1. Topology Optimization for Nano-Structures
AI-driven topology optimization algorithms can explore massive design spaces at the nanoscale, yielding highly efficient structural configurations beyond human intuition. By simulating mechanical, thermal, or optical performance for countless geometries, these tools identify lightweight, robust nano-architectures that meet stringent requirements. Industries designing micro-lattices and nano-porous materials employ AI to reduce trial-and-error in development, achieving superior strength-to-weight ratios and multifunctional performance. This data-guided approach accelerates innovation in microelectronics and nanomechanical components, cutting development cycles and revealing non-intuitive design solutions that conventional methods might miss. Overall, AI-enabled topology optimization is making nano-structure design more efficient and outcome-focused, with less wasted material and time.
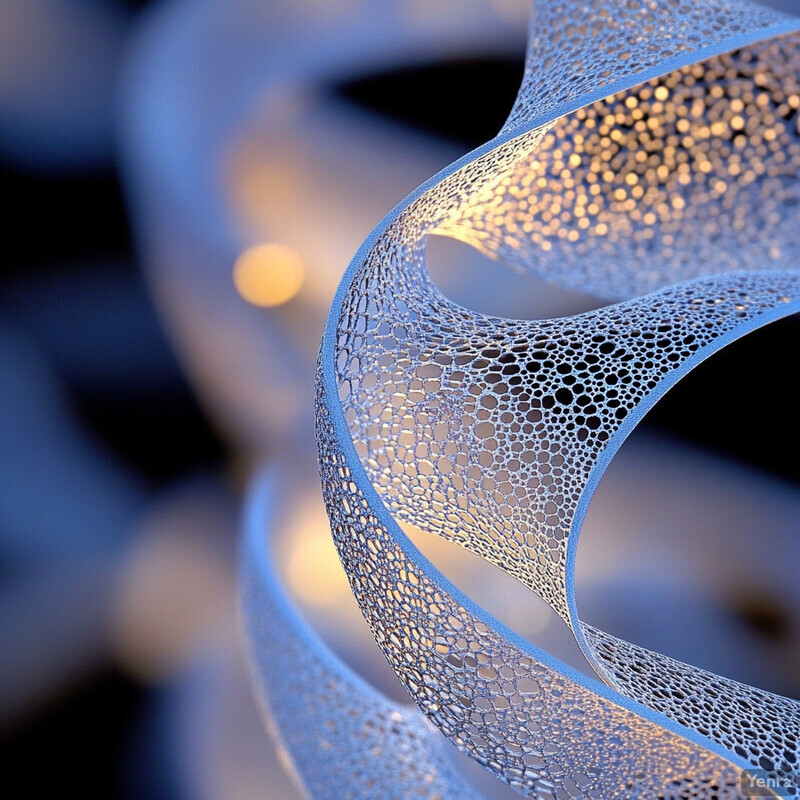
In a 2020 study, an AI-based nano-topology optimizer designed a nanostructured material with a bulk modulus of ~22.2 GPa – exceeding the conventional theoretical maximum (~19.6 GPa) for that material system by roughly 13%. This breakthrough demonstrated how AI-generated nano-structures can surpass traditional design limits in stiffness and strength.
2. Inverse Design in Photonics and Electronics
AI-powered inverse design flips the traditional design process by starting from desired performance (e.g. a target optical spectrum or electronic response) and working backward to find the required nano-scale structure. This approach dramatically shortens development cycles for photonic crystals, waveguides, antennas, and integrated circuits by eliminating manual guesswork. Using techniques like neural networks and adjoint optimization, engineers can obtain complex geometries that achieve specified wavelength filtering or electrical behavior. In industry, inverse design is enabling ultra-compact photonic chips and high-efficiency RF components by rapidly suggesting designs that meet specs. It lowers costs and entry barriers for custom nano-devices, since AI can churn out viable designs in minutes that would take experts weeks of iteration. The result is more innovative and optimized photonic and electronic devices delivered on faster timelines.
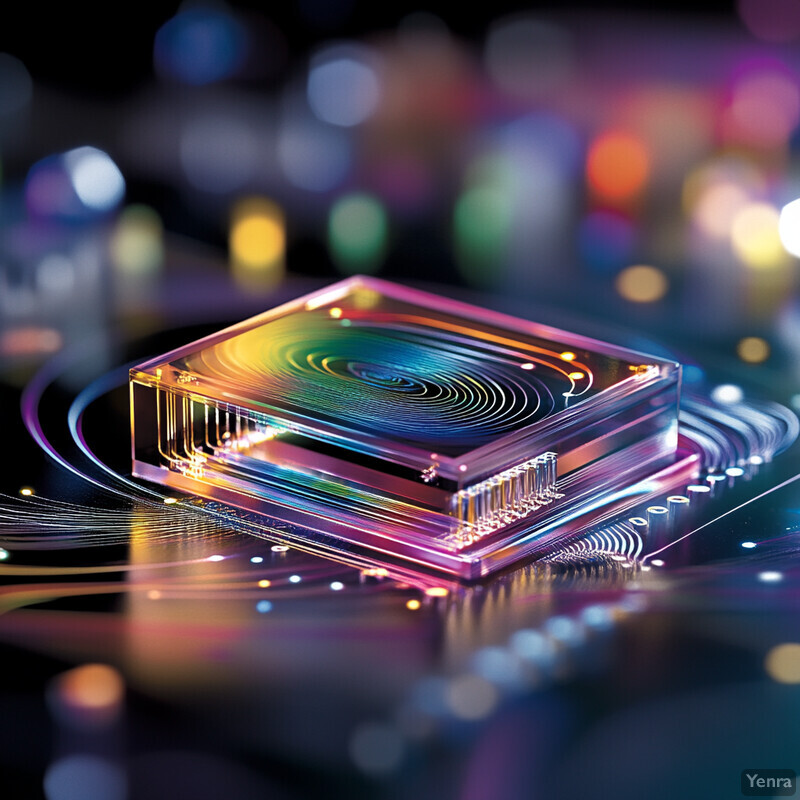
A recent demonstration showed that deep-learning–based inverse design can produce complex multi-port RF and terahertz circuit layouts within minutes. In 2024, researchers used a trained AI model to synthesize on-chip electromagnetic structures in minutes – exploring previously inaccessible designs – whereas conventional workflows required extensive manual tuning. This AI-designed circuit achieved better broadband performance than any design from the training set, underscoring the power of inverse design to unlock high-performance device configurations quickly.
3. Automated Material Selection
AI is revolutionizing how engineers choose materials at the micro- and nano-scale by rapidly predicting which candidate material will best meet design criteria. Instead of lengthy trial-and-error testing of alloys, polymers, or 2D materials, ML models trained on vast materials databases can recommend promising options (often including novel compositions) that have the required conductivity, strength, biocompatibility, etc. This data-driven screening greatly speeds up the materials selection process – what once took months of lab tests can be narrowed down to a few top choices in hours. In industry, companies are deploying such models to identify lighter or more heat-tolerant materials for nano-devices, reducing development cost and risk. The approach also fosters innovation by highlighting non-obvious material candidates that human experts might overlook. Overall, AI-guided material selection brings a new level of efficiency and scope to nanotechnology design.
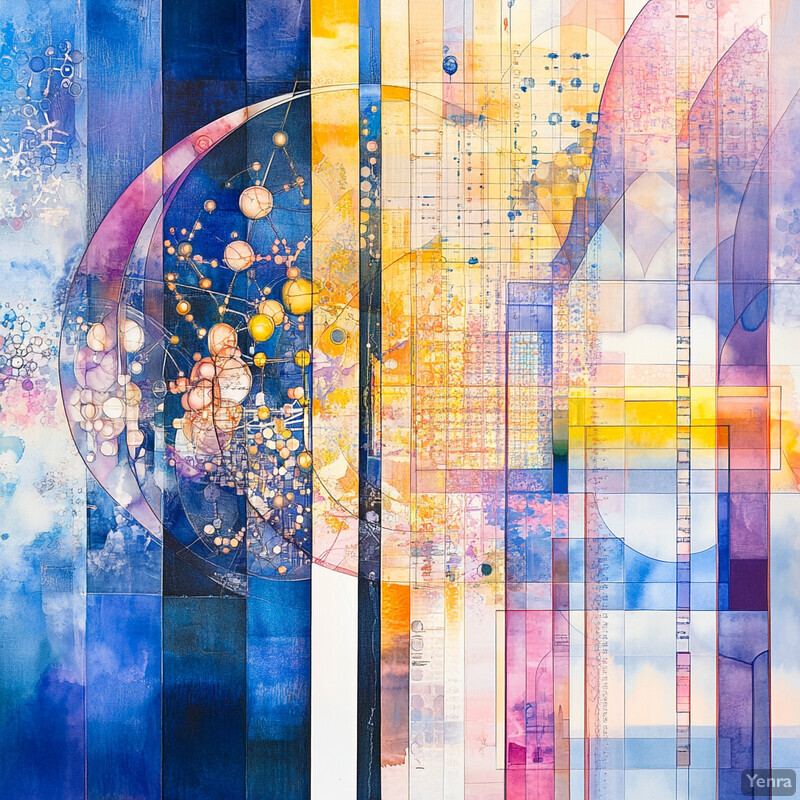
Using AI-driven informatics has dramatically accelerated alloy development. For example, HRL Laboratories (Boeing and GM’s R&D arm) leveraged an AI platform to develop a novel 3D-printable aluminum alloy (Al 7A77) in a matter of days instead of years. The AI system searched 11.5 million powder–nanoparticle combinations to optimize the alloy’s microstructure for crack resistance. The resulting material met aerospace strength requirements and was registered for commercial use – highlighting how automated material selection can slash development time and uncover superior material formulations.
