1. Dynamic Route Optimization
AI-powered systems continuously adjust delivery routes in real-time based on current traffic conditions, weather, and road closures.
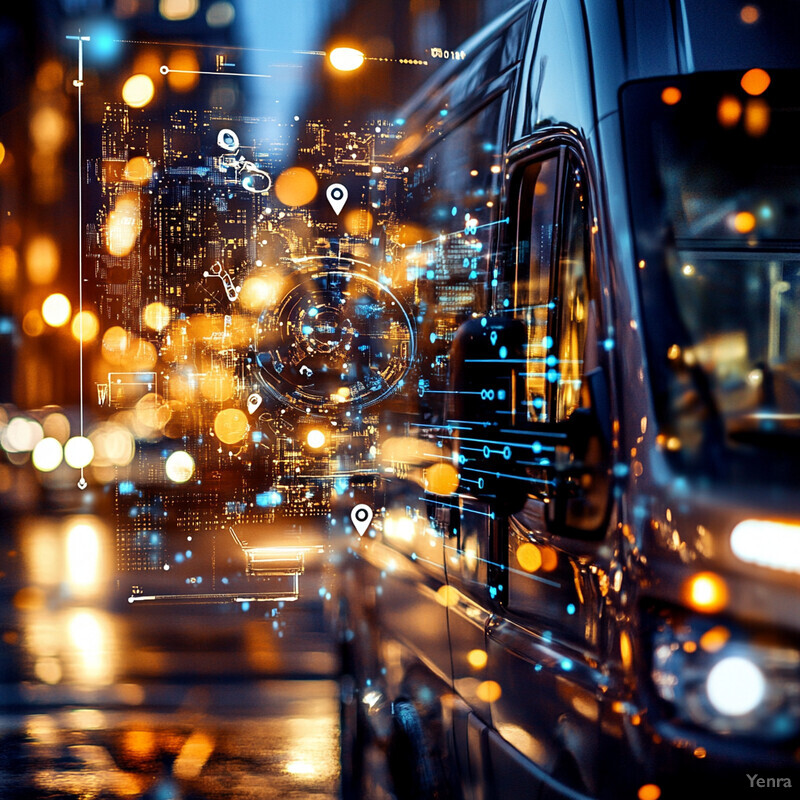
Traditional delivery routing systems often rely on static route plans established at the start of the day. AI-driven dynamic route optimization solutions, however, operate continuously and react adaptively to the ever-changing conditions in mega-cities. By processing streams of real-time data—such as traffic congestion levels, street closures, and updated delivery requests—these systems continually refine their routing decisions. The result is a significant reduction in travel time, decreased fuel consumption, and more on-time deliveries. This capability also allows fleets to better handle disruptions like vehicle breakdowns or sudden spikes in orders, ultimately boosting efficiency and customer satisfaction.
2. Predictive Traffic Modeling
Advanced machine learning models forecast traffic congestion levels, helping delivery fleets avoid bottlenecks before they occur.
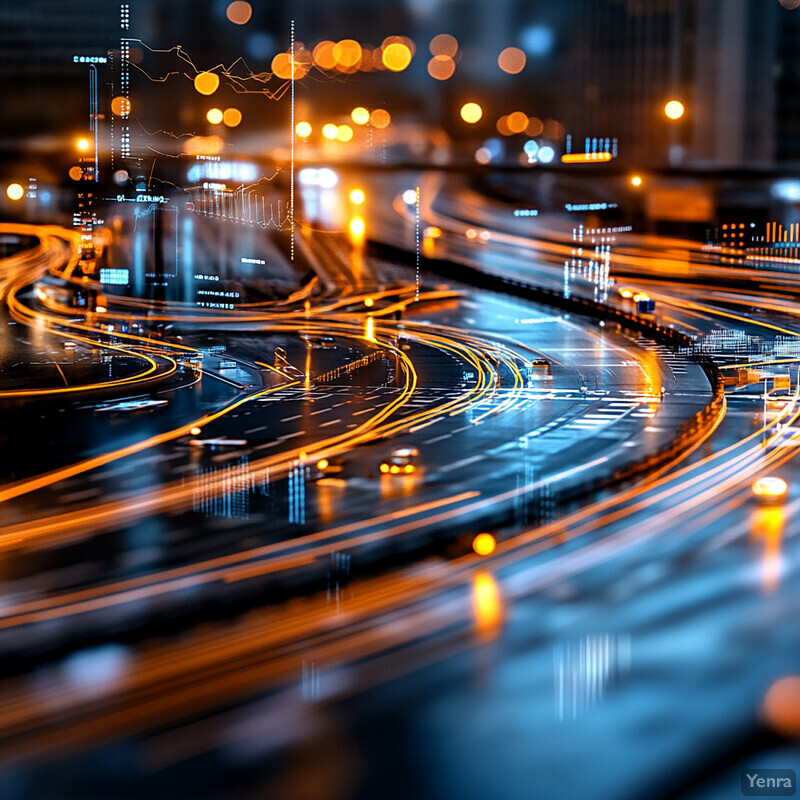
AI-powered predictive traffic modeling tools combine historical traffic patterns with live sensor data from road networks, public transportation, and city infrastructure. Machine learning models identify emerging trends in vehicle flow and congestion, enabling companies to predict where and when bottlenecks will form. By anticipating these slowdowns, delivery managers can preemptively adjust driver routes and timings. The advantage is twofold: vehicles spend less time idling in gridlocks, and customers receive their orders sooner. Over time, these predictive insights also guide strategic decisions on where to allocate resources and how to structure delivery shifts.
3. Adaptive Fleet Management
AI algorithms autonomously assign deliveries to the most suitable vehicle and driver, balancing workload and capacity across the fleet to increase overall efficiency.
