1. Improved Data Processing and Integration
AI techniques allow analysts to handle and merge massive, heterogeneous datasets—from historical price feeds, macroeconomic indicators, and demographic information to alternative data sources like social media sentiment—streamlining the entire data preparation and feature engineering pipeline.
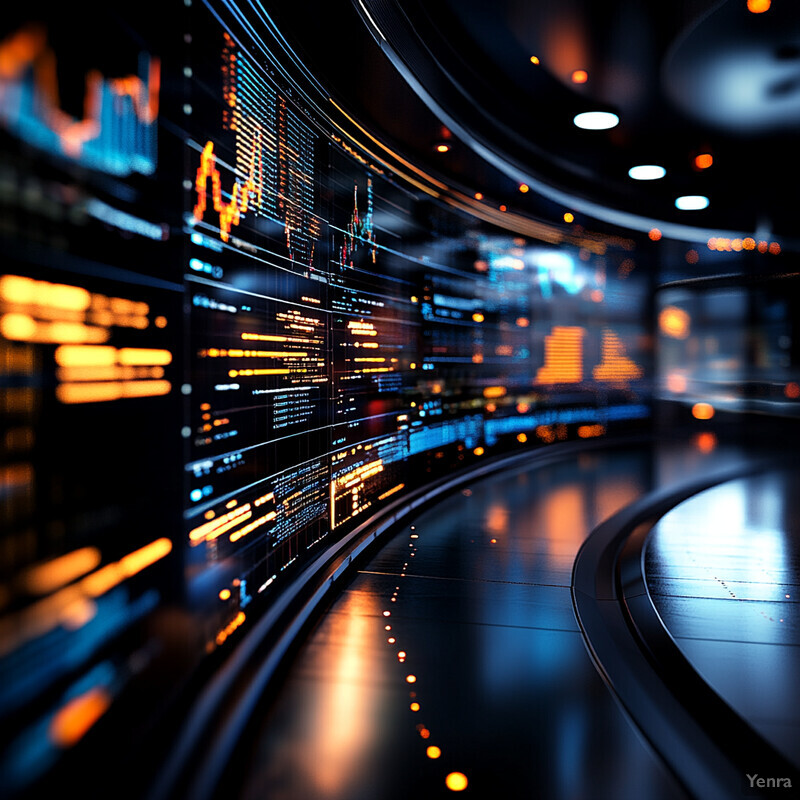
AI-driven tools empower analysts to process vast amounts of financial, economic, and alternative data at scale, integrating disparate sources into coherent datasets more efficiently than ever before. Traditional economic modeling often struggled with incomplete or unstructured data and required significant manual preprocessing. Today, advanced machine learning (ML) and natural language processing (NLP) techniques can automatically clean, normalize, and harmonize inputs from numerous sources—such as historical price feeds, macroeconomic indicators, corporate filings, weather reports, and social media sentiment—into a single, comprehensive framework. This enhanced data preparation not only reduces errors and biases inherent in manual handling but also expands the range of indicators and signals analysts can consider, ultimately enriching the quality and scope of market simulations and forecasts.
2. Enhanced Predictive Accuracy
Machine learning models, including deep learning architectures, are adept at identifying complex, nonlinear relationships between variables, resulting in more accurate price forecasts, interest rate predictions, and economic growth estimates than traditional linear models.
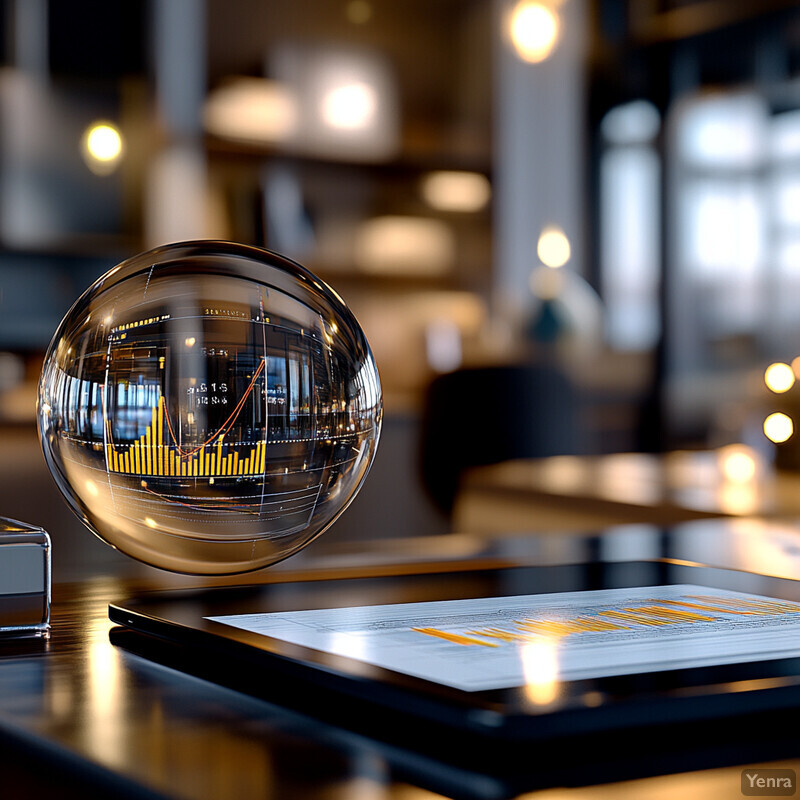
By employing more sophisticated modeling techniques, including deep neural networks, gradient boosting machines, and ensemble methods, AI drastically improves the predictive accuracy of economic and market models. Unlike traditional econometric approaches that rely heavily on linearity and a limited set of explanatory variables, AI models can uncover intricate nonlinear relationships and subtle patterns hidden in vast data. These methods are capable of learning complex dependencies between factors—such as macroeconomic shocks, trade policies, investor sentiment, and technological changes—resulting in forecasts with smaller error margins. Improved accuracy translates directly into better-informed policy decisions, more stable investment portfolios, and a clearer understanding of potential future market trajectories.
3. Real-Time Analysis and Updating
AI-driven forecasting tools can ingest and process new market data in real time, dynamically adjusting forecasts and simulations as conditions evolve.
