1. Accelerated Materials Discovery
AI-driven techniques, such as machine learning-guided high-throughput screening, help researchers rapidly identify promising new materials from vast chemical spaces, cutting down years of trial-and-error experimentation.
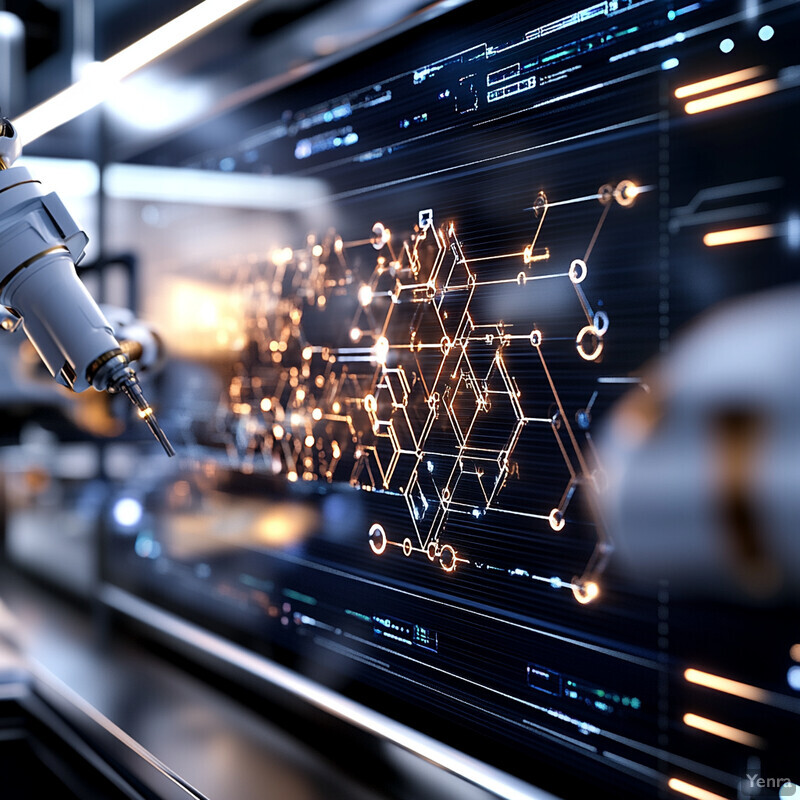
Traditional materials discovery often involves a painstaking, trial-and-error approach that can take years, if not decades, to identify new compounds with desirable properties. By integrating AI-driven high-throughput computational screening with advanced optimization algorithms, researchers can rapidly explore vast chemical and structural spaces. This computational pre-selection significantly narrows down the number of candidates that need to be experimentally synthesized. As a result, new materials with specialized characteristics—such as high-temperature superconductors, ultra-hard ceramics, or catalysts for clean energy production—can be identified and validated in a fraction of the time previously required.
2. Predictive Property Modeling
Advanced AI models can accurately predict key material properties—like mechanical strength, thermal conductivity, and electrical resistivity—before synthesis, reducing the need for costly and time-consuming physical experiments.
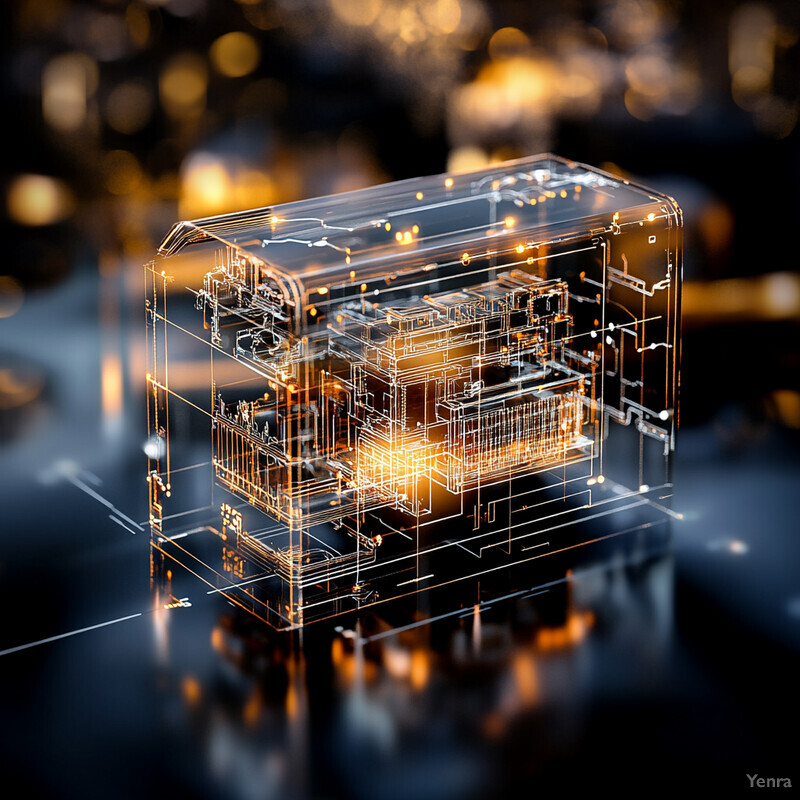
In the materials design process, predicting properties like tensile strength, bandgap energy, or thermal conductivity without actual synthesis saves enormous resources. AI models, particularly those leveraging machine learning and deep learning, can learn patterns from vast datasets of known materials and their experimentally validated properties. These models can then be applied to predict the characteristics of new or hypothetical materials. By enabling researchers to focus only on those candidates with the highest probability of exhibiting the target properties, predictive modeling accelerates R&D cycles and reduces costly experimental failures.
3. Inverse Design
Instead of starting from known compounds, AI can work backward from desired properties to propose candidate structures, streamlining the design of novel materials that meet specific performance criteria.
