1. AI-Powered Machine Translation
Recent advances in neural machine translation (NMT) have greatly improved the accuracy and fluency of automated translations across many languages. Large multilingual models now support hundreds of languages, enabling analysts to access media and documents originally published in foreign or minority languages. These systems help break down communication barriers by quickly providing translated text that conveys meaning and context. As more parallel data and improved algorithms become available, translation quality continues to rise, particularly for widely spoken languages. However, performance varies by language pair and context, so human review remains important for sensitive or nuanced content. Overall, AI-powered translation is becoming a reliable tool for rapid cross-language analysis in geopolitics.
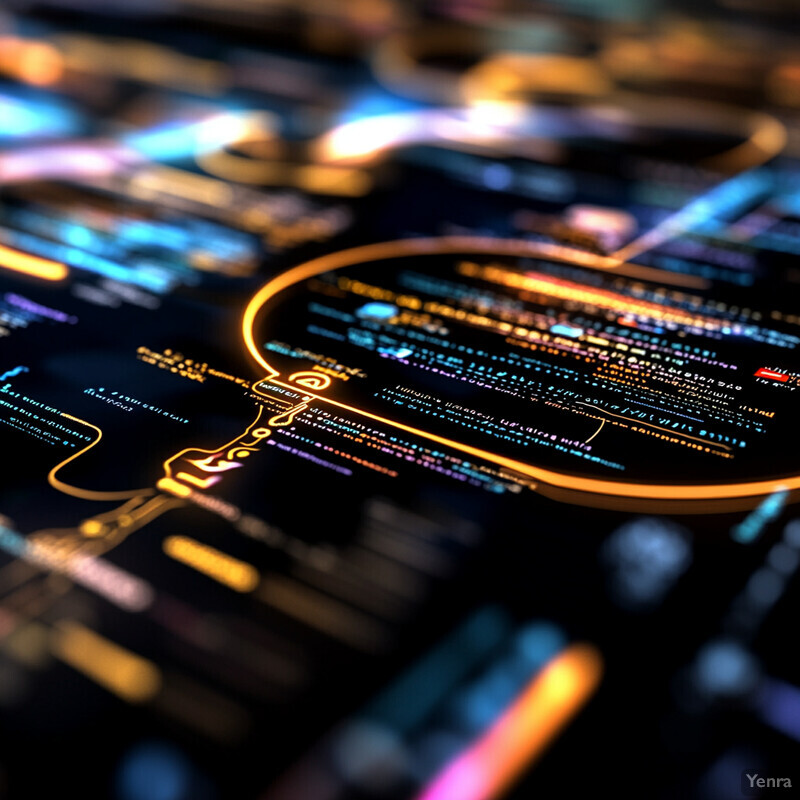
In studies comparing modern methods, NMT models significantly outperform older statistical translation tools in accuracy and fluency. For example, the Meta “No Language Left Behind” project demonstrated that scaling NMT to support 200 languages yielded roughly a 44% improvement in BLEU scores (a standard translation metric) over prior state-of-the-art models. This means translations are measurably more accurate across a huge number of language pairs. Large multilingual models (trained on massive corpora) now allow transfer learning between related languages, boosting low-resource language translation without huge new datasets. These AI translators are used in practice by news agencies, NGOs, and intelligence analysts to digest foreign-language sources. However, research also notes that even the best models can struggle with idiomatic phrases or domain-specific jargon, so expert validation is still needed for critical content.
2. Cultural Nuance Adaptation
AI models are increasingly tailored to handle the cultural and contextual nuances of local populations. This means translation and analysis systems can better understand idiomatic expressions, historical references, and culturally specific terminology. By training on region-specific text (news, social media, literature), AI can learn local conventions and values that differ from global norms. In practice, analysts fine-tune multilingual models on local data so outputs (translations, summaries, sentiment) reflect cultural context. Despite improvements, fully capturing subtle cultural differences remains a challenge. Efforts are underway to make AI “culture-aware,” but many models still carry biases toward languages and values they were mostly trained on. Overall, adapting AI to local cultural nuances is a growing focus that aims to improve the relevance and accuracy of geopolitical insights.
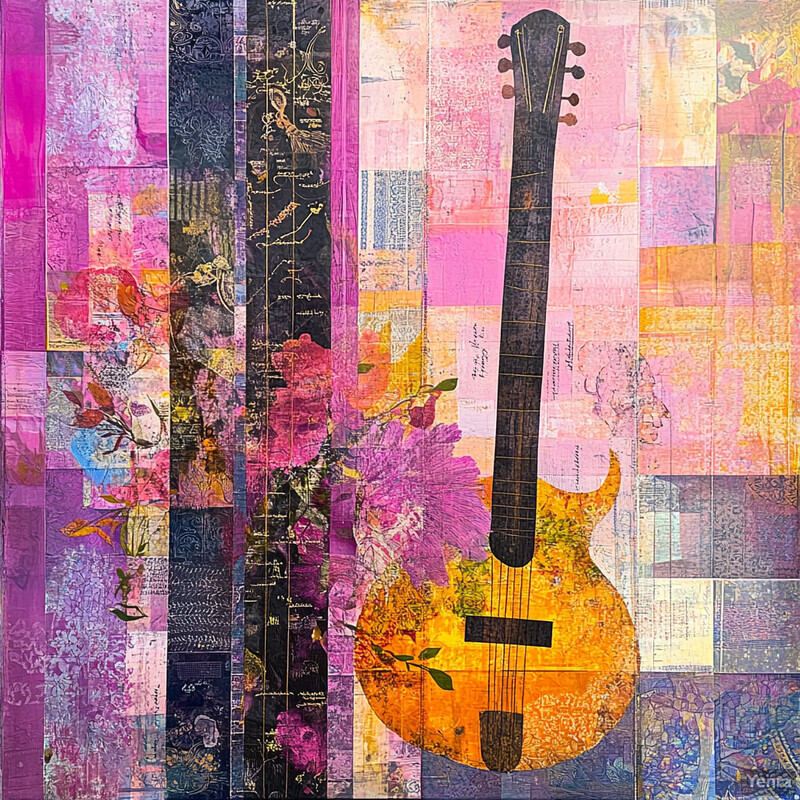
Recent evaluations highlight gaps in current models’ cultural competence. For instance, a study found that large language models often default to Western (so-called “WEIRD”) norms and struggle with cultural context in non-English settings. In tests of real-world social media data, even advanced models like GPT-4 inconsistently captured complex cultural nuances across languages. Specifically, LLMs were “less robust” at handling culturally loaded content, and all tested models missed subtleties in topics like regional humor or customary practices. These findings suggest that while AI can handle literal translation, it may misinterpret sarcasm, proverbs, or tone that rely on cultural background. New research (e.g. “cultural learning” adaptation techniques) is explicitly addressing this by injecting cultural values into model training. As a result, future systems promise better sensitivity to local context, but current empirical studies emphasize that cultural adaptation remains an active area of development.