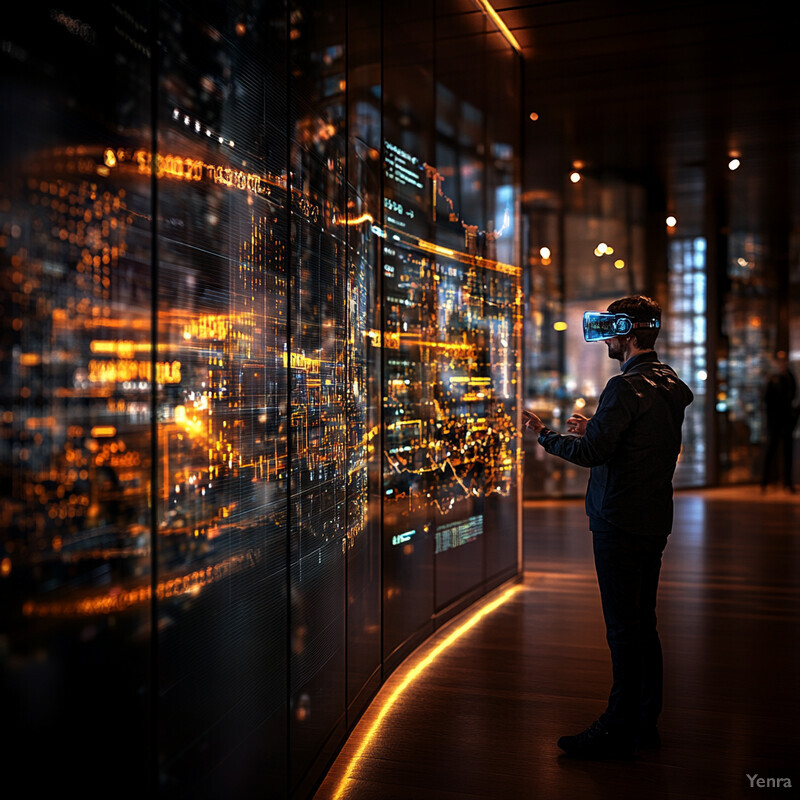
One of AI’s key advantages in economic forecasting is its ability to learn and adapt on the fly. Instead of waiting for quarterly statistical releases or monthly data updates, AI-driven models continuously absorb incoming information from a multitude of sources in real time. Through automated data pipelines and streaming analytics, these models can instantly reassess current conditions, adjusting forecasts and scenarios as soon as new data arrives. This dynamic updating process ensures that decision-makers—be they policymakers, corporate strategists, or fund managers—are always working with the most recent and relevant information. The result is a forecasting environment that closely mirrors the ever-changing nature of real-world markets, providing insights that can guide timely interventions and strategic pivots.
4. Scenario Generation and Stress Testing
Advanced simulation techniques, powered by AI, enable the creation of thousands of realistic economic scenarios that account for correlations, volatilities, and macro shocks.
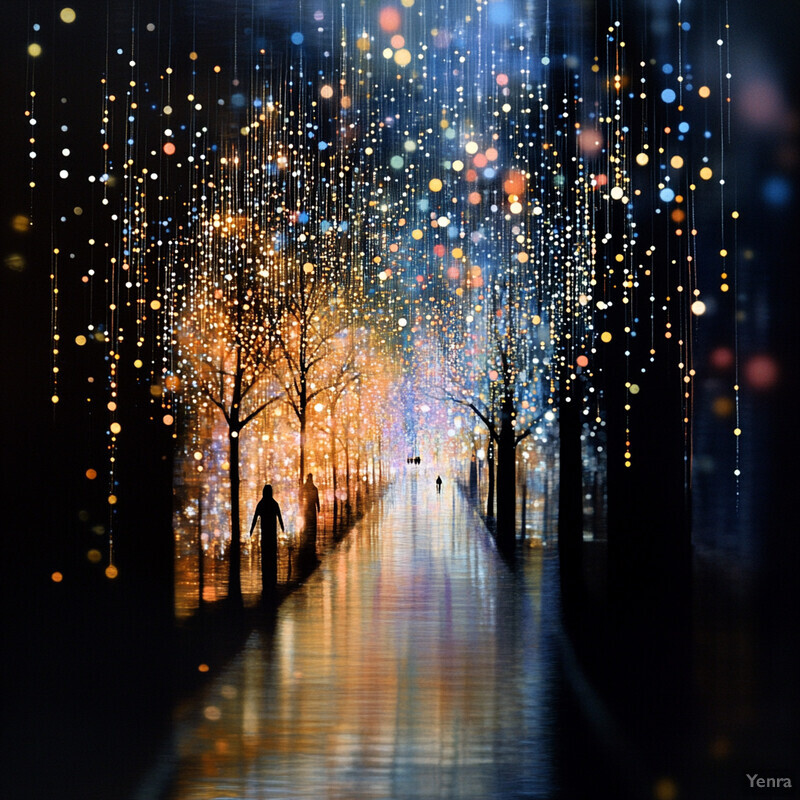
Markets are uncertain, and anticipating how they might evolve under various conditions is crucial for effective planning. AI excels at generating thousands of realistic economic scenarios and stress test environments by simulating a wide range of possible outcomes. Through approaches like Monte Carlo simulations, generative modeling, and bootstrapping techniques, AI can incorporate complex correlations, volatilities, contagion effects, and macro-level shocks into scenario analyses. This level of granularity and breadth allows institutions to rigorously test the resilience of their portfolios, fiscal policies, or strategic plans against unlikely yet plausible events. Having access to such comprehensive stress testing enhances risk management, provides insights into worst-case scenarios, and ensures that economic strategies are robust against unforeseen shocks.
5. Agent-Based Modeling with Reinforcement Learning
AI enhances agent-based economic models by using reinforcement learning to simulate interactions among heterogeneous market participants, capturing adaptation, strategic behavior, and complex feedback loops.
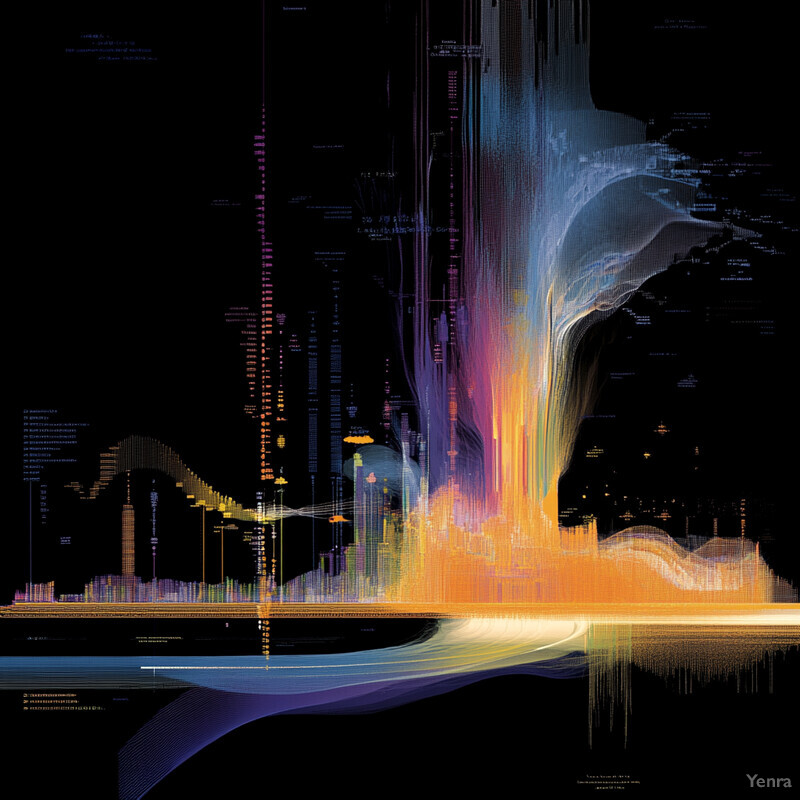
Traditional macroeconomic models often simplify or aggregate market participants into a few representative agents, limiting insights into the diversity of behaviors seen in real markets. AI-driven agent-based modeling, particularly when combined with reinforcement learning (RL), allows simulating heterogeneous actors—each with distinct strategies, preferences, and adaptive capabilities. Reinforcement learning agents learn by interacting with their environment, adjusting their strategies based on rewards and penalties. This approach closely mirrors real-world conditions where traders, firms, and consumers continuously revise their decisions in response to changing economic realities. By capturing the complexity of market ecosystems, these models provide richer, more authentic simulations that can reveal emergent market phenomena and validate the effectiveness of economic policies or regulations before they are implemented.
