1. Advanced Pattern Recognition in Microbiome Data
AI-driven algorithms can rapidly sift through vast sequencing datasets, discerning complex patterns and relationships within microbial communities that are otherwise too subtle or complex to detect manually.
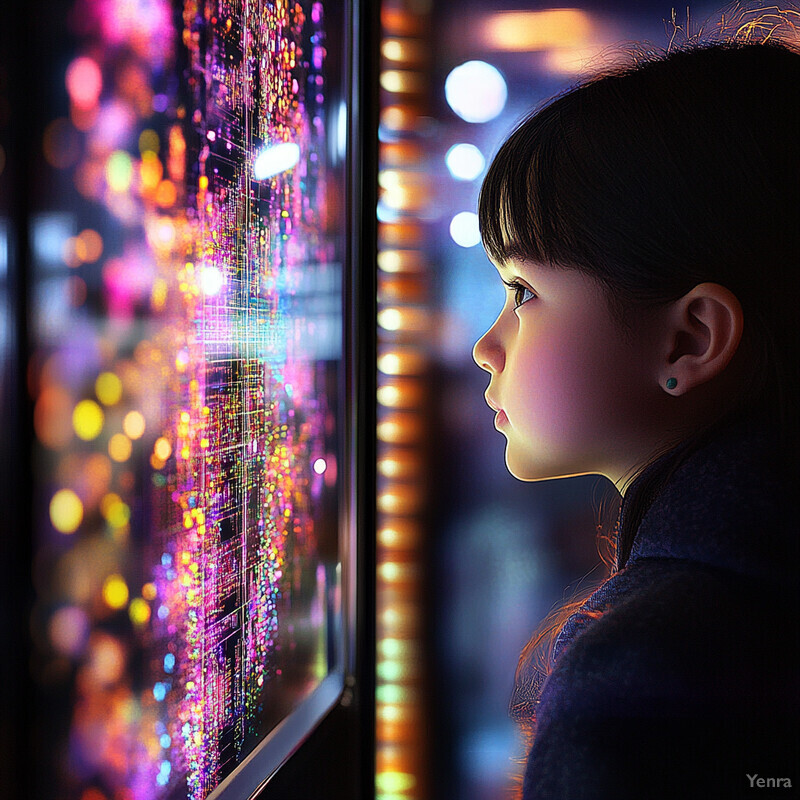
In microbial soil health analysis, one of the fundamental challenges is making sense of the immense complexity of microbial communities. AI algorithms, particularly those leveraging deep learning, excel at recognizing intricate patterns within massive datasets. By applying techniques like convolutional neural networks (CNNs) or graph-based models, researchers can discern subtle relationships among microbial taxa, identify keystone species, and detect rare but critical microorganisms. Moreover, advanced pattern recognition helps uncover how microbial interactions shift under varying soil conditions, crop management practices, and climate stressors. This leads to more informed hypotheses, guiding targeted experiments that refine our understanding of soil microbiome function.
2. Predictive Modeling of Soil Health
Machine learning models can forecast how different environmental factors (climate conditions, irrigation regimes, crop rotations) will influence microbial community structure and overall soil health, guiding proactive management decisions.
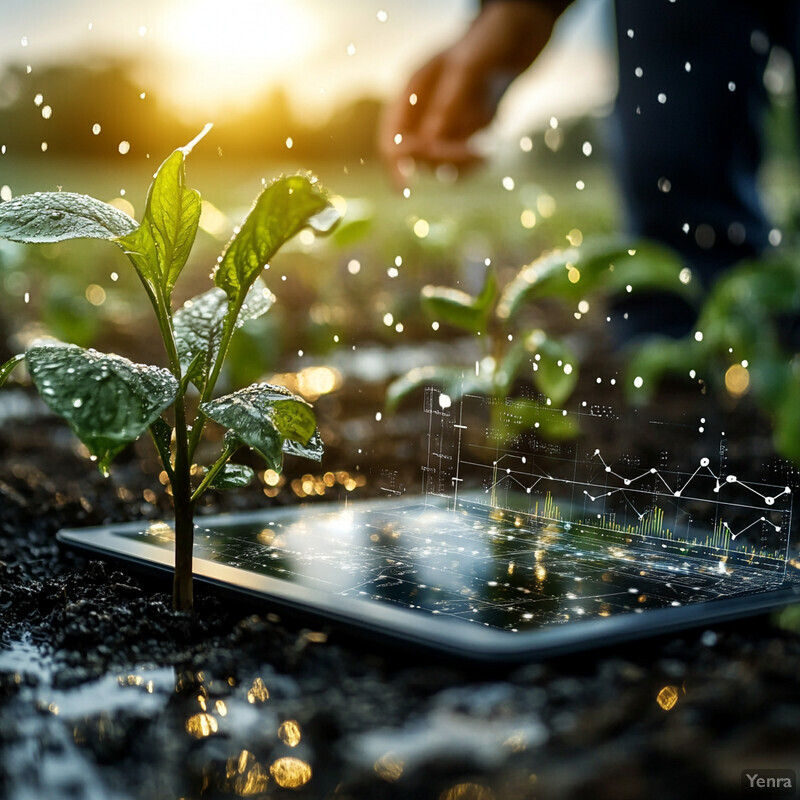
Predictive modeling with AI enables scientists and agronomists to forecast how soil microbial communities will respond to future conditions. With historical climate data, crop management records, and measurements of soil properties, machine learning models can predict shifts in microbial diversity and function. These forecasts guide proactive interventions such as altering irrigation schedules, introducing cover crops, or adjusting fertilizer applications before detrimental changes occur. Over time, predictive models refine their accuracy as they incorporate feedback from actual outcomes. This allows land managers to optimize soil health, enhance crop productivity, and maintain resilience in the face of environmental fluctuations.
3. Automated Classification and Clustering of Microbial Taxa
AI can quickly classify thousands of microbial species based on genetic markers, enabling researchers to characterize soil microbiomes at unprecedented scales and resolutions.
