1. Automated Melody Generation
AI-driven tools can propose original melodic lines based on training from vast musical corpora.
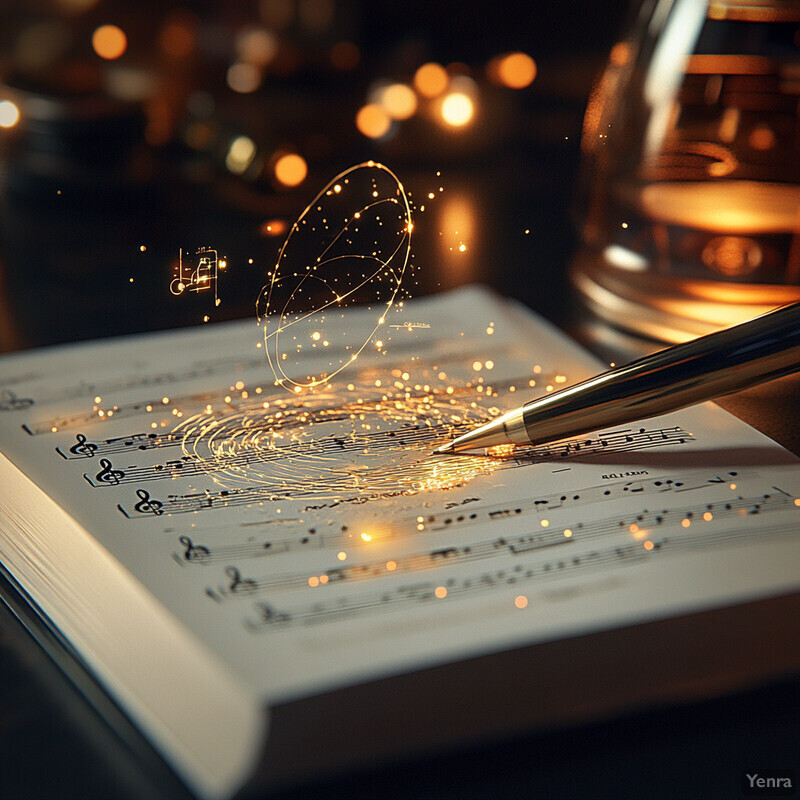
AI-based melody generation systems leverage deep learning techniques, such as recurrent neural networks and transformers, trained on vast datasets of music from diverse genres and historical periods. These models learn underlying melodic structures, common interval patterns, and stylistic tendencies, allowing them to produce compelling new melodies with minimal user input. The composer can feed a simple phrase, a harmonic progression, or even a specific mood, and the AI will respond with one or multiple melodic options that fit the given context. The result is a tool that can jumpstart creativity and help composers quickly test different melodic ideas without getting bogged down in trial-and-error. Over time, these systems can also be refined through user feedback, allowing the AI to adapt and align more closely with a composer’s unique aesthetic preferences.
2. Harmonic Progression Suggestions
AI algorithms analyze harmonic structures from a broad spectrum of music, enabling them to recommend chord progressions that complement a given melody or genre.
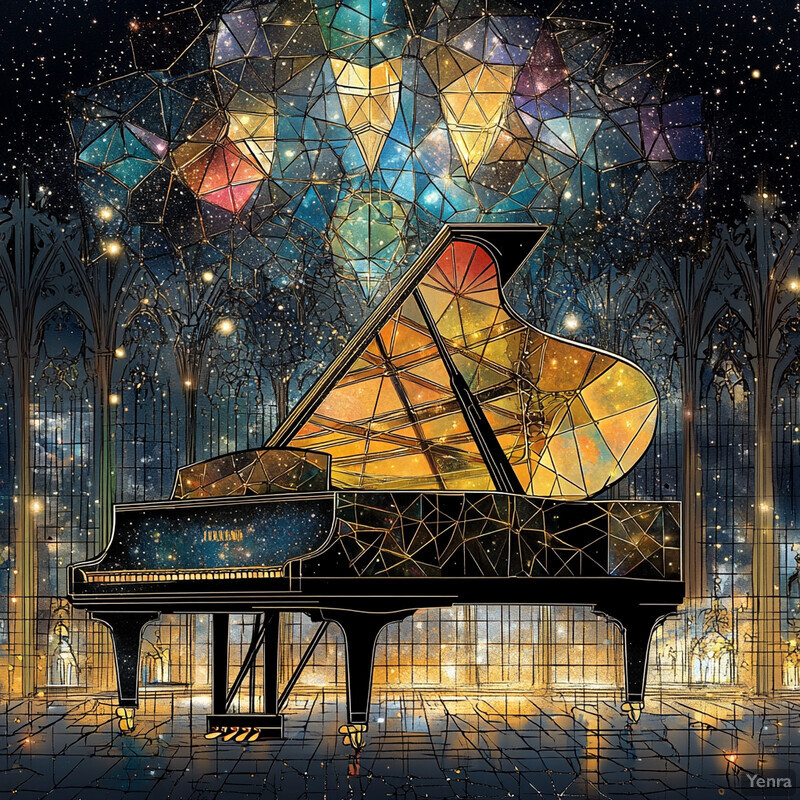
AI tools trained on a broad spectrum of compositions—from Bach’s chorales to modern pop hits—can identify functional harmony patterns, modal interchanges, and sophisticated chord substitutions. By analyzing the underlying harmonic grammar of various styles, the system can suggest chord sequences that blend seamlessly with a given melody or thematic material. For instance, a composer stuck in a creative rut can input a short melodic line and receive a set of harmonic paths that feel fresh yet stylistically coherent. By experimenting with these suggested progressions, the composer might discover new tonal colors or unusual harmonic turns that enrich the piece. Such tools not only expedite the songwriting process but also expose musicians to harmonic strategies they may not have otherwise considered.
3. Style Emulation and Genre Blending
Advanced machine learning models can learn the musical grammar of specific artists, periods, or styles, and then help composers generate music that emulates those influences.