6. Uncertainty Quantification
Bayesian methods and probabilistic programming frameworks powered by AI help quantify the uncertainty surrounding forecasts, allowing analysts to understand not just a point estimate but the confidence intervals and probability distributions associated with predictions.
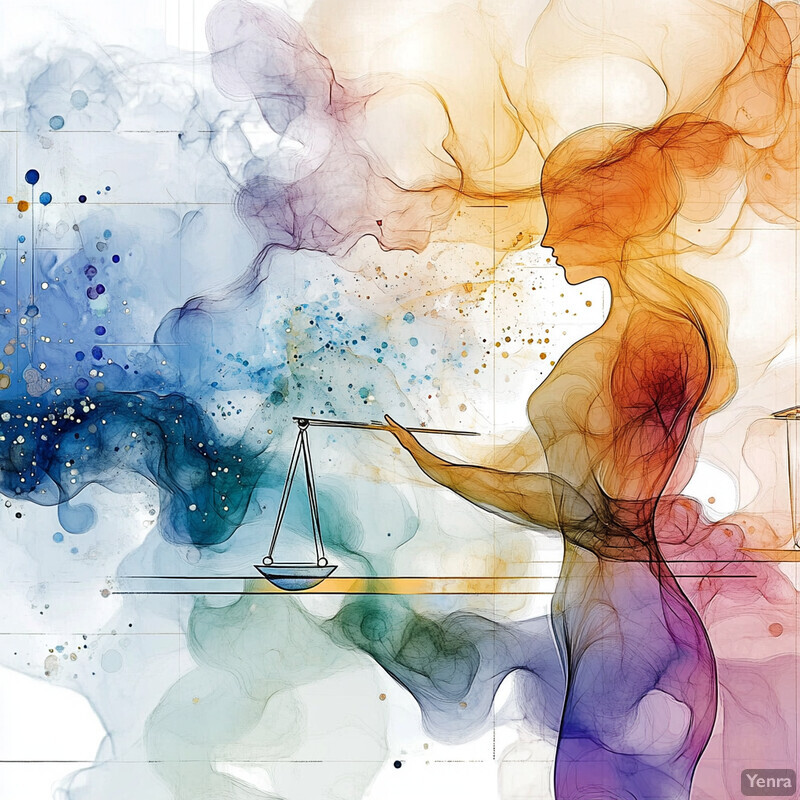
Forecasting is inherently uncertain, and understanding that uncertainty is as important as the forecast itself. AI-driven probabilistic modeling, including Bayesian inference and stochastic modeling techniques, enables analysts to quantify the range and likelihood of various outcomes rather than settling on a single-point prediction. By producing probability distributions, confidence intervals, and scenario likelihoods, these models offer a transparent view into the extent of uncertainty surrounding predictions. Policymakers and investors can therefore better appreciate the risks, weigh potential gains against probable losses, and design contingency plans. Improved uncertainty quantification leads to decisions that are more informed, nuanced, and robust against the inherent unpredictability of economic environments.
7. Automated Feature Engineering
AI-driven tools can automatically extract, select, and transform predictive features from large and complex datasets, reducing manual effort and leading to more robust and generalizable forecasting models.
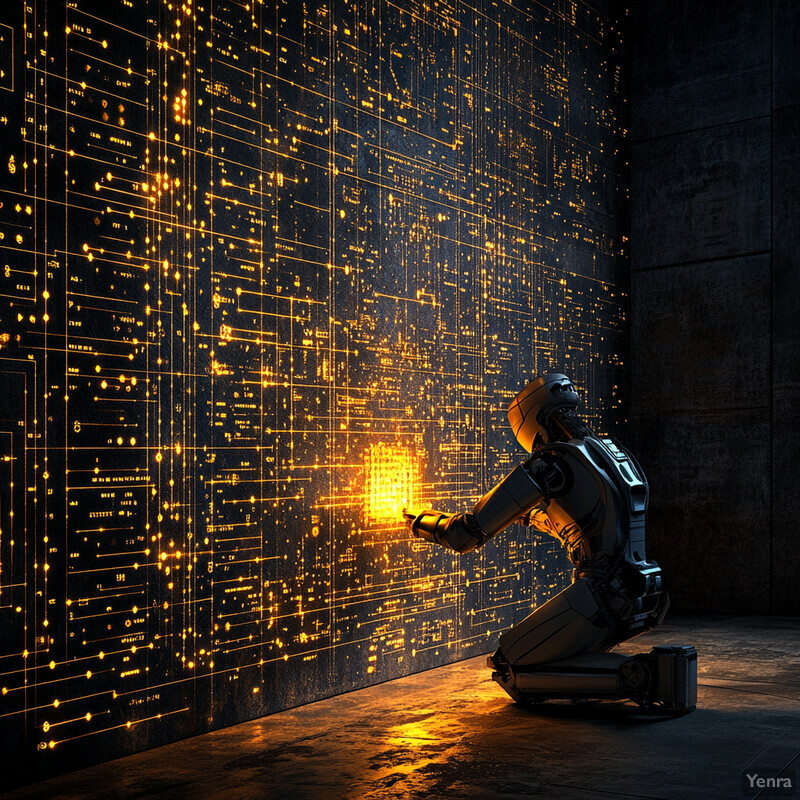
In predictive modeling, feature selection and engineering—identifying the right variables and transformations—is often a laborious, trial-and-error process. AI automates this critical step, using techniques like deep feature extraction, genetic algorithms, and unsupervised learning methods to discover and refine the most predictive variables. Such approaches can unearth latent factors, complex lags, or nonlinear combinations of inputs that would be difficult for humans to detect. By efficiently leveraging the latent information within large datasets, automated feature engineering streamlines model development, speeds up experimentation, and enhances model performance. Ultimately, this reduces the reliance on domain heuristics alone and empowers analysts to focus on interpretation, strategic insights, and model validation.
8. Nontraditional Data Sources and Sentiment Analysis
Natural Language Processing (NLP) algorithms can parse textual data—from financial news, corporate filings, policy reports, and social media—to gauge market sentiment, anticipate central bank policy moves, or detect emerging trends that influence market simulations.
