1. Automated Claim Detection
AI models can automatically identify and extract claims from text, audio, or video content, helping fact-checkers pinpoint which statements need verification.
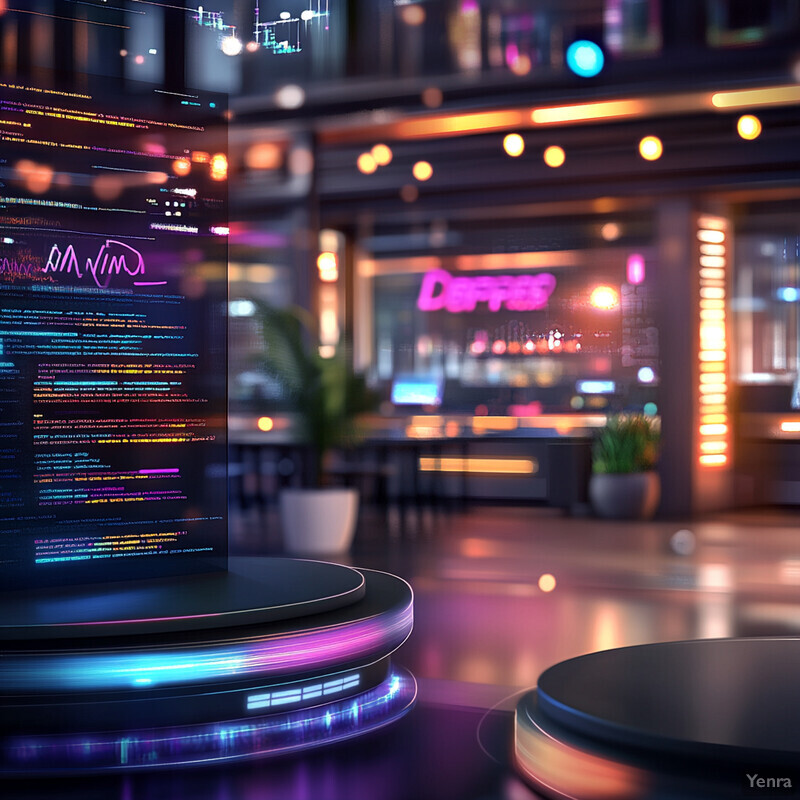
AI-driven claim detection tools use machine learning and natural language processing (NLP) algorithms to sift through large volumes of text, audio transcripts, and video subtitles to identify specific claims that might require verification. By analyzing sentence structure, linguistic cues, and contextual markers, these systems can distinguish between factual statements, opinions, and rhetorical questions. As a result, journalists no longer need to manually comb through content to find claims of interest; the AI does the initial sorting, enabling fact-checkers to focus their time and energy on evaluating the truthfulness of each claim rather than locating them in the first place.
2. Natural Language Processing (NLP) for Contextual Understanding
Advanced NLP techniques allow AI systems to understand the context and nuances of a claim, making it easier to determine whether it’s factual or misleading.