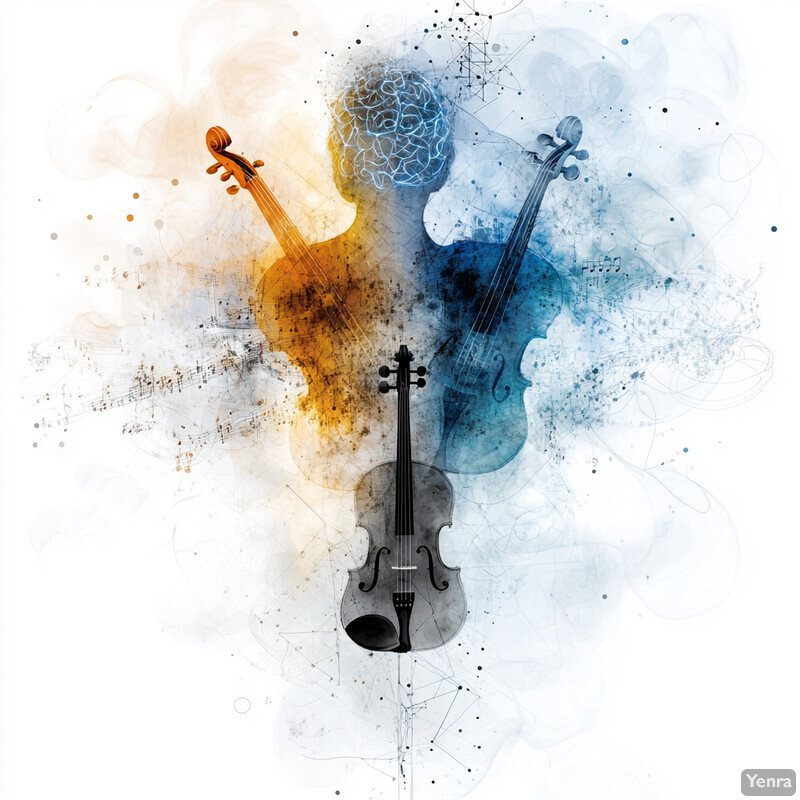
Advanced AI composition engines can be trained to internalize the signature elements of particular artists, historical periods, or cultural traditions. These AI models detect patterns in melodic contour, rhythm, orchestration, and thematic development. By controlling various input parameters, a composer can instruct the system to produce music reminiscent of a Renaissance madrigal, a John Williams-esque film score, or a Radiohead-inspired rock track. Beyond simple imitation, AI systems can also facilitate genre fusion. For example, a composer might blend the rhythmic complexity of West African percussion with the harmonic palette of jazz and the instrumentation of classical chamber ensembles. The AI helps navigate the intricate process of merging these disparate influences into cohesive, innovative hybrids that push creative boundaries.
4. Intelligent Orchestration Tools
AI can assist in orchestrating a piece by suggesting suitable instrumentation and textures.
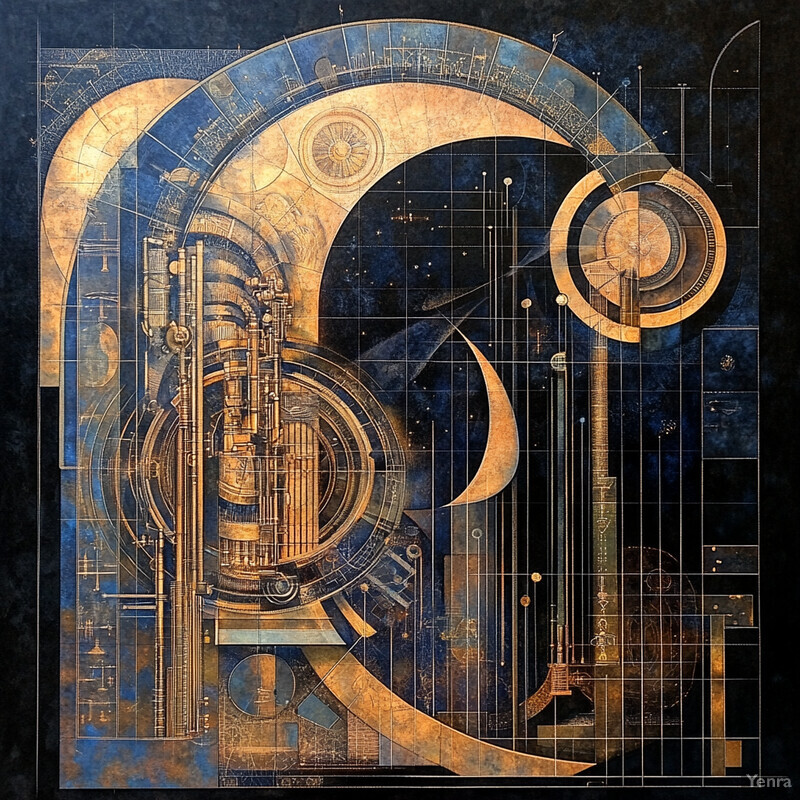
Arranging a piece for a large ensemble requires careful consideration of timbral balance, sonic density, and instrumental technique. AI-driven orchestration assistants analyze thousands of orchestral scores to learn which instrument combinations produce certain colors, how lines should be doubled to achieve depth, and where to place particular voices in the sonic landscape. Given a piano sketch or a lead sheet, the AI can propose orchestrations that highlight the main theme, support it with harmonious textures, and create dynamic builds and releases that maintain listener engagement. Composers can then select, refine, and customize these suggestions, leveraging AI as a knowledgeable orchestrator’s assistant that handles initial conceptual work, saving time and providing new ideas.
5. Adaptive Arrangement Guidance
AI-driven software can take a basic piano score and suggest ways to expand it into a full arrangement for any ensemble.
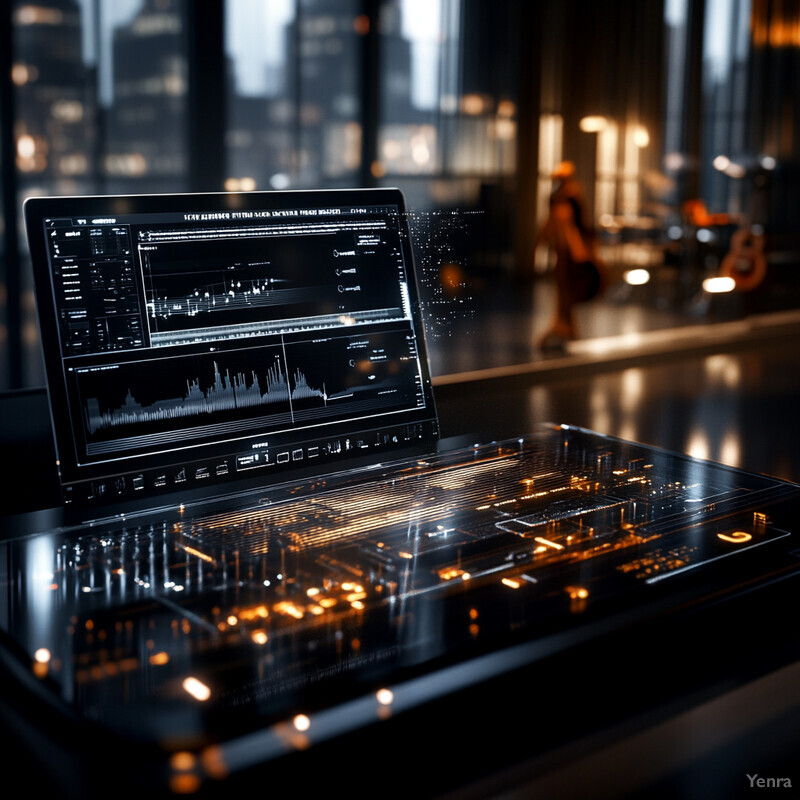
When expanding a bare-bones arrangement into a full-fledged composition, many choices arise regarding instrumentation, form, and internal contrasts. AI can provide guidance by offering multiple variations for voicings, countermelodies, and section transitions. For instance, starting from a simple piano-and-voice demo, the composer can ask the AI for a lush string-based texture in the bridge or suggest a syncopated rhythm section pattern to energize the chorus. The AI uses its understanding of successful arrangement techniques from numerous examples to produce cohesive ideas that match the style, mood, and complexity the composer desires. By quickly generating multiple arrangement scenarios, the system frees the composer to focus on their overall creative vision rather than getting bogged down in the minutiae of trial-and-error.