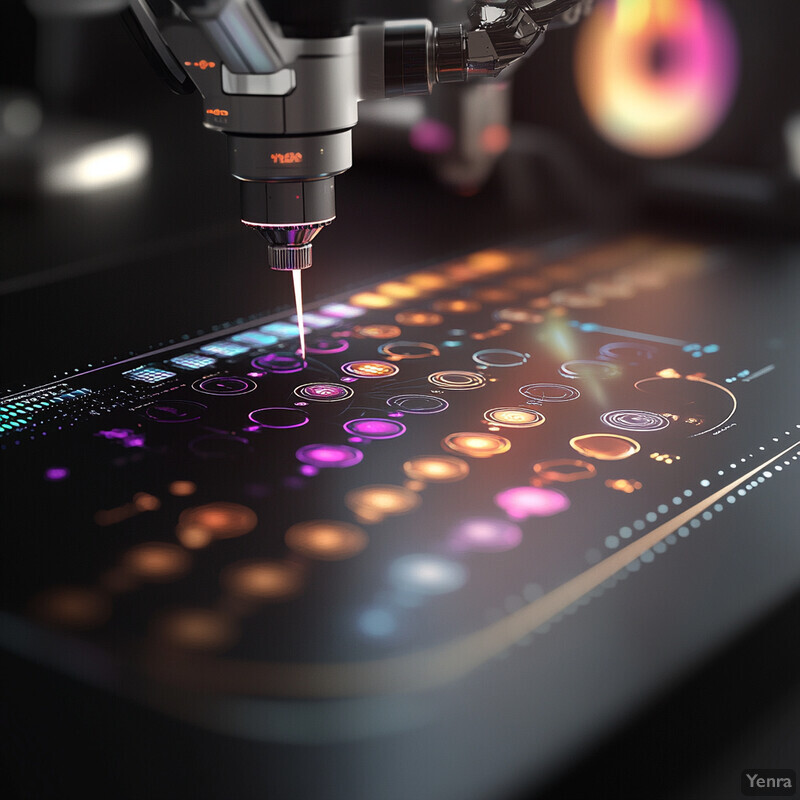
AI-powered classification tools streamline the process of identifying and grouping microbial species in soil samples. Traditionally, this required extensive manual classification or limited reliance on reference databases. Modern algorithms can handle thousands of operational taxonomic units (OTUs) or amplicon sequence variants (ASVs) simultaneously. They leverage genomic signatures, functional gene markers, and metadata to cluster organisms into meaningful taxonomic groups and ecological guilds. Automated classification saves time, reduces human error, and ensures consistency across studies. By accelerating taxonomy assignments, researchers can more quickly pinpoint the presence of beneficial microbes, detect pathogens, and characterize the structure of soil communities.
4. Functional Gene Annotation
By leveraging AI-based annotation tools, scientists can accurately predict the functions of newly discovered microbial genes, revealing how certain microbes contribute to nutrient cycling, disease suppression, or soil structure maintenance.
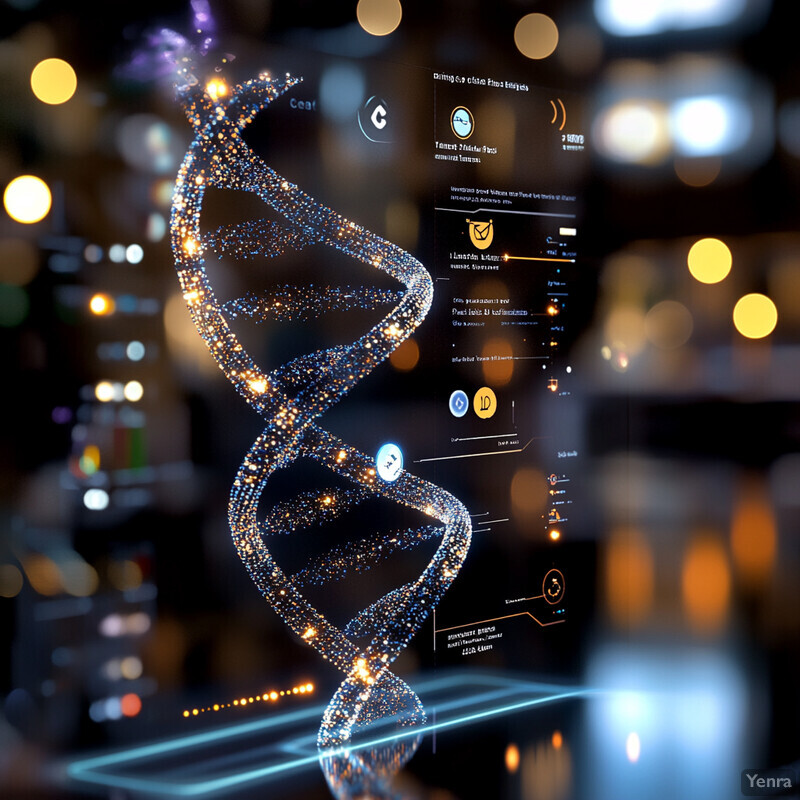
Functional gene annotation translates genetic sequences into biological meaning. Many soil microbial genes remain uncharacterized, representing a treasure trove of potential functions. AI-driven annotation tools employ natural language processing (NLP), pattern recognition, and domain knowledge to predict gene functions based on sequence similarity, structural features, and co-occurrence patterns. Such predictions help illuminate how microbes contribute to nutrient cycling, carbon sequestration, pathogen suppression, or soil aggregation. Functional annotations not only advance fundamental ecological understanding but also have applied benefits, such as guiding microbial strain selection for biofertilizers, improving soil structure, or mitigating greenhouse gas emissions from agricultural fields.
5. Metagenomic and Multi-Omics Integration
Deep learning techniques can integrate metagenomic, metatranscriptomic, and metabolomic data, producing a holistic understanding of microbial community functions and how they impact soil fertility and plant health.
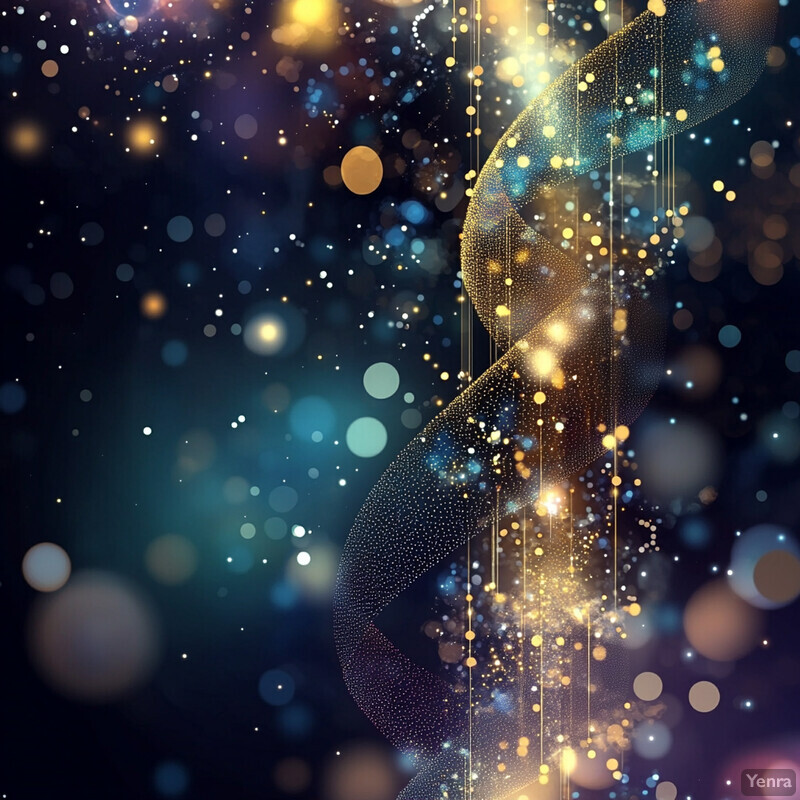
Soil microbial communities are increasingly studied using a combination of metagenomics, metatranscriptomics, metaproteomics, and metabolomics. Integrating these data streams is challenging due to the sheer volume and complexity. AI algorithms, including dimensionality reduction techniques and multimodal learning methods, bring these datasets together into a coherent narrative. By correlating gene abundance with metabolite profiles and expression patterns, AI helps identify which microbes are actively contributing to nutrient turnover, disease resistance, or soil fertility under specific environmental conditions. This holistic understanding informs precision interventions and supports the development of integrated soil health management strategies that enhance productivity and sustainability.