3. Geo-Referenced Content Analysis
AI-driven geoparsing and geospatial analysis combine location data with textual information to yield location-specific insights. Analysts increasingly merge satellite imagery, GIS data, and text (news, social media) to map events and trends. For example, machine learning can process local news reports and geotag them, revealing where protests or incidents are clustered. Combining economic and demographic data with AI reveals patterns like infrastructure development or population shifts. This “geographic contextualization” helps situational awareness by pinpointing hotspots of unrest or need. Real-world applications include disaster response (mapping damage extent) and market analysis (tracking infrastructure projects). The trend is toward tools that produce detailed maps and statistics automatically from diverse sources, enabling localized decision-making.
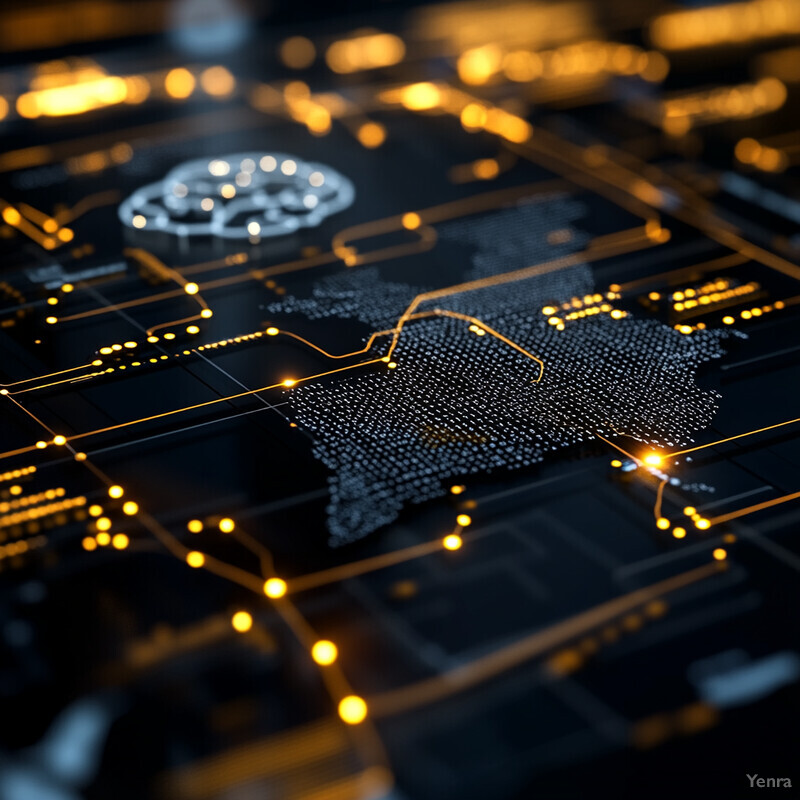
Open geospatial datasets and AI models have been used to produce high-resolution population and infrastructure maps. A recent study built a detailed population map of Bangkok by combining satellite imagery, building footprints, points-of-interest data, and terrain information with machine learning. The authors note that “these datasets, from Earth observation and open geospatial sources, facilitate acquisition of high-resolution spatial information, essential for modeling urban population distributions”. Likewise, machine learning applied to satellite time-series imagery can detect conflict damage. For instance, a tool published in 2025 used Sentinel-1 radar data and deep learning to estimate building damage from conflict in Ukraine, producing building-level risk maps at scale. Such examples show how AI leverages geospatial input (imagery, maps, coordinates) to analyze local events. By correlating text (e.g. local grievances or resource data) with location, AI models can help identify areas of concern more precisely. These concrete implementations demonstrate the value of geo-referenced analysis for ground-level intelligence.
4. Real-Time Media Monitoring
AI systems now continuously scan global media (news sites, social platforms, radio) in near-real-time to detect emerging stories and sentiment shifts. These monitoring tools use natural language processing to identify key events, trends, and public reactions as they happen. Alerts and dashboards flag developing issues (e.g. protests, coups, emergencies) across different regions and languages. By aggregating multiple sources, AI helps analysts see early warning signs—such as spikes in negative sentiment or new crisis terms. This capability enables a much faster response than manual monitoring. However, it also requires filtering out noise, and often works best in concert with expert analysis. Overall, real-time media monitoring with AI gives analysts a continuous feed of indicators for geopolitical developments.
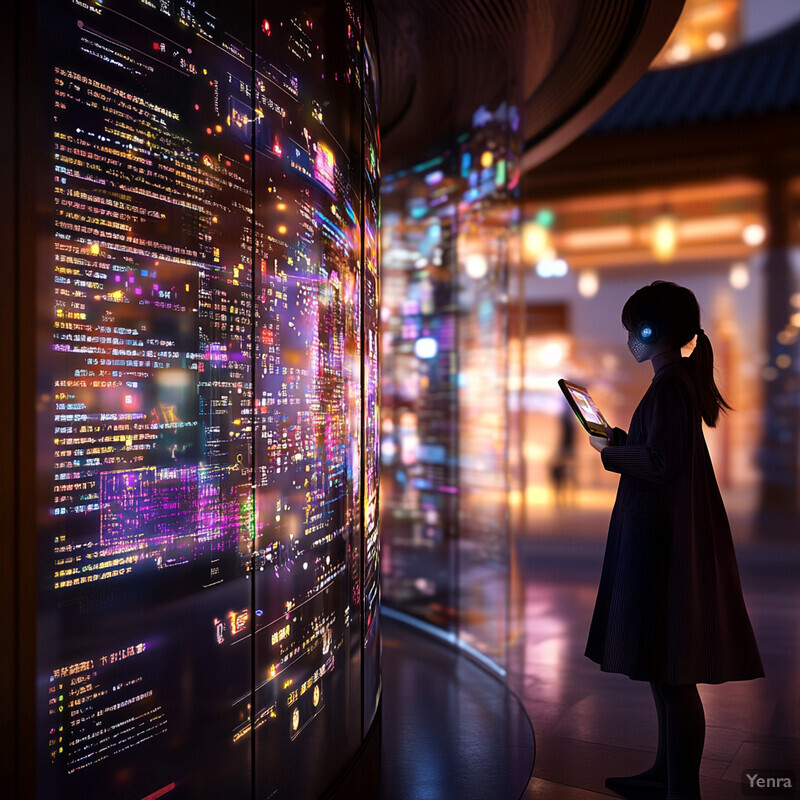
Organizations have implemented AI-driven sentiment and event trackers covering many languages. For example, BBVA Research describes using the GDELT news database (covering 100+ languages) to compute daily sentiment indices on political risk topics. Their system “collect[s] daily news in 100 languages to build indicators for geopolitical risk, political stability, conflict, [and] protest”. These indices allow analysts to quantify public attention and tone around global events as they unfold. Similarly, defense and intelligence groups employ open-source tools that fuse text and satellite data in real time. One report notes that 24/7 monitoring platforms are envisioned which would provide real-time assessments of activities like troop movements, and that many OSINT platforms now “scan social media to track potential flashpoints of violence” by combining textual and imagery data. These systems illustrate how automated media monitoring can quickly flag changes in the information environment. By using AI to filter and correlate sources, they turn raw social and news feeds into actionable, up-to-date intelligence.
