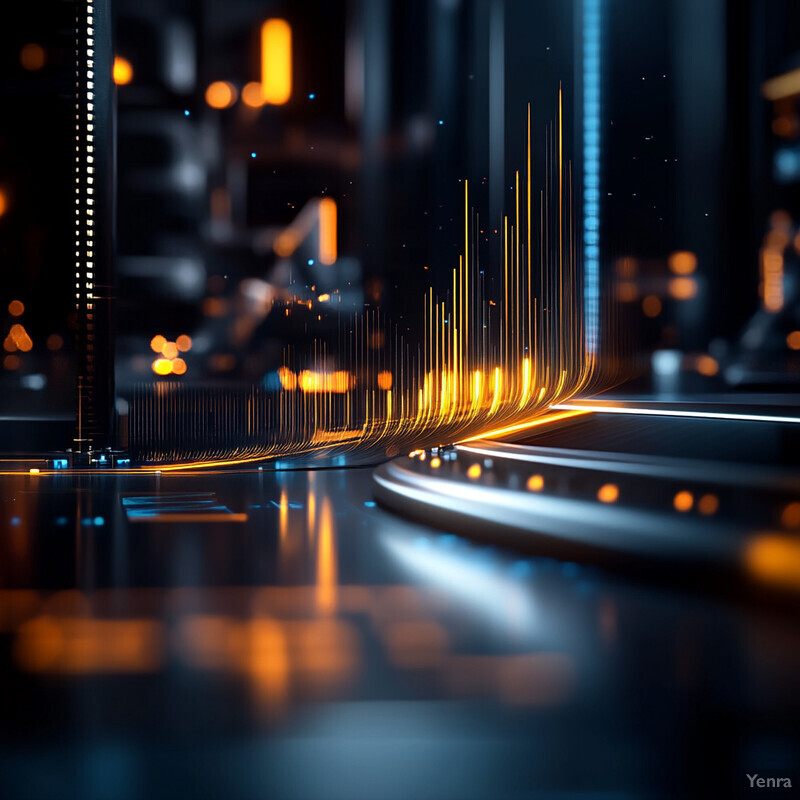
Limiting and clipping are critical steps in mastering, defining the final loudness and preserving a track’s punch. AI-driven limiting tools dynamically respond to the source material, using advanced modeling to identify where and how to apply gain reduction without sacrificing clarity. The algorithm can differentiate between transients that should be preserved and sustained parts that can be gently controlled. This ensures that the final master achieves the necessary loudness without introducing harsh digital distortion or pumping effects. As a result, the music retains its energy and impact, striking a delicate balance between volume and fidelity that is often hard to achieve manually.
11. Cross-Platform Mastering Presets
AI tools can generate tailored mastering profiles for different media formats (vinyl, streaming, broadcast) in minutes, optimizing each track for its final delivery medium.
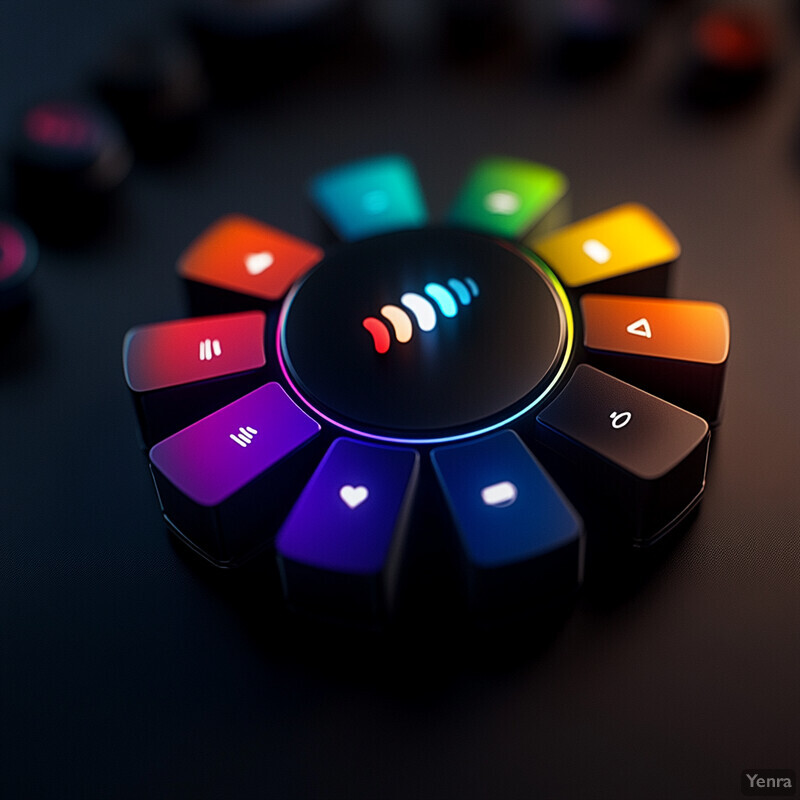
Modern music distribution spans multiple platforms—each with its own loudness standards, preferred bit rates, and target playback conditions. AI can tackle this complexity by creating customized mastering presets optimized for different endpoints. After analyzing each platform’s technical guidelines, the system produces separate masters that adhere to those specifications. This approach ensures that a track will sound consistently high-quality on Spotify, Apple Music, YouTube, terrestrial radio, or even vinyl. By automating this adaptation process, AI simplifies workflow, ensuring that artists and labels can confidently deliver their music to various audiences without compromising on sound quality or compliance.