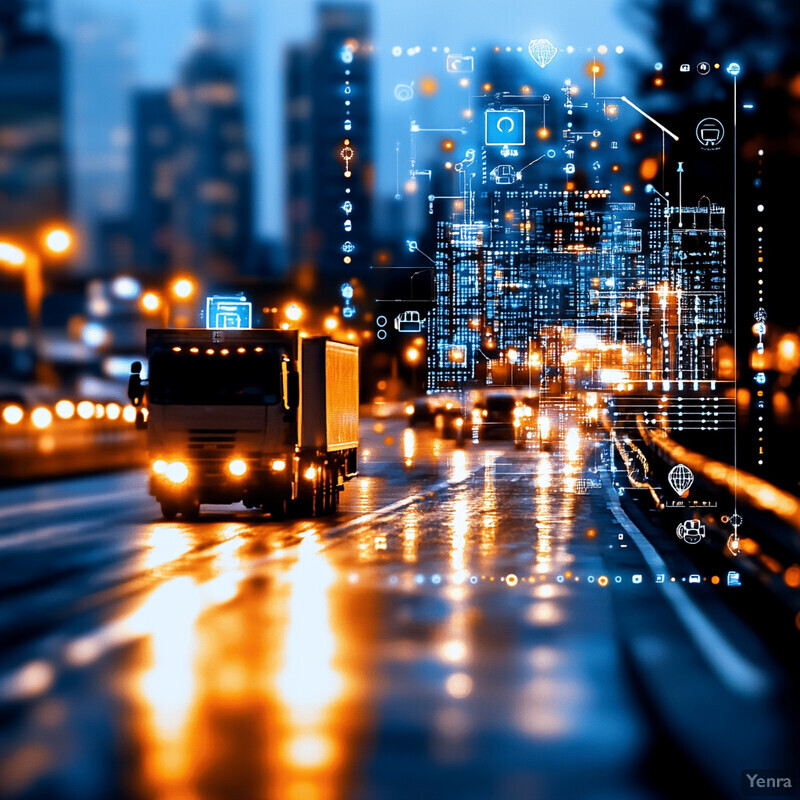
As order volumes rise and fall throughout the day, traditional static assignment of drivers and vehicles becomes inefficient. AI-driven adaptive fleet management systems optimize resource distribution dynamically, assigning the most suitable driver and vehicle to each new request based on factors like proximity, load capacity, and driver skill. This ensures each delivery is completed by the most effective resource at the best possible time. Beyond immediate gains in speed and cost efficiency, these solutions also provide long-term benefits: balanced workloads reduce driver fatigue, maintain consistent service quality, and help manage peak demand periods gracefully.
4. Demand Forecasting
Predictive analytics estimate order volume and peak demand times, enabling companies to pre-position vehicles in high-demand zones.
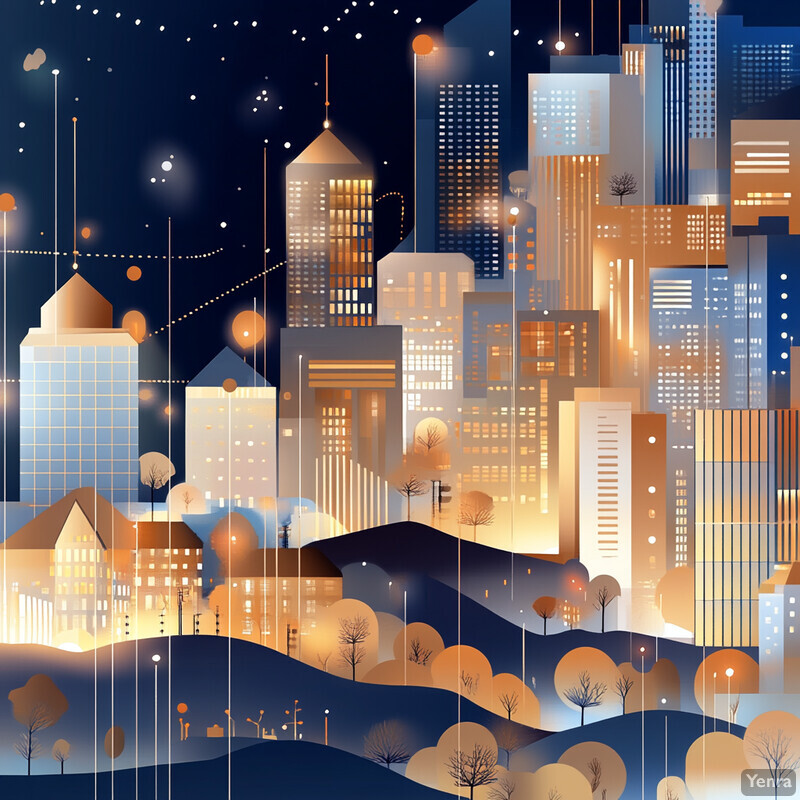
In dense urban areas, predicting demand accurately is a fundamental advantage. Machine learning models ingest large datasets—historical sales patterns, seasonal trends, event calendars, weather forecasts, and socioeconomic factors—to forecast future order volumes. Armed with these forecasts, delivery companies can proactively position their fleets in neighborhoods likely to see higher-than-usual demand, minimizing travel distances and maximizing vehicle utilization. This proactive approach ensures that vehicles and couriers are ready where they are needed, improving last-mile efficiency and enhancing customer satisfaction.
5. Geospatial Analysis
AI-driven geospatial intelligence identifies optimal delivery paths through complex urban landscapes, including congestion hotspots, pedestrian zones, and one-way streets.
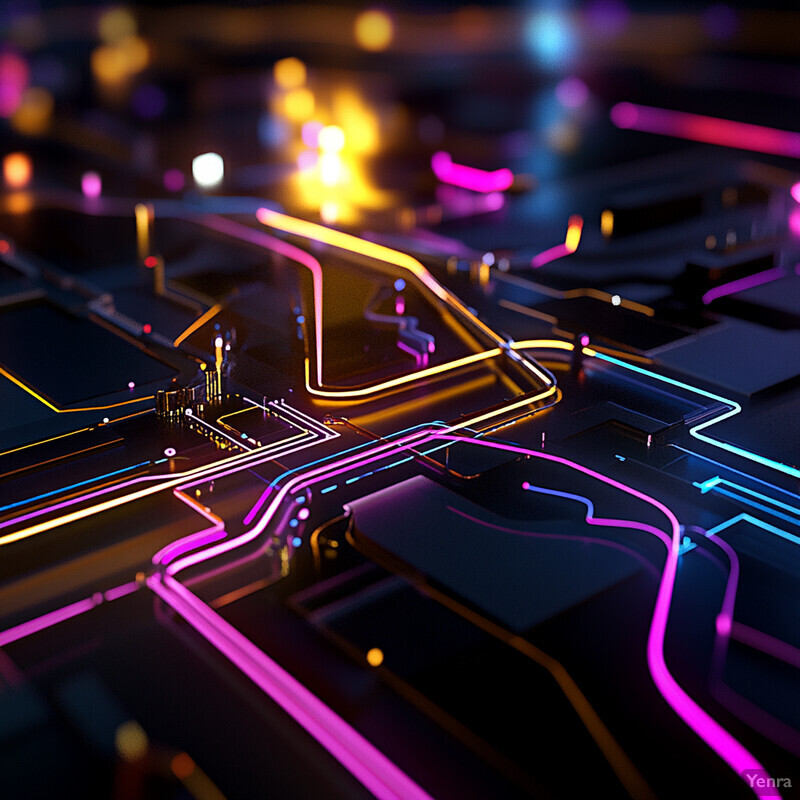
Mega-cities present a complex tapestry of road layouts, traffic rules, and delivery constraints. AI-based geospatial analysis systems integrate high-resolution maps, satellite imagery, and municipal data sets to make sense of this complexity. By identifying efficient paths that minimize left turns, avoid restricted streets, and navigate safely around major thoroughfares, these tools cut down on travel time and errors. The intelligence generated from geospatial analysis also informs decisions on vehicle types, payload distributions, and possible areas to establish micro-hubs or pickup points, contributing to a more holistic and responsive last-mile logistics ecosystem.