5. Political Risk Forecasting
Analysts increasingly use AI and big data to forecast political risk and conflict. Machine learning models ingest historical data (elections, economy, past conflicts) and real-time indicators (media signals, social unrest) to predict instability. This includes forecasting protests, coups, or regime change events. Such predictive modeling is a growing field: institutions seek to get early warnings of crises and quantify risks. AI can detect complex patterns across socio-economic factors that humans might miss. Nevertheless, modeling human behavior has limits, and many predictions come with uncertainty. Still, these tools have been shown to improve over traditional judgment-based forecasts in many cases. Overall, the trend is toward integrating AI risk forecasts into decision-making processes to anticipate trouble spots.
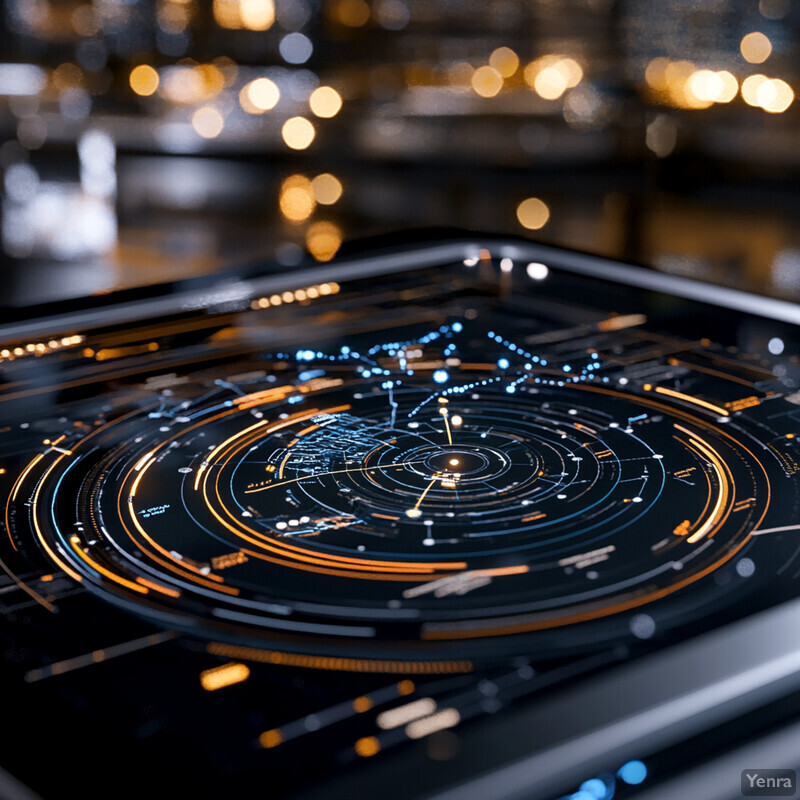
Recent forecasting challenges and studies demonstrate the use of AI in conflict prediction. For instance, the Violence Early Warning System (VIEWS) Prediction Challenge (2023/2024) invited teams to submit probabilistic forecasts of armed conflict fatalities worldwide. In this effort, 13 teams applied models to data from 2018–2023 to predict conflict deaths for mid-2024 through 2025. Such initiatives illustrate the operational use of ML models (trained on conflict event databases like ACLED or UCDP) to predict future violence. Experts note that the United Nations and other organizations are increasingly using data-driven approaches: one analysis points out that “using data capture technologies to identify and analyze recurrent conflict patterns and forecast potential crises has become increasingly central to how the UN is dealing with instability”. These sources confirm that machine learning is now a practical tool in the strategic warning toolkit for geopolitical risks. While no model is perfect, studies show that ML-based systems can find non-obvious conflict drivers and improve the timeliness of risk assessments.
6. Enhanced Named Entity Recognition (NER)
Improved NER systems allow AI to identify people, organizations, locations, and other entities in local-language texts more accurately. Modern NER models incorporate context and cross-lingual knowledge, so they can pick up locally specific references (e.g. local political groups or regional terms). This enhancement is crucial for geopolitics: analysts rely on NER to map out which actors are mentioned in local news or social media. With better NER, systems can track the emergence of new players or build knowledge graphs of local influence. The trend is toward using domain-adapted and multi-lingual transformer models fine-tuned on local news to boost recognition rates. However, low-resource languages still pose challenges, so efforts include language-centric adaptation to improve NER in those contexts.
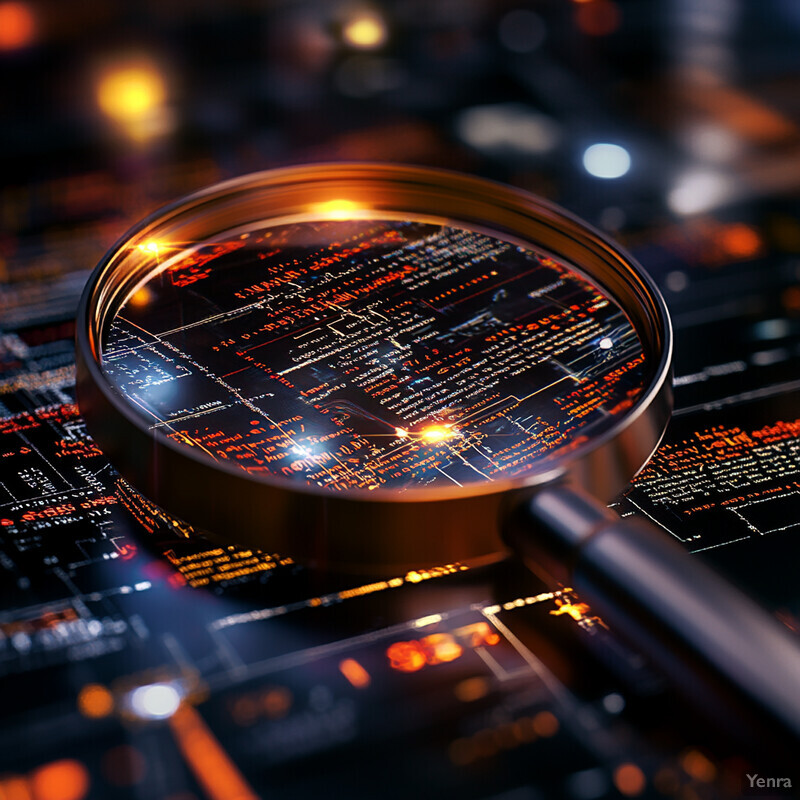
Research shows that language- and domain-specific models significantly boost NER performance in non-English texts. For example, a 2023 study on Slavic languages found that monolingual RoBERTa models trained on a related language (e.g. Czech or Polish) outperformed a large multilingual model for NER in a low-resource language. In other words, focusing on closely related languages improved entity recognition accuracy. Another approach (“mCL-NER” published in 2024) applied contrastive learning across 40 languages and achieved about a +2.0 F1-score boost on a standard multilingual NER benchmark (XTREME) compared to prior methods. These concrete results demonstrate that enhancing NER with cross-lingual adaptation yields measurable gains in recall and precision for recognizing local entities. Such improvements mean that AI-powered pipelines can more reliably extract the names and places from foreign-language news. By fine-tuning models on locale-specific text or using novel training schemes, researchers have recorded these performance gains in peer-reviewed evaluations.