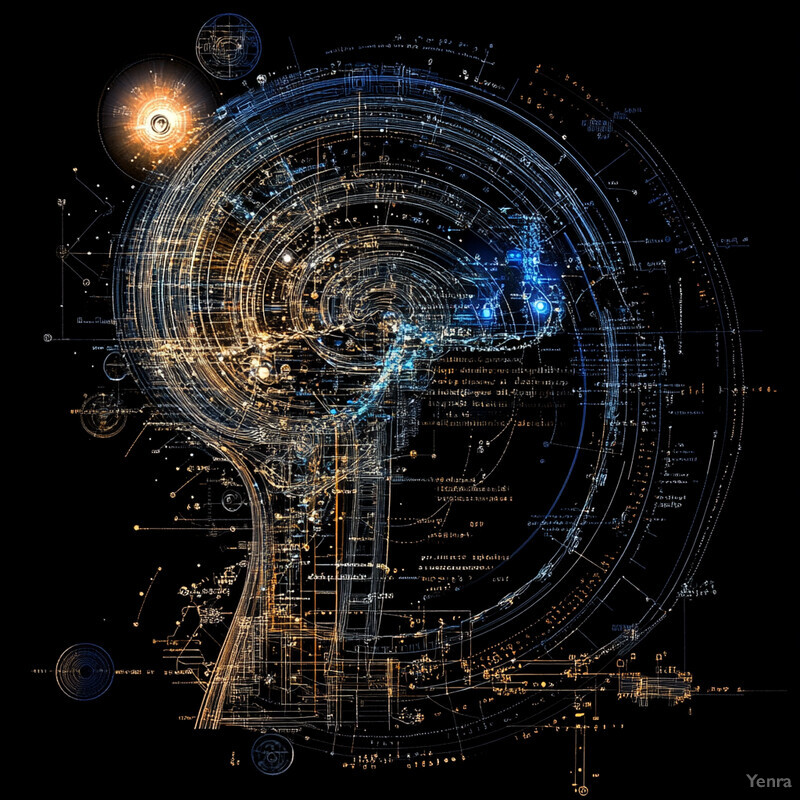
Advanced NLP models can interpret a claim’s meaning by examining syntax, semantics, and pragmatics. Beyond merely recognizing keywords, these models understand the broader discourse context, references to people and events, and the subtle differences between similar statements. This deep comprehension allows NLP systems to discern when a claim is expressed indirectly, sarcastically, or with cultural nuance. As a result, the AI can more accurately assess what exactly is being asserted, reducing the likelihood of misunderstandings and ensuring that human fact-checkers are equipped with precise insights into the nature and intent of the statements they examine.
3. Real-Time Fact-Checking Suggestions
AI-driven tools can provide live suggestions or alerts to journalists as they write or broadcast content, reducing the time needed to verify claims.
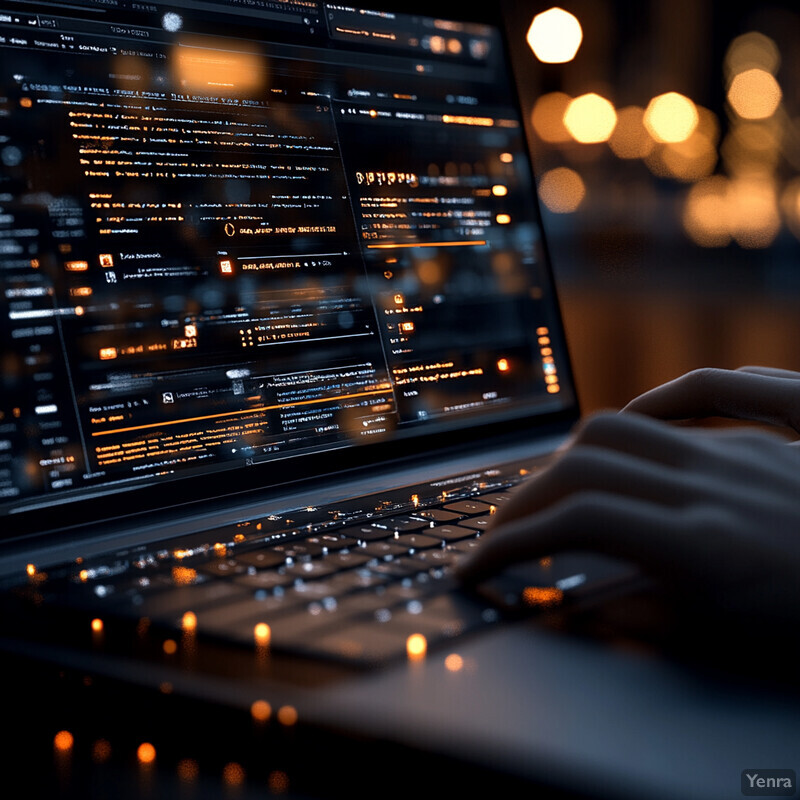
Integrating AI tools directly into journalists’ workflows means that as reporters write articles, scripts, or social media posts, the system can flag questionable claims and suggest reliable sources for verification. This happens almost instantaneously, allowing professionals to correct inaccuracies before publication. The immediacy is crucial: rather than waiting until after misinformation has spread, journalists can prevent it at the source. Over time, these systems learn from user feedback, honing their accuracy and adaptability to the particular style and subject matter of the newsroom, ultimately fostering a habit of verification that improves overall content quality.