6. Contextual Delivery Windows
Machine learning can analyze historical data—like recipient availability or preferred delivery times—to schedule stops when customers are most likely to receive packages.
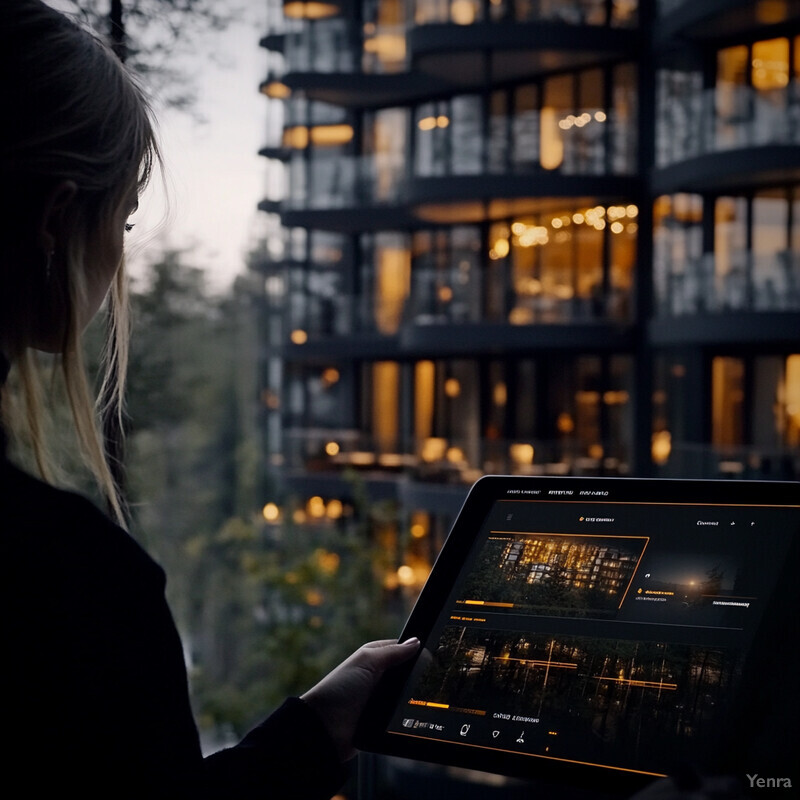
Customers’ availability varies widely across a city, with factors such as work schedules, building security protocols, and personal routines influencing when they can receive packages. AI solutions mine past delivery data and customer feedback to learn these patterns. This insight allows companies to schedule deliveries for when a recipient is most likely home or at a pickup point. Not only does this reduce the number of failed delivery attempts, but it also streamlines the route by cutting out unnecessary revisits. Ultimately, both the delivery service and the customer benefit from more convenient and predictable interactions.
7. Real-Time Incident Detection
Using computer vision, sensors, and connected infrastructure data, AI identifies on-the-ground issues (accidents, construction) and proactively reroutes drivers.
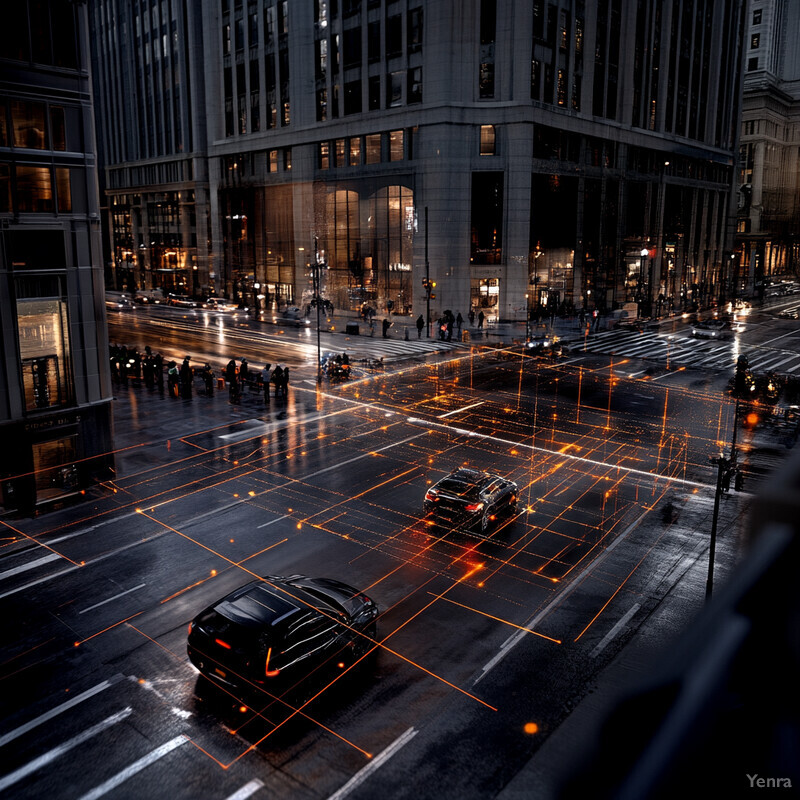
Traffic accidents, construction projects, and sudden road closures can create unexpected delays and route disruptions. AI systems employ computer vision from cameras, real-time feeds from municipal authorities, and IoT sensor data to detect such incidents as they happen. By quickly processing and analyzing these signals, the system alerts dispatchers and triggers automated rerouting protocols. This agility ensures that delivery vehicles can skirt around emerging issues, maintaining tight delivery schedules and minimizing frustration for both drivers and customers.
8. Micro-Depot Optimization
AI helps companies determine where to place mini-distribution centers or lockers closer to customers, shortening final delivery times.