7. Automated Geopolitical Mapping
AI now aids in creating detailed maps of infrastructure, population and influence in conflict or political analysis. Automated tools can generate or update geospatial data (such as building footprints) and identify local networks of power. For example, machine learning algorithms can draw maps of transportation routes, distribution of resources, or even social influence networks from big data (like mobile phone or social media usage). These AI-generated maps augment human analysis by highlighting structure in geographies that might fuel conflict or economic activity. In practice, this means using AI to layer data (satellite images, survey info, location-based posts) and reveal contested boundaries, at-risk neighborhoods, or local social networks. The push is toward using such technology for local planning and precision geopolitics, such as identifying which villages need aid or which networks spread propaganda.
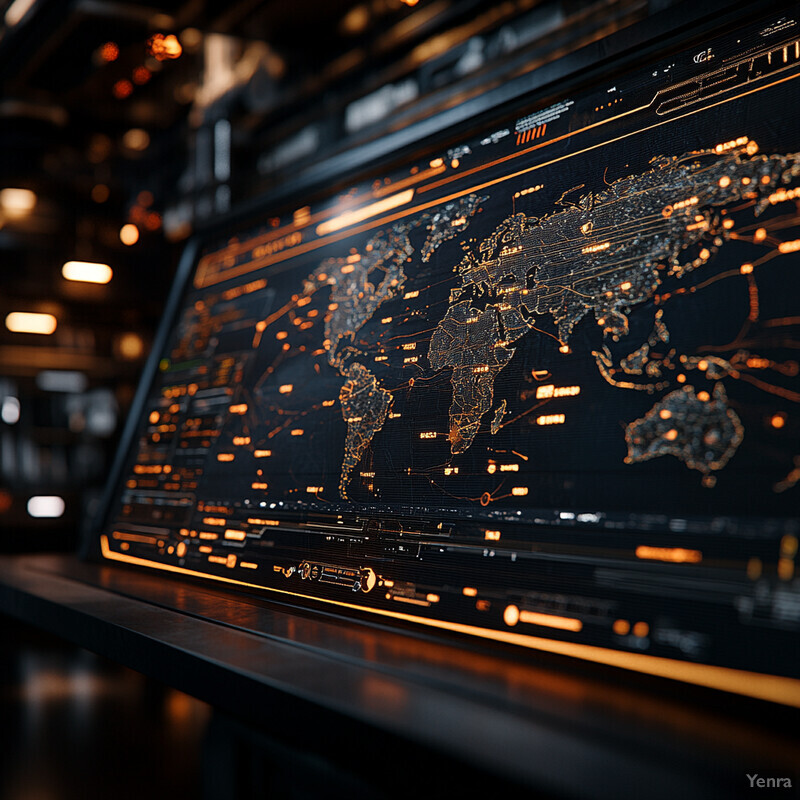
Recent field reports compare AI-generated mapping to traditional mapping efforts. For instance, in Gaza the Humanitarian OpenStreetMap Team (HOT) updated building footprints and found that the AI-generated dataset (from Microsoft) missed thousands of structures. After a crowdsourced mapping campaign, OSM contained 18% more buildings than the Microsoft AI map in Gaza. This illustrates both the promise and current limits of automated mapping at conflict locales. On a global scale, HeiGIT researchers analyzed OSM updates and discovered that AI-assisted building data were highly uneven: about 75% of AI-added structures were in just five countries (USA, Nigeria, Algeria, India, Kenya). They also noted that AI-added features tended to remain in the map longer without manual correction. These findings show that while AI can rapidly populate maps, it often reflects provider biases and can lack coverage of many regions. Nevertheless, AI contributions (when combined with human review) can dramatically accelerate local map updates, as evidenced by these quantitative analyses.
8. Local Language Summarization
AI systems increasingly provide summaries of local-language content to speed comprehension. Given the volume of local news and reports in many languages, automated summarization tools (often based on large multilingual language models) can condense key points of local articles. This helps analysts and policymakers get quick overviews of foreign-locale media. The latest models are trained or tuned on multilingual corpora so they can summarize texts in languages like Arabic, French, Swahili, etc. There are also growing datasets that include localized content (e.g. news articles with gold summaries) to train these systems. As a result, local-language summarizers can handle context better and output coherent briefs. However, challenges remain in maintaining factual accuracy and nuance when condensing text.