4. Credibility Scoring of Sources
Machine learning algorithms can assess the credibility of a source based on its historical accuracy, editorial standards, and reliability, helping journalists quickly evaluate whether a particular source is trustworthy.
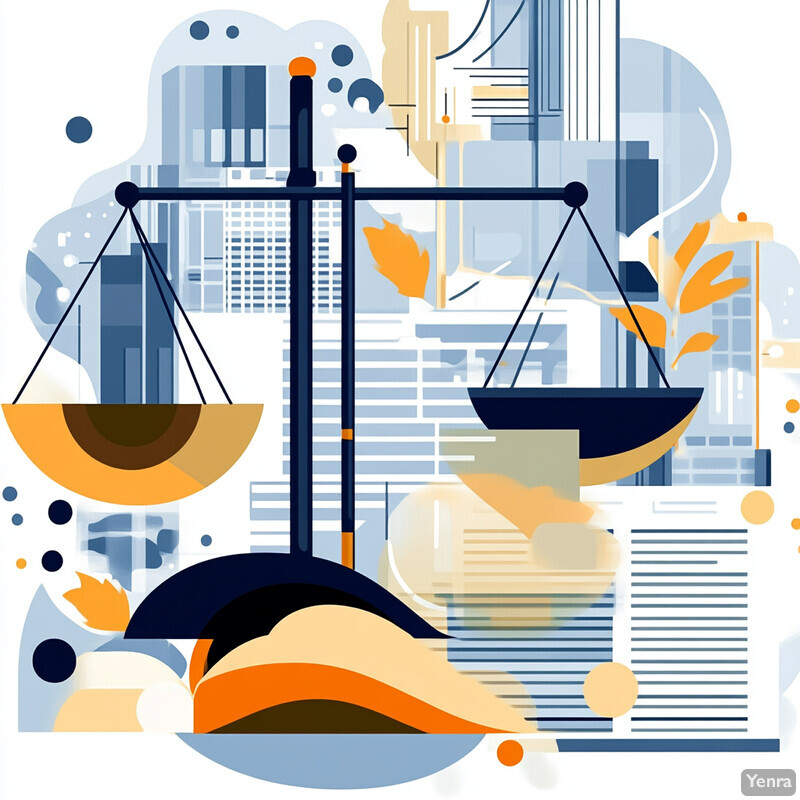
AI-driven credibility scoring relies on analyzing a wide range of indicators, from historical accuracy and the editorial standards of the source to patterns of misinformation or bias. Machine learning models might factor in the reputation of the outlet, its political leanings, transparency in corrections, expert evaluations, and even user feedback. This analysis produces a dynamic credibility index, which journalists can consult when deciding whether to rely on information from that source. Having an at-a-glance reliability metric ensures reporters spend their time verifying information from more questionable outlets, while consistently accurate sources can be trusted more readily.
5. Automated Cross-Referencing with Databases
AI can rapidly compare newly made claims against large databases of verified facts, news archives, and public records, streamlining the verification process.