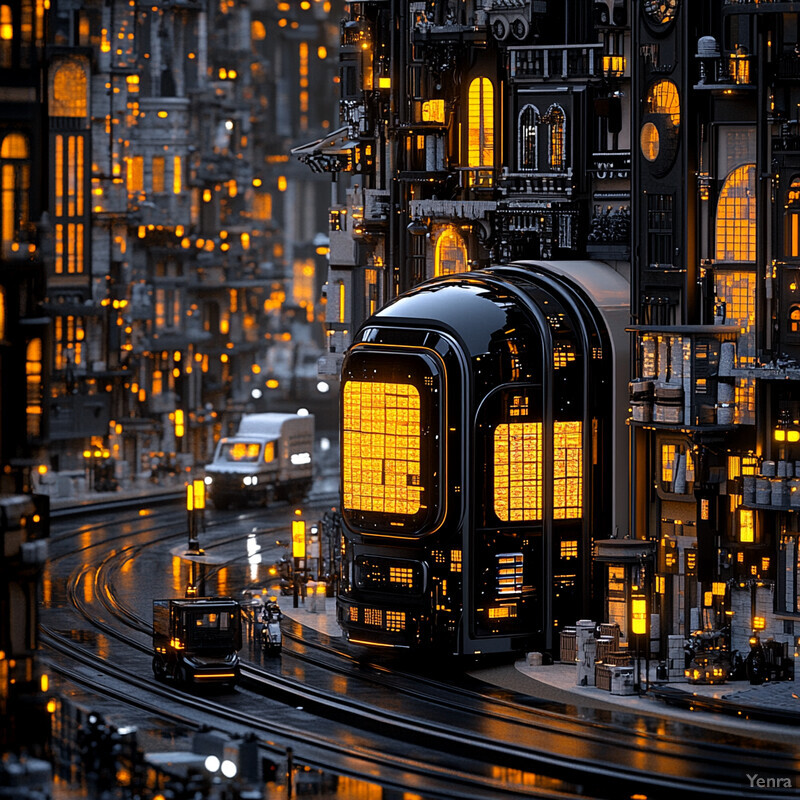
In sprawling urban landscapes, establishing strategically placed micro-depots or locker hubs is essential for efficient last-mile delivery. AI algorithms model urban density, property costs, travel times, and customer order patterns to identify where these small-scale distribution centers should be located. Proper placement of micro-depots reduces the distance drivers must cover to reach customers, shortens delivery times, and can decrease operational costs. Additionally, the presence of micro-depots helps companies respond more quickly to fluctuations in demand, as restocking or retrieving packages becomes less reliant on distant, centralized warehouses.
9. Energy Efficiency in EV Fleets
AI manages electric vehicle (EV) fleet routing by accounting for battery levels, charging station availability, and optimizing routes that minimize energy consumption.
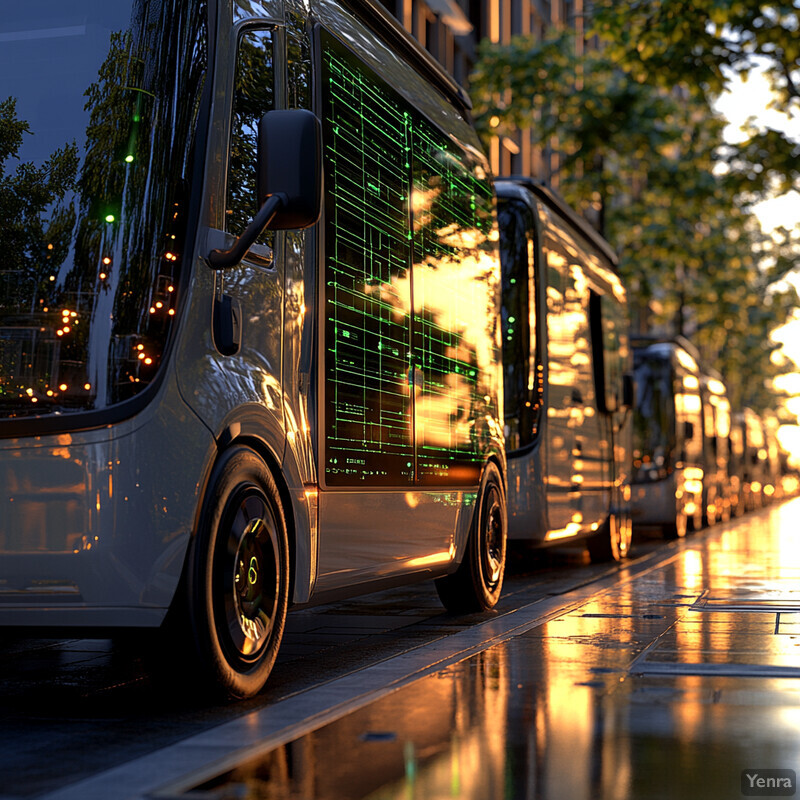
With growing environmental concerns, many delivery companies are transitioning to electric vehicles (EVs). AI-driven route optimization for EV fleets involves careful consideration of battery range, charging station locations, and trip lengths. Advanced algorithms ensure that EVs follow routes that enable them to complete deliveries without running out of charge, factoring in where and when charging stops are most cost-effective. This approach not only cuts down on operational costs but also supports companies’ broader sustainability goals, helping them meet regulatory emissions standards and appeal to eco-conscious customers.
10. Sustainability Integration
Algorithms factor in emission levels and select greener routes, aligning last-mile delivery strategies with sustainability goals.
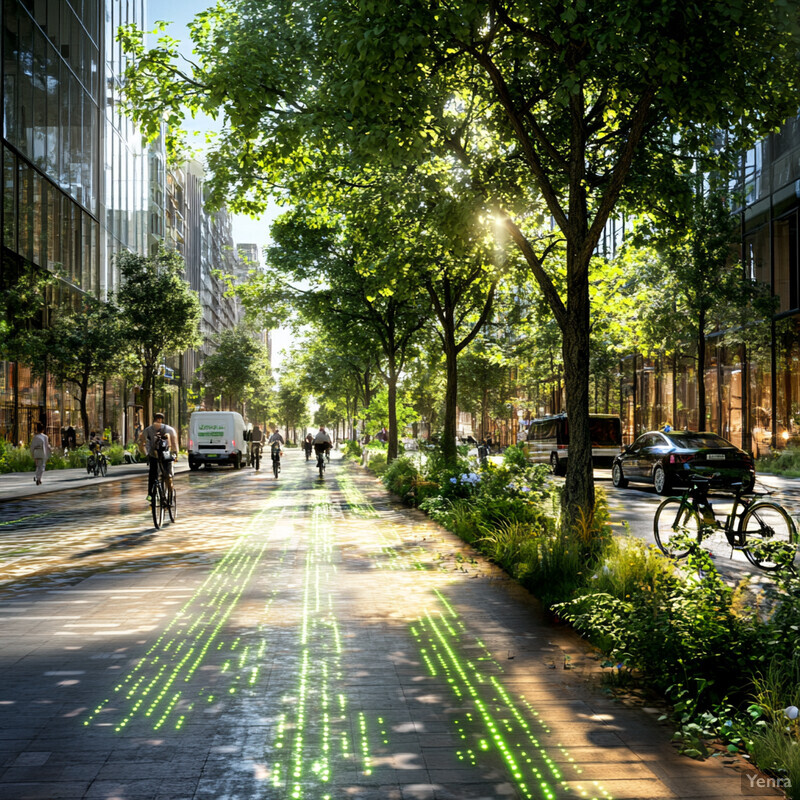
As megacities strive to reduce carbon footprints and promote cleaner air, last-mile delivery networks must adapt. AI tools that integrate sustainability metrics into routing decisions analyze factors such as fuel consumption, emissions per mile, and idle times. By selecting routes that produce fewer emissions or incorporating alternative delivery modes (like bikes or electric scooters), companies show environmental responsibility. Over time, these greener approaches become standard practice, aligning logistics operations with the city’s environmental objectives and creating a positive brand image for companies that prioritize sustainable transportation.