6. Dynamic Accompaniment Systems
Tools can generate live, intelligent accompaniments that react in real-time to a performer’s tempo and expressive nuances.
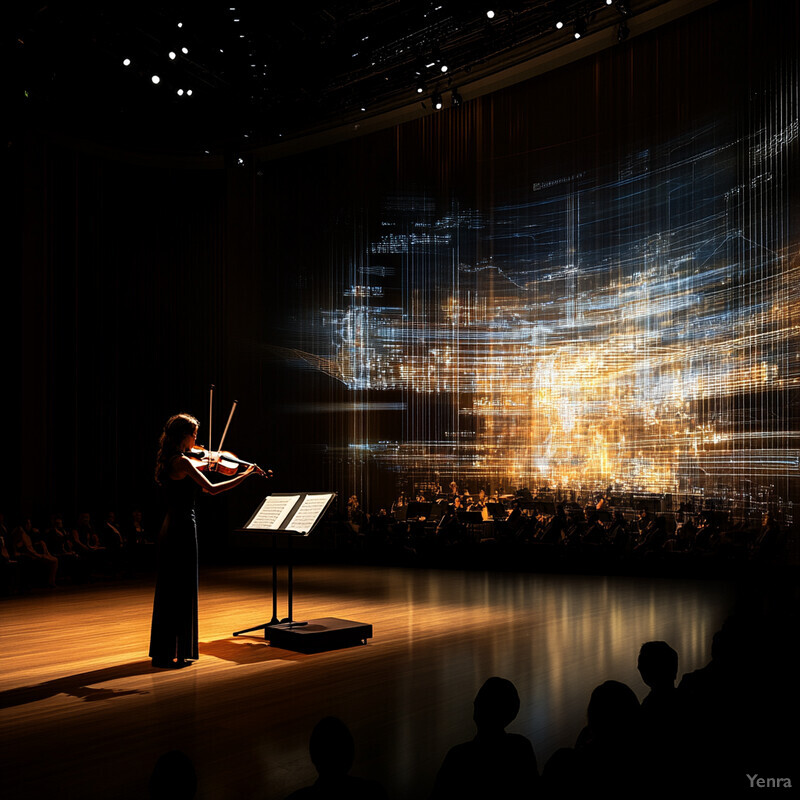
In live performance or composition prototyping environments, AI can function as a responsive accompanist. By analyzing a performer’s tempo, articulation, and expressive intent, the system adjusts its own playback in real-time, maintaining a natural musical conversation. This allows composers to simulate the presence of a skilled ensemble or accompanist without hiring live musicians for every rehearsal. For instance, a solo violinist exploring a new concerto can rely on the AI to provide a responsive orchestral backdrop that slows down, speeds up, or crescendos along with the performer’s interpretation. This real-time adaptation fosters an interactive creative process and helps composers refine their material in a more authentic performance context.
7. Emotion-Targeted Composition Assistance
By correlating musical features (tempo, mode, instrumentation) with emotional responses, AI can recommend adjustments to create or enhance specific moods.
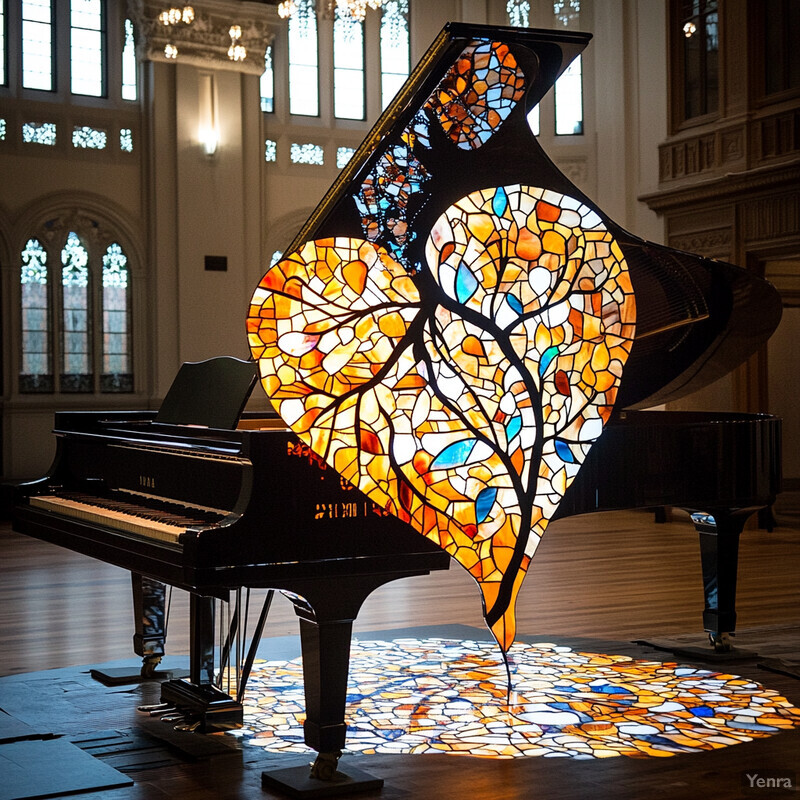
Music’s primary function often lies in evoking emotions. AI systems trained to correlate musical parameters—tempo, dynamics, texture, modality, harmonic tension—with emotional responses can help composers fine-tune their works to achieve specific affective states. A composer seeking a “heroic” feeling might receive suggestions like brass fanfares, dissonant-to-consonant chord resolutions for tension and release, or a gradually accelerating tempo. Conversely, for a “peaceful” atmosphere, the AI might propose gentle arpeggiated chords, warm string pads, and sparse percussion. By using models that map musical elements to emotional outcomes, composers can work more efficiently toward their expressive goals, ensuring their music resonates with the intended audience on a visceral level.
8. Motivic and Thematic Development
Machine learning can identify recurring motives and themes in a piece and suggest ways to transform or vary these ideas.