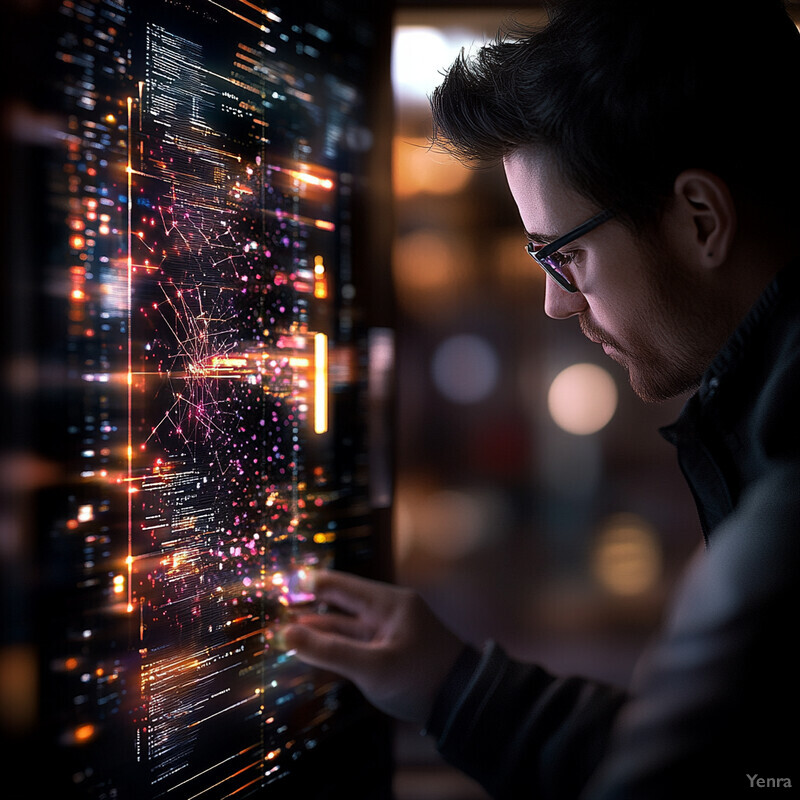
Inverse design leverages AI to start from the desired material functionality—such as a certain strength-to-weight ratio or a specific optical absorption spectrum—and work backward to suggest the atomic or molecular structure that would yield that functionality. Using generative models and optimization techniques, AI can propose compositions that may never have been considered, effectively broadening the design space beyond human intuition. This approach transforms the discovery process into a more goal-oriented endeavor, leading to the rational creation of groundbreaking materials for electronics, aerospace, healthcare, and more.
4. Multi-Scale Modeling
Machine learning algorithms enable the integration of data from atomistic simulations, molecular dynamics, and continuum-scale models, resulting in more comprehensive insights into material behavior across scales.
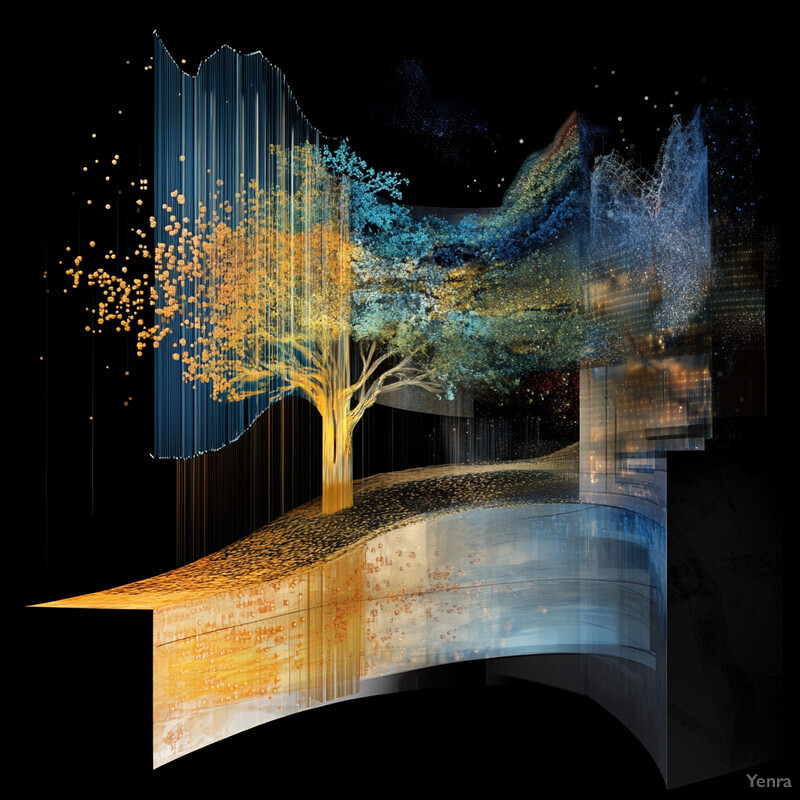
Materials behavior often emerges from phenomena occurring across multiple length and time scales—from quantum-level electron interactions to macroscopic mechanical responses. AI helps integrate these different scales by harmonizing datasets from electronic structure calculations, molecular dynamics simulations, continuum-level models, and real-world experimental data. Through machine learning, it is possible to capture complex correlations across scales, enabling researchers to build more accurate, holistic models. This multi-scale integration leads to better predictions of material performance under real application conditions.
5. Enhanced Materials Databases
AI tools can automatically extract and curate information from academic literature, patents, and databases, building more robust, searchable repositories that help scientists quickly locate materials data.
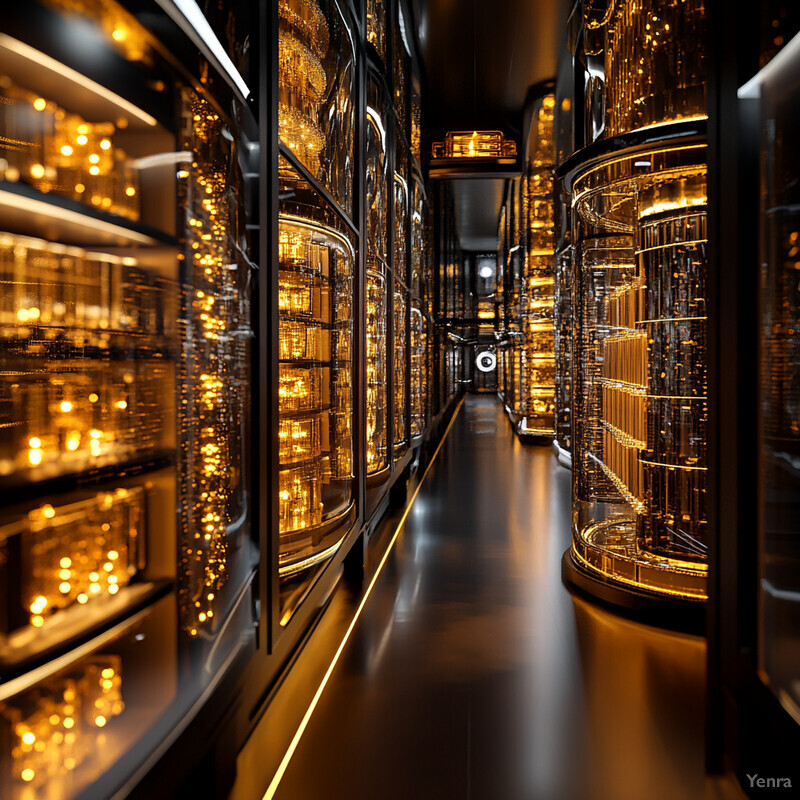
The exponential growth of scientific literature and patents makes manual data curation impractical. AI-driven text mining and natural language processing tools can comb through thousands of scholarly articles, extracting relevant data—such as material compositions, preparation methods, and performance metrics—and populating comprehensive databases. These expanded, enriched repositories make it easier for scientists to discover new insights, identify trends, and avoid redundant research. In turn, these improved databases serve as high-quality training sets for more accurate AI models, creating a virtuous cycle of knowledge enhancement.