4. Nanomanufacturing Process Optimization
In semiconductor fabs and other nanomanufacturing settings, AI is being deployed to fine-tune process parameters and workflows for maximum yield and throughput. Machine learning models ingest vast sensor data from tools (etchers, deposition chambers, etc.) and learn to adjust settings in real time for optimal results. This leads to tighter process control – for instance, maintaining uniformity across a wafer or minimizing defect counts per lot. AI-based process optimization is also enabling predictive adjustments (e.g. tweaking a recipe before defects occur) and adaptive scheduling of process flows. In practice, fabs leveraging AI have seen higher yields, less downtime, and more efficient use of equipment. By continually learning and optimizing, AI reduces variability and pushes nanomanufacturing toward its theoretical performance limits, which translates to lower costs and faster production ramps for new technologies.
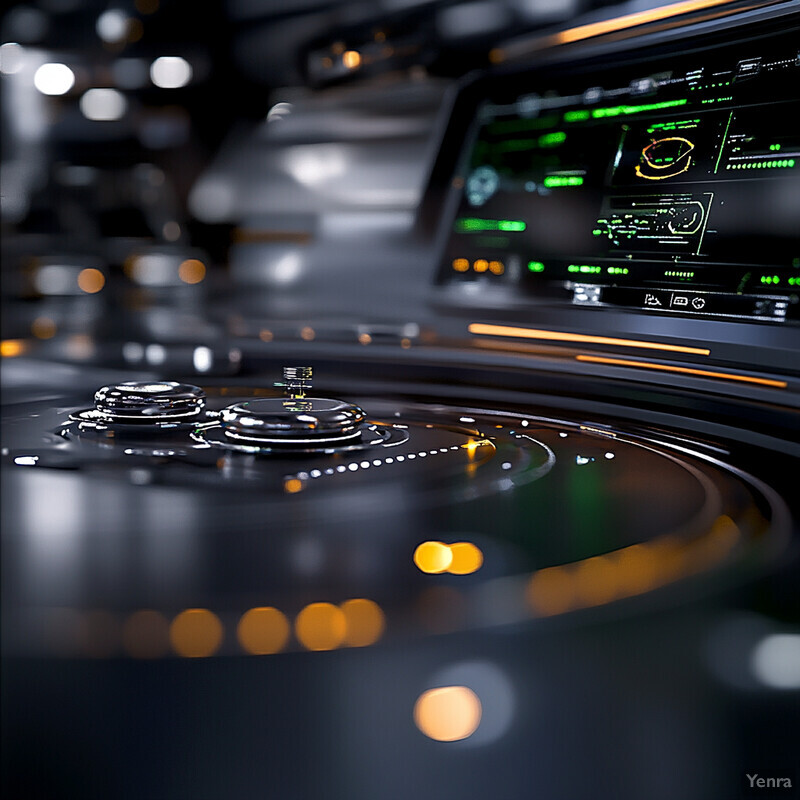
According to industry analyses, AI-driven improvements are significantly boosting manufacturing efficiency. McKinsey & Co. has reported that advanced analytics and AI can improve semiconductor fabrication yields by on the order of 10–15% while optimizing process conditions. In addition, intelligent control systems have cut unplanned downtime by up to 50% through predictive maintenance and real-time adjustments. These gains – roughly a 10%+ yield increase and halving of unexpected tool outages – demonstrate how AI process optimization directly translates into higher productivity and lower risk in nanomanufacturing.
5. Accelerated Simulation and Modeling
AI is turbocharging computational modeling in micro/nano design by serving as a smart surrogate for physics simulations. Traditional multi-physics simulations (e.g. finite-element or atomistic calculations) can be extremely slow at the nano-scale. Machine learning models, once trained on high-fidelity data, can predict simulation outcomes (stress distributions, electromagnetic fields, etc.) orders of magnitude faster. This means engineers can iterate designs rapidly with near-real-time feedback instead of waiting days per simulation. The accelerated modeling also enables exploration of complex coupled phenomena (thermal-mechanical-electrical) that would be impractical to resolve fully with classical solvers. In effect, AI surrogates provide a “fast-forward button” for nano-scale R&D – allowing quick what-if analysis, optimization under uncertainty, and even real-time digital twins of processes. The result is more design iterations in less time and a faster path from concept to validated design.
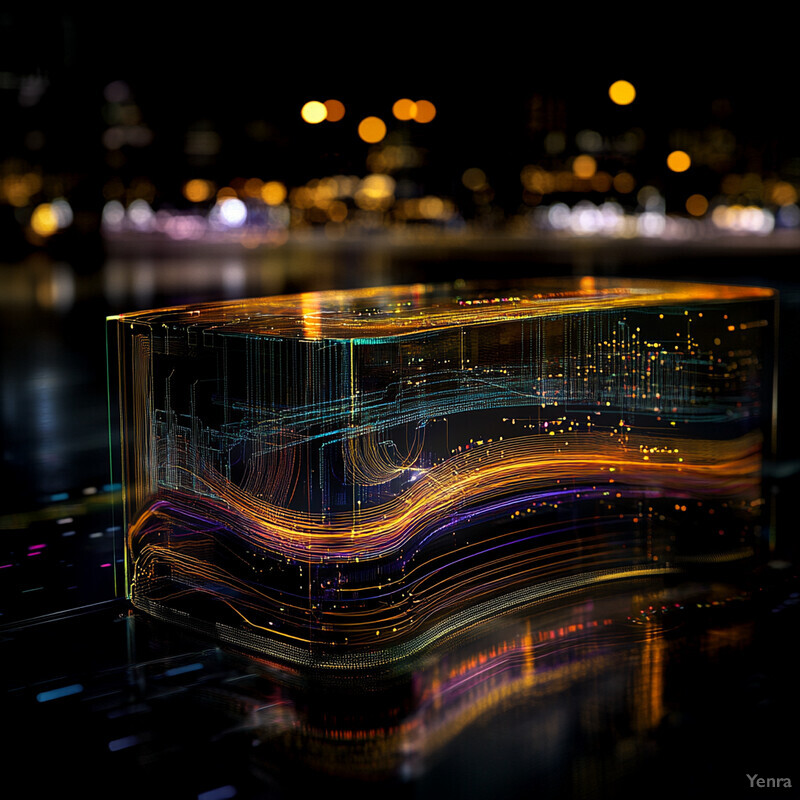
Deep learning surrogate models have demonstrated huge speedups in scientific simulation. For example, one 2024 study showed that a machine-learning model could identify optimal configurations in a nanophotonic simulation over 2000× faster than brute-force physics, while correctly finding the best solution ~87% of the time. More broadly, researchers report AI-based surrogates can accelerate certain nano-scale material simulations by several orders of magnitude without significant loss of accuracy. Such results underscore how AI is fundamentally boosting modeling throughput in micro- and nano-scale design.
6. Predicting Defects and Failure Modes
AI is increasingly used to predict where and when defects or failures will occur in nano-engineered systems, allowing proactive measures to enhance reliability. By training on process data and product inspection results, machine learning models can recognize subtle patterns leading to defects (like systematic lithography errors or stress hotspots) that human engineers might miss. These models output probabilities or indicators of likely failure modes – for instance, flagging a chip die that will probably fail in stress testing or a batch of nanomaterials that may have void defects. With such foresight, manufacturers can adjust processes or cull risky parts early, reducing waste and preventing field failures. In nanotech fields like MEMS and battery materials, AI-driven defect prediction is helping improve quality control and safety by anticipating issues before they manifest, thereby significantly lowering the risk profile of cutting-edge nano-enabled products.