6. Early Pathogen Detection
AI-enabled pattern recognition can detect subtle shifts in microbial composition indicative of emerging soil-borne diseases, enabling early intervention to prevent outbreaks that harm crops.
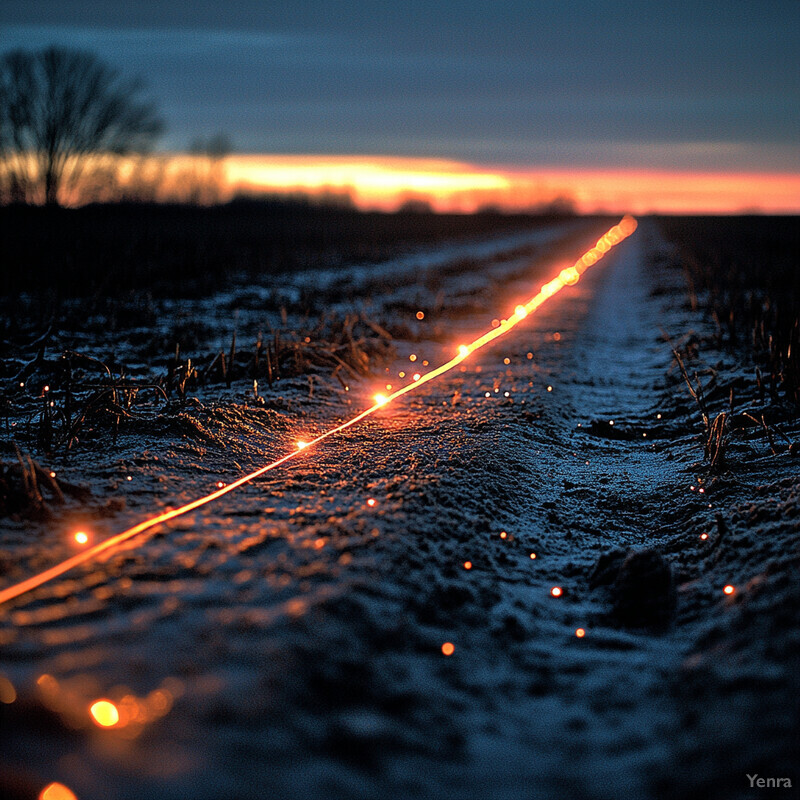
Detecting soil-borne pathogens before they decimate crops is paramount for sustainable agriculture. AI accelerates this process by analyzing shifts in microbial community composition and functional genes that signal disease emergence. Machine learning models trained on known pathogen outbreaks can predict when similar patterns arise in new samples, offering an early warning. Farmers and agronomists can then implement targeted treatments—such as introducing beneficial microbes or adjusting soil amendments—before pathogens spread. By reducing reliance on broad-spectrum pesticides, this proactive approach curtails environmental impact, cuts costs, and protects crop yields, ultimately contributing to healthier agroecosystems and more stable food supplies.
7. Identifying Bioindicators of Soil Health
AI systems can pinpoint key microbial taxa that serve as reliable indicators of soil vitality, allowing land managers to quickly assess the health status of their soils.
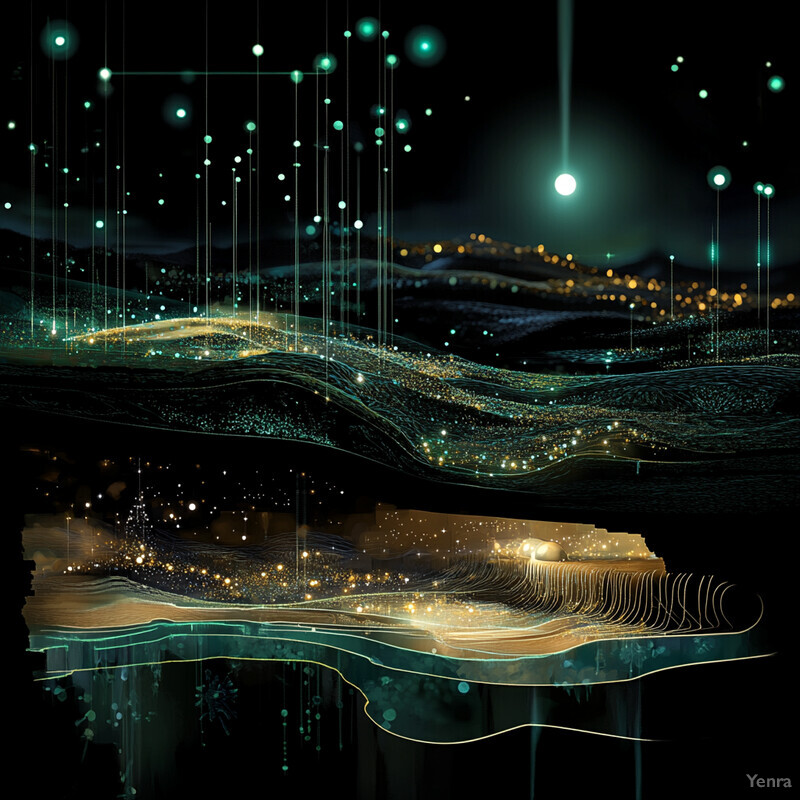
Soil health assessments often rely on a suite of indicators, including physical, chemical, and biological measures. Among biological indicators, certain microbes stand out as reliable proxies for soil vitality. AI helps identify these “bioindicators” by sifting through complex data to find taxa whose presence, abundance, or activity correlates strongly with improved soil function. Through advanced feature selection and ranking techniques, machine learning models highlight key organisms—such as nitrogen-fixing bacteria or phosphate-solubilizing fungi—that signify robust soil systems. Once identified, these bioindicators allow simpler, cost-effective monitoring, guiding agronomic decisions that maintain or restore soil health without extensive, time-consuming analyses.
8. Spatial and Temporal Soil Microbiome Analysis
Combining AI with geographic information systems (GIS) and temporal modeling can map how microbial communities evolve across different field locations and growing seasons, guiding site-specific soil management.