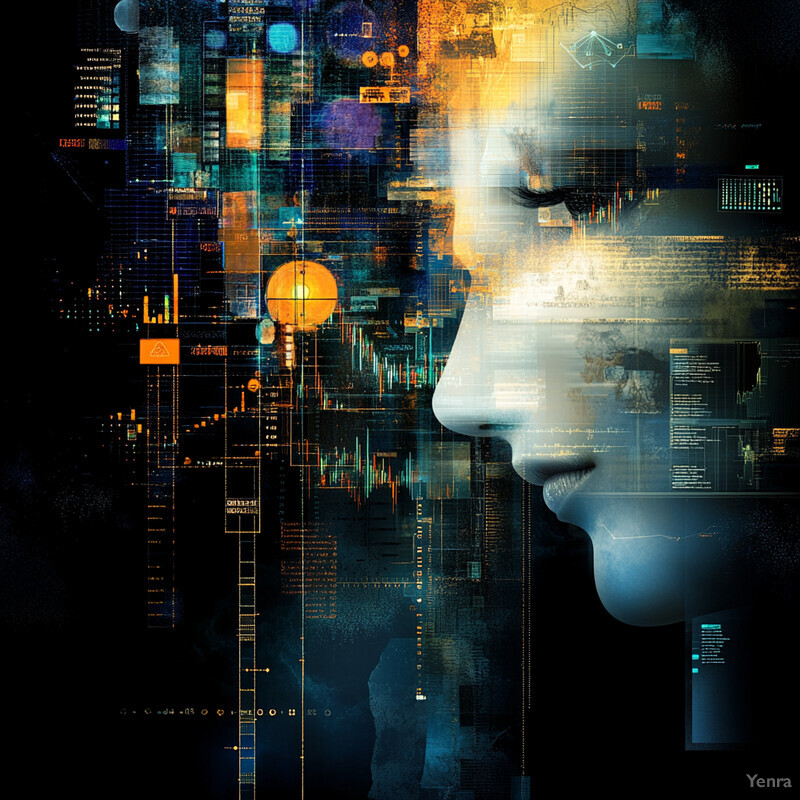
Beyond numerical financial and macroeconomic data, today’s AI models can incorporate an expanding universe of nontraditional data sources to inform market simulations and forecasts. Through NLP and advanced text-mining, machine learning algorithms can process news articles, policy speeches, earnings calls, patent filings, social media chatter, and even satellite images of shipping activity or agricultural fields. These alternative data streams reveal patterns, sentiment, and leading indicators that numerical models might miss—such as consumer optimism in certain regions, policy shifts hinted at by central bank rhetoric, or early signals of supply chain bottlenecks. By augmenting models with these rich, multidimensional signals, forecasters gain a more holistic and timely perspective on market dynamics.
9. Adaptive and Dynamic Models
Instead of relying on static relationships and coefficients, AI models can learn and adapt over time as market structures shift, whether due to technological changes, policy interventions, or evolving global trade patterns.
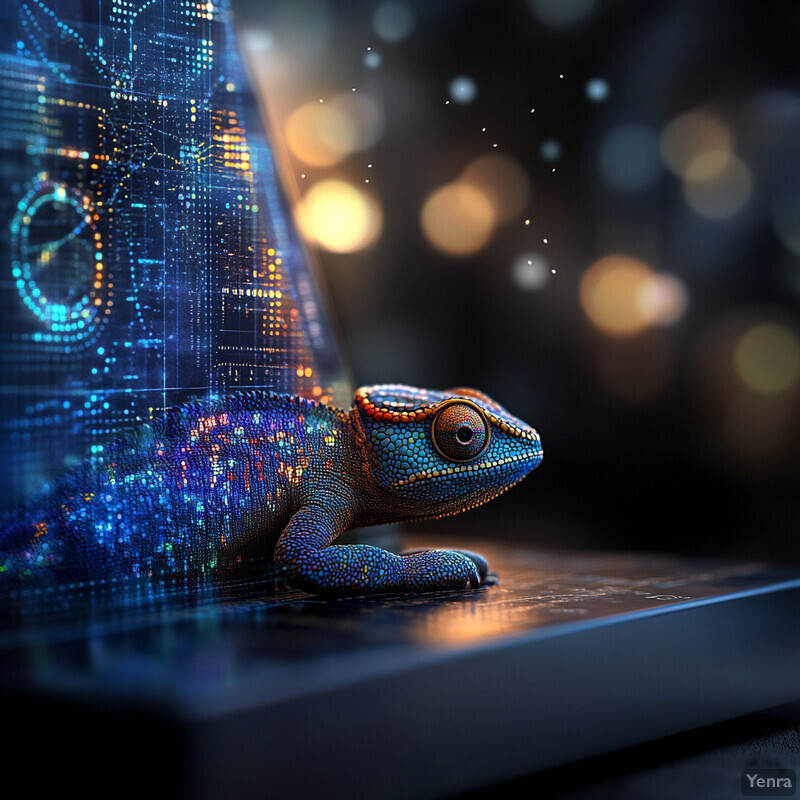
As markets evolve, so too must the models used to forecast them. Rigid frameworks locked into historical relationships often fail when faced with structural breaks, emerging industries, regulatory changes, or technological innovations. AI models can continuously learn and adapt from new data, fine-tuning their parameters or even restructuring their architectures to reflect shifting market conditions. This adaptability helps maintain model relevance and accuracy over time. For instance, if consumer preferences shift due to a new digital platform, or supply chain networks reconfigure after a geopolitical event, the model will gradually recalibrate, ensuring that forecasts remain aligned with current economic realities rather than outdated assumptions.
10. Early Warning Systems for Market Instabilities
By detecting subtle patterns and anomalies in financial and economic data, AI models can provide early warnings about potential market crashes, liquidity shortages, credit defaults, or currency runs, allowing policymakers and investors to take preemptive action.
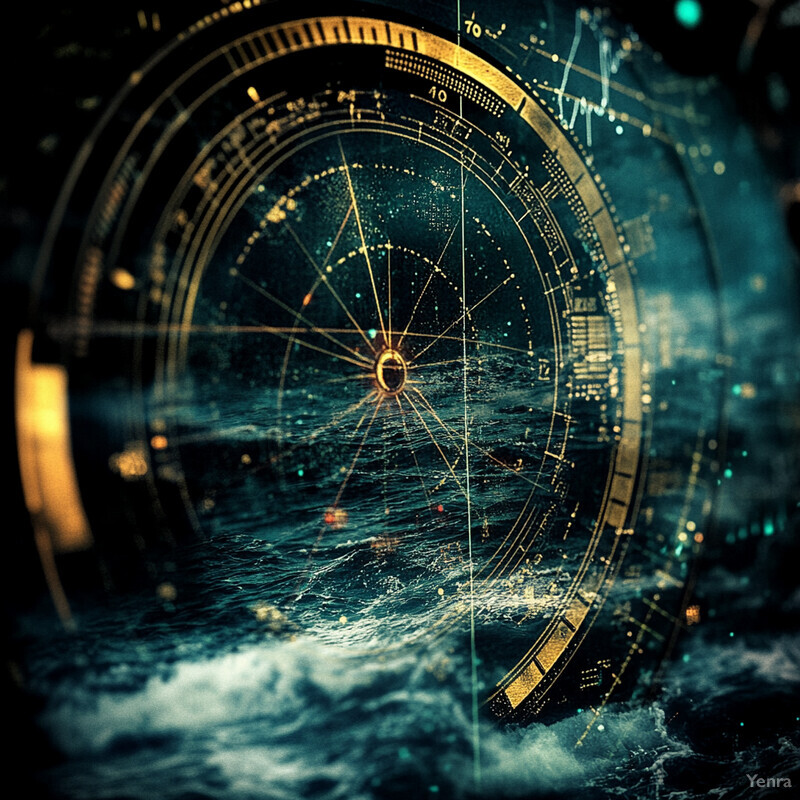
Markets can experience sudden shocks—from credit bubbles and currency crises to rapid commodity price fluctuations—and anticipating these events is critical. AI excels at detecting weak signals and subtle anomalies that might precede significant disruptions. Through continuous monitoring of high-frequency data, anomaly detection algorithms, and pattern-recognition methods, early warning systems can flag emerging imbalances or vulnerabilities. This timely alerting capability allows risk managers, regulators, and investors to intervene sooner—adjusting policies, hedging exposures, or reallocating investments to mitigate damage. As a result, such AI-driven early warning systems enhance market stability, resilience, and overall economic well-being.