11. Machine Learning-Driven Vehicle Maintenance Scheduling
By predicting vehicle downtime, AI ensures that maintenance is done during off-peak hours, reducing route disruptions.
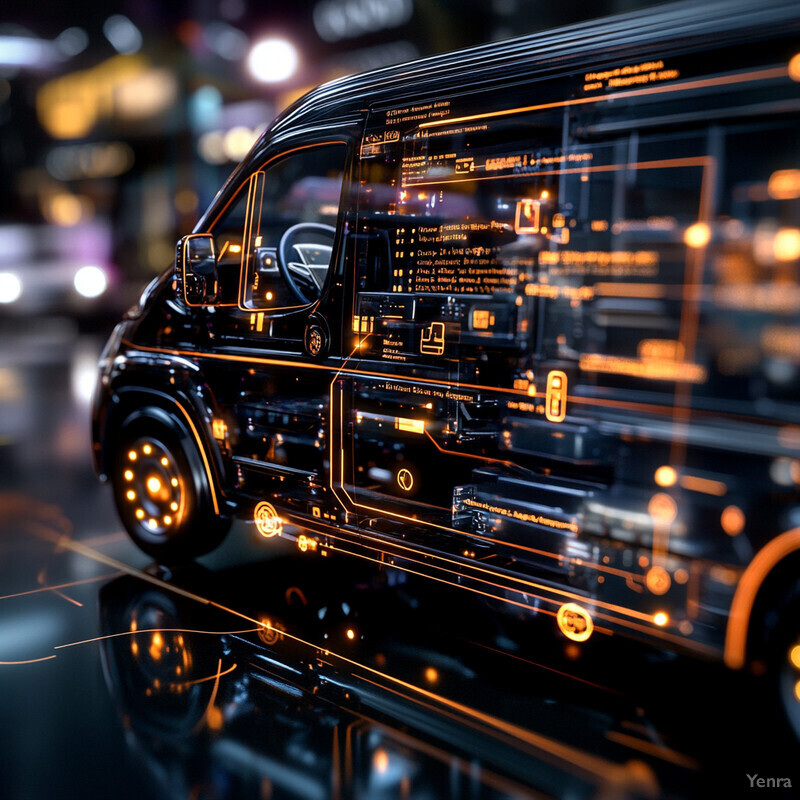
Unscheduled maintenance and breakdowns can derail delivery schedules, causing cascading delays. AI-based predictive maintenance models analyze telemetry data, historical maintenance records, and driving conditions to forecast when a vehicle is likely to need servicing. By scheduling maintenance during off-peak hours or integrating it seamlessly into the fleet’s route planning, companies minimize disruptions. This foresight reduces repair costs, extends vehicle lifespans, and ensures that the delivery network remains robust even during periods of high demand.
12. Personalized Customer Alerts
Intelligent systems predict delivery arrival times more accurately and inform customers well in advance, leading to fewer failed deliveries and more efficient redelivery planning.
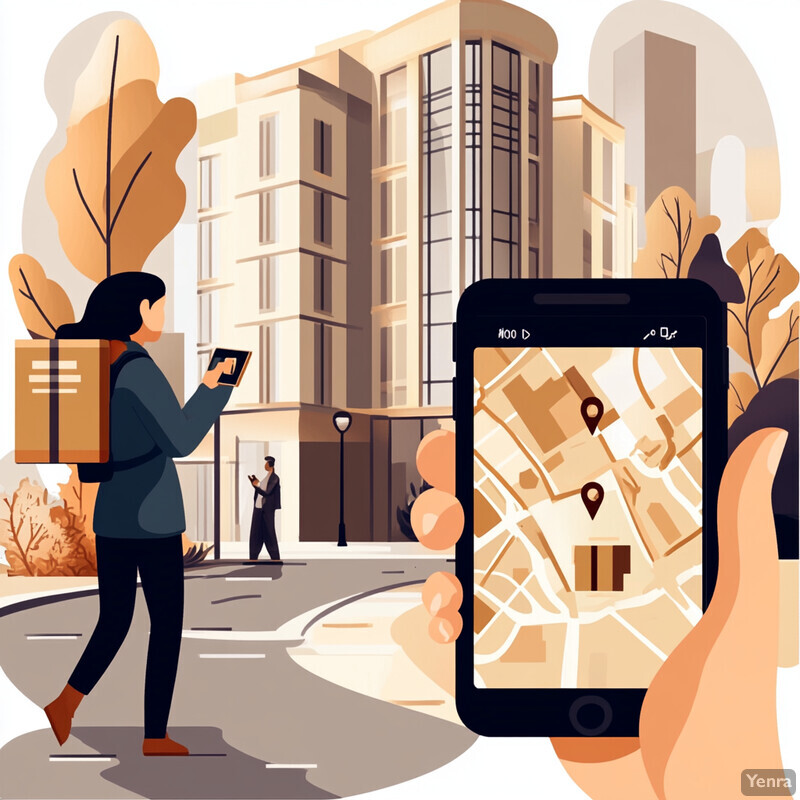
In busy urban environments, customers value transparency and convenience. AI-based systems use predictive analytics to determine precise estimated times of arrival (ETAs) for packages and send proactive notifications. Customers can track their deliveries in real time, adjust their schedules, or provide instructions if they’re unexpectedly unavailable. These personalized alerts reduce the number of failed delivery attempts and unnecessary follow-up trips. Enhancing communication between delivery services and recipients leads to improved trust, satisfaction, and customer retention.
13. Robust Scalability
As megacities grow and delivery volumes surge, AI-based solutions can scale operations without loss in routing efficiency, ensuring consistent on-time performance.