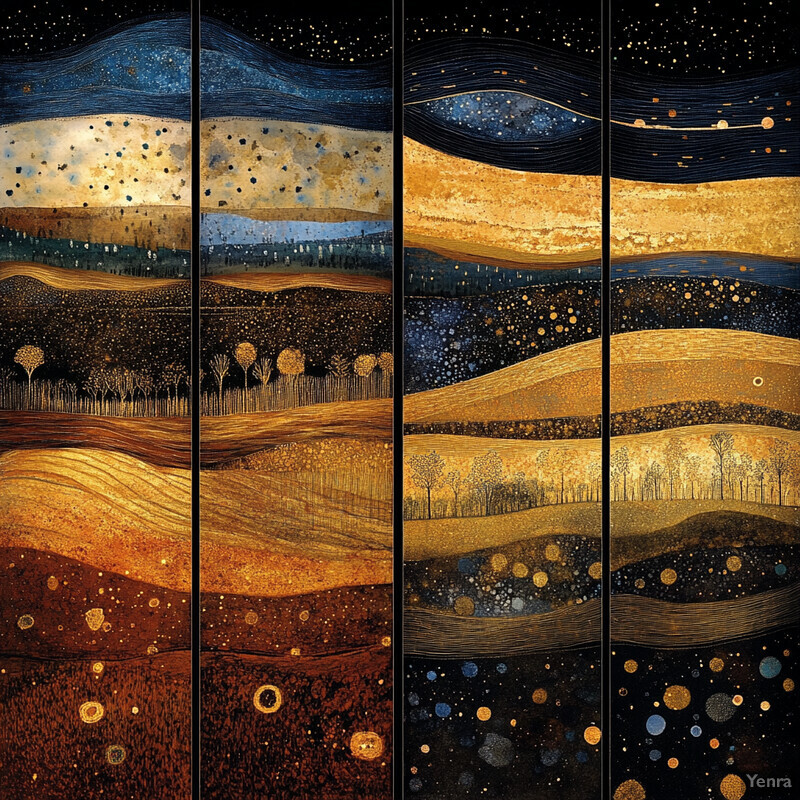
The soil microbiome is not static; it changes across spatial gradients and temporal scales. AI, combined with spatial analysis tools, can model how microbial communities shift from one part of a field to another, responding to differences in soil texture, moisture, pH, and management practices. Over time, these models capture seasonal patterns or the effects of crop rotation. Such insights help farmers practice site-specific soil management, applying amendments or planting cover crops where needed most. Temporal models also allow for long-term planning, ensuring that the soil microbiome remains stable and resilient amid climate change and evolving agricultural demands.
9. Optimization of Soil Nutrient Cycling
Machine learning can unravel correlations between certain microbes and nutrient availability, suggesting amendments or management practices that enhance nutrient efficiency and reduce chemical inputs.
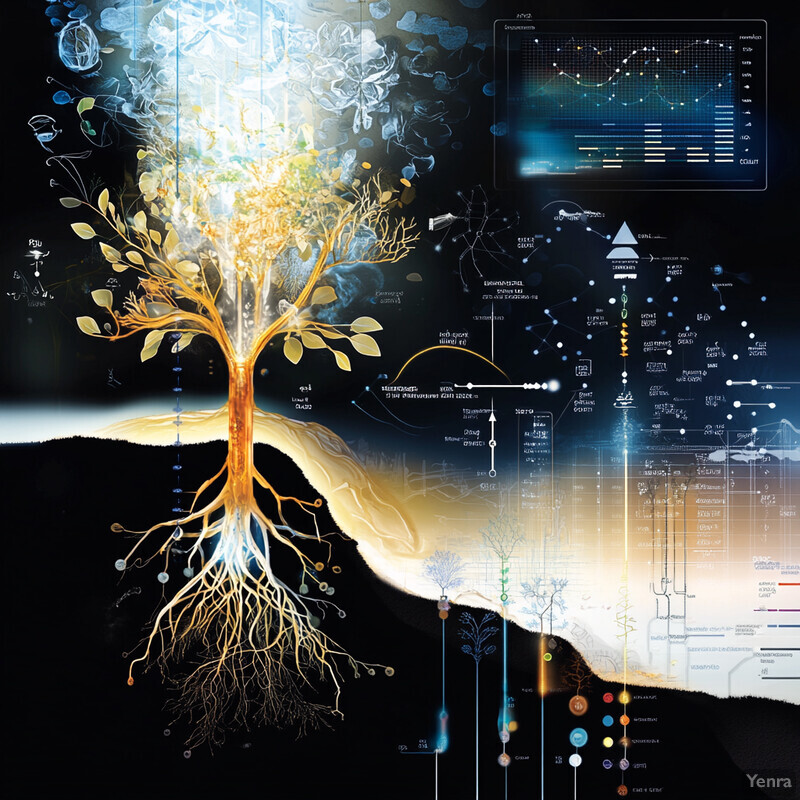
Soil microbial communities play a critical role in nutrient cycling, converting nutrients into forms accessible to plants. AI-driven approaches uncover hidden correlations between microbial populations, functional genes, and nutrient availability. Through advanced regression and predictive modeling, machine learning can suggest management practices that optimize nutrient release, minimize losses, and reduce the need for synthetic inputs. For example, if AI identifies key microbes that enhance nitrogen mineralization under certain conditions, farmers can adjust irrigation or incorporate legumes as green manures. By aligning microbial functions with plant needs, AI helps maintain soil fertility and promotes sustainable, resource-efficient agriculture.
10. Precision Agriculture Decision Support
AI-driven analytics can feed into precision farming tools, helping farmers select the right crop rotations, cover crops, or soil amendments based on microbial community feedback.
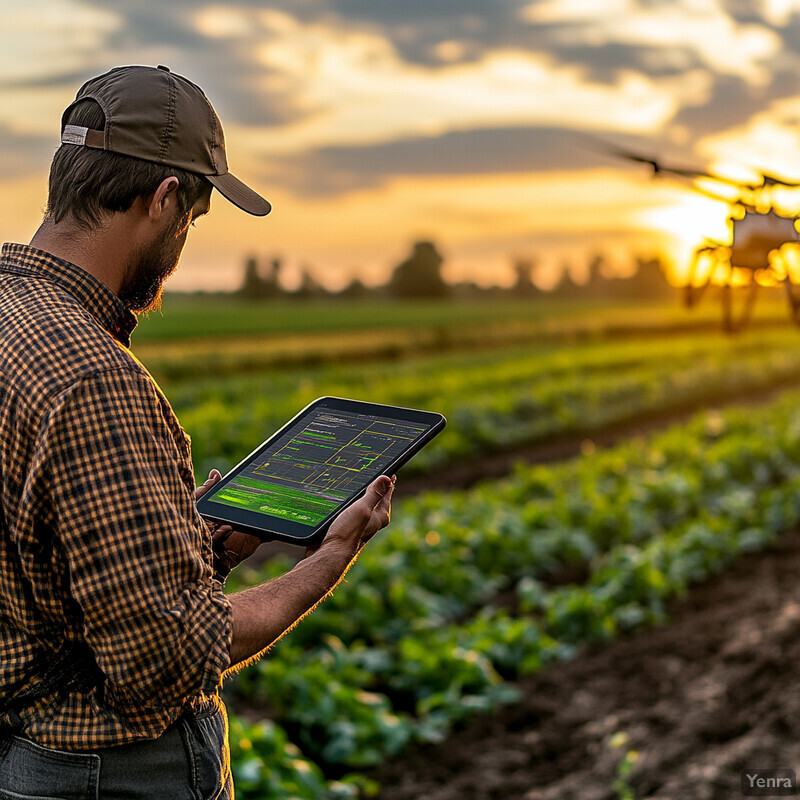
Integrating microbial soil health analysis with AI-driven decision support tools enables precision agriculture practices. By continuously analyzing soil samples, sensor inputs, weather forecasts, and historical yield data, AI systems provide recommendations that are fine-tuned to local conditions. These recommendations might include when to plant cover crops, how much organic matter to add, or which microbial inoculants to apply. This tailored guidance allows farmers to manage soils at a micro-level, improving productivity while preserving soil health. Precision agriculture supported by AI ultimately helps achieve higher yields, cost savings, and environmental stewardship, serving as a key pillar of sustainable farming.