11. Integration of Behavioral Economics
AI can incorporate behavioral data—such as heuristics, biases, and investor sentiment—into forecasting models, helping to simulate market scenarios that more closely mirror real human decision-making rather than purely rational assumptions.
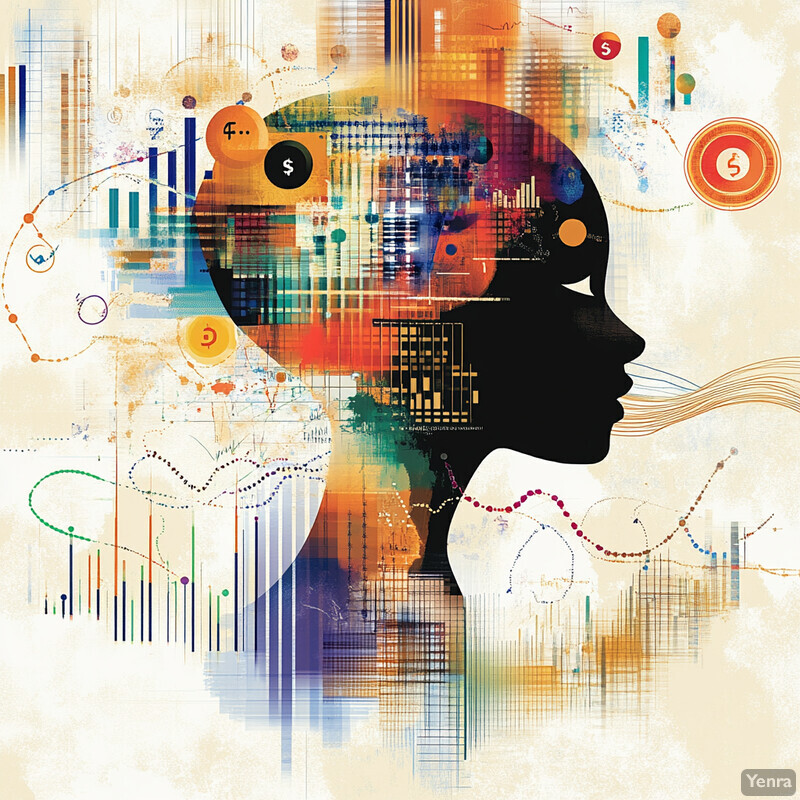
Traditional economic models often assume rational agents, but real-world decision-making is influenced by psychological factors, biases, heuristics, and sentiment. AI can incorporate these behavioral complexities into forecasting models by analyzing data on investor sentiment, consumer confidence, and documented cognitive biases. Advanced simulation frameworks can replicate how herd behavior, overconfidence, or panic spreads through networks of agents. Incorporating these behavioral elements leads to more realistic simulations that capture human decision-making patterns. This approach provides deeper insights into how narratives, beliefs, and emotions shape market outcomes, ultimately improving the relevance and reliability of both policy simulations and investment strategies.
12. Customized Forecasting for Niche Markets
AI’s scalability and automation permit the creation of forecasting models tailored to very specific sectors, regions, or niche asset classes, enabling institutions to gain insights into smaller, less liquid markets that might have been overlooked by traditional methods.
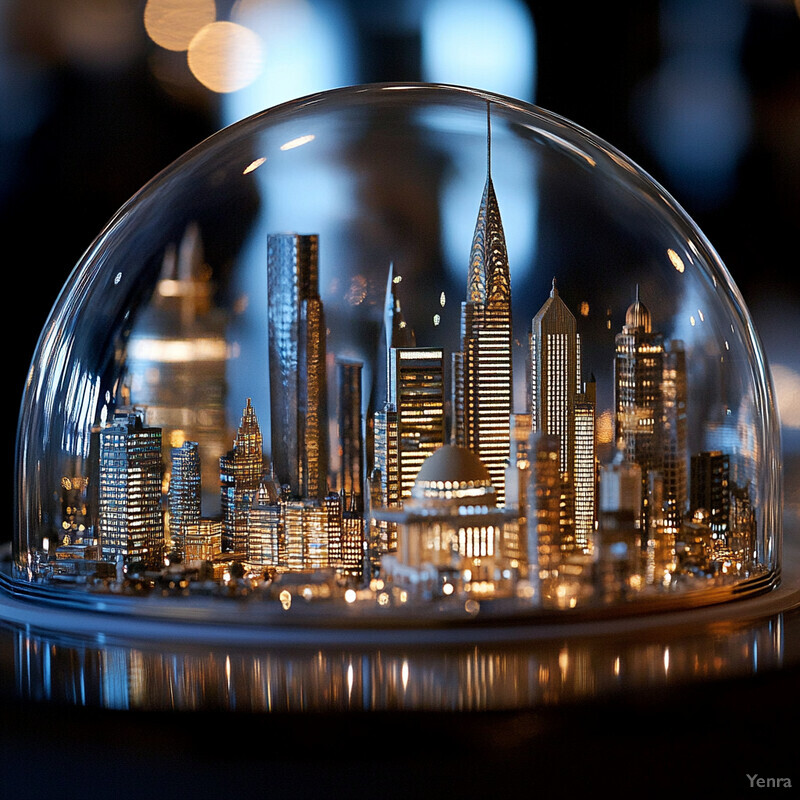
Many traditional models focus on large, liquid markets or broad macroeconomic aggregates. Yet AI can be trained on smaller, specialized datasets to deliver granular forecasts for niche markets—such as rare metals, local real estate segments, emerging technology startups, or region-specific commodities. Automated modeling tools and efficient learning algorithms allow for scalable customization, meaning analysts can develop reliable models even for domains where data may be sparse but still valuable. Access to specialized forecasts empowers local businesses, regional policymakers, and specialized asset managers to make informed decisions, enhance competitiveness, and better understand localized economic conditions.
13. Multivariate Time Series Modeling
AI techniques excel at handling complex time series with multiple interdependent variables, allowing for refined economic forecasts that account for overlapping factors, lags, and seasonal patterns.