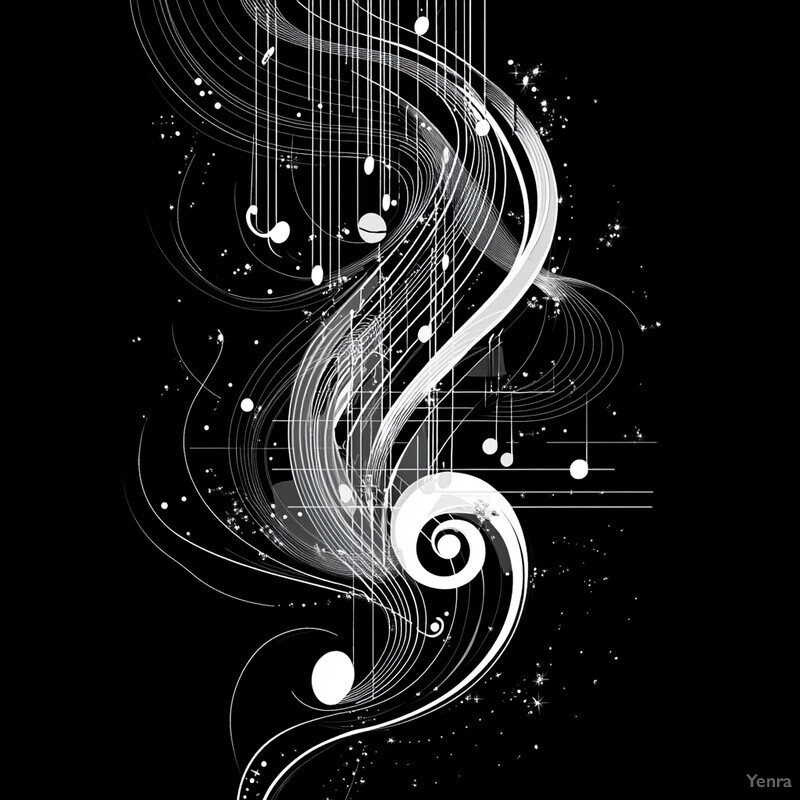
A cohesive composition often revolves around the intelligent manipulation and variation of a central motive or theme. AI can identify recurring melodic, rhythmic, or harmonic gestures and propose a range of transformations—augmentation, diminution, inversion, retrograde, or changes in rhythmic profile. By offering systematic approaches to motivic development, AI encourages composers to explore thematic evolution they might not have considered. The composer can then integrate these variations in different sections, creating a sense of unity and coherence throughout the piece. Over time, this capability also serves as a teaching tool, helping less experienced composers learn how great masters achieve thematic cohesion.
9. In-Depth Structural Analysis and Feedback
AI tools can map out a composition’s macrostructure and offer feedback to improve pacing, coherence, and dramatic arcs.
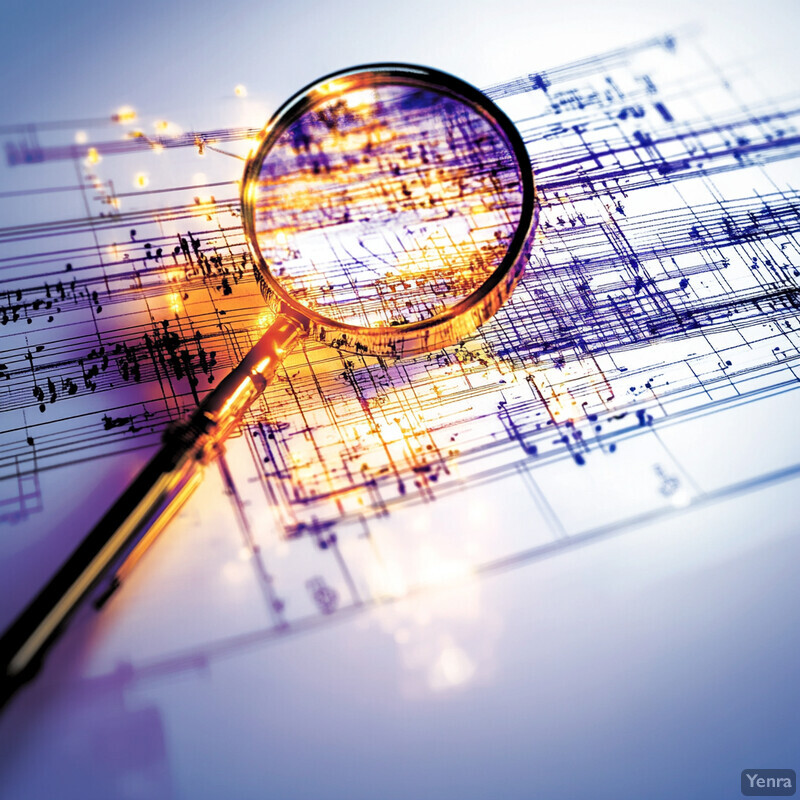
Well-structured music balances repetition and contrast, tension and release, and ensures that ideas unfold at a pace that feels satisfying. AI-based analysis tools map out the macrostructure of a piece, identifying sections, transitions, climaxes, and resolutions. They can detect imbalances—maybe a section feels too long, a climax arrives too early, or a motif is underdeveloped. The system can then suggest modifications to pacing, offer reordering of sections, or recommend adding transitional material. By giving composers a “bird’s-eye view” of their work, these tools facilitate more architecturally sound compositions and help refine the narrative flow of the music.
10. Automated Mixing and Mastering Assistance
While not strictly compositional, AI-driven audio processing tools integrated into composition software ensure the composer hears a near-finished sound.
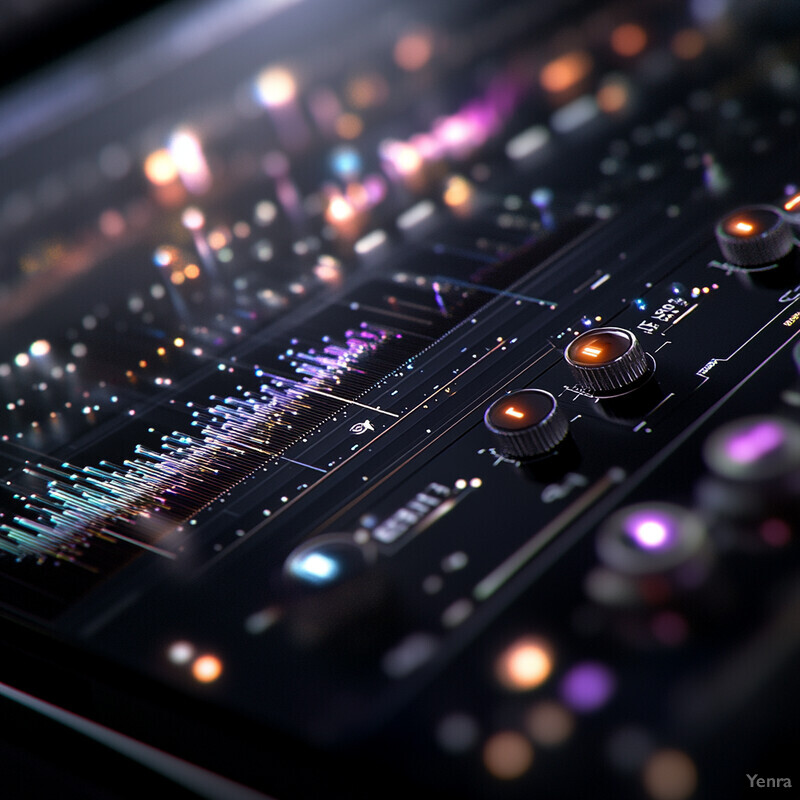
While mixing and mastering primarily concern audio engineering rather than compositional structure, the creative process often benefits from hearing a piece in a near-finished sonic state. AI-driven mixing and mastering assistants analyze the frequency spectrum, dynamic range, and balance levels of professional recordings, applying similar processes to a composer’s draft. This ensures that even in early composing stages, the music sounds polished enough for accurate judgment of arrangement decisions. Hearing a realistic mockup with balanced EQ, spatial reverb, and proper loudness helps composers better understand the impact of their choices and can inspire further refinements in orchestration and structure.