11. Carbon Sequestration Modeling
Advanced AI models can predict how shifts in microbial populations affect soil carbon storage, aiding efforts to enhance carbon sequestration and combat climate change.
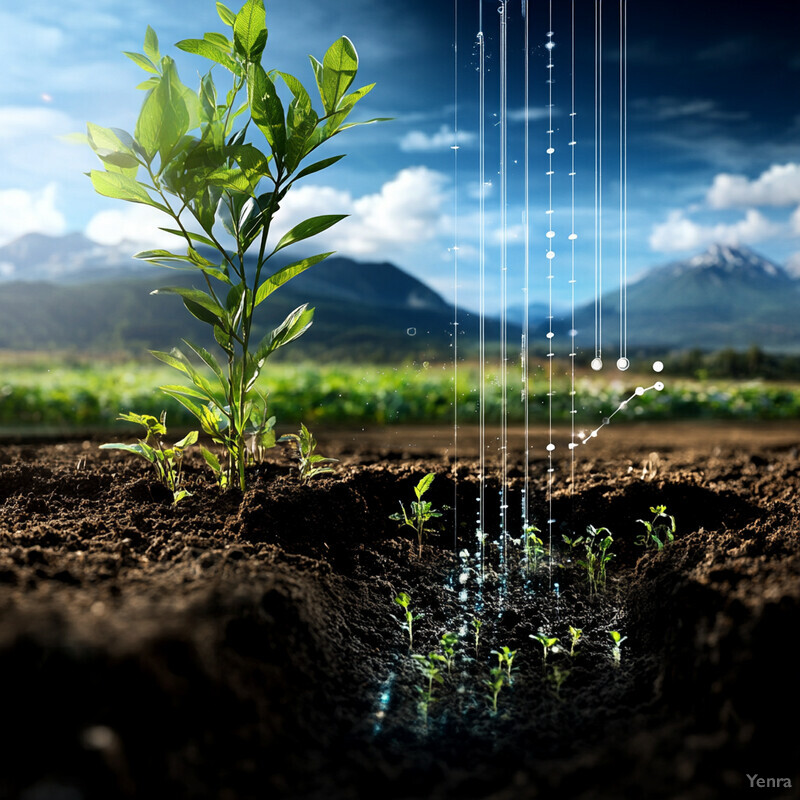
Climate change mitigation efforts increasingly focus on enhancing soil carbon sequestration. AI can model how microbial communities influence the formation and stabilization of soil organic carbon. By analyzing data on microbial community composition, functional genes, and environmental conditions, machine learning models can predict which management strategies—such as adding biochar or adjusting crop rotations—maximize carbon storage. Over time, as the models learn from real-world carbon sequestration outcomes, they become better at identifying practices that increase carbon pools while maintaining crop productivity. This informed approach supports both agricultural profitability and broader environmental objectives, contributing to global climate resilience.
12. Soil Microbe Discovery
AI-based clustering and anomaly detection can highlight previously unknown or understudied microbes, expanding the catalog of beneficial soil organisms that could improve soil fertility or resilience.
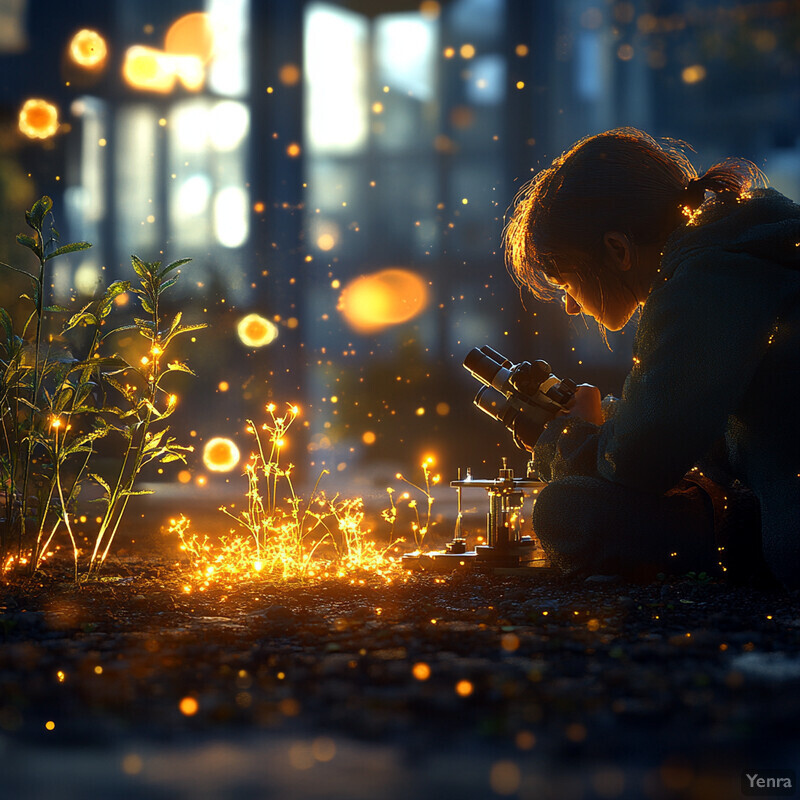
Despite years of research, soil harbors an astonishing diversity of unknown microbes. AI can assist in discovery by clustering sequences that differ from known taxa, flagging new operational taxonomic units that warrant further study. By focusing on genomic features, functional predictions, or ecological patterns, AI can recommend which unknown microbes might be beneficial for improving soil health. This accelerates the discovery pipeline, allowing scientists to isolate and experiment with candidate organisms that fix nitrogen more efficiently, break down pollutants, or enhance plant growth. In turn, these discoveries advance the frontier of soil microbiology and enable innovative applications in agriculture.
13. Microbial Network Analysis
Using AI, researchers can construct and analyze complex interaction networks among soil microbes, revealing cooperative or competitive relationships critical to ecosystem stability.