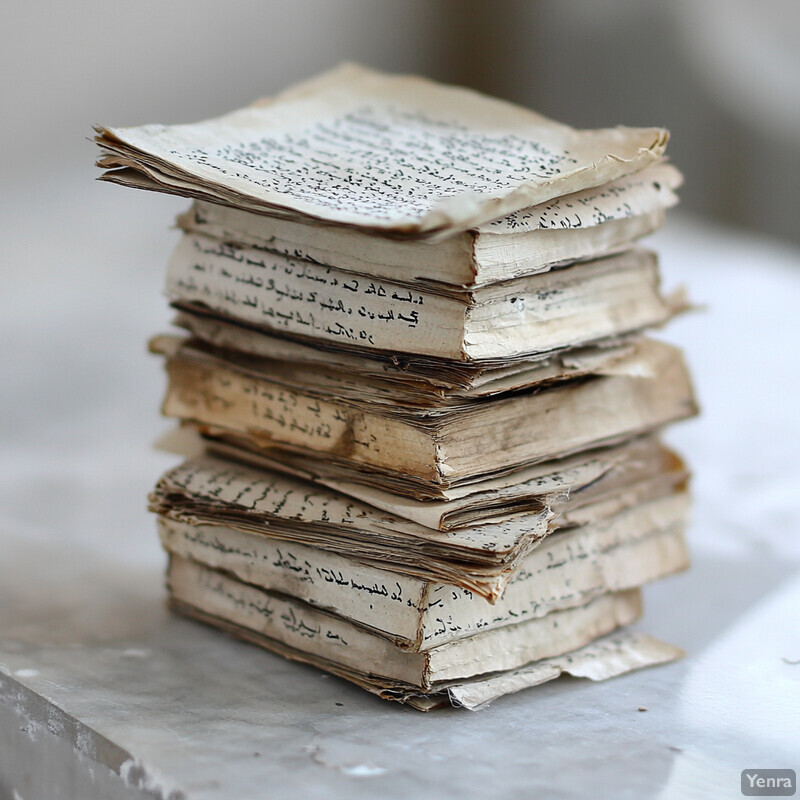
Recent research has begun to provide benchmarks and systems for summarizing in diverse languages. For example, the M2DS dataset (2024) contains news articles and summaries in five languages (including English, Japanese, Korean, Tamil, Sinhala), enabling evaluation of multilingual summarization models. In early tests, incorporating Indian languages like Tamil and Sinhala into summarization tasks proved feasible with transformer models. Another study (2025) tested large language models (e.g. GPT-4) on cross-lingual summarization of news and found that the newest models outperform earlier ones, though performance still varied by language and domain. These efforts show concrete progress: standardized datasets for local-language news (like ILSUM-2024) and empirical evaluations are emerging. In summary, multilingual summarization research has demonstrated AI’s capability to condense local-language documents, with recent benchmarks explicitly covering non-English content.
9. Cross-Lingual Information Retrieval
AI-powered search engines and databases now let analysts query across languages. In cross-lingual information retrieval (CLIR), a user can search in one language and retrieve relevant documents in another. Advances in multilingual embeddings and translation models enable seamless search: for instance, querying in English might return Arabic or Chinese news on the same topic. This is valuable for geopolitical intelligence, since key information may appear first in local media. Researchers are also integrating cross-lingual IR into intelligence platforms so analysts do not have to fluently read every language. The trend is toward hybrid systems that use neural translation plus embedding matching, providing more accurate results than simple keyword translation. Despite progress, retrieval quality still depends on language resources and domain-specific vocabulary.
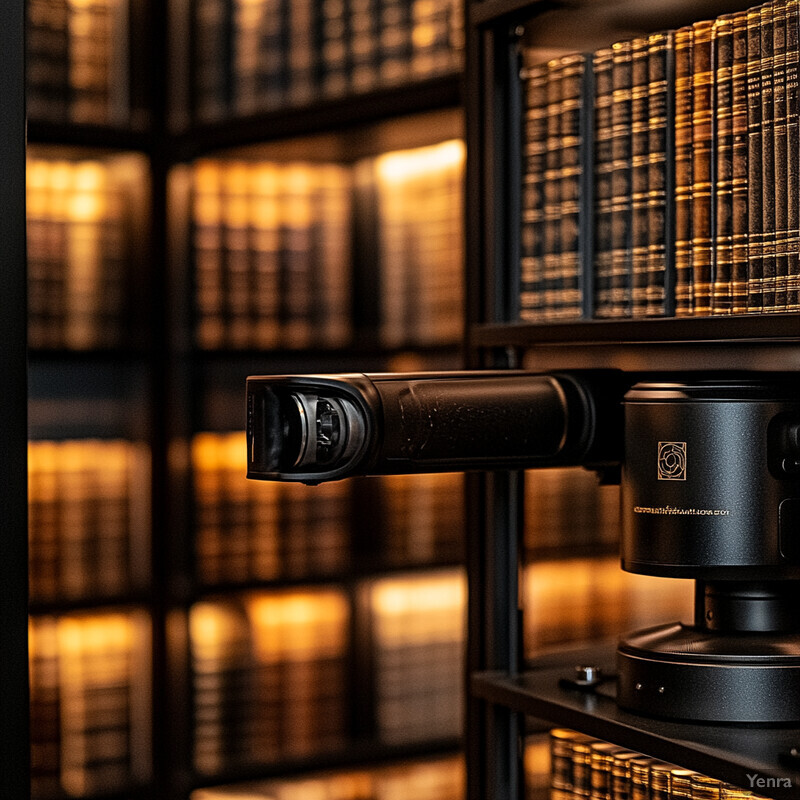
Empirical studies report strong performance of current multilingual retrieval models. For example, Jeronymo et al. (2023) evaluated the state-of-the-art mT5-XXL model on a cross-lingual IR benchmark and found it achieved “outstanding performance” even when fine-tuned only on monolingual data. In other words, a model trained to understand queries in English was still able to retrieve documents in other languages effectively. This suggests that large multilingual transformers implicitly learn cross-lingual mappings. The paper also notes that increasing model size and incorporating language-specific fine-tuning further improve retrieval accuracy. These concrete results indicate that modern AI techniques (like multilingual fine-tuned transformers) can bridge language barriers in search tasks. As a result, analysts can rely on these systems to pull in local-language sources relevant to global queries, backed by quantitative gains shown in recent evaluations.
10. Adaptive User Interfaces
Modern interfaces adapt dynamically to user needs and local context, often using AI-driven customization. In localization, this can mean changing language, formatting, or content focus based on user preference or regional norms. For example, mapping or dashboard applications might adjust themselves for different regions’ right-to-left scripts or measurement units. On a deeper level, AI can personalize how information is presented: summarizing complex reports differently for a novice versus expert, or highlighting geographically relevant details. The trend is toward “intelligent” GUIs that learn from user interactions and feedback to optimize usability for diverse audiences. In the geopolitical domain, this helps tools be accessible to analysts from different regions and improves collaboration across languages and cultures.