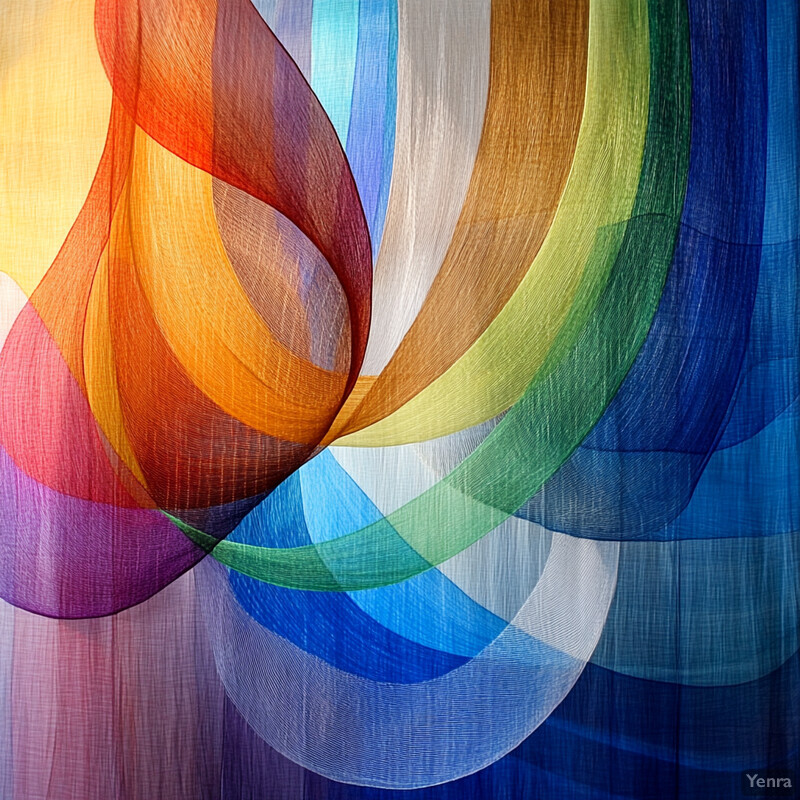
Economic and financial phenomena unfold over time, often with multiple interdependent factors influencing one another. AI-driven time series models, particularly sequence models like LSTM (Long Short-Term Memory) networks and Transformer architectures, excel at capturing long- and short-term dependencies within multivariate data. These models can handle overlapping, seasonal, or lagged effects among variables—like interest rates, employment figures, commodity prices, and exchange rates—improving the holistic understanding of how changes in one indicator propagate through the system. The outcome is more accurate temporal forecasts that help decision-makers anticipate and navigate trends, cycles, and turning points more effectively.
14. Geospatial and Granular Data Utilization
High-resolution data—such as satellite imagery, shipping data, and localized economic indicators—can be processed by AI models to enrich simulations and forecasts with spatially and contextually aware insights.
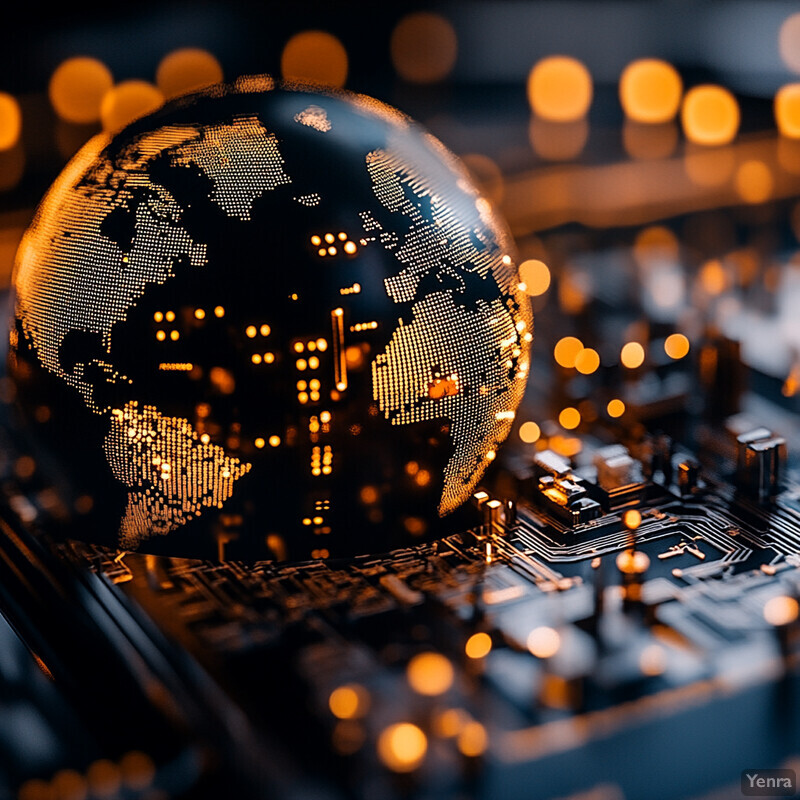
Markets and economies do not operate uniformly across geographies. AI models can integrate geospatial data—such as regional economic indicators, shipping routes, localized demand signals, and even weather patterns—into their forecasting processes. By combining location-based variables with traditional macroeconomic data, these models produce more contextually aware forecasts and simulations. This level of granularity can inform policies that address regional disparities, guide infrastructure investments based on localized economic conditions, or help companies tailor distribution strategies to specific areas. In essence, incorporating geospatial and granular data makes economic forecasts more nuanced, realistic, and actionable.
15. Automated Model Validation and Backtesting
AI systems can perform rigorous validation, cross-validation, and backtesting of economic models automatically, ensuring that the chosen approach provides robust and stable predictions across different time periods and market conditions.
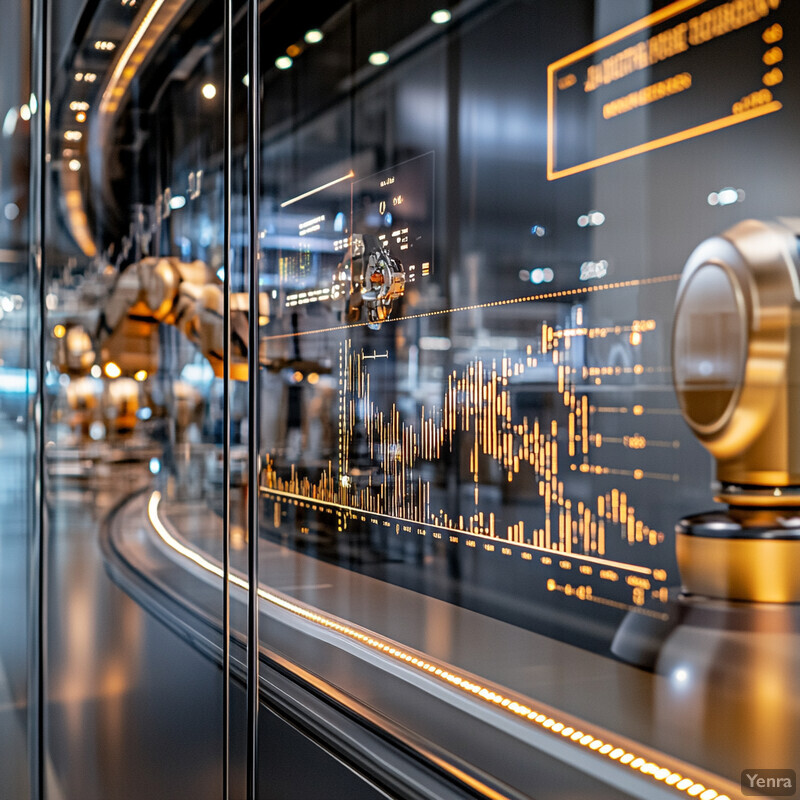
Validating the reliability of economic models is a critical but labor-intensive step. AI can automate much of the validation and backtesting process, systematically comparing model predictions against historical data and alternative models. Through this automated approach, analysts can identify model weaknesses, biases, or periods of poor performance more quickly and comprehensively. By continuously refining the validation process, analysts ensure that only robust, stable, and well-calibrated models are used for policymaking or investment strategy. Automated validation and backtesting also foster transparency and trust in the modeling framework, as stakeholders can be confident that the chosen models have been thoroughly vetted.