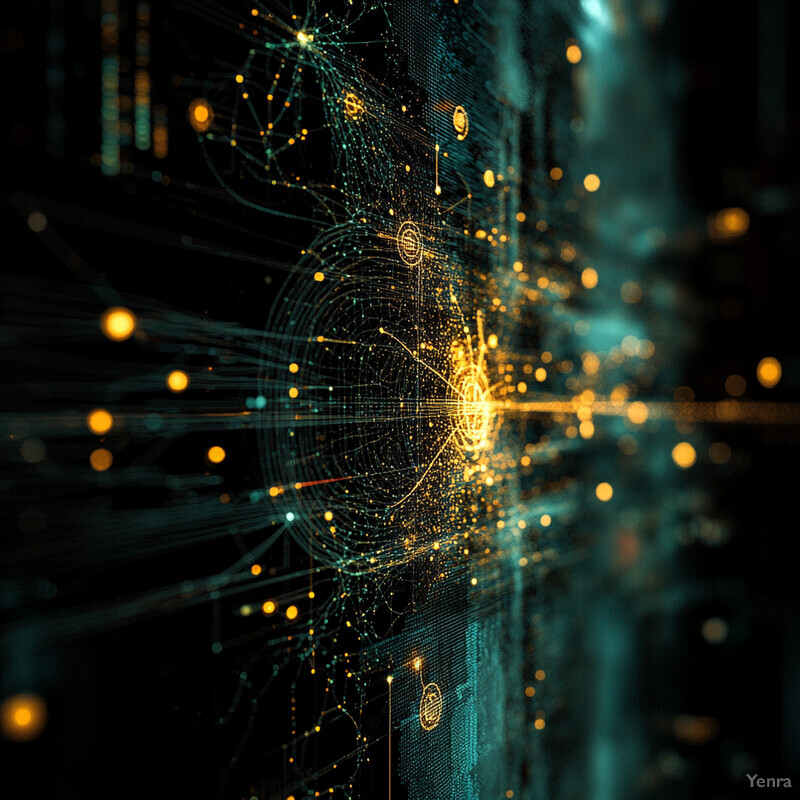
Microbes form intricate interaction networks, influencing each other’s abundance, activity, and overall community stability. AI-driven network analysis techniques—such as graph-based modeling, community detection algorithms, and centrality measures—help scientists visualize and quantify these relationships. Understanding which microbes are “hubs” or “bridges” in the network illuminates ecosystem functions and identifies key players in nutrient cycling or disease suppression. By modeling how networks change under stressors (drought, toxins, pathogens), researchers can design interventions that restore beneficial interactions. This perspective fosters resilient soil ecosystems, ensuring consistent agricultural output even as environmental conditions shift.
14. Stress and Contaminant Detection
AI can spot microbial signatures associated with heavy metals, pesticides, drought, salinity, and other stressors, enabling timely remediation strategies that restore healthy microbial balances.
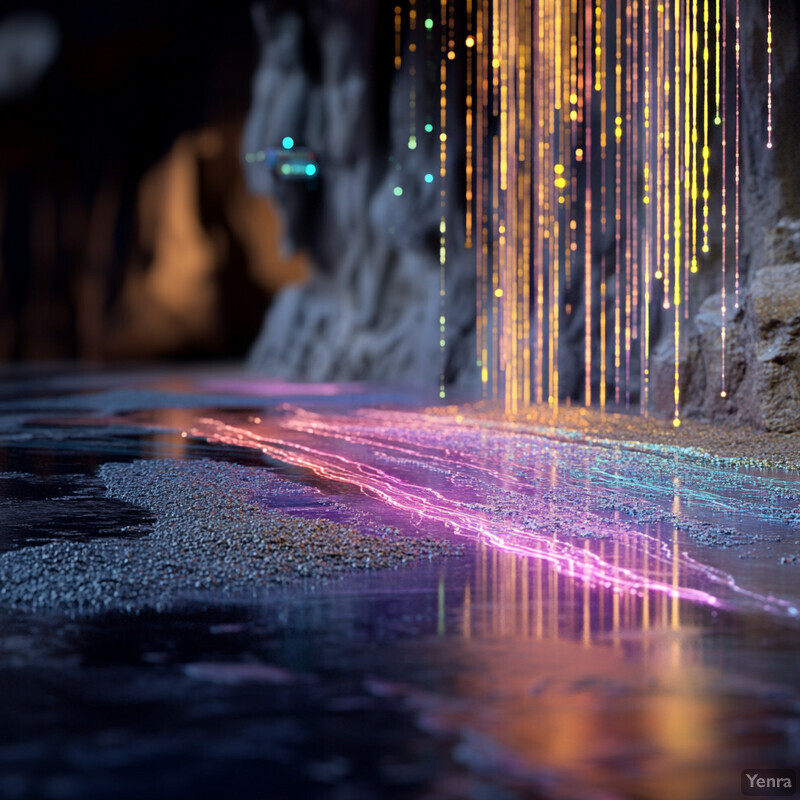
Environmental stressors and contaminants can drastically alter soil microbial communities, often with detrimental effects on plant health. AI can detect early signs of these shifts by recognizing patterns in microbial community composition that differ from healthy baselines. For example, certain microbial taxa may proliferate in response to heavy metals or pesticide residues. Machine learning models can flag these patterns rapidly, guiding targeted soil remediation efforts or changes in management to prevent further harm. This proactive approach preserves soil quality, safeguards crop yields, and reduces the ecological footprint of agricultural practices by minimizing unnecessary chemical interventions.
15. Predicting Responses to Agricultural Interventions
Machine learning models can forecast how a microbial community will respond to new management practices, fertilizers, bio-stimulants, or biological control agents, reducing trial-and-error in field management.
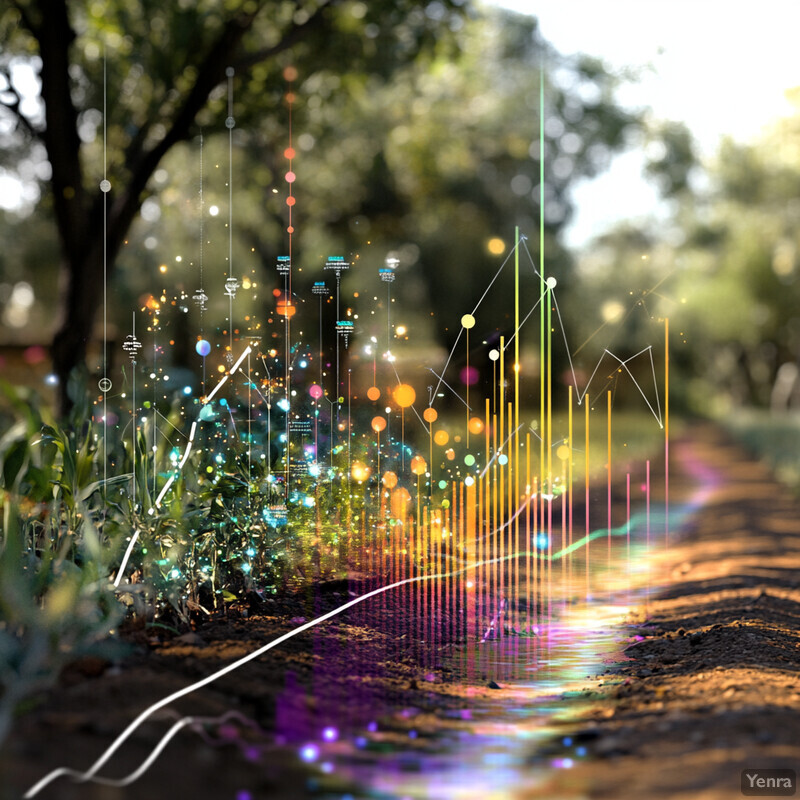
Before investing in new soil management strategies, farmers and researchers want to know how microbial communities will react. AI excels at simulating various scenarios—such as applying a novel bio-stimulant or introducing a specific cover crop—and predicting microbial responses. By combining historical data, environmental variables, and knowledge of microbial functions, predictive models can estimate outcomes like enhanced nutrient cycling or reduced pathogen load. This foresight reduces trial-and-error, saving time and resources. Ultimately, better predictions improve the likelihood of successful interventions, supporting sustainable soil management that’s both cost-effective and ecologically sound.