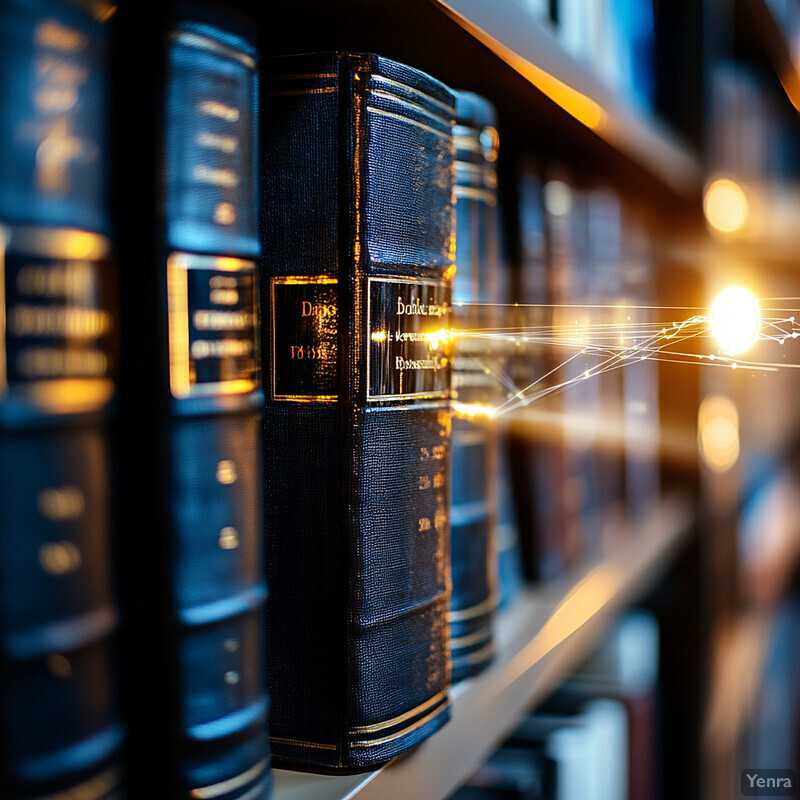
AI-powered cross-referencing connects new statements to massive repositories of previously verified facts, public records, reputable academic publications, and vetted journalistic archives. The system automatically identifies relevant data points, historical events, or official statements that confirm or refute a given claim. By mapping current assertions to established truths or recorded occurrences, fact-checkers quickly gain context and support for their conclusions. This rapid, automated linkage reduces the time-consuming nature of traditional research, allowing more thorough checks to be completed in a fraction of the time.
6. Duplicate Content Detection
Tools can identify repetitive or recycled misinformation campaigns across different platforms, enabling journalists to track the evolution and spread of disinformation.
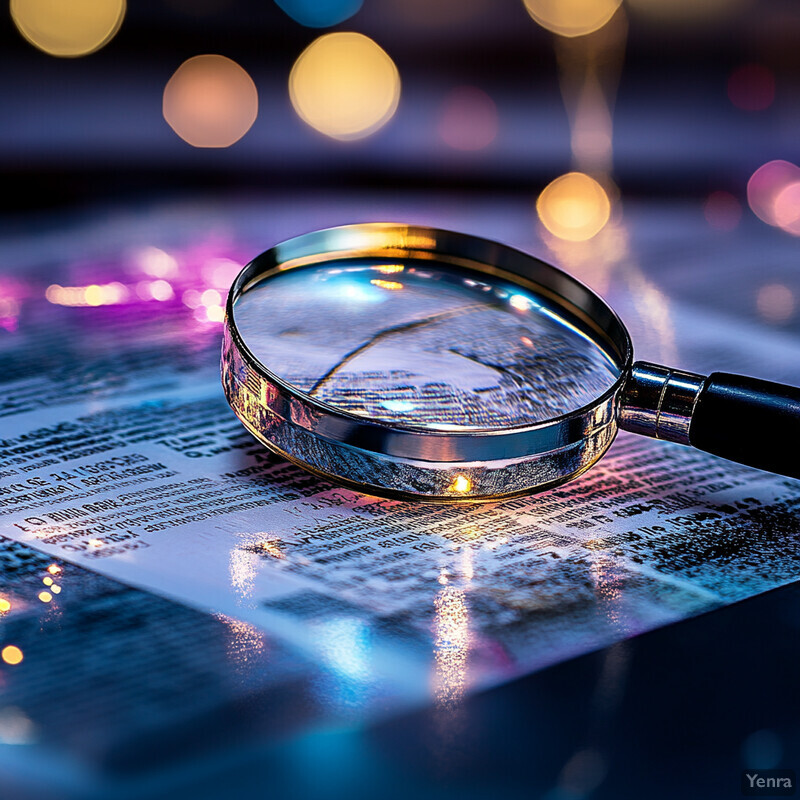
Misinformation often spreads through the repetition of the same or similar content across multiple outlets and platforms. AI-equipped tools can detect patterns of duplication, pinpointing when a misleading claim resurfaces. By highlighting these repetitions, fact-checkers and journalists can trace the path of a rumor, identify which sources are amplifying it, and determine whether it is part of a coordinated disinformation campaign. Understanding how claims propagate enables more strategic interventions, such as clarifications, debunking articles, or outreach to platforms that host the repeated falsehoods.