6. Uncovering Hidden Relationships
By analyzing large datasets, AI can reveal complex, non-linear correlations between processing conditions, material structures, and final properties, offering new understanding that surpasses traditional modeling.
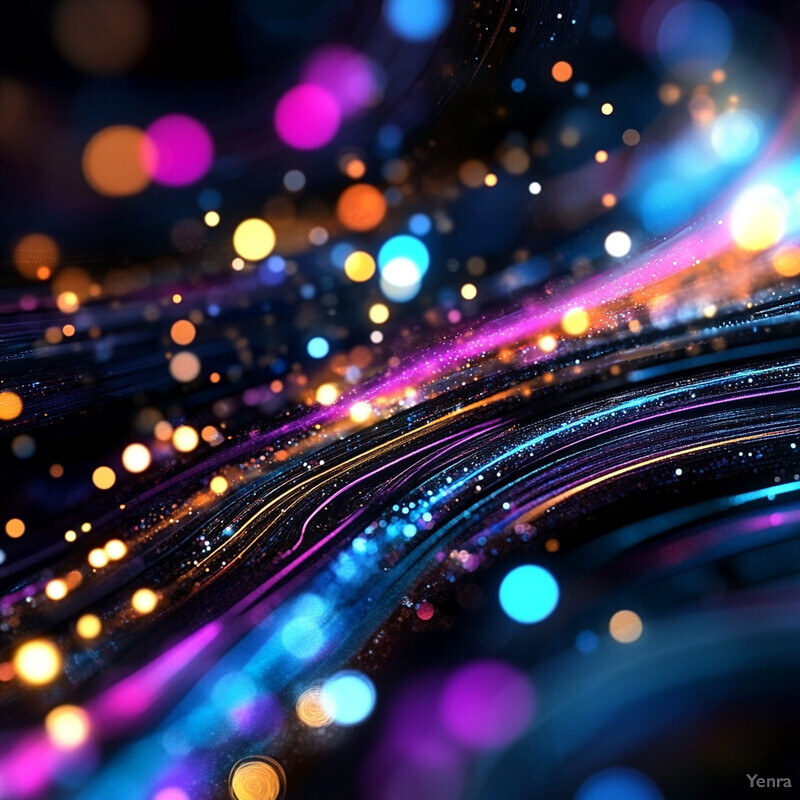
Materials scientists often struggle to identify subtle, non-linear relationships between structure, process conditions, and properties. AI excels at detecting patterns in high-dimensional data that are not easily discernible through conventional statistical methods. By applying machine learning algorithms, researchers can uncover complex correlations—such as how nanoscale grain boundaries influence macroscopic ductility or how minor compositional tweaks affect thermal stability. This deeper understanding enables more systematic design strategies, helping to refine materials for superior performance and reliability.
7. Guiding Experimental Synthesis
Robotics integrated with AI-driven models can guide synthesis conditions to produce desired materials more efficiently, essentially closing the loop between simulation and experiment.
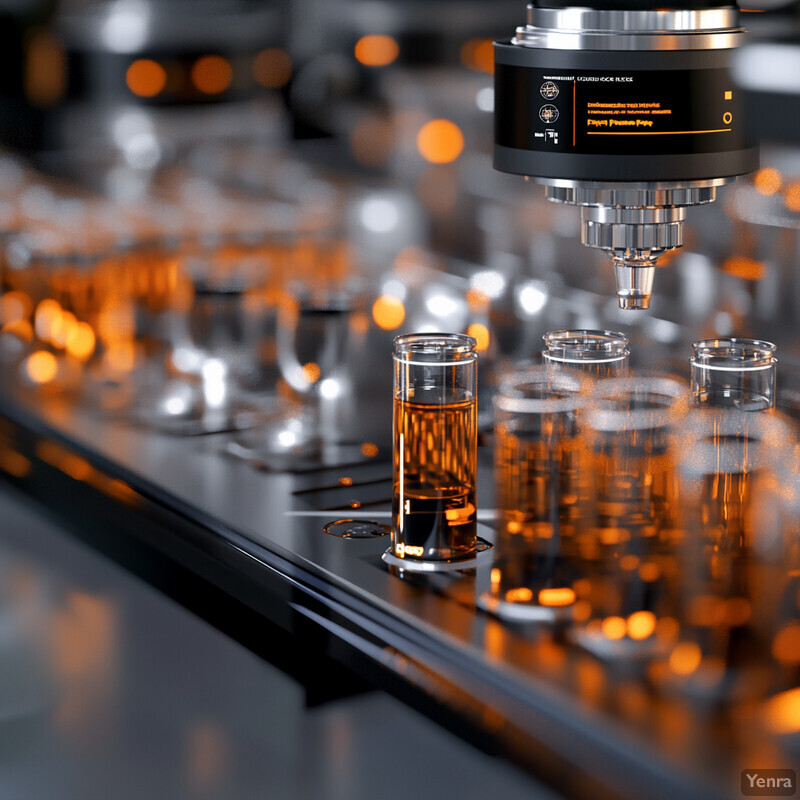
AI does not only aid in the virtual discovery of new materials but also assists in the practical experimentation phase. When integrated with robotics and automated lab equipment, machine learning models can suggest optimal synthesis routes, reaction conditions, and processing parameters. For example, algorithms can adjust temperature gradients, reagent concentrations, or reaction times to yield better material purity and uniformity. By closing the loop between prediction, synthesis, and characterization, AI-driven experimental platforms significantly shorten the path from concept to prototype.
8. Surface and Interface Optimization
AI can predict how structural or chemical modifications at surfaces and interfaces will alter a material’s functionality, essential for improving catalysts, sensors, and semiconductor devices.