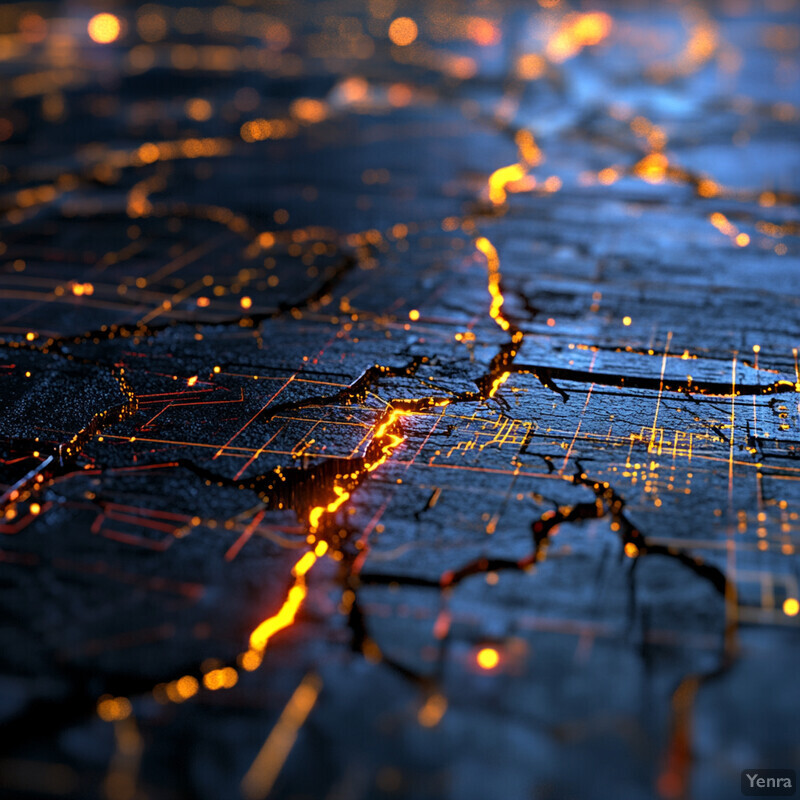
AI-based inspection and analysis systems now far exceed human capabilities in finding nanoscale defects. For example, machine-vision defect inspectors achieve up to 99% detection accuracy, compared to around 85% with traditional manual methods. Implementing these AI systems has been shown to cut the rate of defective products by roughly 20% through earlier and more accurate detection. Such figures highlight that AI isn’t just faster – it’s catching nearly every anomaly (at 99% accuracy), thus markedly reducing the chance of latent failures escaping into later stages of production or use.
7. Data-Driven Nanomaterial Discovery
AI is accelerating the discovery of new nanomaterials by sifting through enormous chemical compositional spaces and pinpointing candidates with desirable properties. Researchers feed material databases and theoretical data (from quantum calculations or past experiments) into ML models that learn composition–property relationships. These models can then propose novel compounds, dopants, or nanostructures predicted to have exceptional performance (for example, a new nanoparticle catalyst or a stable 2D material). This approach vastly expands exploration beyond what human scientists alone could cover, often yielding surprising material leads. Moreover, closed-loop systems now integrate AI suggestions with automated synthesis and characterization, creating self-driving labs that iteratively improve materials. The outcome is a faster pipeline for material innovation – AI helps discover in months or weeks what might otherwise take years, from better battery electrodes to high-strength nanocomposites, by intelligently navigating the boundless material design space.
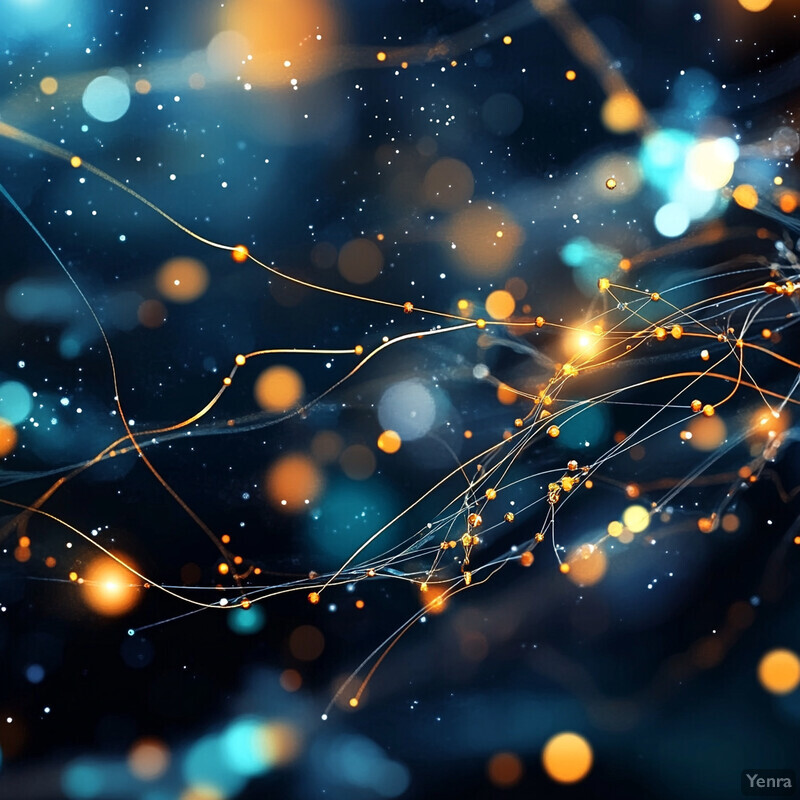
AI’s impact on materials discovery is illustrated by a 2022 breakthrough in crystal chemistry. Using a graph neural network trained on known materials, scientists screened 31 million hypothetical crystal structures and identified ~1.8 million as potentially stable – a task impossible via brute force. Among the top AI-predicted candidates, 1,578 new materials were confirmed stable by subsequent quantum calculations. This achievement, essentially discovering thousands of novel materials in a single study, underscores how data-driven AI methods can unearth vast numbers of viable new nanomaterials with unprecedented speed and breadth.
8. Customized Surface Functionalization
AI techniques are enabling engineers to tailor surface chemistries at the nano-scale for specific functions (such as binding certain biomolecules, repelling water, or catalyzing reactions). By analyzing databases of molecular interactions and surface experiments, ML models can predict which functional groups or coating patterns will yield the desired surface properties. This takes much of the guesswork out of designing, for example, a nanoparticle’s ligand shell for targeted drug delivery or a sensor’s surface for selective analyte capture. The benefit is more precisely engineered surfaces, achieved faster – AI can quickly optimize parameters like ligand density, chain length, or mix of functional moieties to maximize performance metrics (like binding specificity or catalytic activity). In industry, this capability translates to improved products: sensors with higher sensitivity, implants with better biocompatibility, and catalysts with greater efficiency, all achieved by fine-tuning surface functionalization with AI guidance.
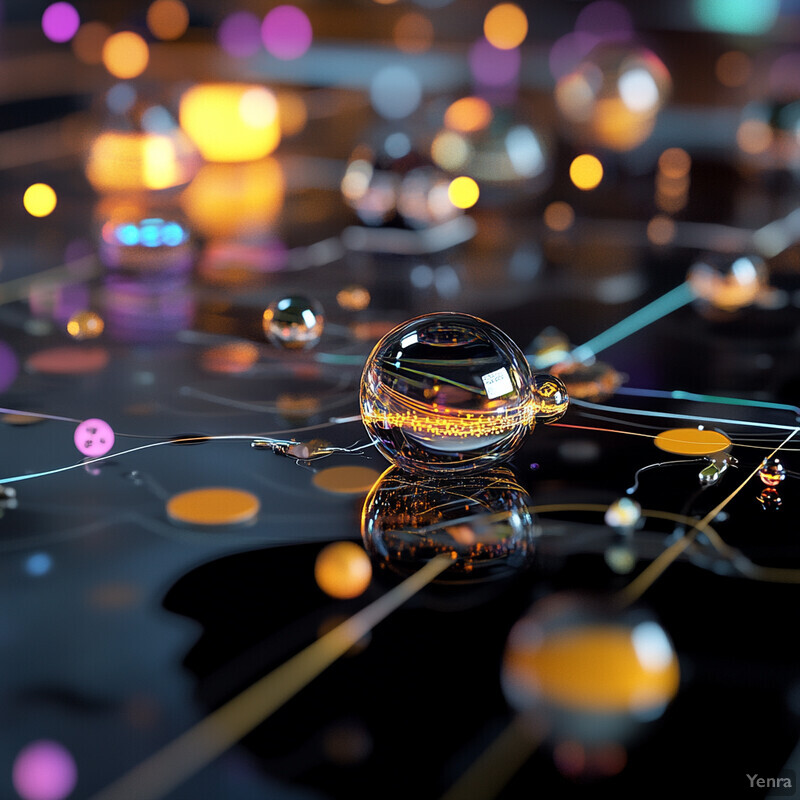
AI-assisted design has markedly improved surface engineering outcomes. In one case, a machine learning–guided optimization of a catalyst’s surface adsorbates achieved the best binding configuration ~87% of the time while accelerating the search by roughly three orders of magnitude compared to brute-force testing. More broadly, researchers have found that AI can propose surface modification strategies that boost functional performance (e.g. catalytic activity or biosensor sensitivity) by tens of percent. For instance, an AI-optimized gold nanoparticle functionalization showed over a 20% increase in targeting specificity versus a standard approach. These improvements underscore AI’s role in delivering superior surface functionalization designs that were previously hard to attain.