11. Genre-Specific Arrangement Templates
AI systems trained on particular genres can provide ready-made 'skeletons' for drum patterns, bass lines, and harmonic rhythms.
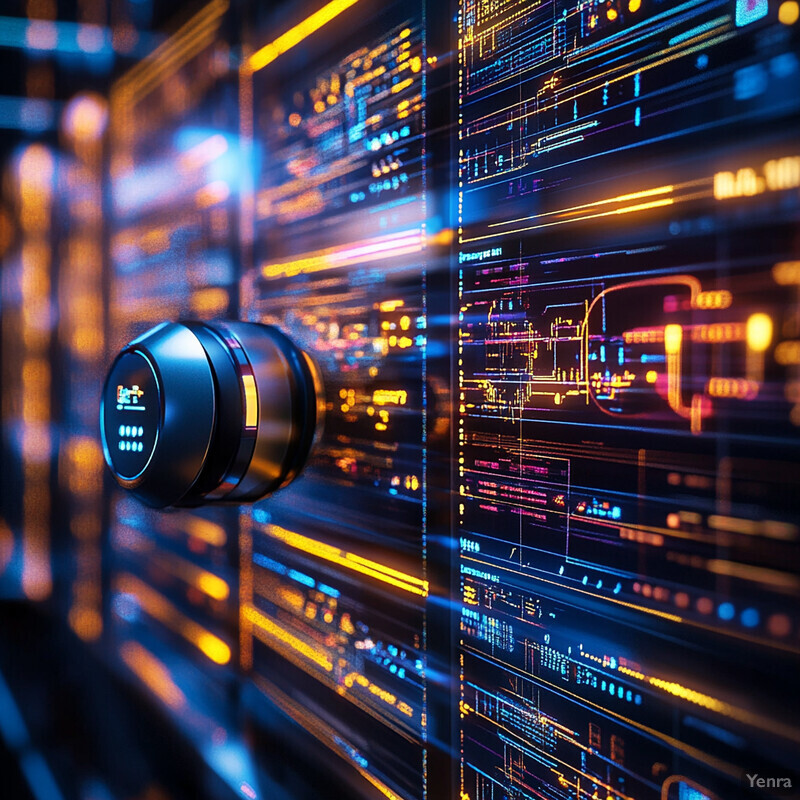
Many genres—like EDM, hip-hop, or a particular regional folk music—have established patterns for rhythm sections, bass lines, harmonic rhythms, and instrumental roles. AI tools that have studied extensive genre-specific repertoires can provide ready-made arrangement templates, acting as a starting point for composers. Instead of beginning with a blank slate, they receive a skeleton arrangement typical of their chosen style, which they can customize and build upon. This is especially useful for composers who wish to dip their toes into new styles without spending years learning the norms. By quickly establishing a genre-appropriate foundation, composers can focus on their unique thematic ideas rather than reinventing genre conventions from scratch.
12. Adaptive Loop Generation for Electronic Music
Tools can instantly generate loop variations—rhythmic, harmonic, or melodic—that evolve and adapt to a user’s input.
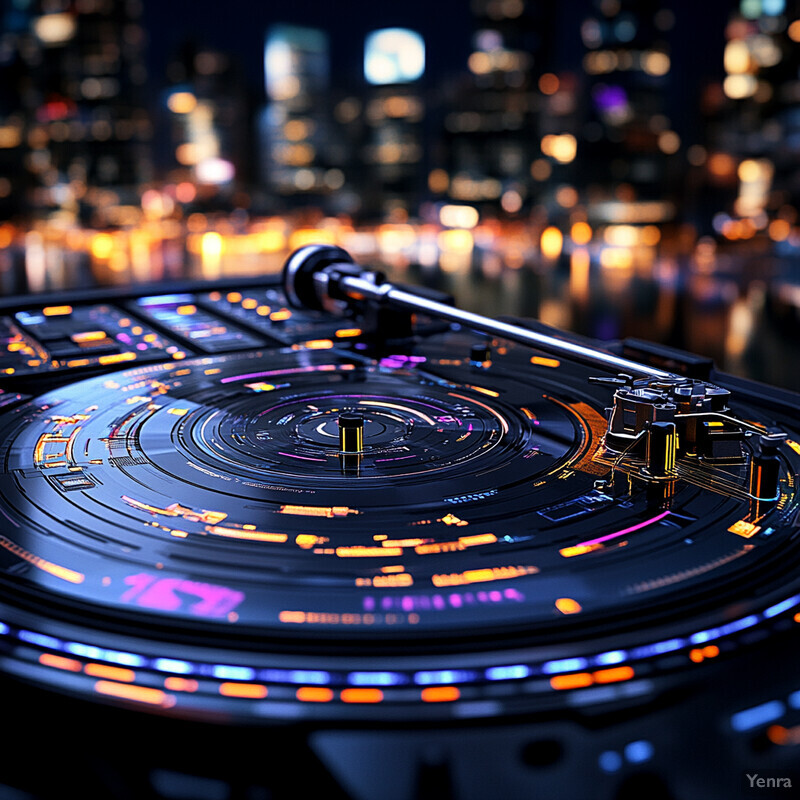
Loops—short repeated sections—are essential building blocks in electronic and dance music. AI can intelligently generate and adapt loops to suit evolving compositional contexts. Suppose a producer starts with a four-bar drum pattern. The AI can introduce subtle variations in the hi-hat pattern, layer in a complimentary synth bass, or suggest chord stabs to keep the loop from feeling stagnant. As the piece progresses, the AI adapts these loops, making them more complex or texturally dense, ensuring a dynamic and evolving soundscape that maintains the listener’s interest. By automating loop mutation, composers can rapidly explore different grooves, timbres, and moods without manually tweaking each iteration.
13. Improvised Continuation and Call-and-Response
Given a fragment of melody, harmony, or rhythm, AI can produce plausible continuations or 'responses.'