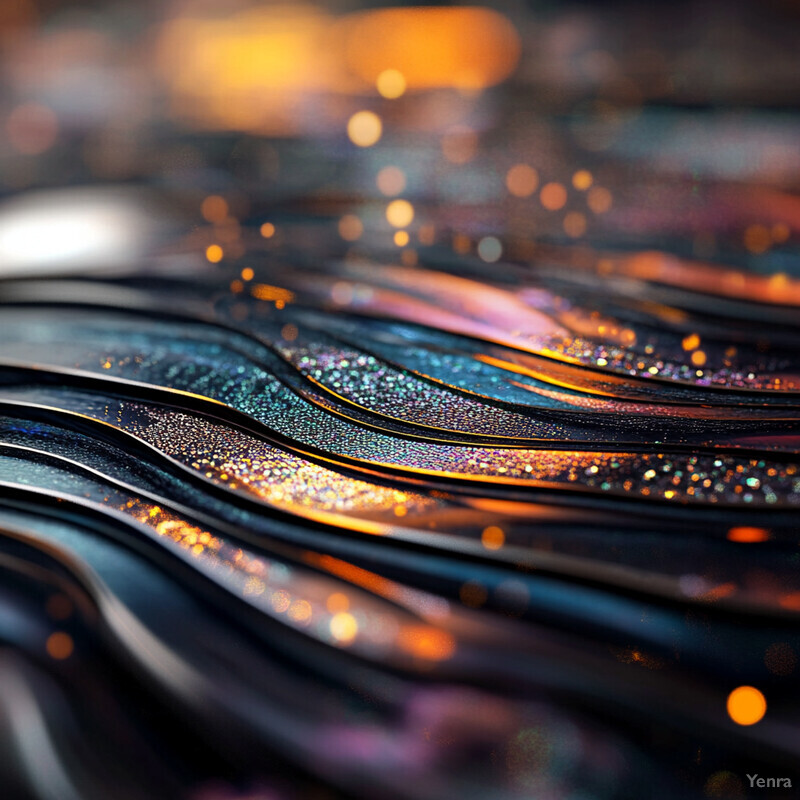
Many of a material’s functional properties—such as catalytic activity, adhesion, corrosion resistance, or electronic behavior—are governed by its surfaces and interfaces. AI techniques can model how changes in surface composition, roughness, or functionalization affect overall performance. Through advanced simulations and optimization algorithms, scientists can identify which dopants or coatings will enhance a material’s reactivity or stability. With these insights, they can design advanced catalysts, sensors, or semiconductor devices that operate more efficiently and last longer under working conditions.
9. Quality Control and Defect Detection
Machine vision and deep learning algorithms can rapidly identify defects or inconsistencies in synthesized materials, aiding in quality assurance and process refinement.
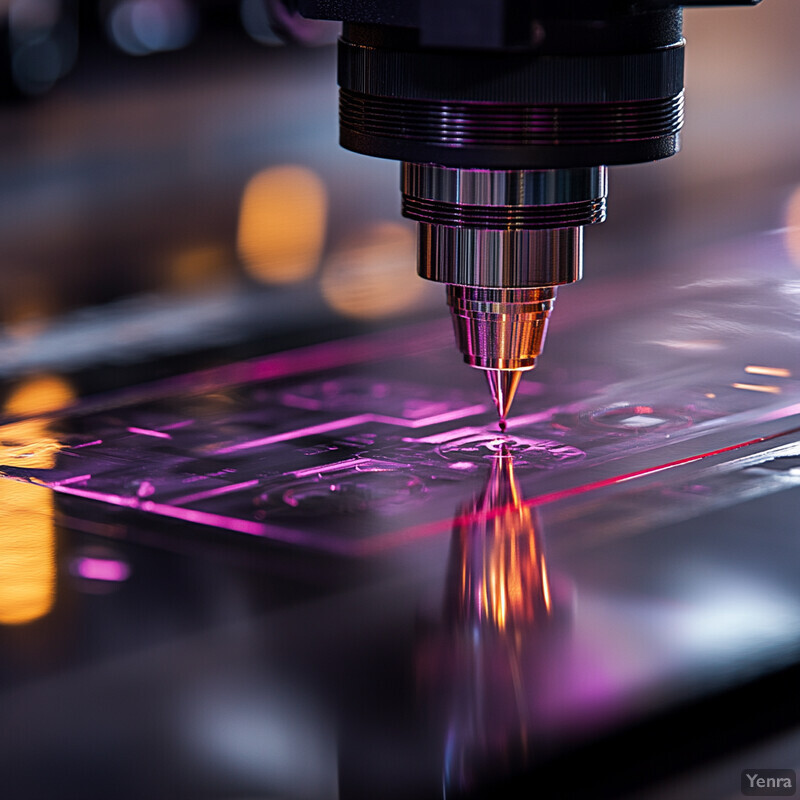
Even after a promising material is discovered, ensuring its consistent quality and performance can be challenging. AI-driven image recognition and computer vision tools can inspect materials—such as rolled steel, semiconductor wafers, or polymer films—in real-time, identifying microstructural defects or irregularities with exceptional accuracy. This allows manufacturers to intervene early in the production process, reducing scrap, downtime, and costly rework. The result is a more reliable supply chain and products that meet stringent industry standards and customer expectations.
10. Data-Driven Material Genomics
Just as genomics revolutionized biology, “materials genomics” uses AI-driven big data techniques to understand and classify materials in ways that accelerate innovation and application development.
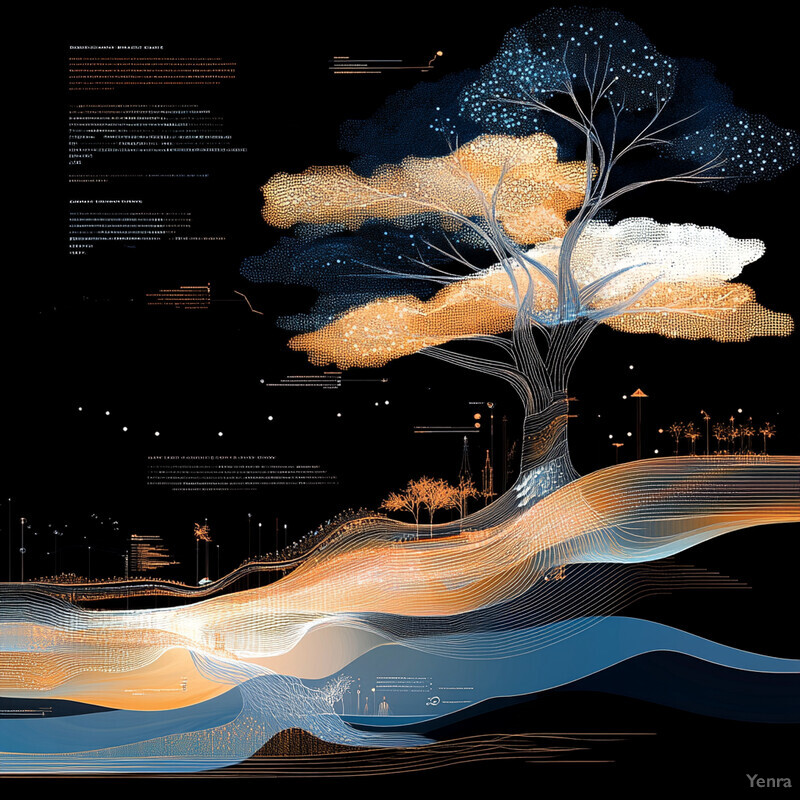
Borrowing concepts from genomics, materials genomics aims to catalog and classify materials based on their fundamental building blocks and properties. AI algorithms can group similar materials, identify evolutionary trends in compositions, and discover “families” of compounds that share beneficial traits. This data-driven approach allows researchers to quickly pinpoint promising candidates for specific applications. Similar to how genomic data revolutionized medicine, materials genomics is transforming how scientists understand, classify, and predict the behavior of matter at various scales.