16. Interpretable and Explainable AI Tools
Advances in explainable AI allow analysts and policymakers to understand the drivers behind AI-based forecasts, improving the trustworthiness of models and facilitating informed decision-making even when models are complex.
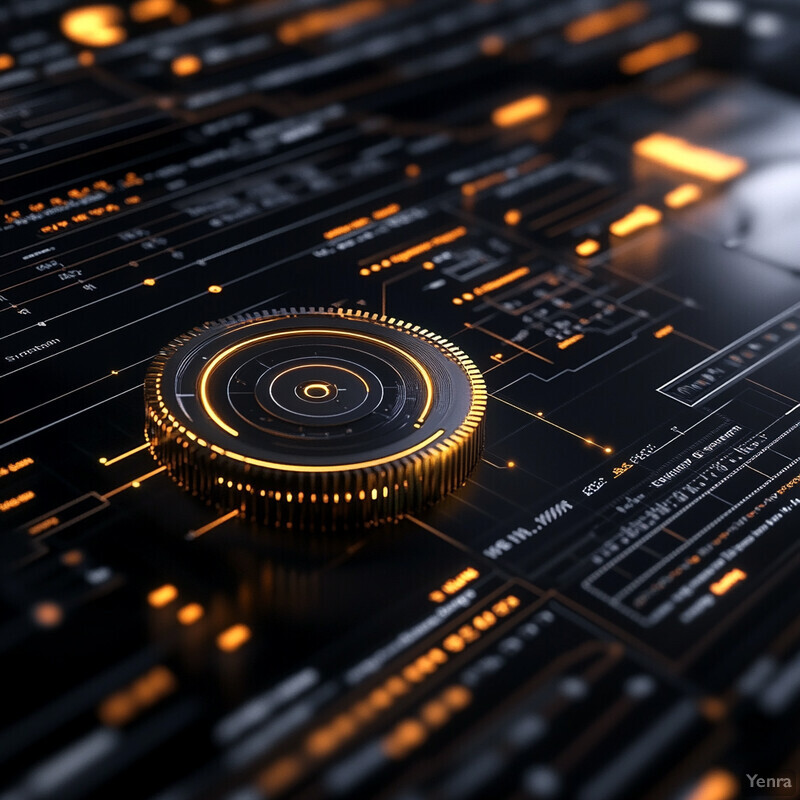
As AI models become more complex, understanding their inner workings becomes increasingly important. New techniques in explainable AI help analysts, policymakers, and stakeholders see which variables are most influential, how changes in one factor alter the forecast, and why the model reached a particular conclusion. This transparency improves trust and makes it easier to communicate insights to non-technical audiences. For regulators and executives, interpretability fosters accountability and confidence in using AI-based forecasts to guide major decisions. Ultimately, explainable AI bridges the gap between a model’s complexity and a decision-maker’s need for clarity and rationale.
17. Integration with Optimization and Decision Support
AI-driven forecasting models can be directly coupled with optimization frameworks, providing policy planners, central banks, and investment managers with actionable recommendations on capital allocation, risk mitigation, or macroeconomic policy choices.
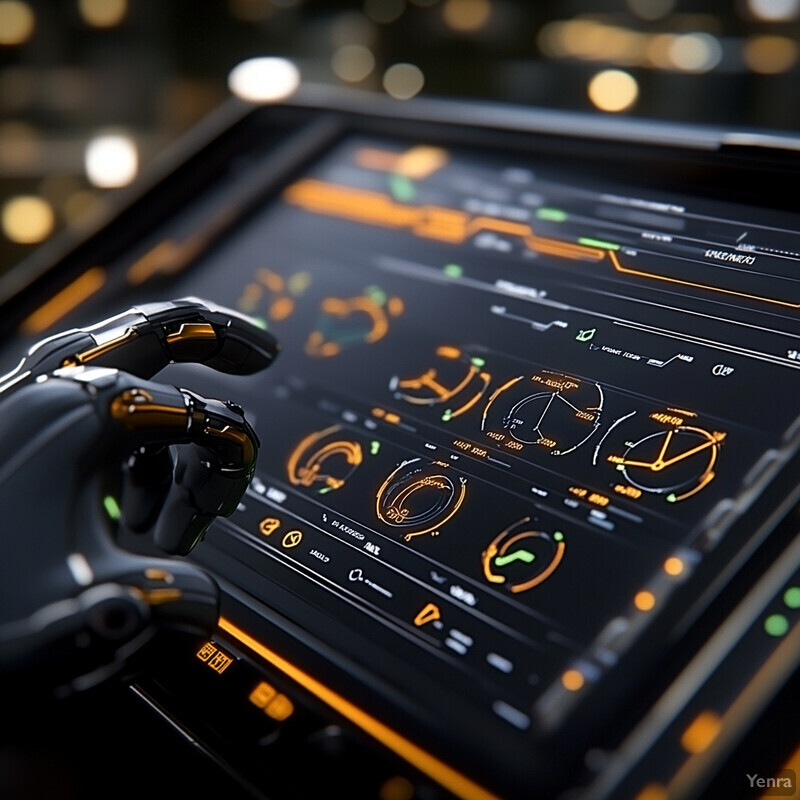
Forecasting is often just one step in a decision-making process. AI can seamlessly integrate forecasting models with optimization frameworks, enabling automatic generation of actionable recommendations. For instance, once an AI model predicts future commodity prices and demand trends, it can feed those results into an optimization system that suggests ideal inventory levels, hedging strategies, or energy generation plans. By coupling forecasting and optimization, organizations can move from reactive decision-making to proactive, data-driven strategy development. This end-to-end approach transforms forecasts into tangible policy guidelines, investment allocations, and operational plans that respond dynamically to evolving economic landscapes.
18. Reduced Subjectivity and Bias
While no system is completely free of bias, AI can reduce certain human biases by relying on data-driven decisions, incorporating feedback loops that minimize errors influenced by subjective judgments or entrenched market conventions.