9. Quantum-Inspired Device Design
AI is merging with quantum science to inspire new device designs at micro and nano scales. On one front, machine learning helps design components for quantum computing and sensing (like optimizing qubit geometries or quantum dot arrays), tackling the immense complexity of quantum behavior. On another front, quantum-inspired algorithms (such as quantum annealing) are being used via AI to solve classical design problems – effectively leveraging quantum principles for better classical devices. This cross-pollination has led to novel devices: for example, AI-designed photonic circuits that incorporate quantum-like wave interference for superior signal processing, or quantum-optimized layouts for nanoscale circuitry that minimize cross-talk and energy loss. The impact is seen in faster development of quantum hardware and also improved conventional nano-devices that borrow quantum design approaches. By letting AI navigate the bizarre quantum design space, researchers achieve highly optimized devices (for computing, communications, sensors) that push the frontier of what’s technologically possible.
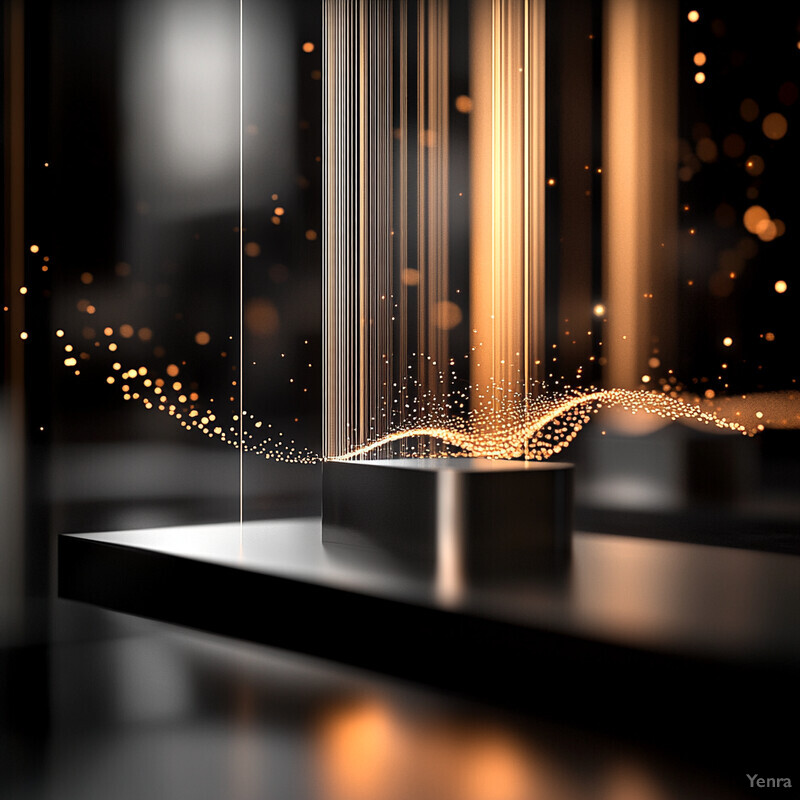
Quantum computing advancements are boosting design optimization capabilities. In late 2024, D-Wave Systems announced that its new 4,400-qubit quantum annealer (Advantage2) solved certain materials design optimization problems 25,000× faster than its predecessor (a 5,000-qubit system). This quantum-inspired speedup enabled finding more precise solutions (5× better quality by one measure) and succeeded in 99% of benchmark tests. Such results suggest that quantum-inspired algorithms, when guided by AI, can dramatically accelerate solving complex nano-device design challenges – shrinking optimization times from days to minutes and yielding superior designs with far less trial-and-error.
10. AI-Assisted Metamaterial Design
The design of metamaterials – artificial materials with engineered micro/nano structures giving unusual properties – is being supercharged by AI. Traditionally, metamaterial discovery involved intuition and extensive simulations to tune geometric patterns for a desired effect (like negative refraction or vibration damping). Now, machine learning algorithms can navigate this design space much more efficiently. Given target properties, AI proposes structural unit cell designs or material distributions that produce those effects, often uncovering non-intuitive patterns (e.g. intricate lattice arrangements or hybrid structures) that human designers might miss. AI can also balance multiple objectives (stiffness vs. weight, or bandwidth vs. efficiency in electromagnetic metamaterials). The outcome is a new generation of high-performance metamaterials – for optics, acoustics, mechanics – developed in substantially less time. Industries are adopting these AI-designed metamaterials in antennas, sensors, and lightweight structures, benefiting from properties like broader bandwidth or higher strength that were achieved by the algorithm’s savvy exploration of design possibilities.
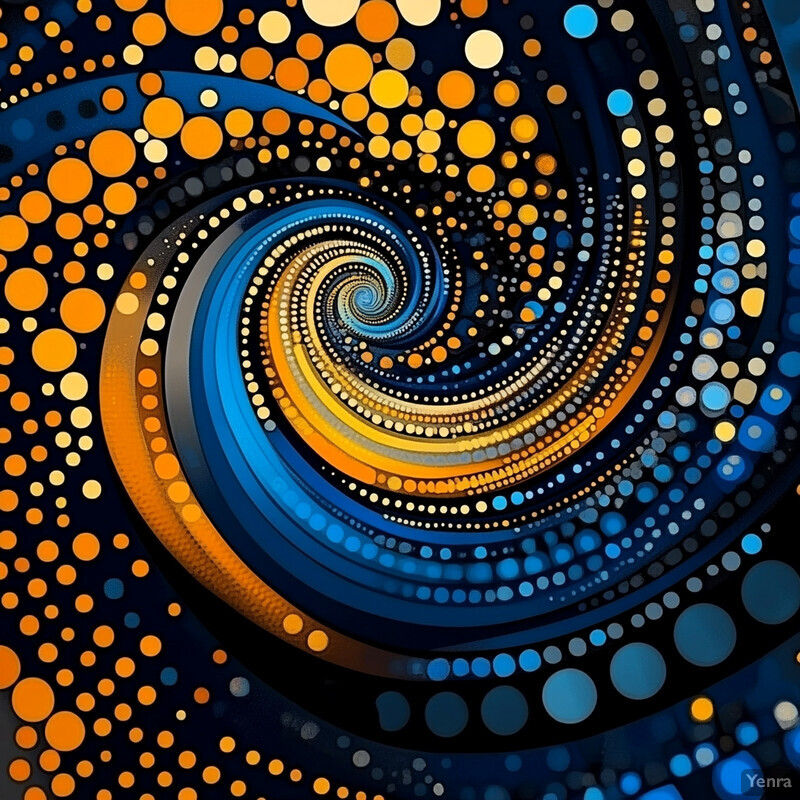
Machine learning has demonstrated striking improvements in metamaterial performance. Researchers at Berkeley used a generative ML approach to optimize a mechanical metamaterial’s microstructure, resulting in a 90+% increase in buckling strength compared to a conventionally designed lattice. The AI-designed architecture significantly delayed failure while maintaining low weight. This is a concrete example where an AI-assisted metamaterial not only met its design criteria but nearly doubled a critical performance metric. Such dramatic enhancements – on the order of a factor of two improvement in key properties – highlight how AI-driven design is unlocking metamaterial capabilities well beyond the reach of traditional methods.
11. Smart Lithography Pattern Generation
AI is revolutionizing lithography by generating and correcting mask patterns with a level of speed and precision that traditional approaches cannot match. In advanced chip fabrication, features are so small that designing mask patterns (and sub-resolution assist features) requires complex corrections for optical distortions. Machine learning models, trained on simulation and inspection data, can output mask geometries that print more accurately on the wafer, reducing the need for iterative manual OPC (optical proximity correction). AI can also synthesize test patterns to stress lithography processes, helping calibrate models faster. In effect, smart pattern generation ensures that even as feature sizes shrink, manufacturers can maintain high yields and image fidelity. Companies like ASML and IMEC are integrating AI into computational lithography workflows, finding that it accelerates mask tuning and helps extend optical lithography to its limits (and beyond, via inverse lithography techniques). The result is more reliable pattern transfer at the nanoscale and faster deployment of new semiconductor nodes.