11. Developing Sustainable Materials
AI models can pinpoint eco-friendly materials or suggest less energy-intensive processing routes, supporting green chemistry and sustainable engineering goals.
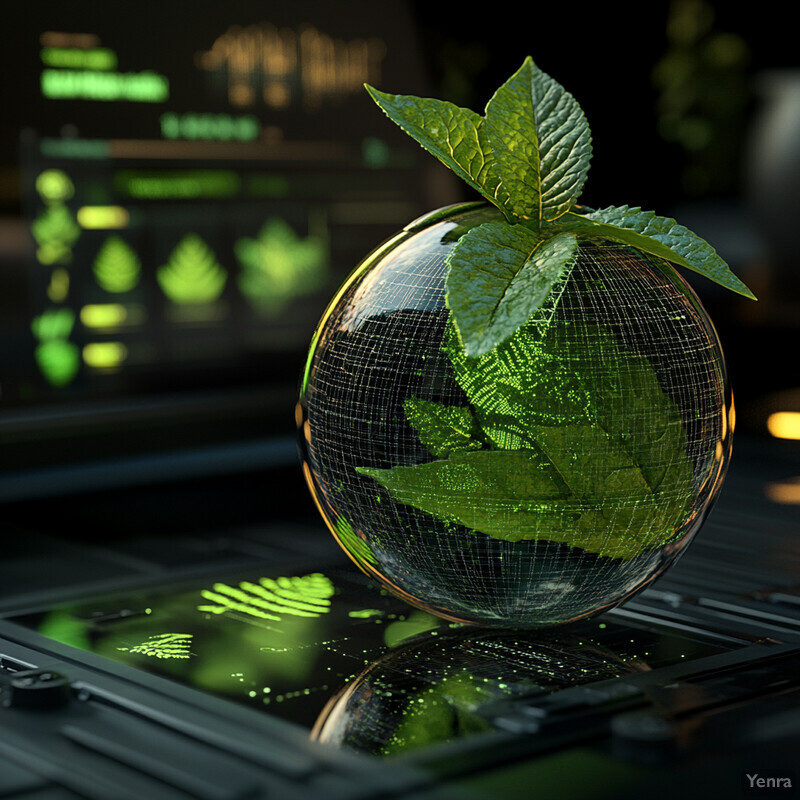
Sustainability is a growing priority in materials science. AI can analyze data on environmental impact, toxicity, energy consumption, and lifecycle costs to recommend greener alternatives. For example, machine learning models can suggest which bio-derived polymers might replace petroleum-based plastics or which recycling processes are most efficient. By identifying sustainable compounds and production methods, AI helps reduce the ecological footprint of industries ranging from construction and packaging to electronics and automotive.
12. Optimizing Additive Manufacturing Processes
For 3D printing and other additive manufacturing methods, AI can predict optimal print parameters—like laser power or print speed—leading to improved mechanical properties and surface finish.
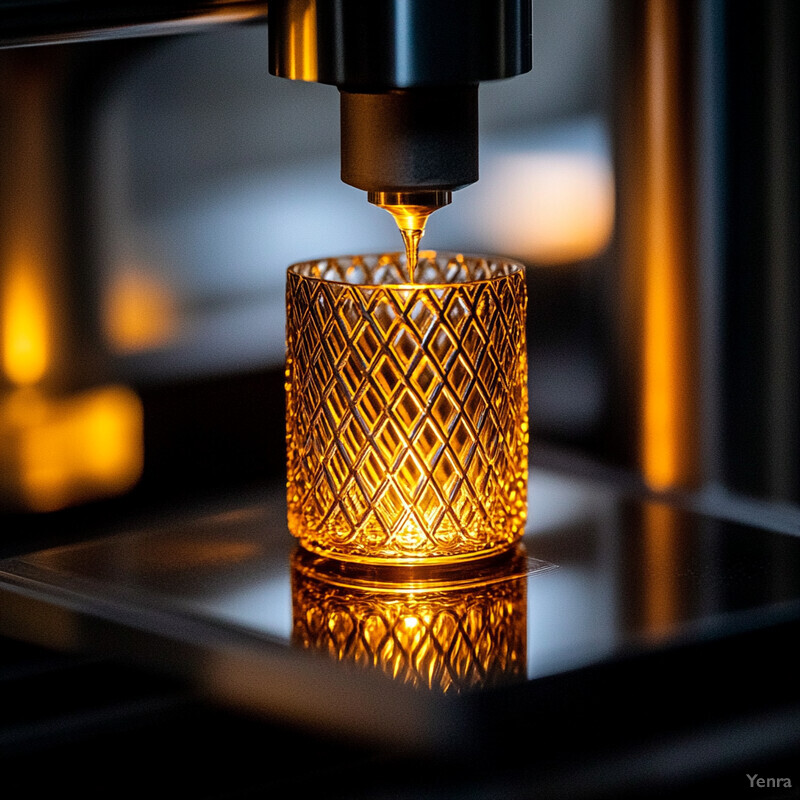
Additive manufacturing (AM), commonly known as 3D printing, requires precise control over numerous parameters to achieve the desired mechanical, thermal, and aesthetic properties. AI-driven models can predict how variations in print speed, laser power, layer thickness, or cooling rates affect the final material’s microstructure and quality. By guiding the selection of optimal printing conditions, AI helps engineers produce stronger, lighter, and more reliable components. This leads to cost savings, reduced material waste, and the ability to produce complex geometries unattainable by traditional methods.
13. Stability and Longevity Predictions
AI-based simulations can forecast how materials will degrade under real-world conditions, helping engineers preempt failures and design more durable products.
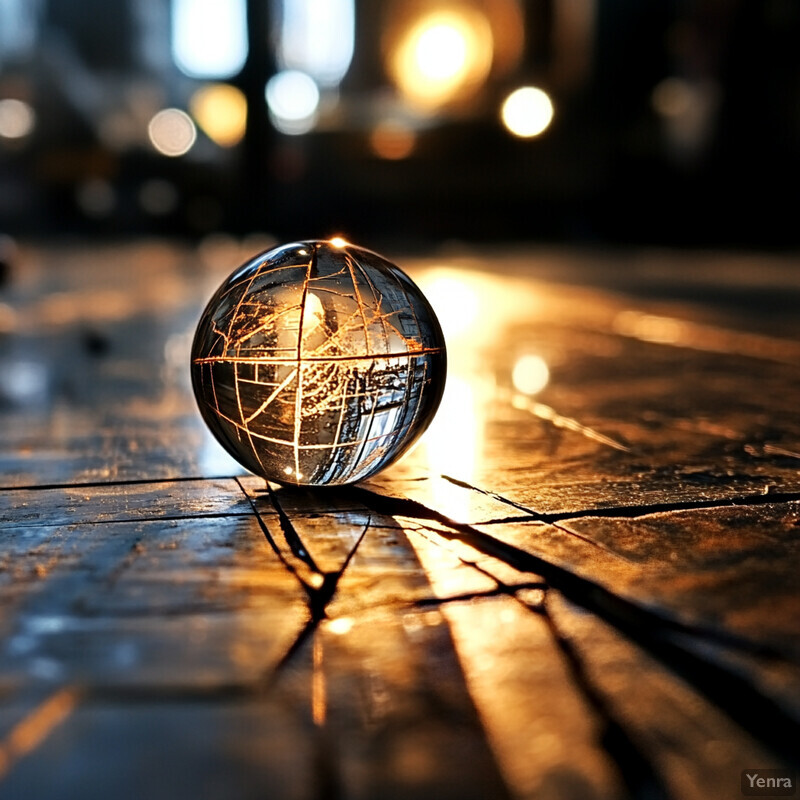
Many advanced materials must withstand harsh environments—such as extreme temperatures, corrosive chemicals, or high mechanical loads—over long periods. AI can forecast the long-term stability, degradation mechanisms, and failure probabilities of these materials by analyzing historical performance data and simulating accelerated aging conditions. By predicting how materials will behave over years or decades, researchers can design more durable products, reducing maintenance costs and improving overall safety and reliability.