12. Predictive Correction Suggestions
Some AI-driven systems analyze a mix and provide users with recommendations for adjustments—such as bass roll-off or subtle de-essing—before final mastering, streamlining the workflow for both audio engineers and musicians.
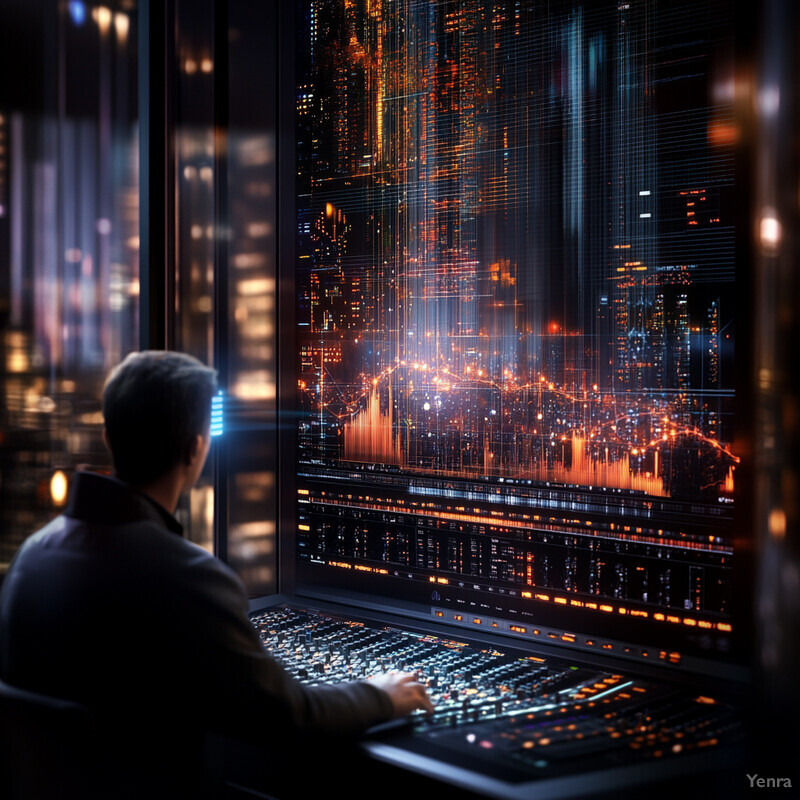
Before the mastering stage even begins, AI-driven tools can evaluate the raw mix and provide suggestions to improve it. By identifying potential trouble spots—excessive sibilance, boomy bass, overly bright cymbals—these systems guide the user to make beneficial mix adjustments early on. This proactive step not only speeds up the mastering process by starting with a more balanced source but also helps novice engineers learn best practices. Predictive suggestions empower users to achieve better results, reduce guesswork, and maintain a streamlined workflow. With AI’s guidance, the transition from mixing to mastering becomes more efficient and coherent.
13. Metadata Analysis and Integration
AI can automatically extract and integrate relevant metadata (BPM, key, genre) to help guide mastering decisions, ensuring that processing choices align with the track’s stylistic and structural attributes.