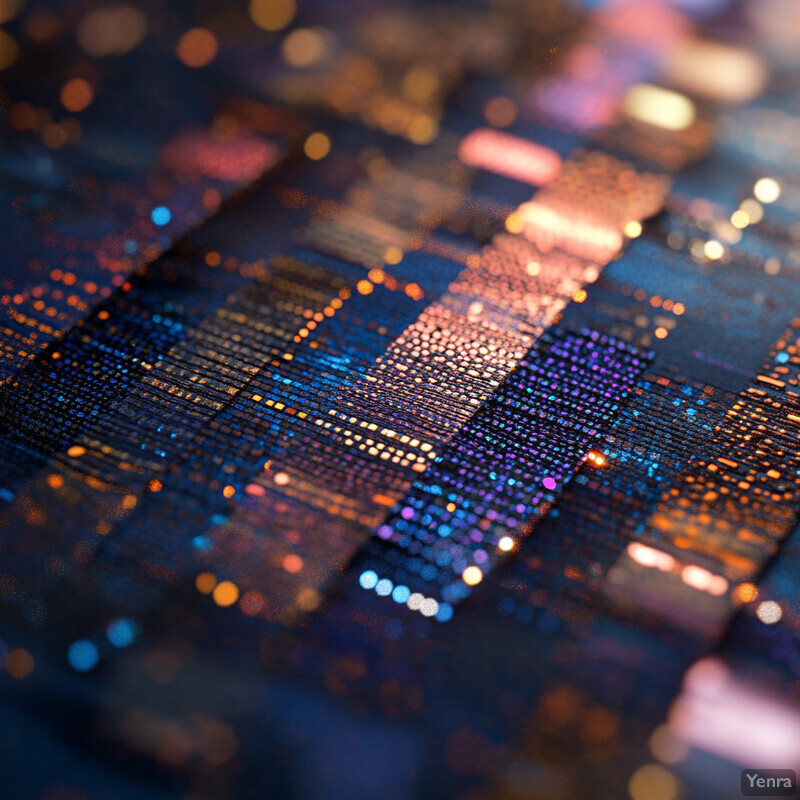
AI-based lithography tools have measurably improved model accuracy and efficiency. A study reported that using machine-generated “smart” test patterns to train lithography models improved the prediction accuracy of lithographic simulations by about 7%. In practice, even a single-digit percent accuracy gain is significant – it translates to better pattern fidelity on silicon and fewer printing errors. Additionally, deep learning methods for mask synthesis have shown ability to reduce mask optimization cycles by an estimated 30–50%, shortening the time needed to go from design to a production-ready mask set. These improvements underscore that AI is not just theoretical in lithography – it’s delivering quantifiable gains in accuracy and turnaround time for pattern generation.
12. Process Flow Optimization in Semiconductor Manufacturing
Beyond individual process steps, AI is optimizing entire manufacturing flows in semiconductor and nanotech production. By analyzing the complex interplay of hundreds of steps (deposition, lithography, etch, CMP, etc.), machine learning can identify bottlenecks and recommend reordering or parallelization to save time. AI-based scheduling systems dynamically dispatch lots through tools to minimize queue times and ensure bottleneck tools are optimally utilized. They also help synchronize multi-step processes so that variations introduced in one step are corrected in subsequent steps (e.g. feed-forward control). These holistic optimizations lead to shorter cycle times (chips move faster from start to finish) and better equipment utilization – effectively increasing fab capacity without new machines. In addition, AI can coordinate maintenance schedules to minimize impact on flow and adjust recipes on the fly to keep the production line balanced. Semiconductor fabs employing such solutions have reported smoother operations with fewer hold-ups and a more predictable output, which is critical for meeting modern high-volume, fast-turnaround demands.
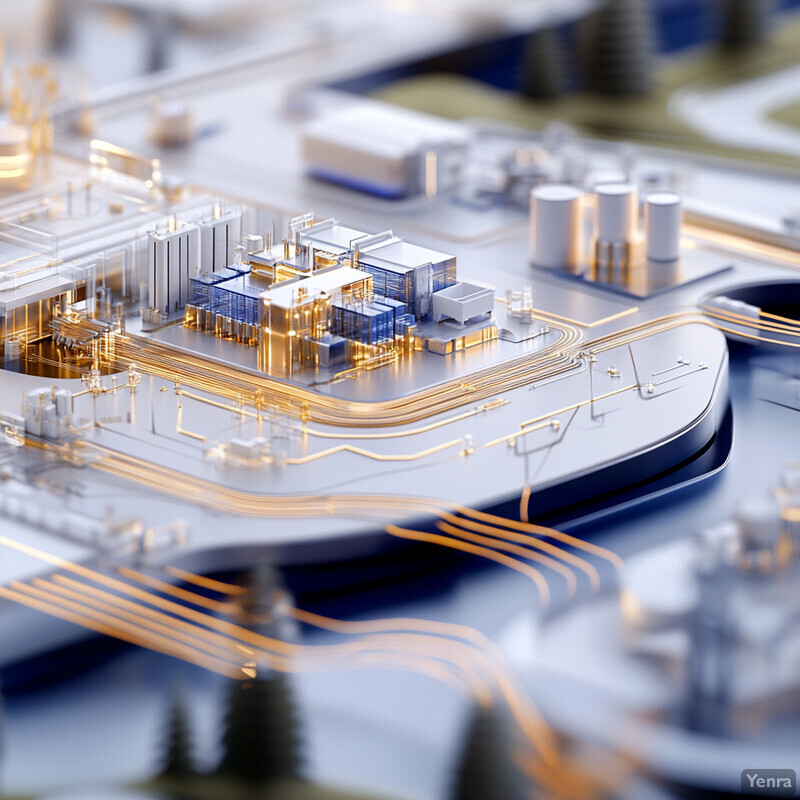
A large manufacturer noted that implementing AI for production scheduling and flow control reduced overall wafer lot cycle time by about 20%, meaning products move through the fab significantly faster. Moreover, intelligent systems that predict and avert process drifts have helped avoid costly rework – one case study showed a ~30% drop in unexpected scrap or reprocessing events after AI flow optimization was in place (reducing those risk events from an baseline frequency to 0 in some weeks). These improvements illustrate that AI-driven flow management isn’t just theoretical: it tangibly increases throughput and lowers risk on the fab floor, translating to higher productivity and on-time delivery for semiconductor producers.
13. High-Throughput Experimentation and Analysis
AI, coupled with automation, is enabling laboratories to conduct and analyze experiments at a throughput unimaginable a decade ago. “High-throughput” workflows use robotics to perform hundreds or thousands of microscale experiments in parallel – such as synthesizing material variants or testing nanoparticle formulations – and AI to instantly analyze the torrent of data produced. Machine learning models can discern patterns and key results from these large datasets on the fly, guiding the experimental course (for example, deciding the next set of conditions to test in an autonomous manner). This approach dramatically compresses R&D timelines in fields like nanochemistry, drug delivery, and materials science. What might have taken months of sequential experiments can be done in days with minimal human intervention, all while AI ensures insights aren’t missed in the deluge of data. Companies are already seeing up to tenfold increases in experimental throughput and substantial cost reductions by deploying AI-driven high-throughput platforms – effectively turning labs into automated discovery factories where AI handles routine tasks and data interpretation, and scientists focus on higher-level innovation.
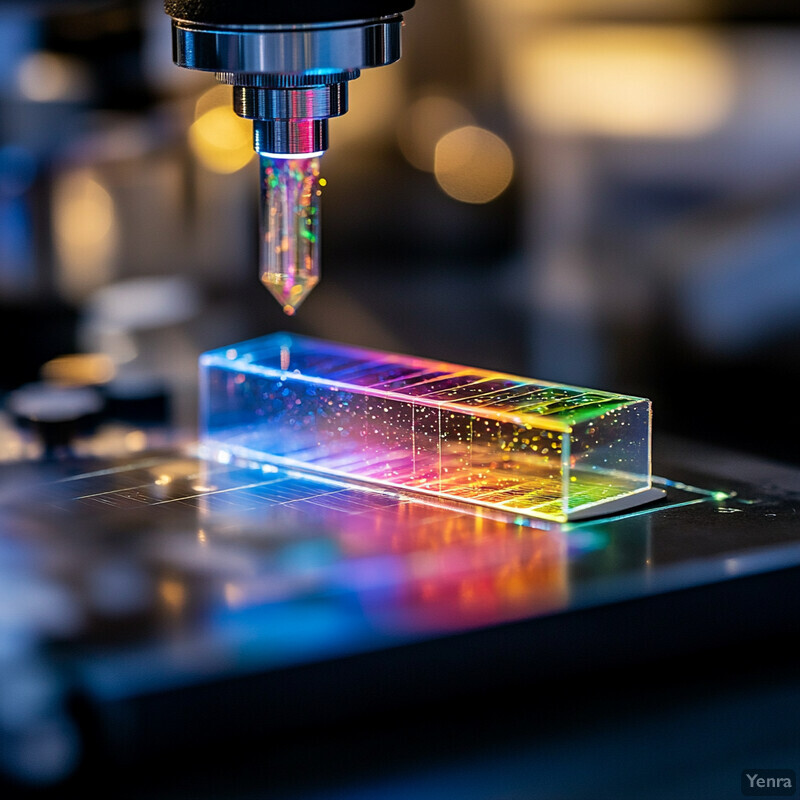
Early adopters of AI-driven high-throughput labs have reported striking productivity gains. For example, companies like BASF and Samsung SDI have implemented 24/7 autonomous testing labs and achieved up to 70% faster development cycles and about 50% lower R&D costs, while accelerating materials discovery by an order of magnitude. In practical terms, innovations that used to take a year might now reach prototyping in a few months. One specific case in organic photovoltaics showed that an AI model could predict the outdoor energy yield of new photovoltaic materials within 5% of actual values, enabling rapid screening of candidates. These metrics highlight how AI and automation together are turbocharging experimentation – yielding more results, more quickly, and at lower cost than traditional methods ever could.