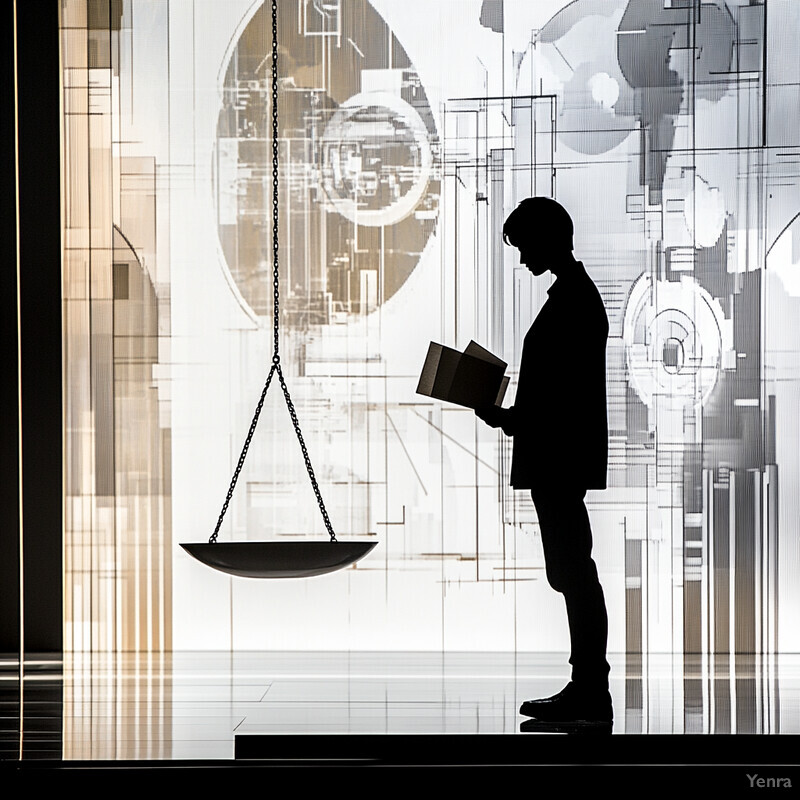
Economic forecasts have historically been influenced by human biases, entrenched ideologies, or selective interpretations of data. AI, while not entirely free of bias, relies primarily on data-driven learning processes, which can reduce certain subjective influences. Over time, feedback loops and rigorous validation steps can further minimize model biases. By grounding forecasts and simulations in empirical patterns rather than personal intuition or conventional wisdom, AI-based forecasting produces results that are more objective and consistently replicable. While domain expertise still plays a crucial role in interpretation, data-driven methodologies ensure that models do not simply reflect the researcher’s preconceptions.
19. Robustness Against Structural Breaks
Advanced AI methodologies, such as transfer learning and meta-learning, can handle situations where traditional models fail due to regime changes, economic shocks, or technological disruptions, making forecasts more resilient to unexpected shifts.
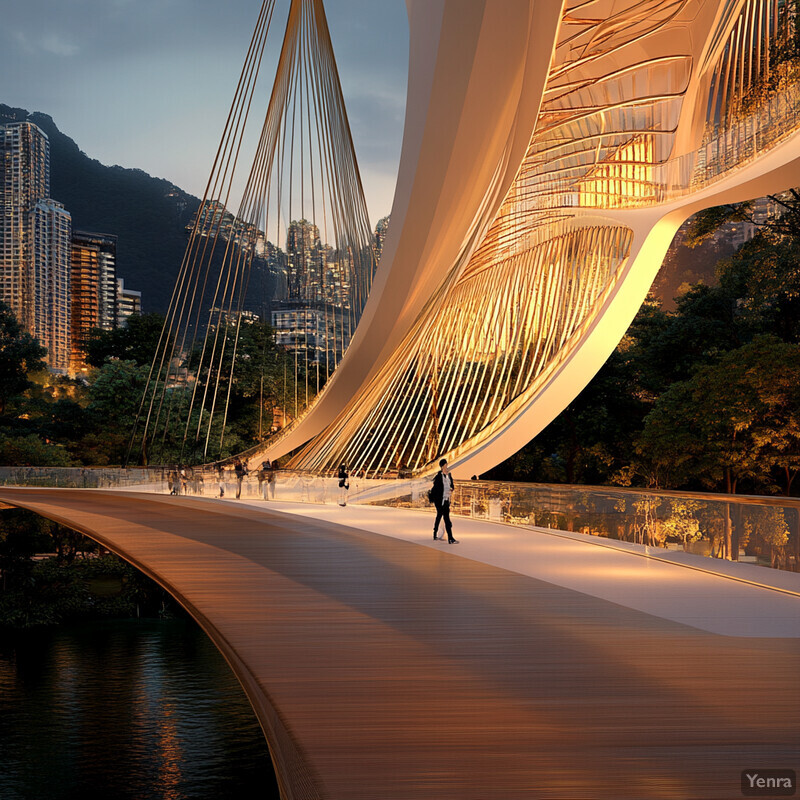
Economies evolve, and unexpected structural changes—such as new technologies, political upheavals, regulatory shifts, or global crises—can challenge traditional forecasting models. AI methods, particularly those leveraging techniques like transfer learning, continual learning, and meta-learning, can adapt to these structural breaks by quickly retraining on new data and partially reusing learned representations. This adaptability allows the forecasting system to remain accurate even as the underlying economic regime changes. By maintaining robustness in the face of unpredictable shifts, AI-enhanced models offer greater reliability and longevity than static, one-size-fits-all approaches.
20. Cost and Time Efficiency
By automating large parts of the forecasting and simulation pipeline, AI reduces the time and expense associated with manual modeling, data cleansing, and scenario testing, freeing analysts to focus on higher-level insights and strategy.
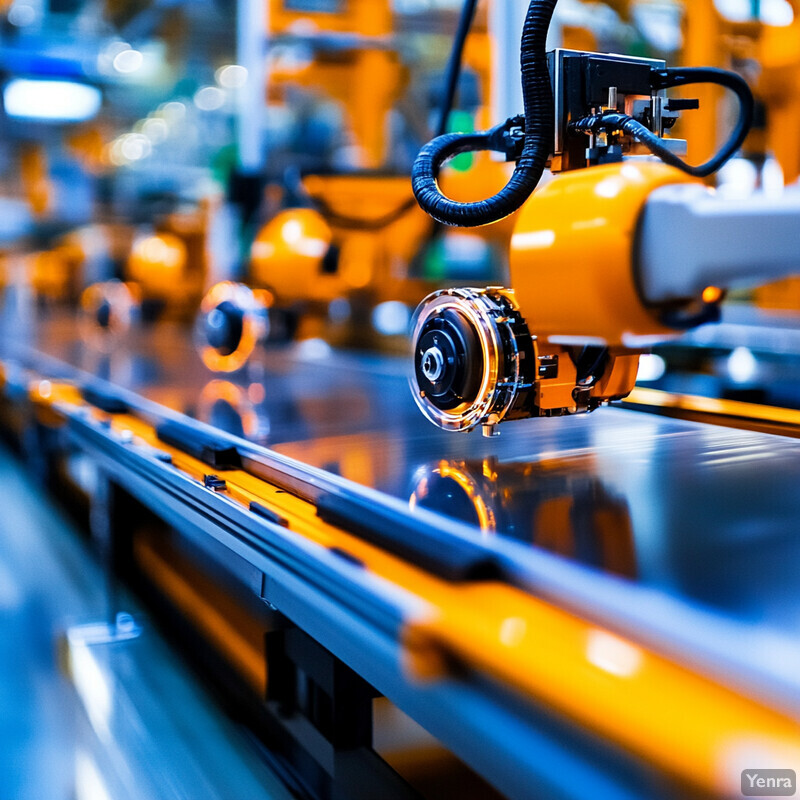
Finally, AI automation streamlines the entire forecasting process, from data collection and cleaning to feature engineering, model building, validation, and scenario generation. Tasks that once required teams of analysts and weeks or months of effort can now be performed in a fraction of the time, at scale. This efficiency lowers the barriers to entry for complex forecasting initiatives, allowing smaller institutions, nonprofits, or emerging-market policymakers to access high-quality forecasts. Reduced costs and faster turnaround times free analysts to focus on higher-level strategic analysis, interpretation, and communication, ultimately improving the speed and impact of economic decision-making across the board.