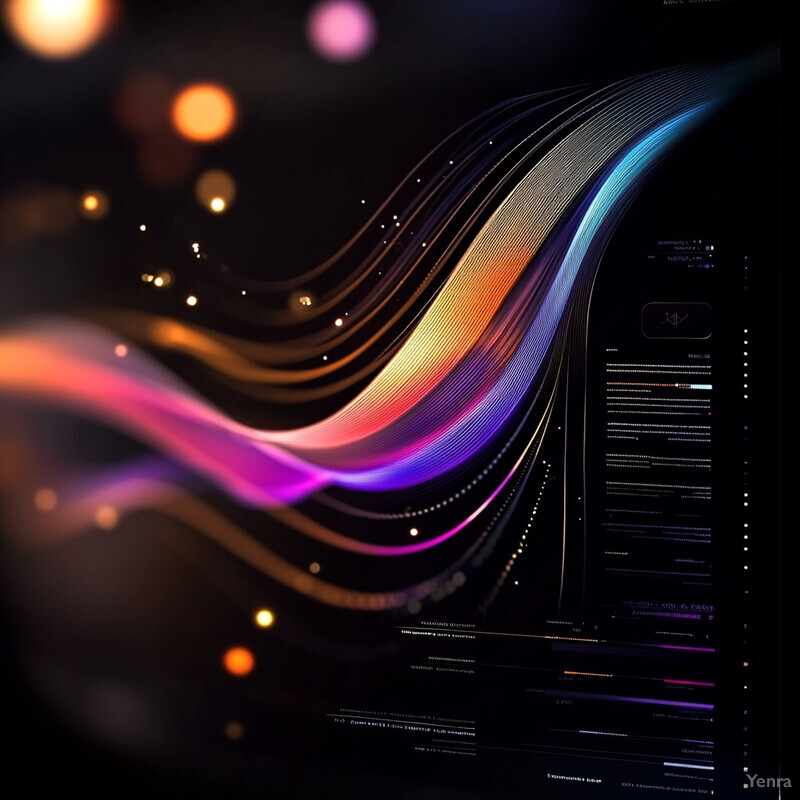
AI doesn’t just consider the audio signal; it also analyzes metadata like tempo, key, genre, and instrumentation. Armed with this contextual information, the system tailors its mastering decisions to the musical attributes, understanding that a classical piano piece needs different treatment than an EDM track. By integrating metadata, AI can more accurately predict the most suitable EQ curves, compression ratios, and spatial effects. As a result, the mastering process becomes more informed, less generic, and more attuned to the specific musical context, ultimately leading to a more polished and stylistically appropriate master.
14. Real-Time Mastering Feedback
Interactive AI systems can offer immediate visual and auditory feedback as adjustments are made, guiding users toward the best possible sound quality without extensive back-and-forth trial.
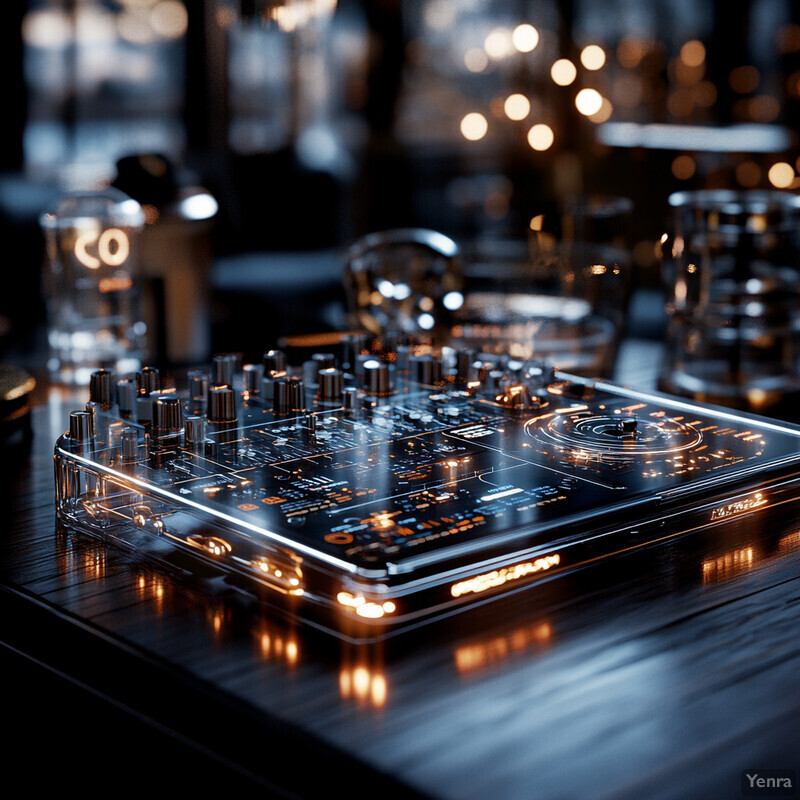
Instead of relying solely on iterative bounces and offline processing, AI-assisted mastering solutions can offer real-time feedback as the engineer makes adjustments. Visualizations show how changes in EQ, compression, or stereo width impact the overall sound. These systems can predict the outcomes of certain parameter tweaks before they’re even applied, helping users avoid missteps and get to the final sound more quickly. Real-time interaction fosters a more intuitive mastering experience, allowing both seasoned professionals and less experienced users to refine their techniques, improve their decision-making, and gain confidence in their skills.