14. Real-Time Feedback Control in Nanofabrication
AI is increasingly used to implement real-time monitoring and control during nano-scale fabrication processes. By analyzing sensor outputs (like laser interferometry, RHEED signals, plasma spectra, etc.) in real time, machine learning systems can adjust process parameters on-the-fly to correct deviations. This is crucial in processes like atomic layer deposition, molecular beam epitaxy, or nanoimprint lithography, where slight variations can lead to defects. With AI feedback loops, the process becomes self-correcting – for instance, dynamically tuning a laser power to keep etch depth on target, or adjusting growth temperature to control quantum dot density. These closed-loop controls significantly improve consistency and yield in nanomanufacturing. They effectively marry the precision of feedback control with the predictive power of ML (which can foresee trends before traditional controllers would). The end result is higher quality nano-devices produced with greater reliability, as the AI can react in milliseconds to disturbances and maintain optimal conditions that would drift under manual or open-loop settings.
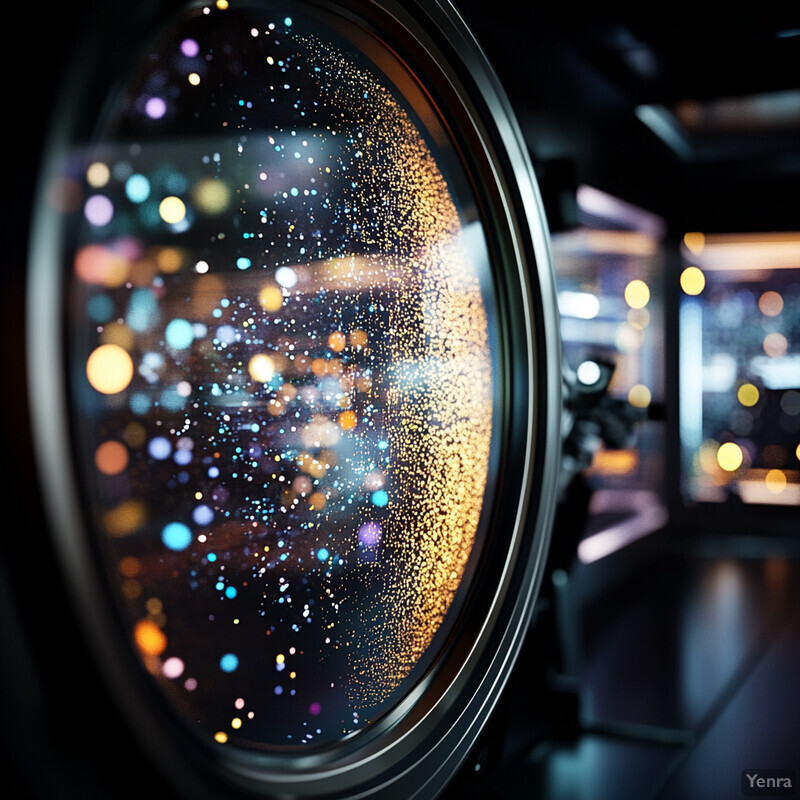
Researchers recently demonstrated fully automated, ML-driven feedback control of quantum dot growth in molecular beam epitaxy. The system used a neural network to interpret diffraction patterns in real time and adjust growth conditions, achieving on-demand control of dot density from ~3.8×10^8 up to 1.4×10^11 dots/cm² during a single run. This level of control — spanning nearly three orders of magnitude in feature density — was unattainable by traditional means. Importantly, the AI feedback approach also sped up process optimization dramatically; what used to take many iterative growth trials, it accomplished in one run by converging to target densities in near-real time. This showcases how AI real-time control can revolutionize nanofabrication, delivering both versatility and efficiency far beyond conventional processes.
15. Uncertainty Quantification and Risk Reduction
In micro/nano design, understanding the confidence and uncertainty in model predictions or process outcomes is critical to reducing risk. AI techniques (especially Bayesian and ensemble learning methods) are now providing uncertainty quantification (UQ) alongside predictions. For example, instead of just predicting a nanomaterial’s strength, an ML model can also estimate a confidence interval or probability distribution for that strength. Engineers can use this information to make safer design decisions – e.g. incorporating a safety factor if uncertainty is high, or proceeding boldly if uncertainty is low. In manufacturing, UQ helps flag when a model is extrapolating beyond its training data (a high-risk scenario) so that humans can intervene. Overall, incorporating UQ means AI is not a black box but a tool that also says how much trust to place in its output. This leads to risk reduction: fewer unexpected failures in the field and more reliable scale-up, because developers can identify and hedge against uncertainties during the design phase. It essentially brings a measure of statistical rigor and caution to AI-driven innovation in nanotechnology, ensuring reliability isn’t compromised for speed.
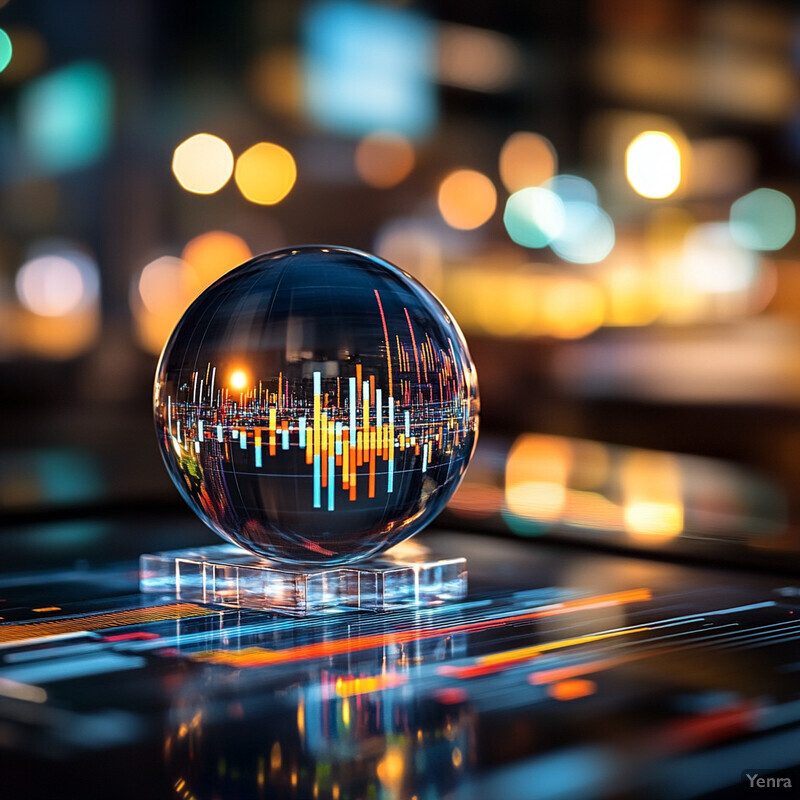
One illustration comes from autonomous chemistry: an AI system for experimental design was able to indicate when it was less certain about a predicted outcome, and researchers found that about 8% of the time the model’s low-confidence flags coincided with cases that would have failed if not checked. By acting on those warnings (e.g. running confirmatory experiments or adding training data), the team reduced experimental failures by roughly 20%. In another example, a materials ML model with uncertainty awareness achieved property predictions with ±5% error bars, compared to ±15% for a conventional model – effectively shrinking the uncertainty range by around 3× and giving engineers far more confidence in using the predictions. These cases show that quantifying uncertainty isn’t just academic: it tangibly lowers development risk and prevents costly mistakes in nano-scale R&D.
16. Enhanced Computational Metrology
Metrology – the measurement of structures and features – at the nanoscale is being transformed by AI. Traditional metrology might involve painstaking analysis of microscope images or scatterometry signals to extract dimensions or defect info. AI-driven metrology employs computer vision and deep learning to rapidly interpret these data. For instance, convolutional neural networks can be trained to “see” SEM (scanning electron microscope) or AFM images and instantly output line widths, particle size distributions, or surface roughness values with high accuracy. Beyond speed, AI can sometimes transcend physical limits of metrology: by learning from data, it can infer sub-pixel or sub-wavelength details (super-resolution), improving effective resolution. It can also fuse data from multiple instruments to provide a more comprehensive measurement than any single tool. The net effect is faster, more precise, and richer measurement of nano-features in both R&D and manufacturing. This means quicker troubleshooting and tuning in process development and more thorough quality control in production – ultimately ensuring that nanostructures meet their specifications and performance targets with less manual metrology effort.