14. Catalyst Design for Energy Applications
Machine learning models assist in identifying and fine-tuning catalysts that enhance reactions for fuel cells, electrolyzers, and solar-to-fuel conversions, increasing energy efficiency and lowering costs.
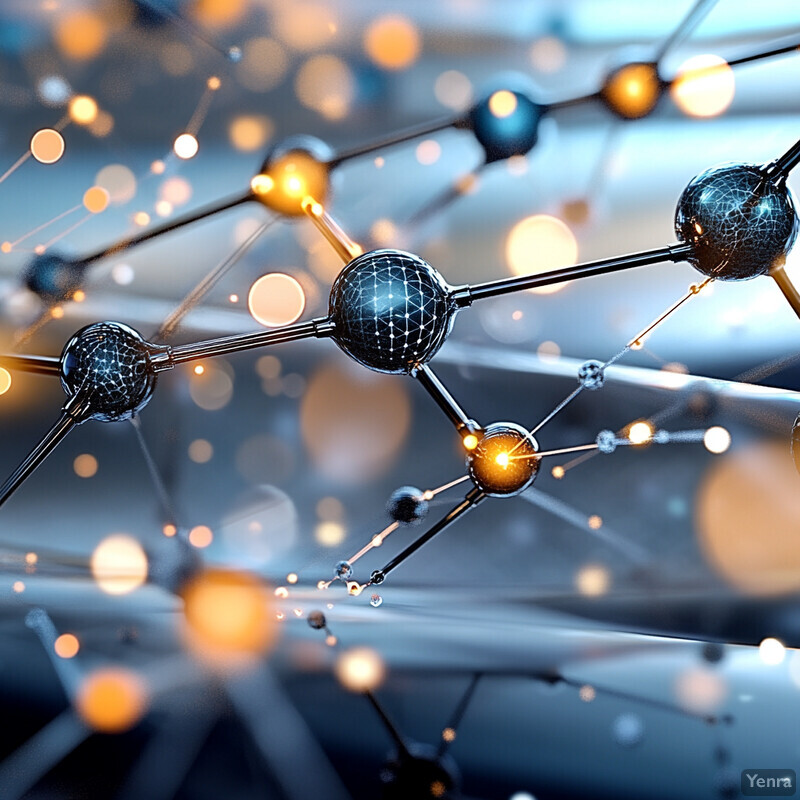
Catalysts play a pivotal role in chemical reactions, particularly in energy-related processes such as hydrogen production, CO₂ reduction, and fuel cell reactions. AI models can assess how changes in catalyst composition, particle size, or support materials influence reaction kinetics and selectivity. By systematically optimizing these factors, AI helps researchers discover catalysts that operate at lower temperatures, require fewer precious metals, or enable higher reaction yields. These improvements have a direct impact on scaling up sustainable energy technologies and improving energy efficiency.
15. Tailoring Electronic and Photonic Materials
AI can guide the discovery of semiconductors, superconductors, and photonic crystals with targeted bandgaps or optical properties, accelerating the development of next-generation electronics and photonics.
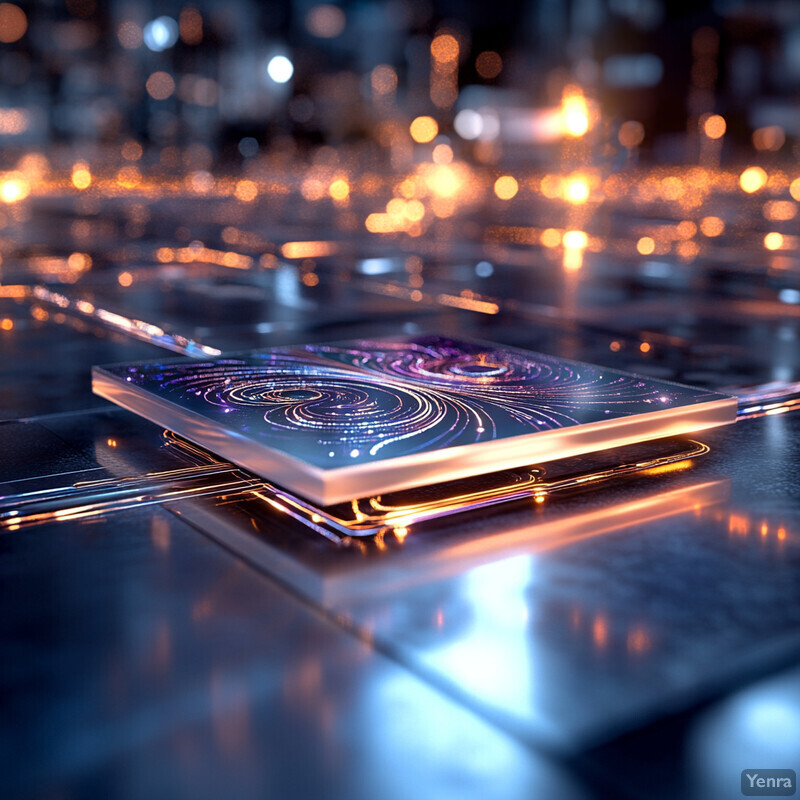
The performance of electronic and photonic devices depends heavily on the band structure, defect density, and optical properties of their constituent materials. AI algorithms can predict which atomic arrangements will yield a desirable bandgap or which crystalline structures will optimize light-matter interactions. As a result, researchers can more rapidly identify semiconductors, superconductors, or photonic crystals that enable faster computing, more sensitive detectors, or more efficient solar cells. This accelerates advancements in electronics, quantum computing, and photonics-based communication systems.
16. Lightweight Alloys for Transportation
Through AI-based predictions, researchers can develop advanced lightweight alloys with high strength-to-weight ratios, critical for improving fuel efficiency in cars and aircraft.