15. Adaptive Stereo Imaging
AI can intelligently widen or narrow the stereo field depending on the content, enhancing stereo depth and presence while maintaining mono compatibility and clarity.
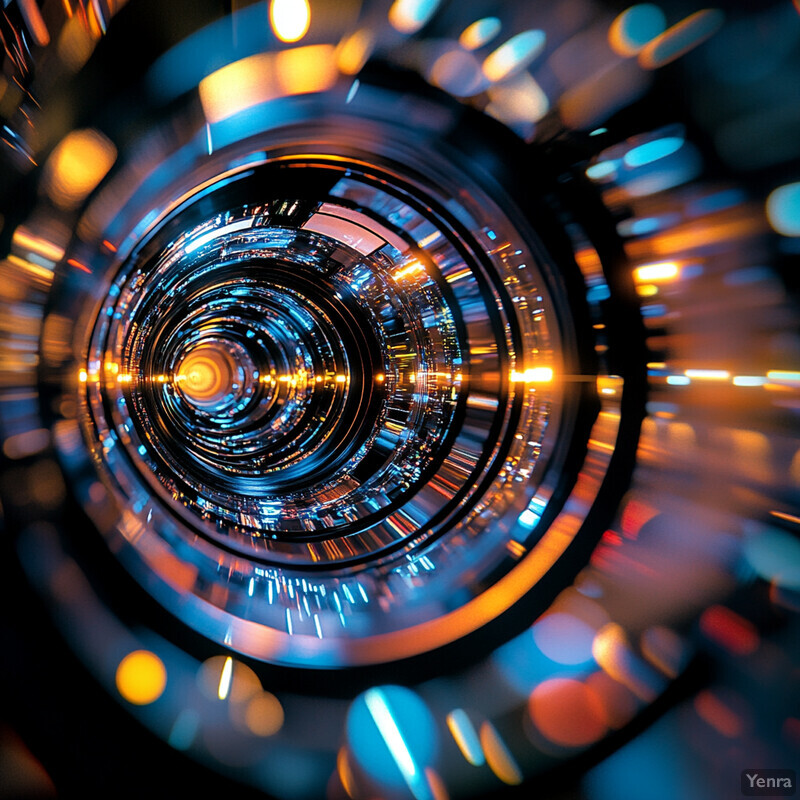
The spatial impression of a track is fundamental to its emotional and sonic impact. AI-driven stereo imaging tools analyze the phase relationships and spatial cues in a recording, identifying where and how the stereo field can be enhanced. They can subtly widen guitars, push certain elements deeper into the soundstage, or ensure that critical components remain centered and focused. By intelligently adjusting the stereo image, AI preserves mono compatibility while giving the music a richer, more immersive quality. The system thus elevates the listening experience on headphones and speakers alike, tailoring spatial characteristics to the genre and composition.
16. Customizable Personal Taste Profiles
Users can train AI mastering engines on their preferred sonic aesthetics by providing examples. The system will learn to apply these learned preferences to new tracks, personalizing the final master in line with individual tastes.