7. Semantic Analysis of Statements
AI can understand synonyms, paraphrases, and related concepts, making it possible to verify not just exact quotes but also statements that convey the same meaning in different words.
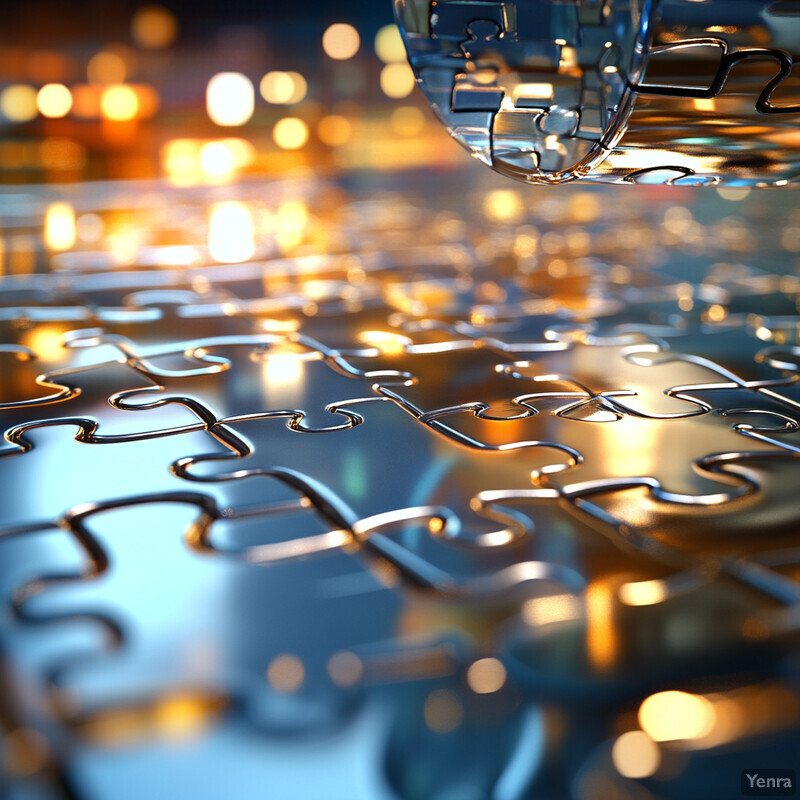
AI systems perform semantic analysis to understand the underlying meaning of statements, recognizing that two sentences with different wording might convey the same claim. This includes identifying paraphrases, synonyms, related concepts, and linked assertions. Consequently, the AI can flag instances where a speaker avoids repeating a fact-checked falsehood verbatim but tries to convey the same idea in new terms. For journalists, this ensures that subtle attempts to reintroduce debunked claims do not slip through the cracks, and that their fact-checking remains effective, comprehensive, and future-proof.
8. Multilingual Fact-Checking
AI-driven translation tools help fact-checkers working with sources in multiple languages, opening access to global documents, reports, and news coverage.
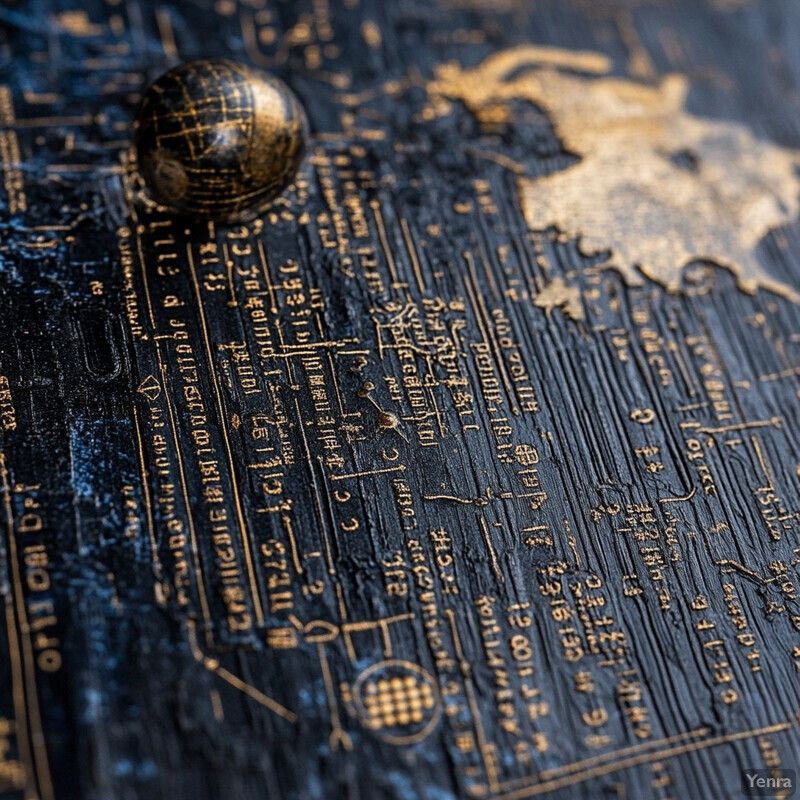
Language barriers can limit the scope of traditional fact-checking. With AI-driven translation and NLP, fact-checkers can now access and verify claims sourced from foreign-language reports, documents, and social media posts. By automatically translating content and preserving contextual nuances, AI enables a truly global approach to verification. Journalists working on international or cross-border investigations benefit immensely, as they can easily gather information from multiple linguistic communities, verify authenticity and accuracy, and produce more informed and nuanced reporting that transcends language boundaries.