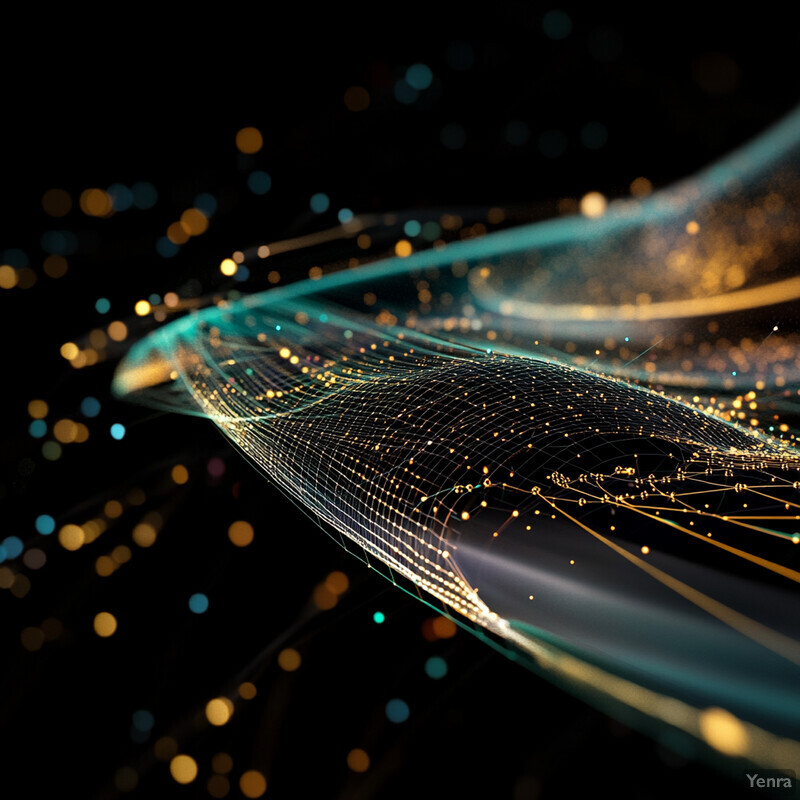
The transportation industry continuously seeks stronger, lighter materials to improve fuel efficiency and reduce emissions. By applying machine learning to massive datasets of alloy compositions, heat treatments, and measured properties, engineers can quickly identify promising lightweight alloys with high strength-to-weight ratios. AI models can also suggest minor compositional tweaks that yield significant performance gains. The outcome is the development of aerospace- and automotive-grade materials that outperform traditional steels or aluminum alloys, enhancing safety and sustainability in transit.
17. Combinatorial Exploration of Compositions
AI can handle combinatorial chemistry experiments in silico, evaluating thousands of possible alloy or ceramic compositions at once, and narrowing down candidates for lab testing.
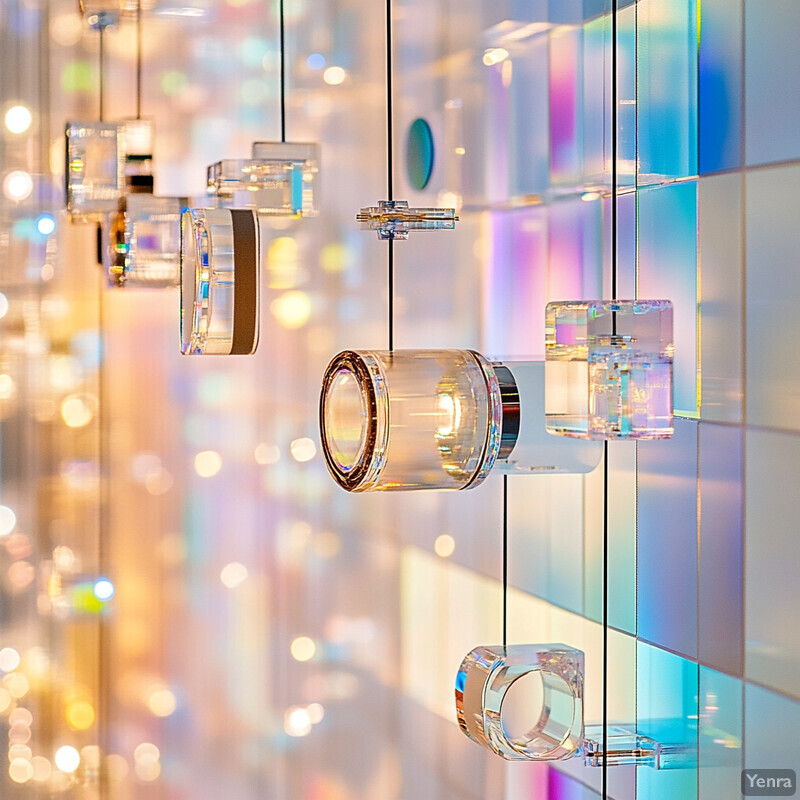
With countless possible combinations of elements to form alloys, ceramics, or polymer blends, it’s nearly impossible to manually test them all. AI excels at rapidly scanning through chemical spaces, using combinatorial algorithms to rank potential candidates based on simulated properties. This approach allows researchers to home in on a shortlist of promising compositions that merit experimental testing, vastly accelerating the identification of new high-performance materials. As a result, novel alloys, magnetic materials, and thermoelectric compounds can be discovered in a fraction of the usual time.
18. Real-Time Data Analysis in Experiments
Integrating machine learning into in-situ characterization tools (like electron or scanning probe microscopes) enables real-time data interpretation, allowing immediate adjustment of experimental conditions.