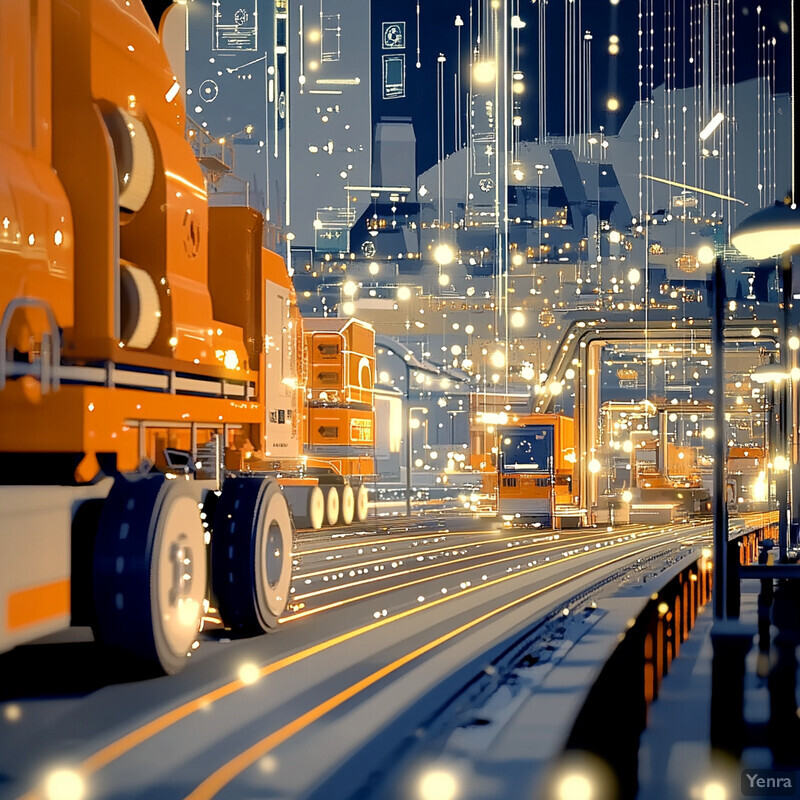
Megacities are dynamic entities where populations and order volumes can skyrocket due to economic growth, special events, or seasonal demand. AI-driven routing systems are inherently scalable, as machine learning models improve as they consume more data. Instead of route planning and fleet management becoming more chaotic with increased volume, these systems adapt and maintain efficiency. By distributing workload intelligently and optimizing resources, they ensure that service quality remains consistent even as the scale of operations grows exponentially.
14. Multi-Modal Routing
Algorithms integrate multiple transportation modes (cargo bikes, drones, vans) into unified route planning, selecting the best vehicle type for each segment of a delivery.
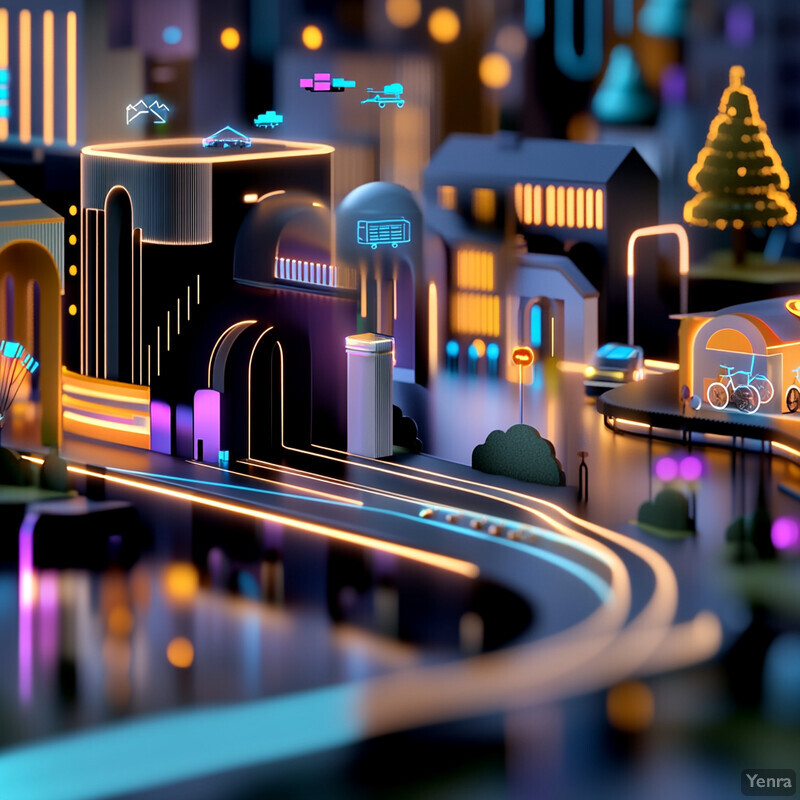
Last-mile delivery need not be limited to a single mode of transportation. AI solutions help companies integrate vans, bikes, drones, robots, and even public transit options into a unified routing plan. The system chooses the best transport mode for each segment, factoring in speed, cost, and environmental constraints. By blending various modes, companies can navigate crowded streets more efficiently, deliver to areas inaccessible by traditional trucks, and even use autonomous shuttles for bulk transfers. This flexibility expands delivery capabilities and reduces dependency on any single transportation method.
15. Precision ETAs
Machine learning refines estimated times of arrival (ETAs) through continuous feedback loops, improving accuracy and customer satisfaction.
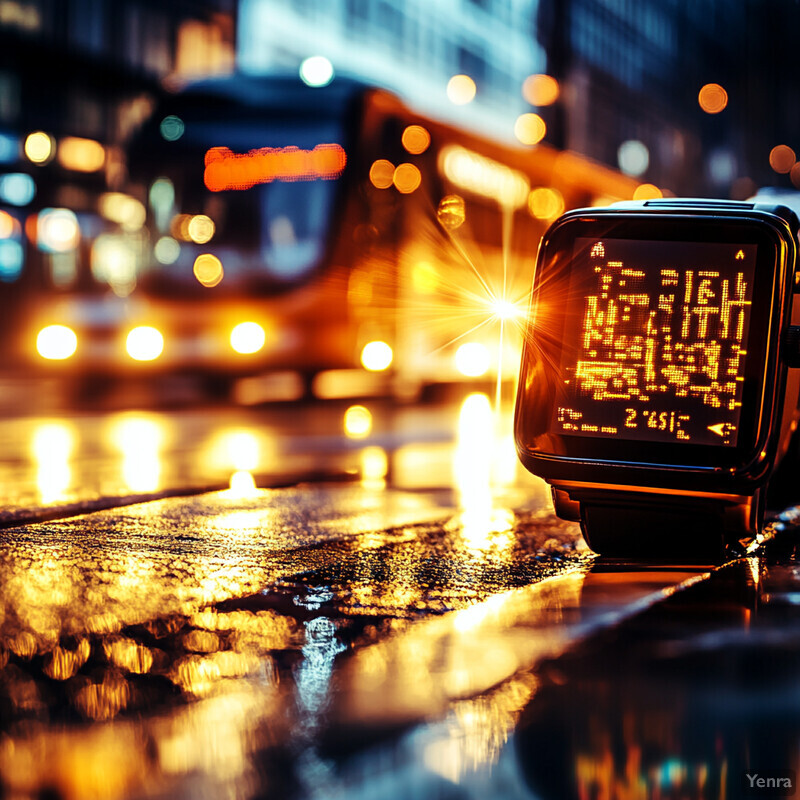
Accurate estimated times of arrival are essential for building customer trust and managing expectations. AI-based solutions refine ETAs over time by analyzing historical delivery data, driver performance, and evolving traffic patterns. These systems incorporate feedback loops to learn from discrepancies between predicted and actual times, steadily improving the accuracy of their estimates. Enhanced precision helps ensure that customers know exactly when to expect their packages, reducing anxiety and improving the overall experience. In turn, more reliable ETAs lower the operational costs related to redeliveries and support services.