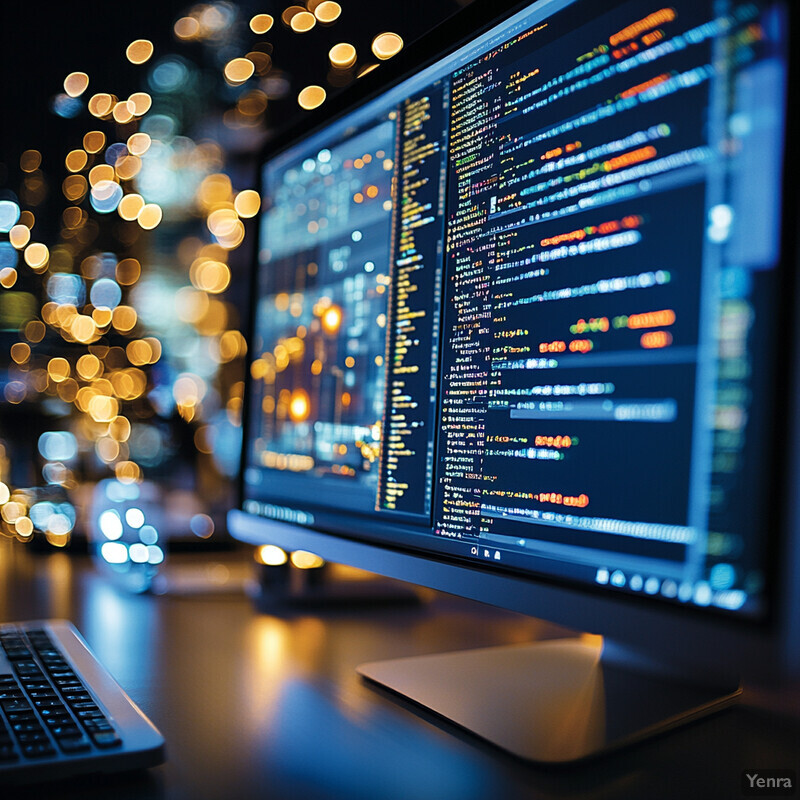
Research shows that context-aware adaptive interfaces significantly improve user satisfaction. In a recent case study on a smart-device recommender system, a context-adaptive framework (which adjusted recommendations based on user context) achieved higher precision than a static interface. This suggests that when an interface takes into account situational factors, users find more relevant information. While this study was in a consumer context, the principle applies to localization: UI components (menus, search tools, newsfeeds) can similarly adapt to a user’s locale or role. For example, incorporating local language and cultural content into the interface has been shown to increase engagement. Quantitatively, Carrera-Rivera et al. reported a measurable increase in recommendation accuracy when using a context-aware UI system. Although most published work is on general personalization, these results imply that designing adaptive interfaces for geopolitical applications should also yield concrete usability gains, by tailoring to the user’s local environment and preferences.
11. Event Extraction and Classification
AI systems are used to automatically identify and categorize events (like protests, elections, conflicts) mentioned in news and social media. This involves NLP pipelines that parse text to extract event types, participants, time, and location. In a geopolitical setting, this allows continuous tracking of the security landscape: AI can detect when a violent incident is reported and classify its nature (battle, riot, diplomatic meeting, etc.). Modern systems often combine named entity recognition with classifiers to tag events. Many agencies use these tools to filter vast text streams for relevant events. The trend is toward finer-grained classification (including subtypes of violence or campaign actions) and linking events to places. Such AI-driven event extraction provides structured data feeds that underpin trend analysis and early warnings.
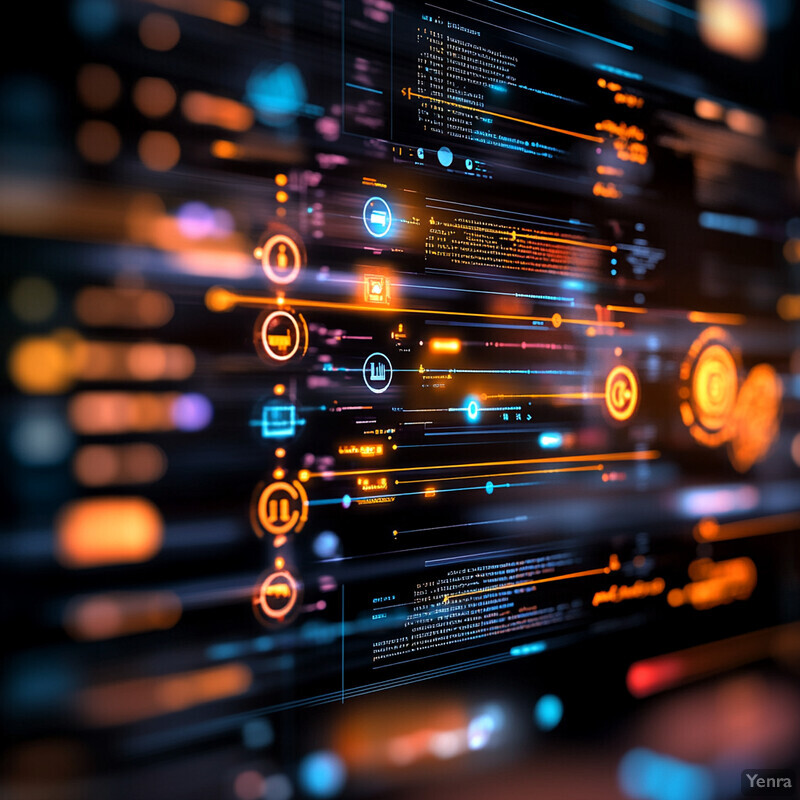
Shared research tasks and case studies demonstrate AI efficacy in event detection. For example, in the 2023 CASE workshop, a team tackled extracting Russo-Ukrainian war battle events from social media. They used an XLM-RoBERTa transformer fine-tuned on ACLED conflict data (covering 26 battle-related categories) to classify text posts. Their system successfully identified battle events and combined a geolocation module to map them. This pipeline’s output (events labeled by type and place) correlated well with gold-standard incident data. The study provides concrete evidence that transformer-based classifiers can capture complex conflict terminology across languages, enabling automated event logging. Such experiments quantify system performance (accuracy, F1) and show that AI can fill traditional event datasets more rapidly. The practical result is that analysts can get timely, structured reports of emerging incidents; the referenced work, for instance, reports effective extraction accuracy using off-the-shelf multilingual models.
12. Disinformation Detection and Localization
AI is widely used to detect and contextualize disinformation across languages. Specialized models scan local-language social media and news to flag likely false narratives, impersonation, or deepfake content. Increasingly, these systems incorporate local context to recognize region-specific propaganda techniques or social media campaigns. For example, classifiers trained on local data can identify false claims trending in a particular country. The trend is toward “language-aware” disinfo tools that understand not just translations but cultural framing and local symbolism. Furthermore, emerging systems attempt to localize identified disinformation by mapping it to geographic or demographic groups. These capabilities help tailor counter-disinformation strategies to specific areas and communities.