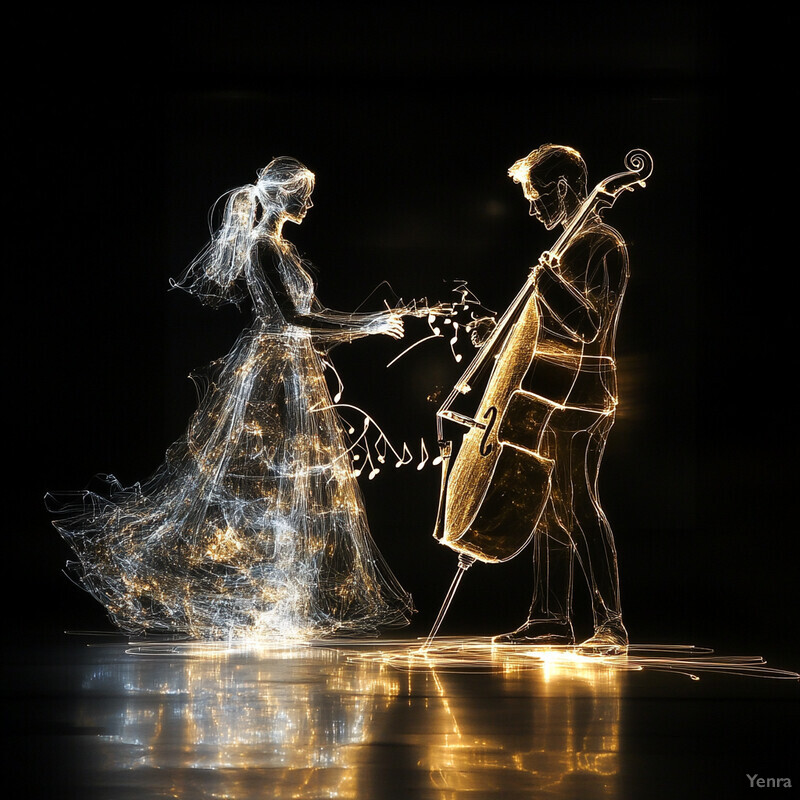
Improvisation is central to many musical traditions, and composers often benefit from a partner who can respond to their ideas spontaneously. AI can fill this role by taking a musical fragment—a phrase, a chord progression, a rhythmic figure—and generating a call-and-response pattern or continuing the material in an organic way. This back-and-forth between composer and machine can spark new directions, surprising harmonic detours, or more adventurous melodic contours. Essentially, the AI acts as a creative foil, offering fresh stimuli whenever the composer feels stuck. Over time, this interaction can lead to more inventive compositions that incorporate the spontaneity of improvisation into a structured work.
14. Lyrics and Text-Setting Guidance
AI can analyze text for its natural rhythmic and phonetic properties and suggest melodic phrases accordingly.
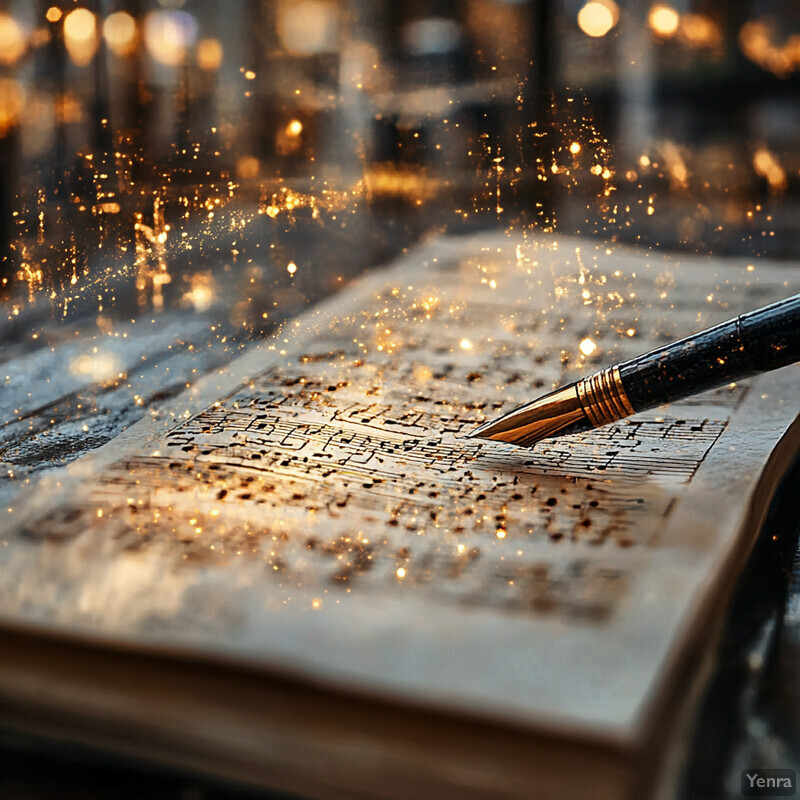
For vocal music, AI can analyze text for its natural rhythmic and phonetic properties and suggest melodic phrases, ensuring that lyrics fit comfortably and expressively within the musical setting. By aligning text with musical phrasing, the AI ensures that the final outcome feels both singable and expressive. If the composer provides a poem or a set of lyrics, the system might suggest a rising melodic line on an emotionally pivotal word or a rhythmic motif that mirrors the cadence of a sentence. This synergy speeds up the challenging process of finding a perfect match between language and melody.
15. Cross-Lingual and Cultural Stylistic Influence
By studying music from various cultures, AI can suggest unusual scales, timbres, and rhythmic cycles.
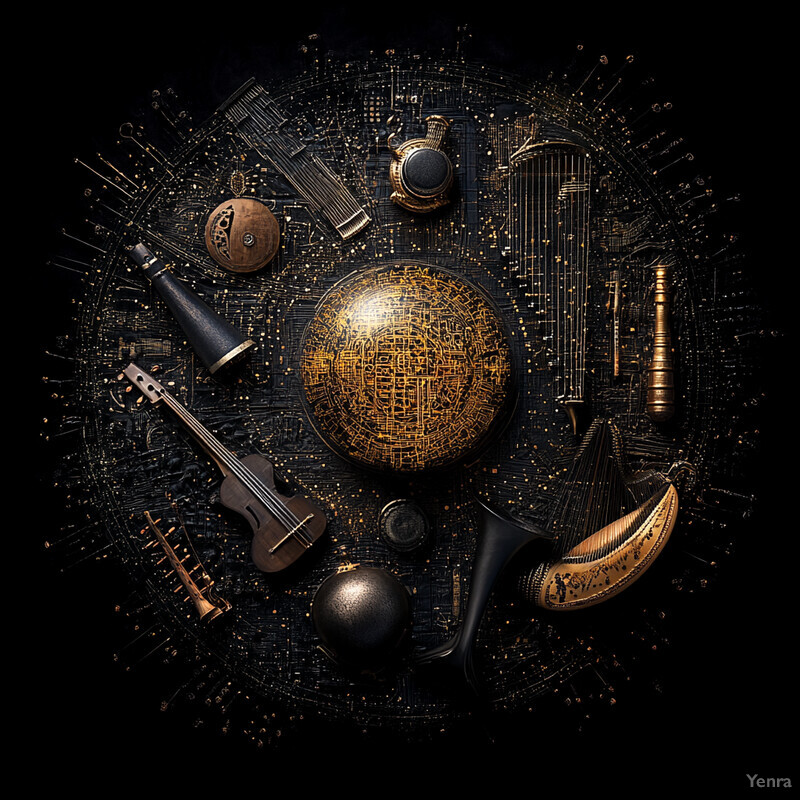
The musical world is vast and culturally diverse, encompassing various scales, modes, rhythmic cycles, and tuning systems. AI trained on wide-ranging repertoires can introduce composers to unfamiliar musical elements—e.g., the microtones of Middle Eastern makam traditions, the rhythmic complexity of Indian tala, or the pentatonic melodies of East Asian folk music. By suggesting scales, intervals, instrument combinations, or rhythmic patterns from different cultures, the AI broadens the composer’s horizon and encourages stylistic innovation. This capability can lead to rich intercultural collaborations and compositions that transcend conventional genre boundaries.