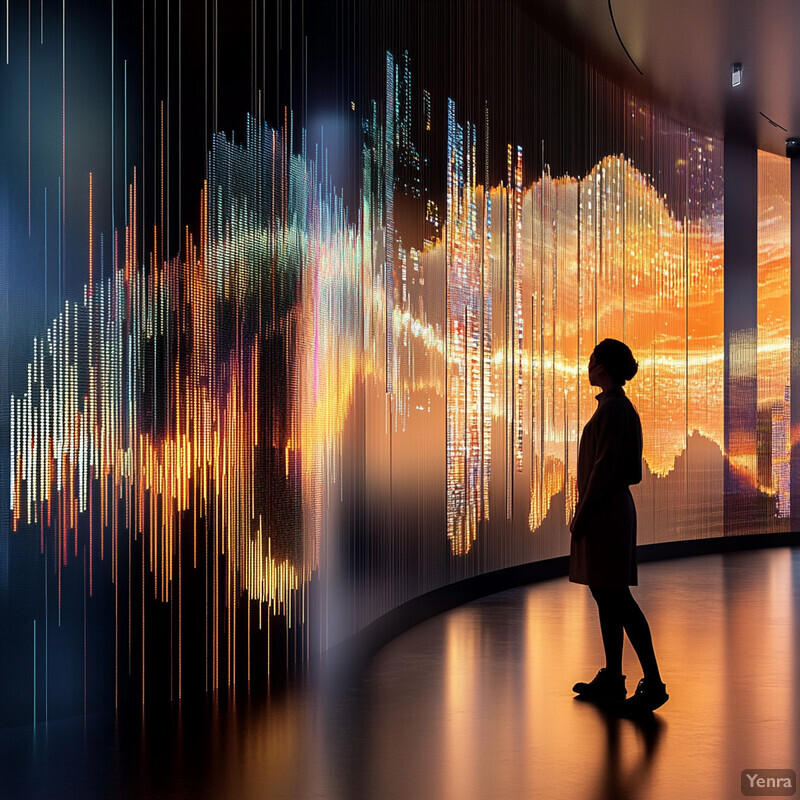
AI mastering engines can learn from user preferences over time. By training the system with examples of desired sound signatures—be it from reference tracks, previous masters, or user adjustments—the AI internalizes these tastes and can apply them to new pieces of audio. This creates a personalized mastering assistant that understands your unique aesthetic and consistently delivers results aligned with your stylistic vision. With each iteration, the AI refines its understanding of what you consider “good sound,” reducing the need for extensive manual tweaking and providing a more efficient, tailored mastering workflow.
17. Automated Vintage Emulation
AI models can replicate the sonic qualities of classic analog gear—tube EQs, tape saturation, vintage compressors—and apply these colorations consistently, giving digital recordings a warm, “old school” feel without manual tweaking.
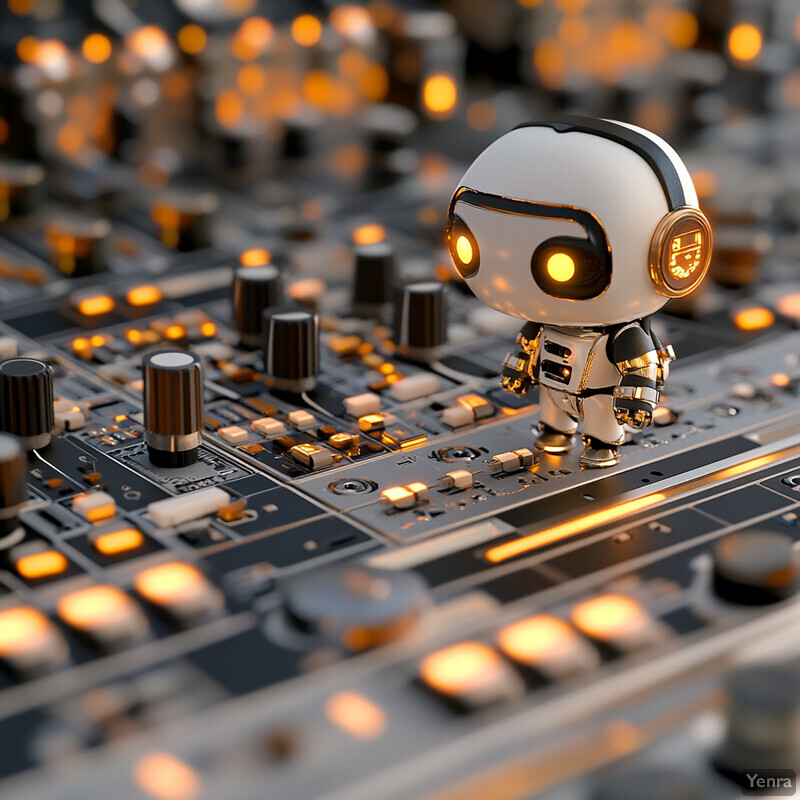
Capturing the elusive warmth and character of analog gear or vintage tape machines is a prized goal in mastering. AI can model the subtle nonlinearities and harmonic coloration introduced by classic processors, applying these sonic signatures at will. Rather than relying on static plug-in presets, the AI dynamically adjusts the intensity and frequency response of these emulations based on the specific audio content. This flexibility ensures that the vintage vibe is applied where it enhances the music, resulting in masters that combine the best of both worlds—modern clarity with timeless analog warmth.