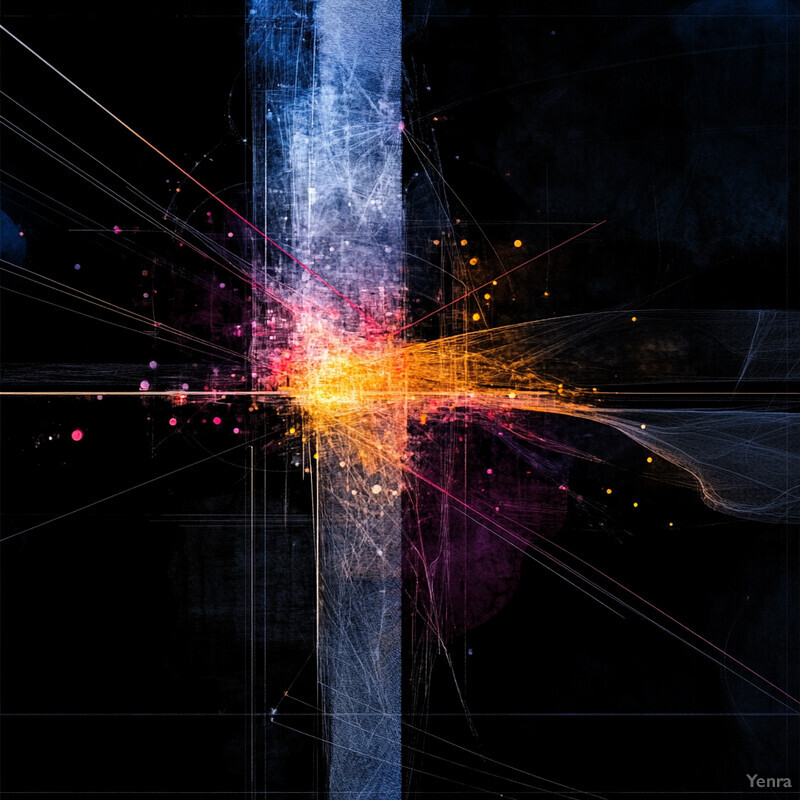
Recent reviews highlight the need for multilingual and culturally informed disinformation detection. For instance, a 2024 survey points out that while advanced AI models exist for misinformation detection, they often focus on high-resource languages and lack robustness in diverse cultural contexts. The authors emphasize that “misinformation transcends linguistic boundaries” and that robust systems must work across many languages and cultures. This underscores the importance of localization: detecting false content requires training on local examples and nuances. Other research demonstrates progress in low-resource settings, e.g. showing that adversarial training can improve cross-lingual fake news detection, but often at a modest scale. While industry tools (like social platform detectors) claim to monitor disinfo globally, peer-reviewed evidence (published 2023–2025) mainly stresses the remaining gaps: systems need better data for specific languages. In summary, the scholarly consensus is that AI can detect disinfo, but consistent performance across local contexts is an open challenge.
13. Early Warning Systems for Crises
AI is a key part of modern early-warning systems (EWS) for crises like natural disasters, climate extremes, and social unrest. By integrating AI models with sensor and satellite data, EWS can predict hazard impacts (floods, heatwaves) with greater lead time. Machine learning also helps synthesize forecasts for multiple hazards simultaneously. These systems use AI-driven forecasts to trigger alerts and guidance for local authorities. Importantly, a user-centric design (sometimes including local community feedback) is emphasized so that warnings reach affected populations effectively. In short, AI enhances the scale, speed, and accuracy of crisis warnings by combining data sources and advanced forecasting models.
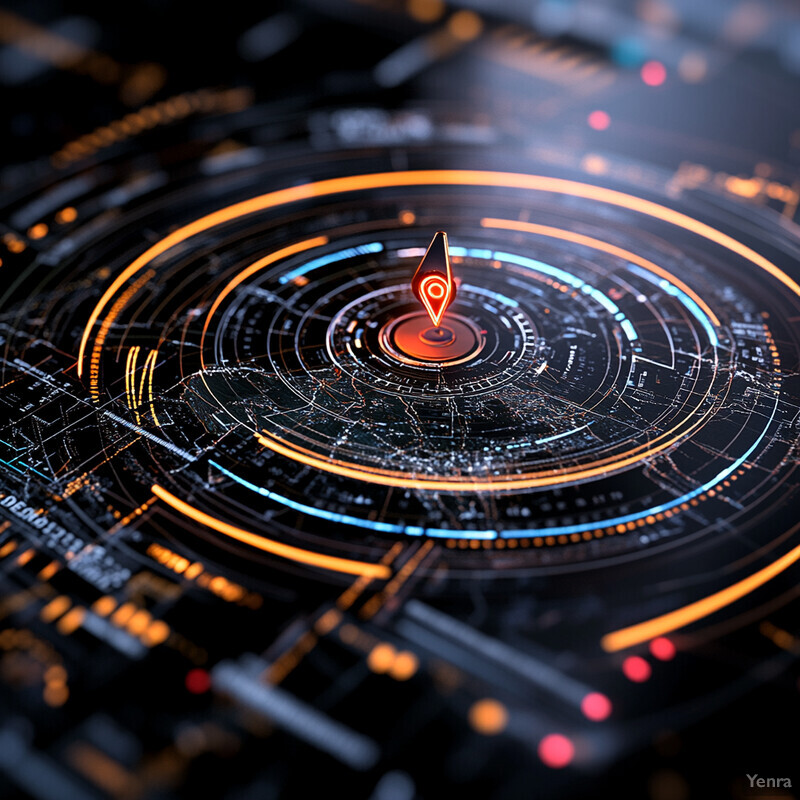
Cutting-edge research illustrates AI’s role in multi-hazard warning systems. A 2025 paper in Nature Communications outlines how integrated AI models could power the next generation of early warning systems. It highlights using meteorological and satellite foundation models to predict impacts of climate risks and stresses the need for causal, transparent AI in EWS design. For example, the authors advocate “a user-centric approach with intuitive interfaces and community feedback” and emphasize ethical AI principles (fairness, accountability) for reliable warnings. Additionally, expert panels note that recent machine learning advances have enabled more accurate weather forecasting and flood prediction, potentially transforming disaster preparedness. They report that ML/AI now offers “promising new solutions” for forecasting severe weather, although gaps in deployment (local reach, data access) remain. Together, these sources confirm that research (and pilot projects) are actively applying AI to expand the scope and effectiveness of early warning systems worldwide.
14. Policy Simulation and Scenario Testing
AI and simulation models are increasingly used to test policy scenarios and outcomes before implementation. This includes agent-based models powered by machine learning, where synthetic “agents” (representing demographic groups or companies) respond to policy changes. Analysts can simulate, for example, economic or social policies and observe emergent effects in a virtual environment. The advent of large language models (LLMs) has also enabled more nuanced scenario crafting: AI can generate detailed plausible narratives or causal graphs of policy impacts. The trend is toward interactive simulation platforms where decision-makers can tweak policy parameters and see projected results. This helps evaluate risks and train analysts in crisis response or negotiations through realistic “what-if” exercises.