16. Soil Health Scoring Systems
AI can integrate multiple indicators—microbial diversity, functional gene abundance, nutrient profiles—into a single soil health score or index, simplifying decision-making for farmers and agronomists.
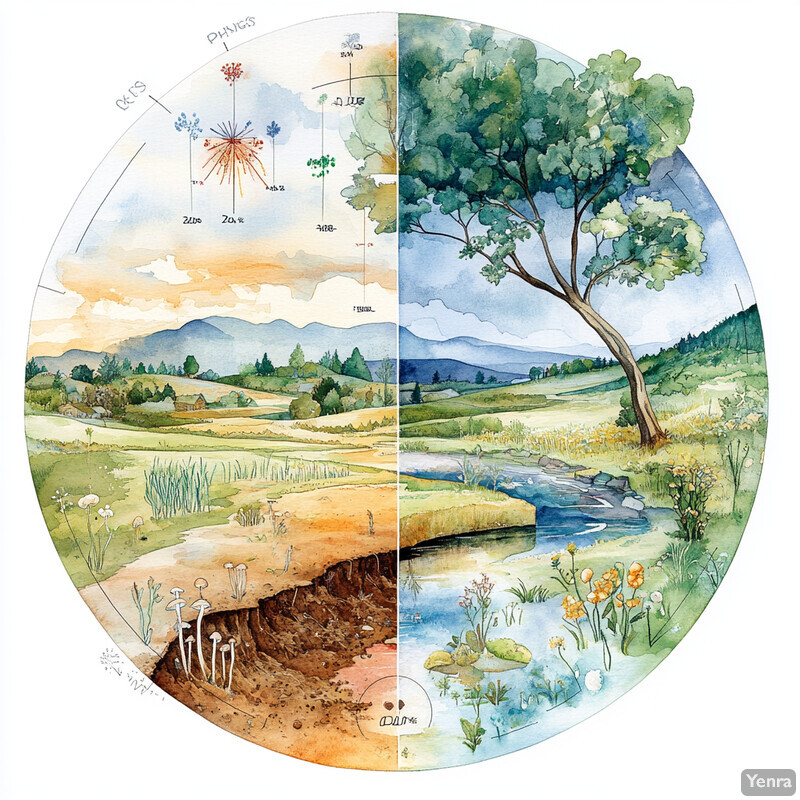
Soil health is multifaceted, encompassing physical structure, chemical balance, and biological diversity. AI can integrate these dimensions into a single, comprehensive scoring system that incorporates microbial community metrics. Through algorithms that weigh different variables—such as microbial richness, abundance of beneficial taxa, nutrient availability, and stable carbon content—AI generates an aggregate soil health index. This simplification helps farmers, agronomists, and policymakers quickly assess the status of a field and track improvements over time. With standardized scoring systems, it becomes easier to set benchmarks, evaluate management strategies, and direct resources toward methods that reliably uplift soil health.
17. Real-time Microbial Monitoring with Sensors
Coupled with sensor technologies, AI can interpret continuous data streams related to moisture, temperature, and nutrient levels, correlating these with microbial activity to refine on-the-fly management decisions.
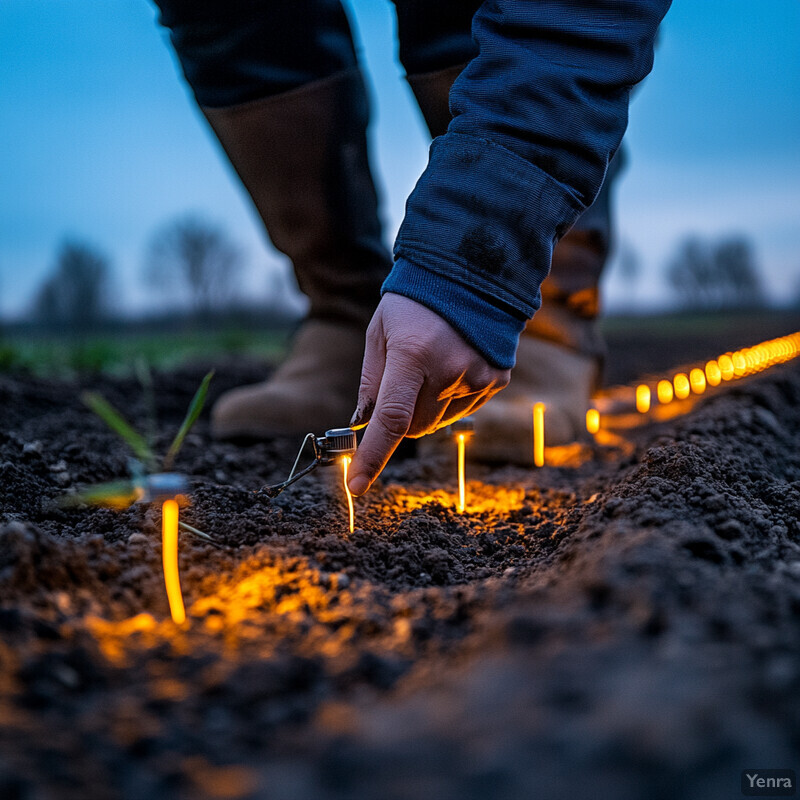
As soil sensors become more sensitive and portable, integrating them with AI allows for real-time analysis of microbial activity and related soil parameters. Sensor arrays measuring moisture, temperature, nutrient ions, and even microbial respiration can feed into machine learning models that correlate sensor readings with microbial processes. Farmers can receive immediate feedback, making on-the-fly adjustments to irrigation, fertilization, or tillage. This dynamic management optimizes conditions for beneficial microbes, supports plant growth, and prevents nutrient leaching or soil degradation. In essence, real-time AI-driven monitoring turns soil management into a responsive, data-rich practice.
18. Microbial Trait Prediction
AI can predict microbial traits (e.g., enzyme production, nitrogen fixation capacity) directly from genetic data, bypassing lengthy laboratory experiments and expediting the identification of beneficial strains.