16. Complex Polyrhythm and Microtonal Support
Advanced AI models can work with intricate rhythmic structures or microtonal pitch sets.
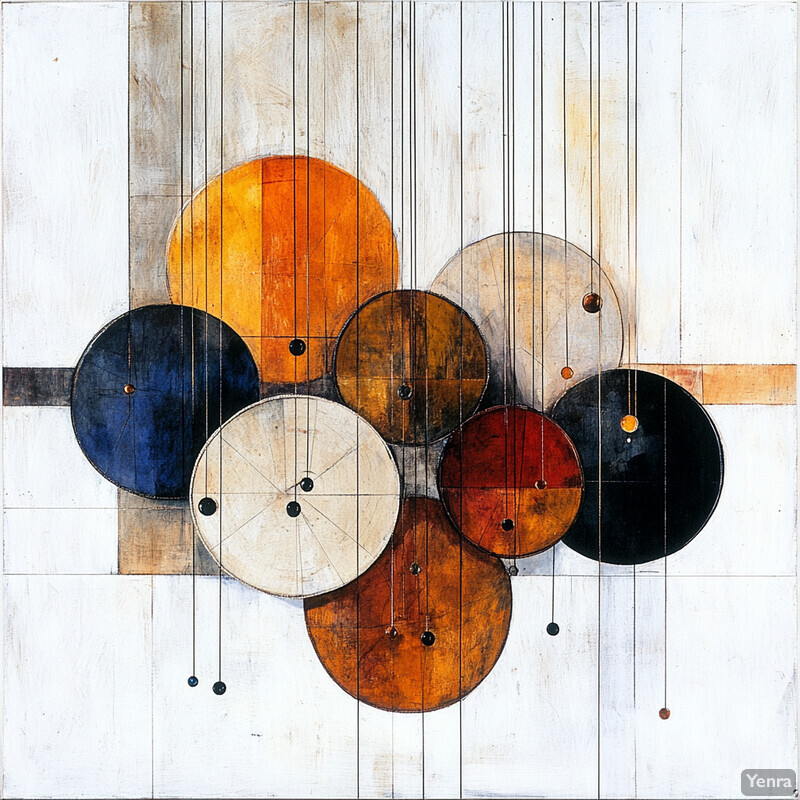
Exploring intricate rhythmic layers, odd meters, or non-standard tuning systems can be daunting. AI can serve as a guide through these complexities by suggesting polyrhythmic patterns or microtonal intervals that mesh well together. For example, if a composer wants to combine a 7/8 pattern with a 5/4 overlay, the AI can propose carefully aligned subdivisions or complementary rhythmic motifs. Similarly, when working with microtones, the AI can indicate which intervals might produce pleasing consonances or striking dissonances within a given microtonal framework. As a result, composers can confidently delve into advanced musical territories, supported by a tool that demystifies complexity and fuels experimentation.
17. Real-Time Adaptive Composition for Interactive Media
AI can rearrange and recombine musical fragments on-the-fly, ensuring the music matches a player’s actions or narrative states.
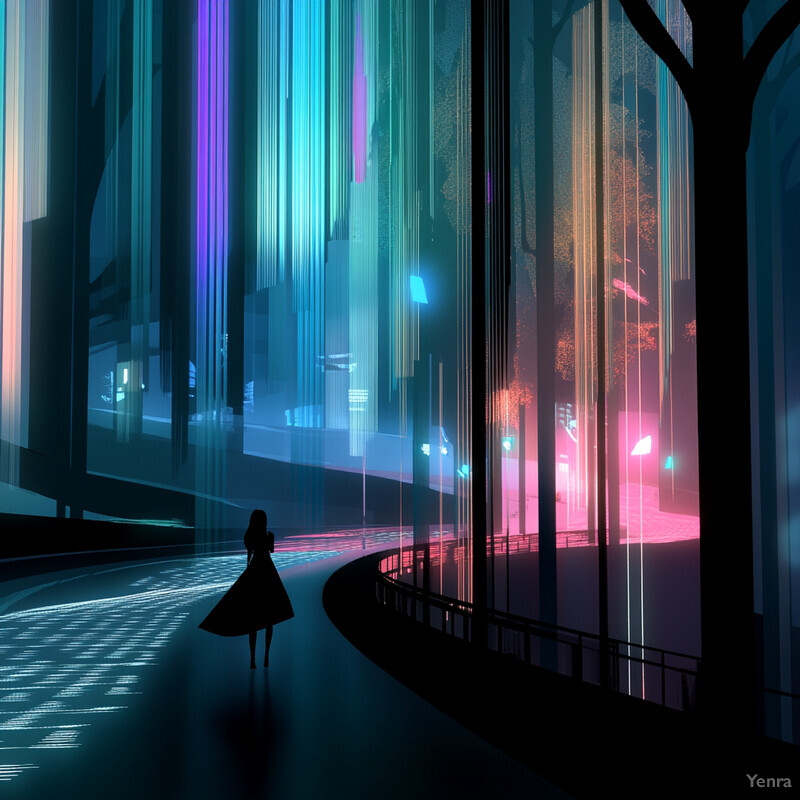
In gaming, virtual reality, and interactive installations, music must adapt to unpredictable user actions or environmental conditions. AI can reassemble, rearrange, and recombine pre-composed musical fragments on the fly, ensuring a seamless sonic experience that reacts to the moment. If the player enters a tense scenario, the music might gradually shift into a minor mode with insistent percussion; upon returning to a safe zone, it might revert to calmer textures. This dynamic, context-sensitive approach empowers composers to create branching musical narratives without needing to compose separate tracks for every possible outcome. Instead, they can rely on AI to intelligently integrate and adapt their material in real-time.
18. Streamlined Collaborative Workflows
Cloud-based AI composition tools can highlight discrepancies or harmonize conflicting ideas among collaborators.