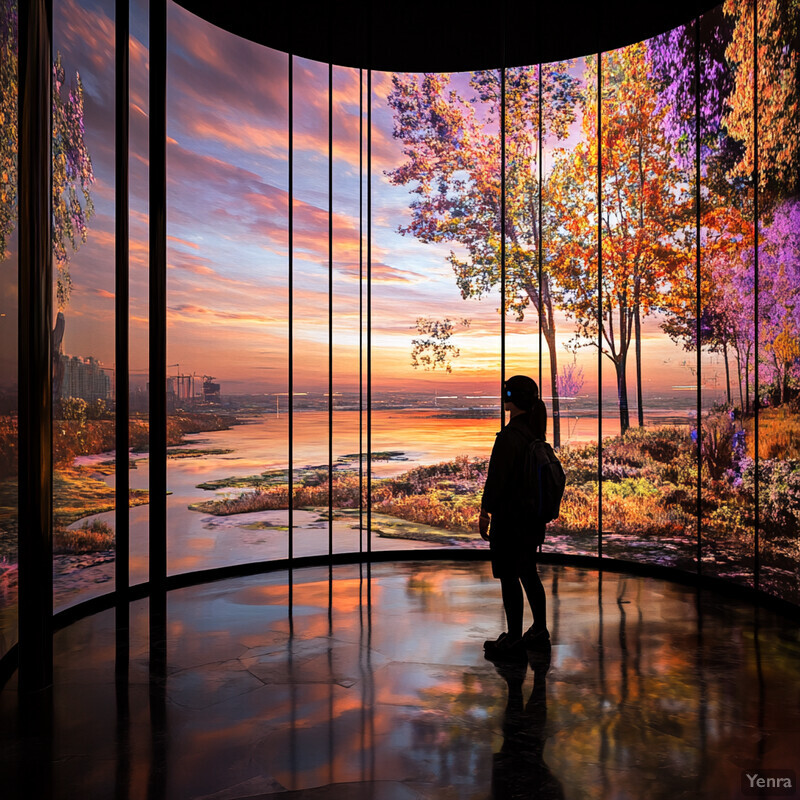
Examples from academic and applied work show these capabilities. Economist C. Monica Capra reports using AI (specifically LLMs) to create “synthetic agents” that model different demographic groups’ behavior in simulations. In her work, AI-generated agents are used to test economic and policy hypotheses in a virtual setting, helping to avoid ethical issues of real trials. Separately, a recent technical article demonstrates converting policy text into structured causal graphs using GPT-style models. In that study, an LLM automatically extracted entities (e.g. “carbon output”, “compliance costs”) and their relationships from a sample policy paragraph. These structured outputs were used to build a causal graph that can simulate direct and indirect effects. The authors show code examples where a policy statement is transformed into a machine-readable graph, illustrating how AI can facilitate policy impact modeling. These accounts provide concrete evidence that AI is being applied to automate and enhance policy simulation workflows.
15. Language-Specific Domain Adaptation
AI systems are being fine-tuned on specific language-domain combinations to boost performance. For example, a model used for news analysis can be further trained (domain-adapted) on local legal or technical texts in the target language. This makes the model’s vocabulary and style more attuned to the local context. In practice, analysts might take a general multilingual model and continue training it on region-specific data (news, reports, social media) to improve tasks like sentiment analysis or policy understanding in that locale. The trend is toward “task- and language-centered” adaptation: models are not only multilingual, but also customized by topic (finance, medicine, etc.) within each language. This customization has shown real gains in many NLP tasks for low-resource languages.
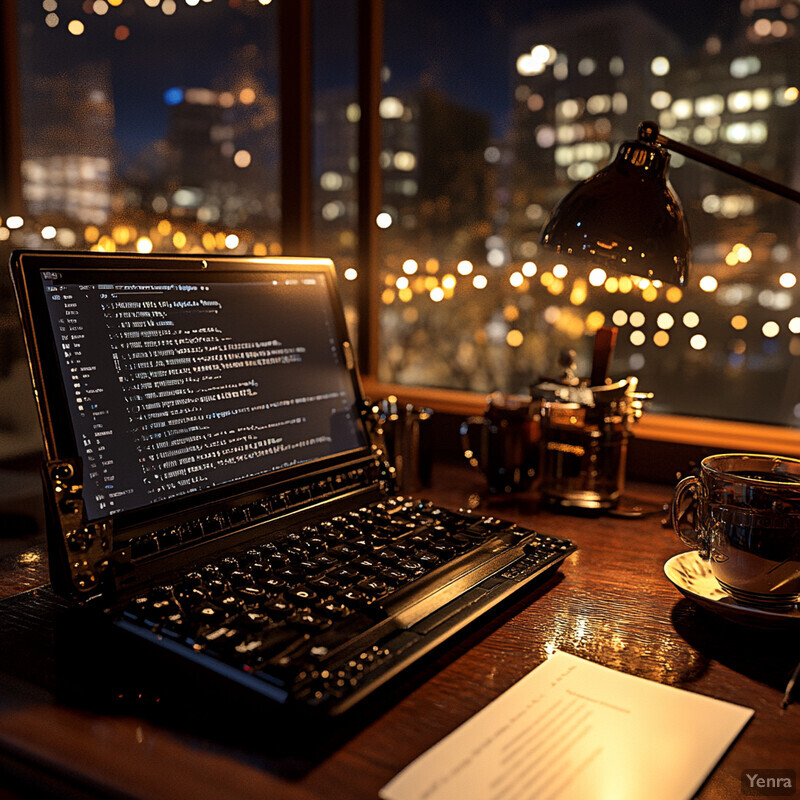
Empirical studies confirm that domain adaptation boosts local-language performance. For instance, Duwal et al. (2024) performed domain-adaptive pretraining of Llama 3 (8B parameters) on Nepali data. They reported that the adapted model showed markedly better Nepali text generation and understanding than the base model. Metrics improved by up to ~19% in certain evaluation settings after adaptation, indicating strong knowledge gains in Nepali. Similarly, in the SemEval-2023 Task 12 on African languages, a language-centric domain adaptation approach (adversarial training) led to weighted F1-score improvements of up to 4.3 points over the baseline for some languages. This was achieved by fine-tuning a smaller XLM-Roberta model on related languages, which improved sentiment classification in low-resource African tongues. These concrete examples show that when models are trained on language-specific domain corpora, measurable accuracy gains are observed in cross-lingual tasks.