18. Real-Time Cloud Collaboration
AI mastering solutions hosted in the cloud allow multiple parties (producers, engineers, artists) to instantly hear and compare different mastering results, streamlining decision-making and approval processes.
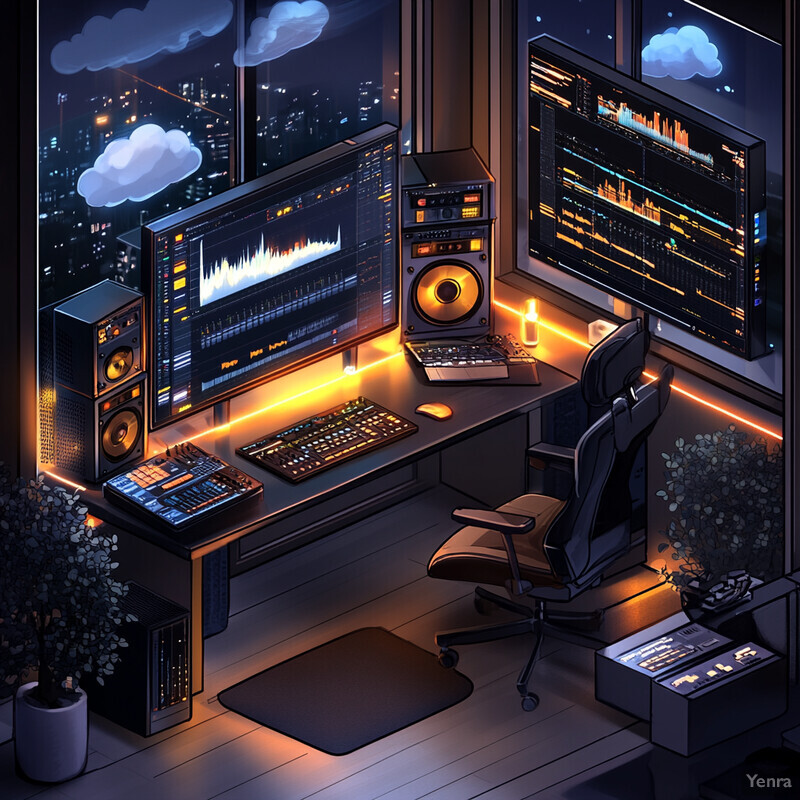
Modern mastering processes often involve multiple stakeholders—artists, producers, A&R executives, and mastering engineers—who may be located around the globe. AI-powered, cloud-based mastering platforms allow everyone to hear proposed changes in real time, facilitating instant feedback and faster decision-making. Stakeholders can audition various mastering chains or subtle adjustments on the fly, ensuring that the final product meets everyone’s expectations. This streamlined, collaborative environment reduces endless email exchanges and file transfers, speeding up approval cycles, and ultimately delivering a finished master more efficiently than traditional workflows.
19. Contextual Loudness Matching
Machine learning models can match the loudness and tonal profile of tracks within a playlist or album context, ensuring a seamless listening experience without noticeable volume jumps or tonal mismatches.