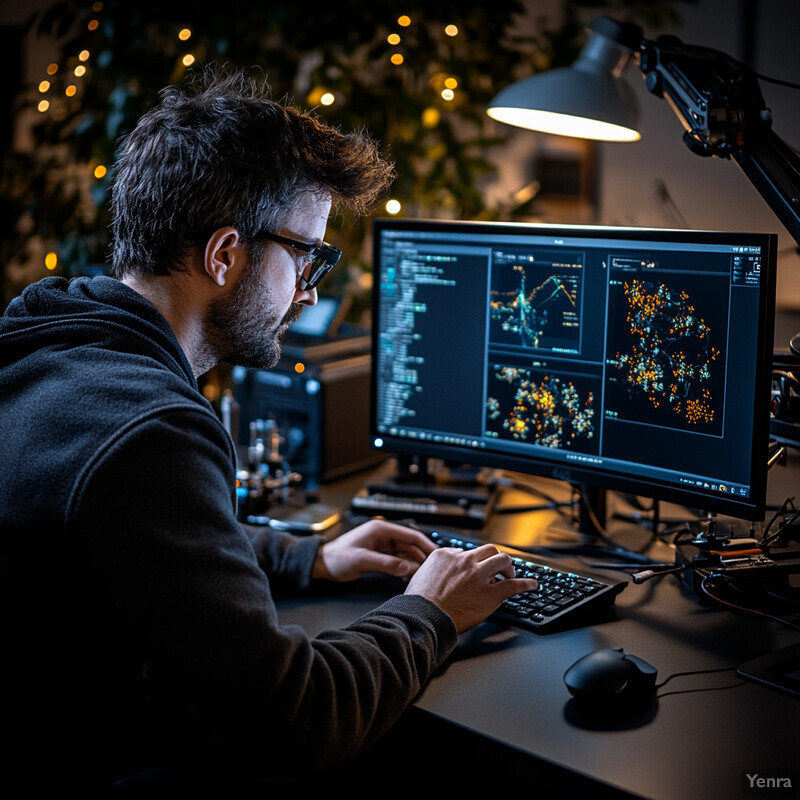
Advanced characterization techniques like electron microscopy or synchrotron X-ray diffraction generate large volumes of complex data at unprecedented speed. AI-driven analysis tools can process these streams of information in real-time, identifying phase transitions, crystallographic defects, or chemical gradients as they occur. This immediate feedback enables experimenters to adjust parameters on-the-fly—such as temperature, pressure, or doping levels—to achieve better results. Ultimately, this synergy between live experimentation and AI assistance leads to more dynamic, insightful, and efficient research workflows.
19. Improved Structural Adhesives and Polymers
AI-driven molecular simulations help design polymer networks and adhesive formulations with enhanced mechanical, thermal, and chemical resistance properties.
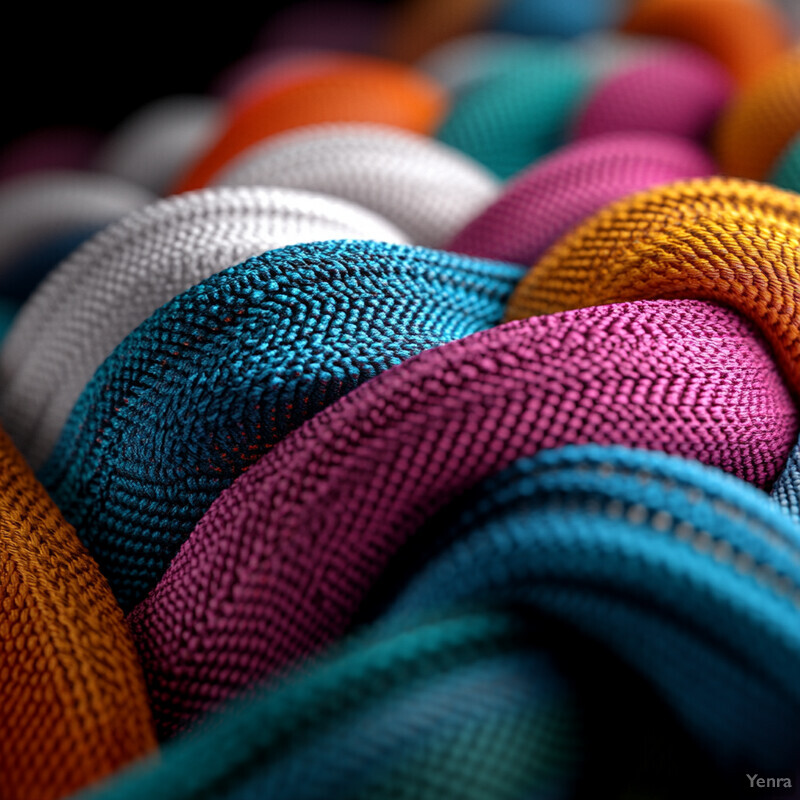
Polymers and adhesives are crucial in applications ranging from construction to electronics assembly. AI modeling can predict how polymer chains interact, how additives modify mechanical properties, and how curing conditions influence bonding strength. By systematically exploring these variables, researchers can design adhesives with enhanced thermal resistance, improved chemical stability, and superior mechanical performance. These materials support more reliable electronic devices, longer-lasting bonded joints in vehicles, and robust composites for infrastructure.
20. Accelerated Battery and Energy Storage Material Development
By predicting ion diffusion, electrode-electrolyte interface stability, and electrolyte decomposition pathways, AI aids in identifying next-generation battery materials with higher capacities, longer lifetimes, and improved safety.
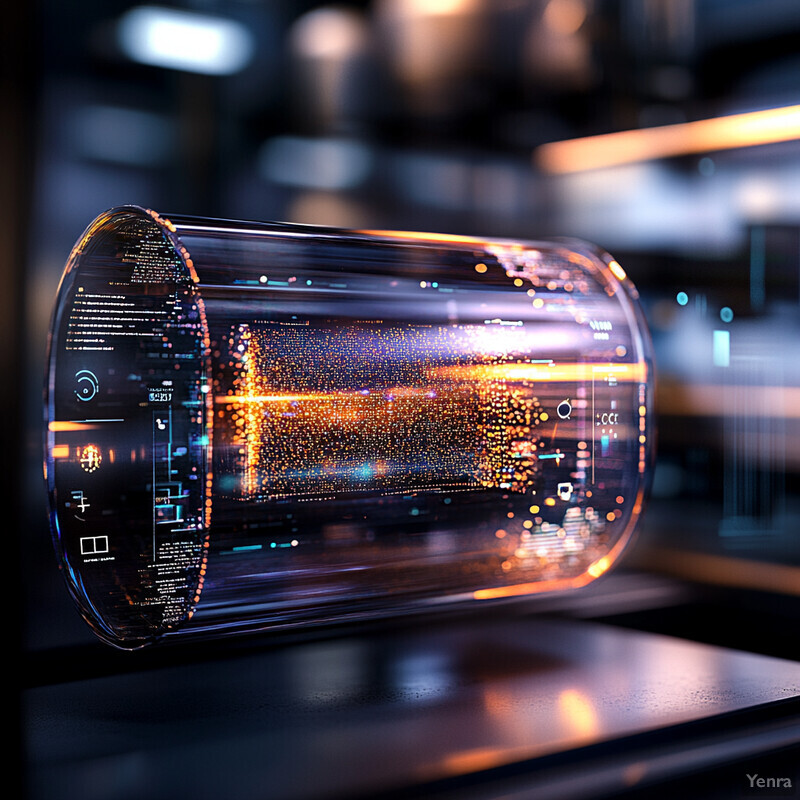
One of the biggest challenges in next-generation energy storage is identifying materials that can store more charge, operate more safely, and last longer. AI accelerates this search by predicting how ions move through electrode materials, how interfaces between electrodes and electrolytes behave, and what degradation pathways might occur during repeated charging cycles. By using machine learning to evaluate the vast design space of possible battery chemistries, structures, and additives, researchers can swiftly pinpoint candidates that promise higher energy density, better cycle life, and safer operation. This acceleration is critical for meeting global demands for renewable energy and efficient power storage solutions.