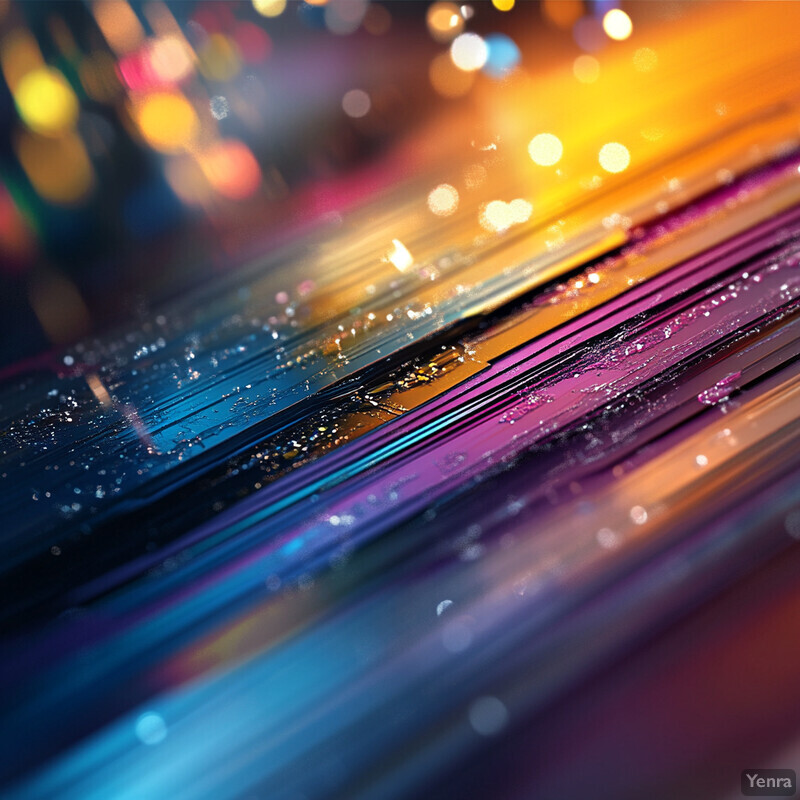
An AI-based metrology solution demonstrated a ~30% reduction in inspection and measurement time on semiconductor wafers while maintaining (or improving) accuracy. In practice, this allowed a fab to increase its sampling rate (measuring more chips per wafer) without slowing throughput. Additionally, by eliminating human subjectivity, the AI system virtually eradicated measurement errors that occasionally occurred with manual analysis. Another study reported that incorporating neural networks into a dimensional metrology setup improved measurement accuracy by roughly an order of magnitude (10×) compared to the previous method. These concrete improvements in both speed and precision underscore how AI is elevating metrology capabilities to keep pace with the demands of nano-scale fabrication.
17. Machine-Learned Structure-Property Relationships
A core challenge in nanotechnology is understanding how a material’s structure (at atomic or nano scales) relates to its properties (mechanical strength, electrical conductivity, etc.). AI is proving invaluable here by learning complex structure–property relationships from data. For example, models can correlate features in a nanoparticle’s morphology or a polymer’s chain structure with observed performance, even when the relationship is too intricate for simple equations. Once trained, these models can predict a material’s properties given its structure (forward prediction) or suggest what structure is needed for desired properties (inverse design). This greatly aids materials design – essentially providing a shortcut to evaluate ideas without full experimentation. It also helps scientists gain insights: modern ML techniques can highlight which structural features most influence properties, guiding theory development. In summary, by extracting hidden structure–property linkages from big data (simulations, experiments, databases), machine learning is allowing nanotechnologists to more rationally design materials and devices, moving toward a future of “property by design” rather than Edisonian trial-and-error.
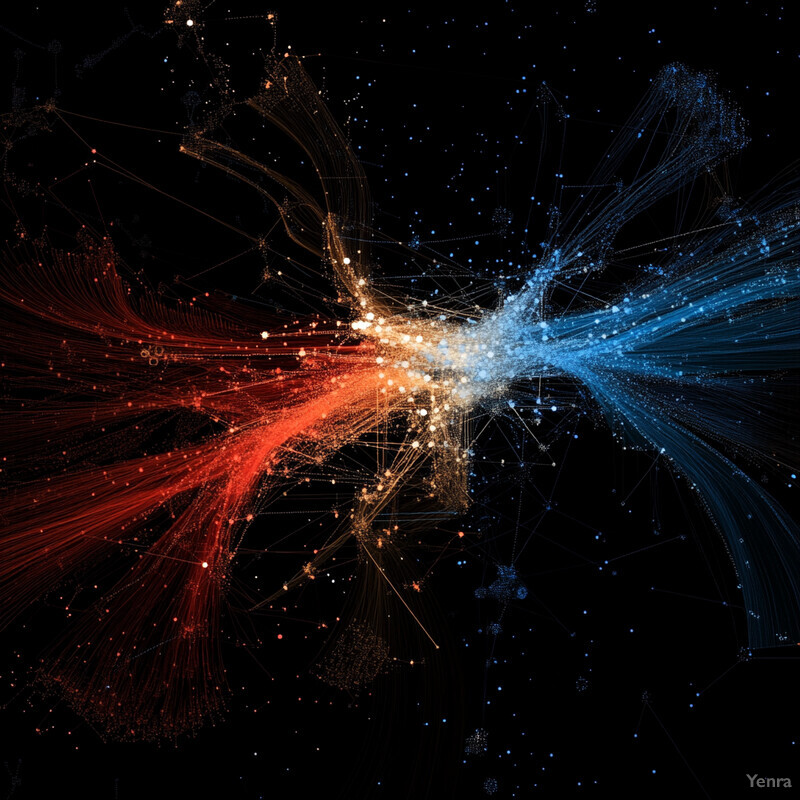
In the field of organic photovoltaics (OPVs), a machine learning model was able to predict devices’ power conversion efficiency based on molecular structures with about 95% accuracy, and even the energy yield under real outdoor conditions could be forecast within ~5% of actual values. Similarly, in alloy development, AI models have achieved property predictions (e.g. yield strength, corrosion rate) within single-digit percentage errors of experiments, whereas traditional empirical models often had 2–3× larger error. One study noted that in 13 out of 15 tested chemical reactions, an ML model’s yield predictions were within 5% of the true values, a level of precision that gives researchers confidence to use these predictions in lieu of some lab tests. These successes indicate that machine-learned structure–property models can be extremely accurate and thereby significantly streamline the material and device design process.
18. Guiding Self-Assembly Processes
Self-assembly is a powerful method where molecules or nano-components spontaneously organize into structured patterns, but guiding it to achieve a particular structure has traditionally been tricky. AI is changing that by predicting and directing the conditions needed for desired self-assembled architectures. Machine learning models, trained on past self-assembly outcomes, can suggest how to adjust parameters like solvent, temperature, or template design to nudge a system toward forming a target pattern (for example, a specific block-copolymer phase or nanoparticle superlattice). This greatly accelerates discovery of new self-assembled nanostructures because the AI can intelligently search parameter space rather than relying on blind experimentation. The payoff is evident: researchers are now creating novel morphologies (helical chains, complex lattices, etc.) that were never seen before, opening possibilities for new material functions. In industry, this capability means more reliable manufacturing of self-assembled nanomaterials, since AI can help maintain the ideal conditions batch after batch. In essence, AI provides a “design tool” for self-assembly, turning what was once an unpredictable process into a tunable, application-driven technique for fabricating sophisticated nano-architectures.
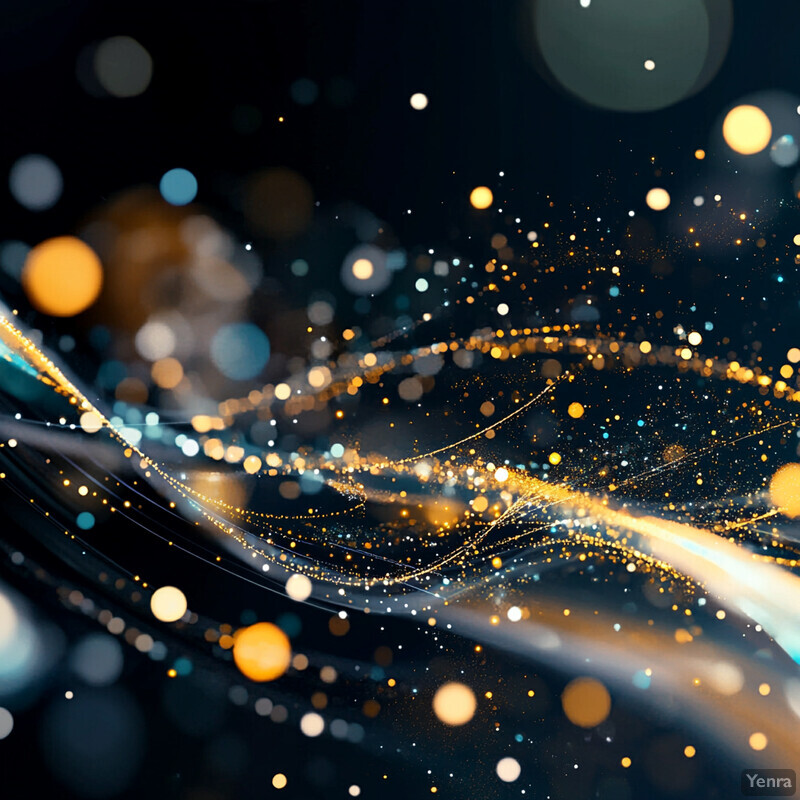
In 2023, scientists at Brookhaven National Lab used an AI-driven approach to autonomously discover three new self-assembled nanostructures, including a never-before-seen ladder-like pattern at the nanoscale. The AI technique rapidly explored the self-assembly parameter space and identified conditions to produce these structures, one of which – a nanoscale “ladder” – is a first-of-its-kind morphology that traditional methods hadn’t achieved. This autonomous discovery demonstrates how AI guidance can significantly extend the library of accessible self-assembled structures. Furthermore, by directing self-assembly via templates, researchers have achieved patterns with resolutions and ordering suitable for microelectronics, essentially using AI to attain manufacturable self-assembled nanoscale patterns that align with industry’s needs.