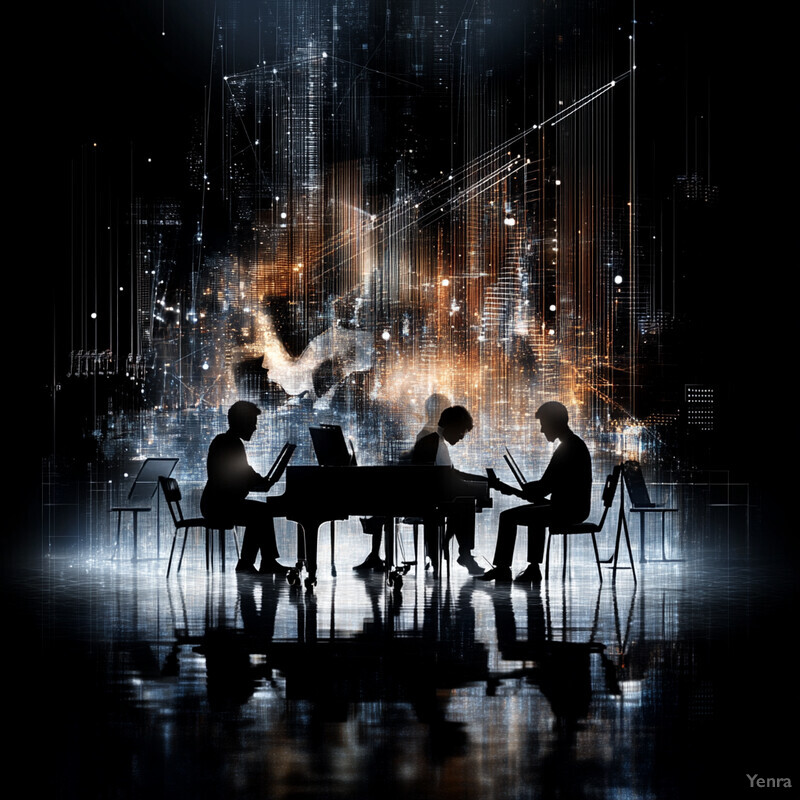
Large-scale projects—like film scores, musicals, or game soundtracks—often involve multiple composers, orchestrators, and music editors working together. AI can facilitate collaboration by highlighting inconsistencies in style, tempo, or harmonic language across different sections contributed by different team members. It can propose reconciliations, such as adjusting a chord progression or modifying a thematic statement to align more closely with the main themes. Furthermore, cloud-based platforms with integrated AI tools enable team members to share and refine ideas remotely. By ensuring internal coherence and offering compromise solutions, AI promotes a more efficient and harmonious collaborative environment.
19. Intelligent Transcription and Arrangement from Recordings
Audio-to-score AI technologies can swiftly convert recorded performances into notation and suggest instrumental arrangements.
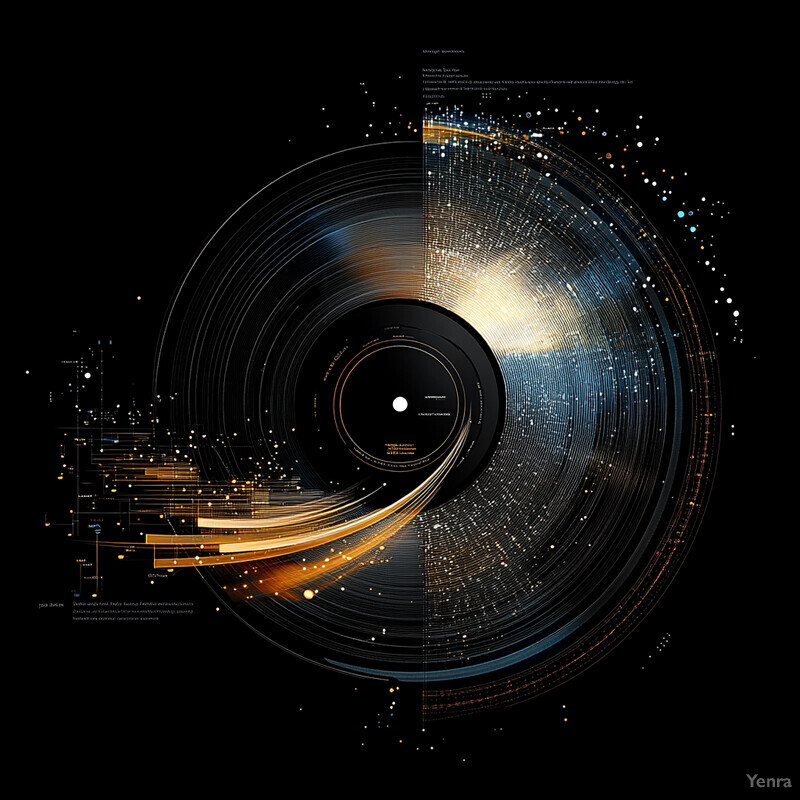
Turning a raw audio recording into a fully notated score is time-consuming. AI systems equipped with transcription capabilities can rapidly identify pitches, rhythms, dynamics, and timbral characteristics from audio. Once a piece is transcribed, the same system can suggest ways to arrange it for different ensembles or adapt it for new contexts. For example, a composer who recorded an improvisation on guitar can receive a piano score generated by the AI, along with suggestions on how to enrich the arrangement with woodwinds or strings. This accelerates the creative workflow and encourages composers to experiment with transforming their music into new formats and settings.
20. Personalized Learning and Feedback for Composers
Educational AI tools can assess a user’s compositions, providing targeted suggestions to improve form, voice-leading, and orchestration.
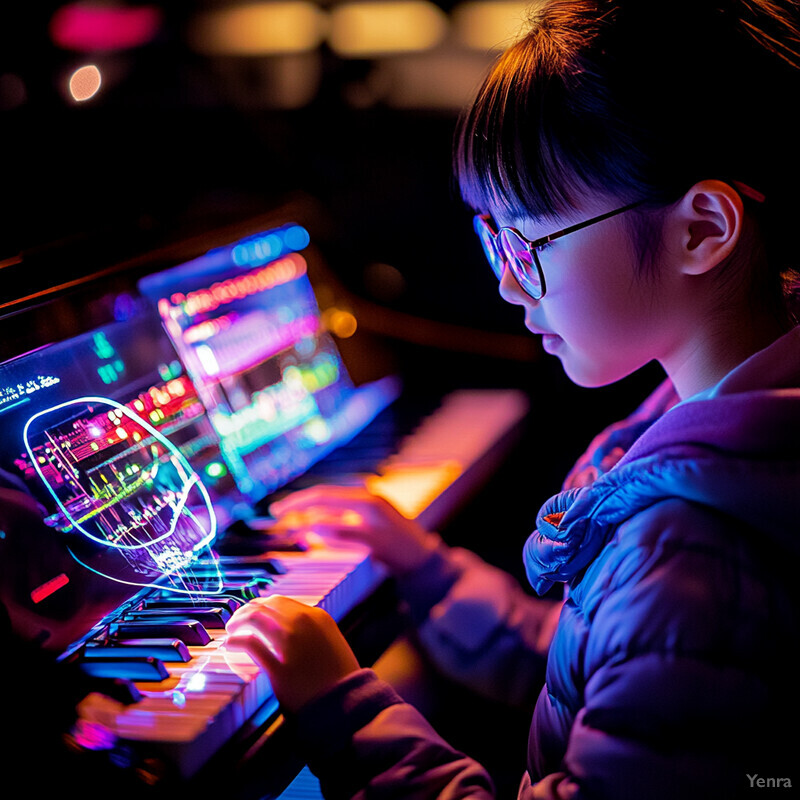
Educational AI platforms can function as virtual composition tutors, analyzing a user’s work for errors in voice-leading, weaknesses in thematic development, or imbalances in structure. The AI provides targeted feedback, highlighting sections that could benefit from more contrast, suggesting smoother harmonic connections, or recommending that a certain melody be reiterated for thematic coherence. This interactive coaching helps developing composers improve their craft by offering concrete suggestions based on best practices derived from extensive musical corpora. As the user progresses, the AI can adapt its advice, providing a personalized learning path that addresses each individual’s strengths and areas needing growth. This not only accelerates learning but also builds confidence, equipping composers with the skills to stand on their own feet creatively.