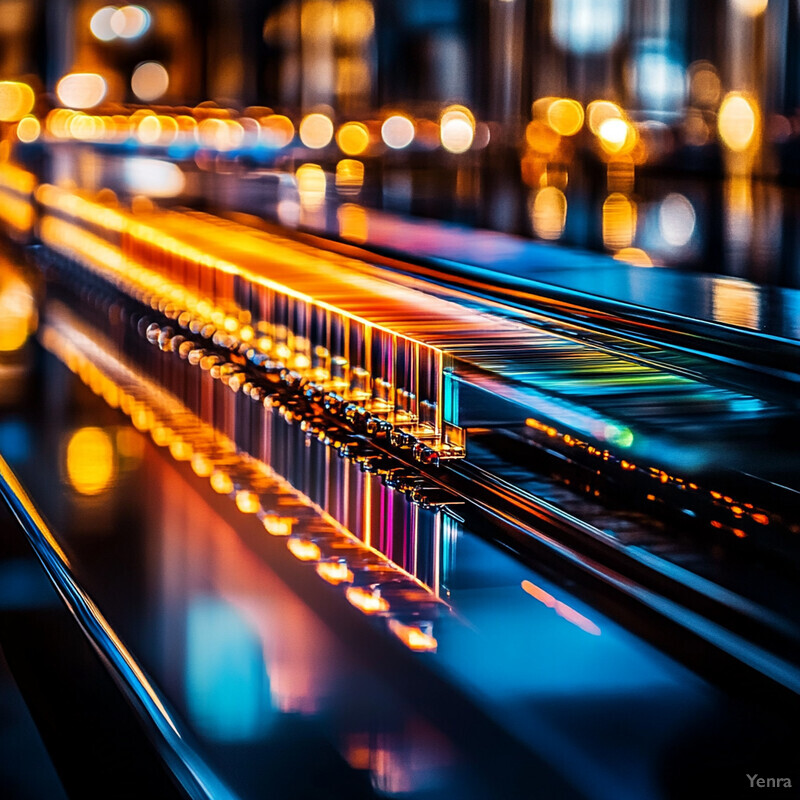
Maintaining consistent loudness from track to track, especially within an album or playlist, is key to a satisfying listener experience. AI can analyze an entire collection of songs, understand their relative volume levels, and make intelligent adjustments so that no single track stands out for being too quiet or too loud. This ensures a smooth journey for the listener, who can move seamlessly from one track to the next without constantly reaching for the volume control. By smoothing out the sonic landscape in context, AI enhances the flow and coherence of curated sets, album sequences, and streaming playlists.
20. Continual Self-Improvement
Because AI systems learn from their mistakes and successes, they grow more effective over time. As the technology is exposed to more genres, production styles, and mastering references, it continually refines its decision-making process, leading to ever-improving automation capabilities.
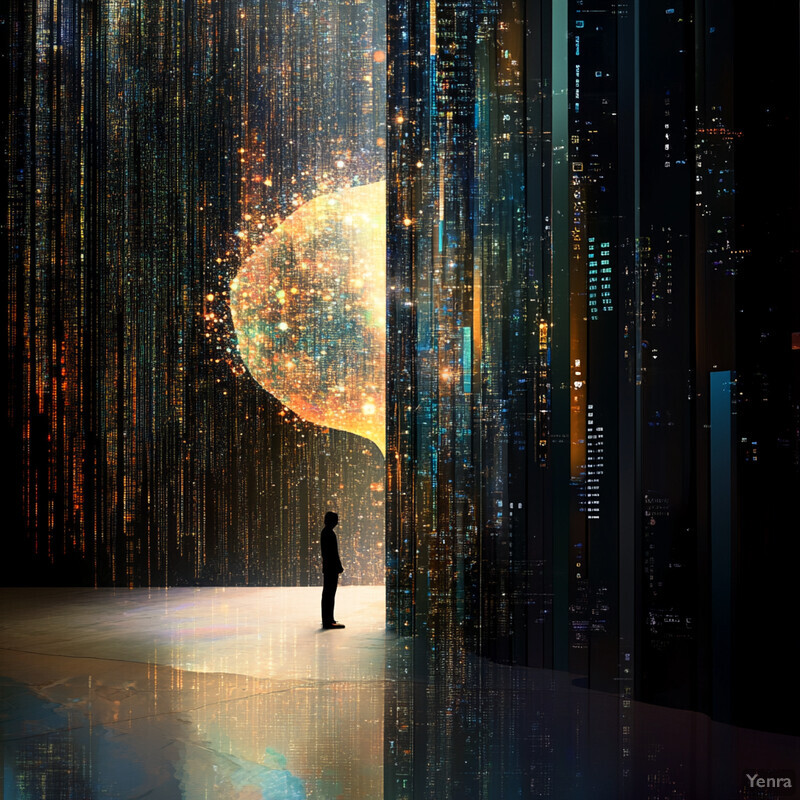
Unlike static processors, AI mastering systems continually learn and adapt from the data they consume and the feedback they receive. Over time, they hone their decision-making processes, refine their style transfer capabilities, and improve their detection of subtle audio issues. With each new piece of music processed, the system gains knowledge about different genres, production styles, and listener preferences. This ongoing evolution means that the technology grows more accurate, efficient, and musically sensitive, staying at the cutting edge of mastering best practices and consistently raising the bar for automated music enhancement.