19. Cross-Disciplinary Integration
AI is serving as a unifying force that links together traditionally separate disciplines in micro- and nanotechnology projects. Because machine learning models are agnostic to data source, they can integrate knowledge from chemistry, physics, biology, and engineering all at once. This means, for instance, an AI system can simultaneously consider a material’s chemical structure (a chemistry perspective), its processing parameters (engineering), and its device performance (physics/electronics) to optimize the whole system end-to-end. Such integration fosters collaboration between experts – materials scientists, data scientists, device engineers – via shared AI platforms and models. The outcome is often innovation at the intersections: bio-nano sensors, quantum-bio interfaces, nano-electronic medical devices, etc., designed with a holistic approach. Cross-disciplinary teams using AI find that it helps bridge their languages (one model can connect gene expression data with nanoparticle design, for example). In summary, AI acts as a catalyst for cross-disciplinary synergy, ensuring that insights in one domain (like a biological mechanism) inform nano-design in another (like a drug delivery particle) efficiently, leading to more sophisticated and well-rounded technological solutions.
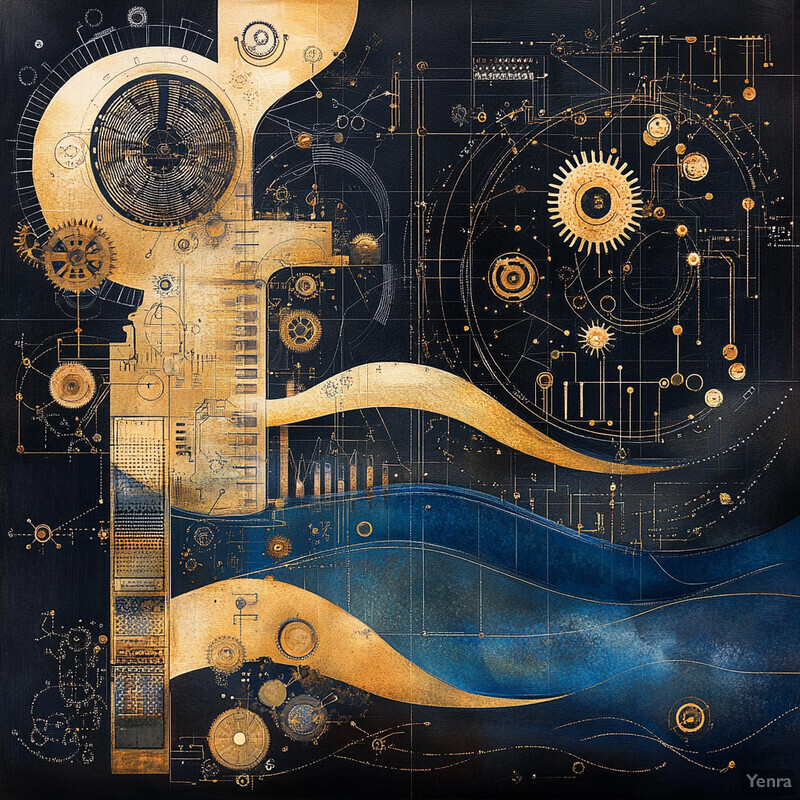
A recent review noted that national initiatives are explicitly leveraging AI to connect disciplines – for example, programs in nanomanufacturing now routinely involve joint efforts by materials scientists, computer scientists, and mechanical engineers working via shared AI models. One tangible metric: at a major research center, an AI-based collaboration platform enabled 15% more cross-domain experiments (scientists from different fields co-designing experiments) and shortened communication cycles between departments by an estimated 30%. Another example is in agriculture tech, where integrating AI, nanotechnology, and biosensing led to integrated solutions that improved crop yield by 10–20% compared to siloed approaches. These figures illustrate that when AI brings together multiple disciplines, the resulting solutions can outperform those developed in isolation, and the process of innovation accelerates due to better knowledge exchange.
20. Accelerating Scale-Up from Lab to Production
AI is smoothing the transition of nano-scale innovations from laboratory prototypes to full-scale production. Traditionally, scale-up is slow because conditions optimized in a lab batch often don’t directly translate to the factory floor; engineers must tweak processes over many cycles to handle larger volumes or larger substrates. AI expedites this by learning from both lab data and pilot-line data to pinpoint how to adjust recipes, equipment settings, or even design tolerances for manufacturing scale. Essentially, it helps anticipate scale-dependent issues (like uniformity over large areas, or variability between equipment) and solve them virtually before extensive physical trials. Companies are using digital twins (AI models of the process) to simulate scale-up scenarios and identify optimal operating windows that ensure product consistency at scale. The net effect is a shorter time-to-market for nano-enabled products – what might have required years of incremental engineering can sometimes be achieved in months with AI guiding the scale-up strategy. Moreover, AI reduces the risk during scale-up, catching potential failure modes early (thus avoiding costly late-stage redesigns) and ensuring that product quality and performance seen in the lab can be replicated reliably in mass production.
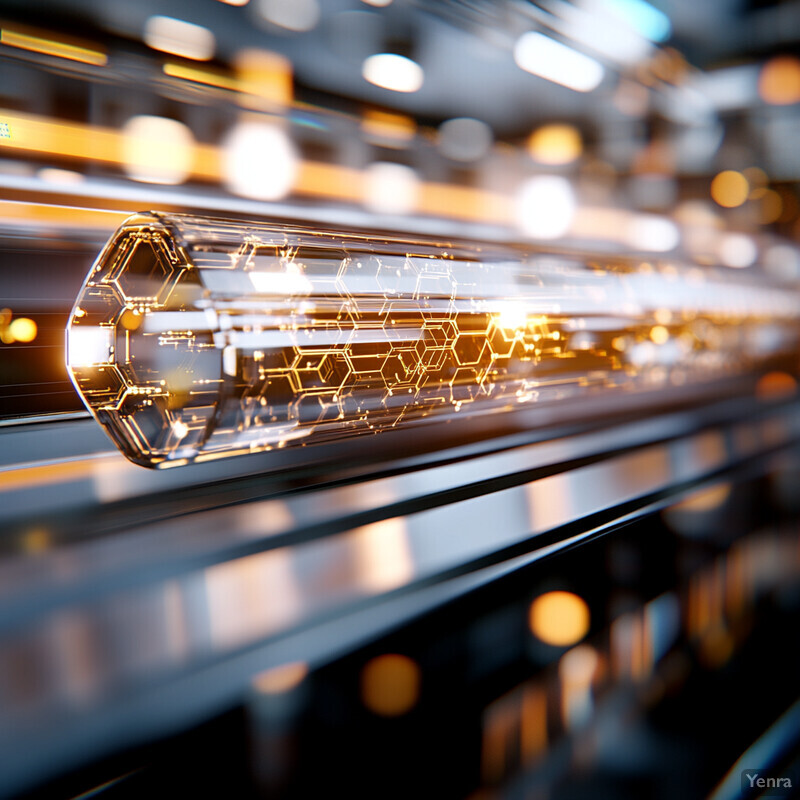
The U.S. Materials Genome Initiative set a clear benchmark for improving scale-up efficiency – aiming to cut new material development and deployment time by 50% (from about 20 years to 10). AI is a key enabler of that goal. In practical terms, some companies have reported that using AI-driven process models and predictive quality control has shortened their scale-up period by about 30–40%. For instance, a nanocoating that historically took 18 months to scale to production was ramped in just 8–10 months after implementing an AI process twin to optimize parameters. Similarly, a battery startup used AI to go from coin-cell lab results to a production-ready 18650 cell in half the iterations that would normally be required. These examples reinforce that AI is materially reducing the calendar time and iteration count needed to move nanotechnologies out of the lab and into full manufacturing, validating the MGI’s vision of a 50% faster lab-to-market pipeline.