16. On-Demand Crowdsourced Delivery
AI can incorporate gig-economy couriers into routing plans, deciding when and where to deploy flexible crowd-delivery options.
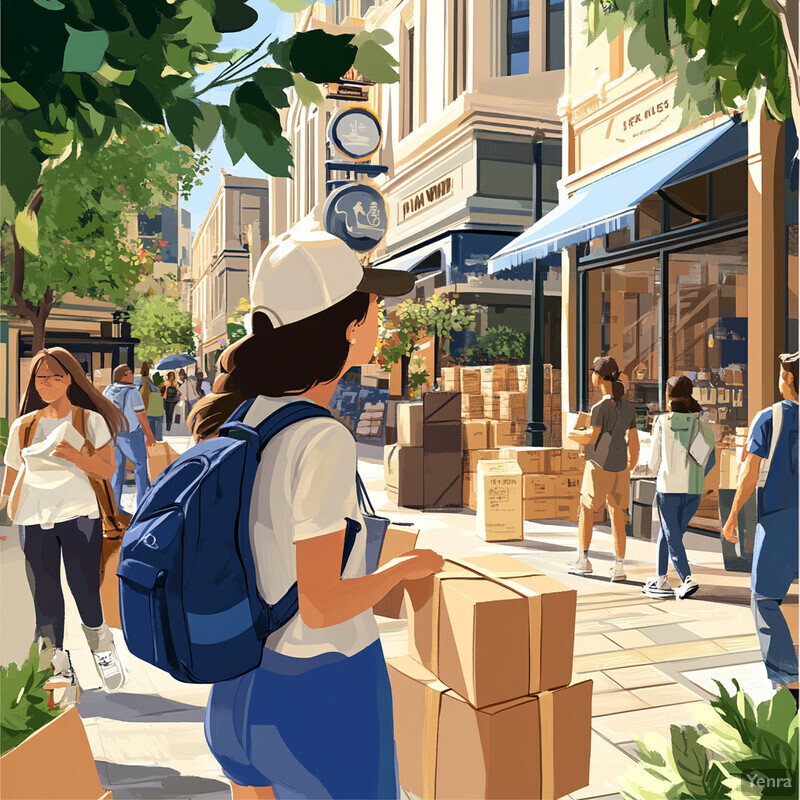
In fast-paced urban economies, crowdshipping—engaging freelance couriers who work on-demand—is increasingly common. AI automates the integration of these gig-economy workers into established delivery networks. The system identifies when it’s beneficial to involve crowdsourced couriers based on current demand, driver availability, and order urgency. It then dynamically assigns tasks, ensuring that both full-time drivers and freelancers operate at peak efficiency. This fluid workforce model provides scalability, reduces overhead, and enables companies to meet surge demand quickly without long-term commitments.
17. Intelligent Load Balancing
By predicting package density and weight distribution, AI optimizes parcel loading to maintain balanced, fuel-efficient routes.
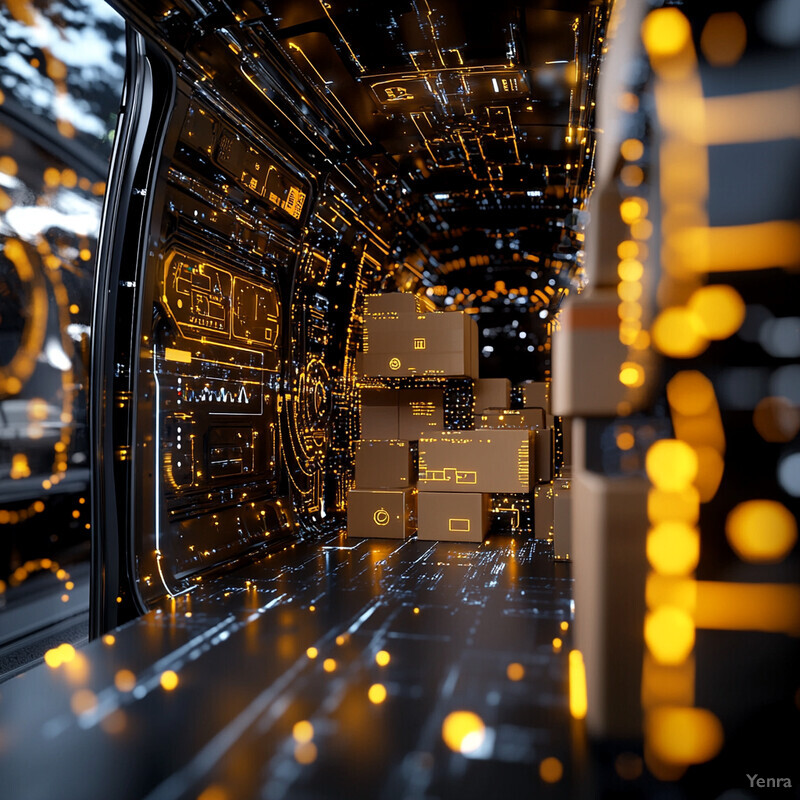
Ensuring each delivery vehicle operates at optimal capacity is a complex task. AI-driven load balancing systems analyze package sizes, weights, and delivery destinations to allocate them efficiently across the fleet. Balancing loads reduces fuel consumption, maintenance costs, and transit times. By keeping each vehicle operating near its ideal capacity, companies can accomplish more deliveries in fewer trips, thereby reducing congestion and emissions. This optimization also simplifies warehouse operations, as loading teams can rely on the system’s guidance to streamline the preparation of outgoing shipments.
18. Risk Assessment Modeling
Advanced models evaluate route risks (high-theft areas, complex security regulations) and adjust routing to ensure safer deliveries.