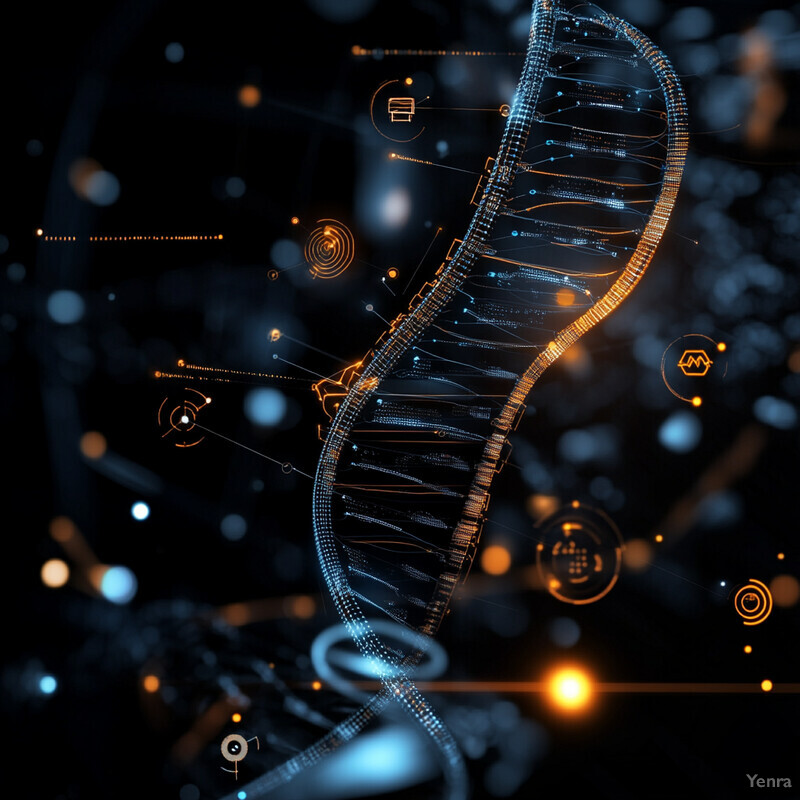
Characterizing microbial traits traditionally requires labor-intensive culturing and biochemical assays. AI can streamline this by predicting traits directly from genetic data. By training machine learning models on existing genomic and phenotypic datasets, AI can infer a microbe’s potential functions, such as nitrogen fixation, phosphate solubilization, or pathogen antagonism. These predictions accelerate the identification of beneficial strains for inoculants or biocontrol agents. As more microbial genomes are sequenced, trait prediction models become increasingly accurate, empowering researchers and farmers to harness microbial functions strategically, enhancing soil health and crop productivity without resorting to excessive chemical inputs.
19. Rapid Soil Diagnostics at Scale
By automating sample analysis and interpretation, AI can drastically speed up the soil testing process, making large-scale soil health assessments faster, cheaper, and more consistent.
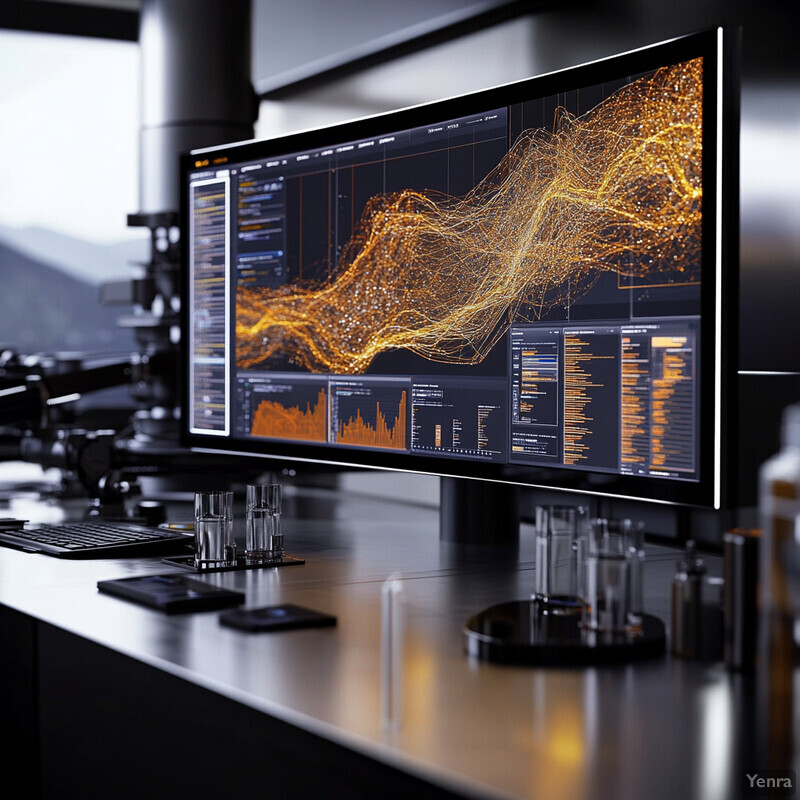
Scaling soil health diagnostics to regional or national levels is a major undertaking. AI simplifies this by automating data processing, classification, and interpretation. Instead of waiting weeks for lab results and manual analysis, large datasets from thousands of samples can be rapidly processed by machine learning models. This acceleration not only cuts costs and time but also ensures consistent quality and comparability across vast geographic areas. Agricultural extension services, government agencies, and environmental organizations can use these insights to guide policy, allocate resources, and support farmers in maintaining robust soil health on a broad scale.
20. Fostering Sustainable Soil Management
Ultimately, by harnessing AI to understand the intricate dynamics of soil microbial communities, stakeholders can shift towards sustainable soil stewardship, optimizing inputs, improving yields, and maintaining long-term soil resilience.
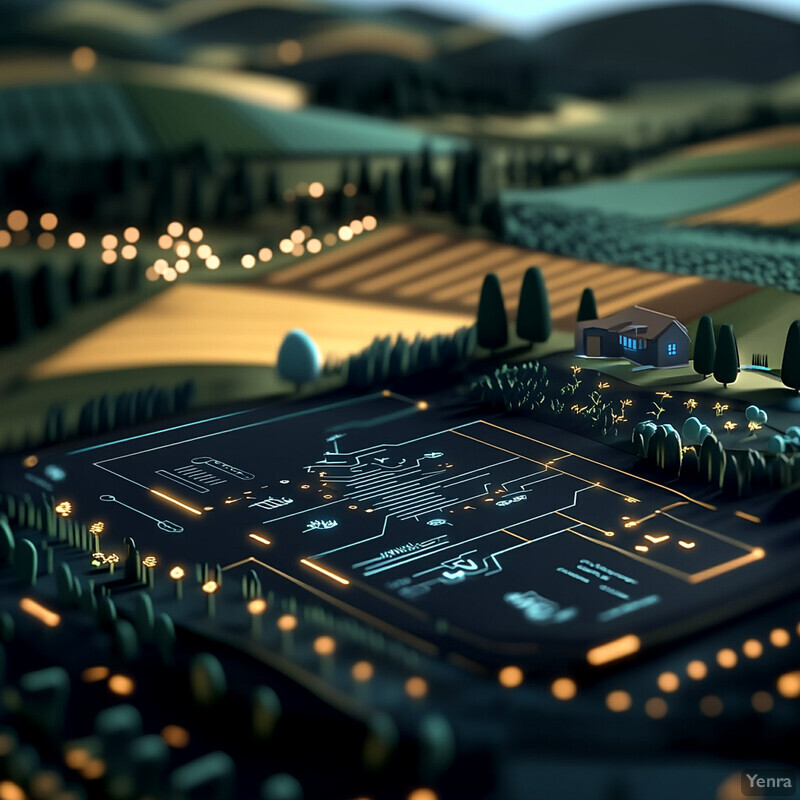
At its core, AI’s contributions to microbial soil health analysis promote long-term sustainability. By revealing complex relationships, guiding proactive interventions, and enabling precision management, AI helps maintain fertile, resilient soils that support high yields without harming the environment. Integrating microbial insights into agricultural decision-making reduces dependence on synthetic inputs, lowers greenhouse gas emissions, and conserves biodiversity. The cumulative effect of these advances is a more stable global food supply, better aligned with environmental imperatives. As AI tools become more accessible, even smallholder farmers can adopt best practices, ensuring that sustainable soil management principles scale equitably worldwide.