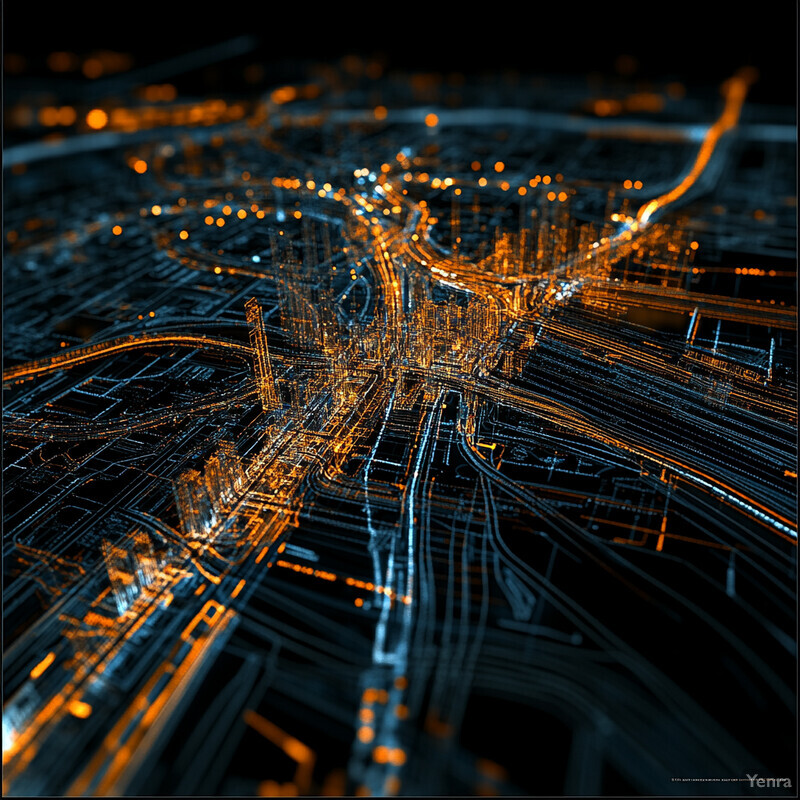
Urban deliveries sometimes cross areas known for higher theft rates, regulatory restrictions, or other security challenges. AI-based risk assessment models analyze crime statistics, environmental conditions, social data, and real-time alerts from local authorities. In doing so, they help companies identify routes that minimize exposure to such risks. By advising drivers to take safer, lower-risk paths—sometimes even when slightly longer—these systems protect cargo, reduce insurance claims, and enhance the security of the entire logistics operation. Safer deliveries also enhance the brand reputation and confidence of both customers and drivers.
19. Behavioral Driver Analytics
AI-based insights guide driver training and incentive programs that improve navigation decisions, leading to smoother and safer last-mile journeys.
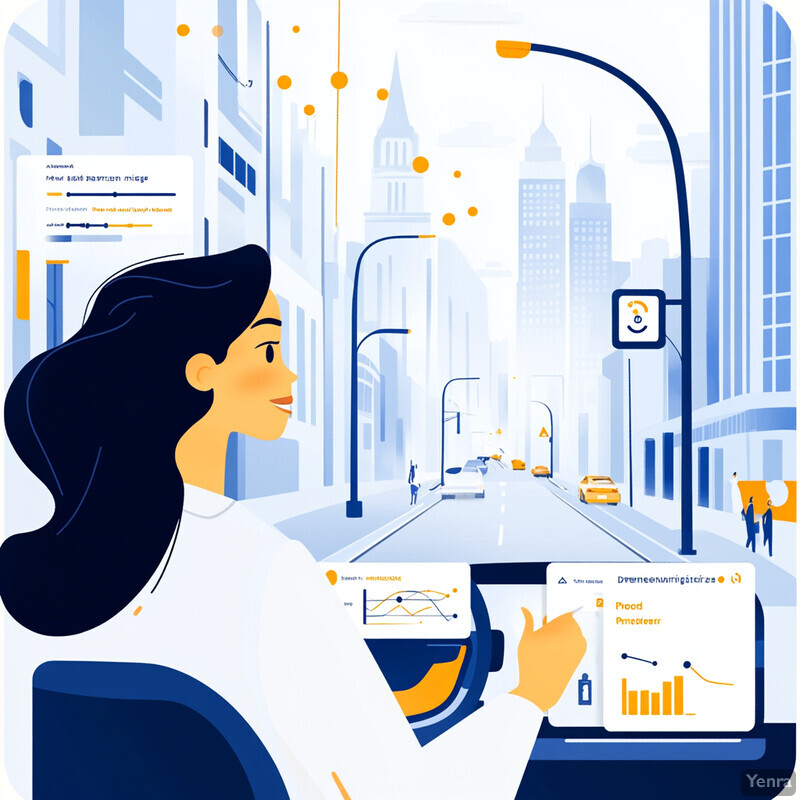
Driver performance and decision-making on the road significantly impact delivery times, safety, and costs. AI-driven analytics track driver behavior—speeding, hard braking, route deviations—and correlate these with delivery outcomes. Over time, this insight helps companies provide targeted training, guidance, or incentives that improve driver performance. Better driving translates into more reliable schedules, reduced accident risks, and improved fuel efficiency. Ultimately, investing in driver analytics elevates the overall quality of the last-mile delivery experience and ensures that human resources are used effectively.
20. Integration with Smart City Infrastructure
By communicating with connected traffic lights, smart parking systems, and urban sensors, AI helps delivery vehicles pass through cities more fluidly, reducing stops and idle time.
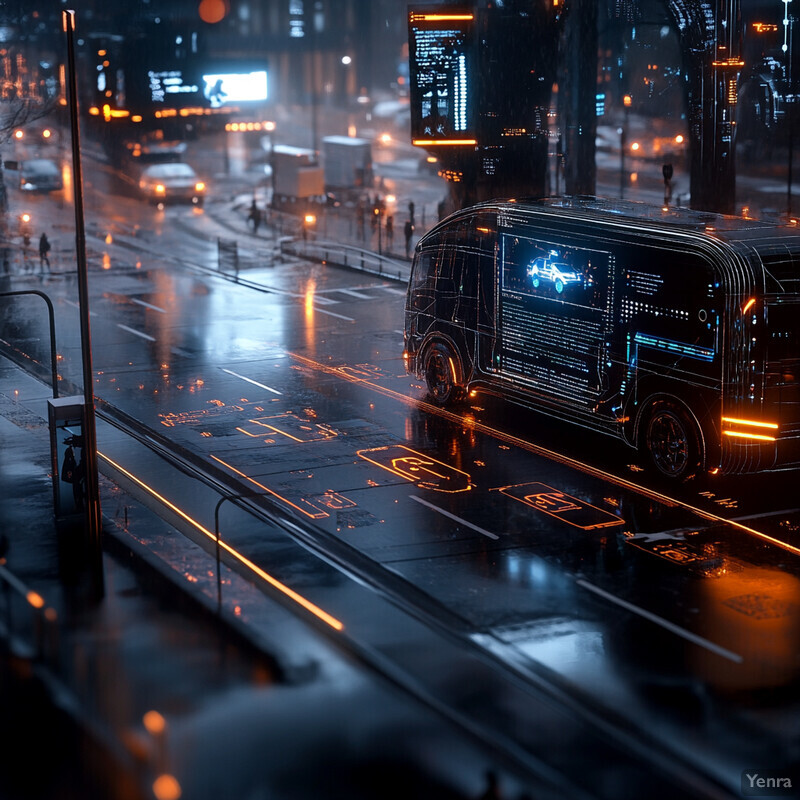
As cities become “smarter,” they deploy connected traffic signals, intelligent parking systems, and extensive IoT sensor networks. AI-powered delivery routing platforms tap into this infrastructure to anticipate and respond to evolving conditions. For example, data from connected traffic lights can help dispatchers time departures or choose routes with synchronized green waves. Smart parking data allows for quicker drop-offs and pickups. By aligning delivery operations with the city’s intelligent systems, companies can reduce idle times, avoid delays, and better integrate into the urban mobility ecosystem, creating a harmonious flow of goods and services across the megacity.