9. Image and Video Verification
Image recognition and deepfake-detection algorithms help journalists verify if images or videos have been manipulated, providing a critical layer of multimedia authenticity checks.
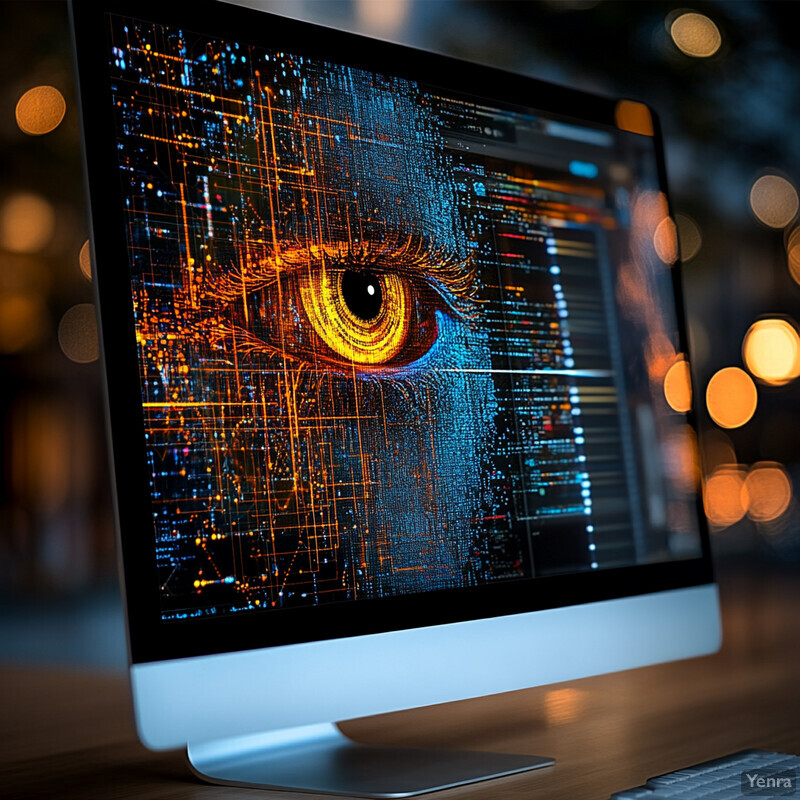
Advancements in computer vision and deep learning techniques enable AI to detect doctored images, manipulated videos, and deepfakes. By analyzing pixel patterns, compression artifacts, shadows, reflections, and other subtle cues, these tools can determine if multimedia content has been altered. Additionally, AI can verify whether a video’s audio track aligns with its visual elements, or whether the context matches metadata like timestamps or geolocation tags. With this capability, journalists can more confidently rely on visual evidence, knowing that they have AI assistance in spotting potentially deceptive imagery.
10. Speech-to-Text Processing for Audio Fact-Checks
AI can transcribe interviews, debates, and podcasts, quickly turning them into text for easier claim identification and verification.
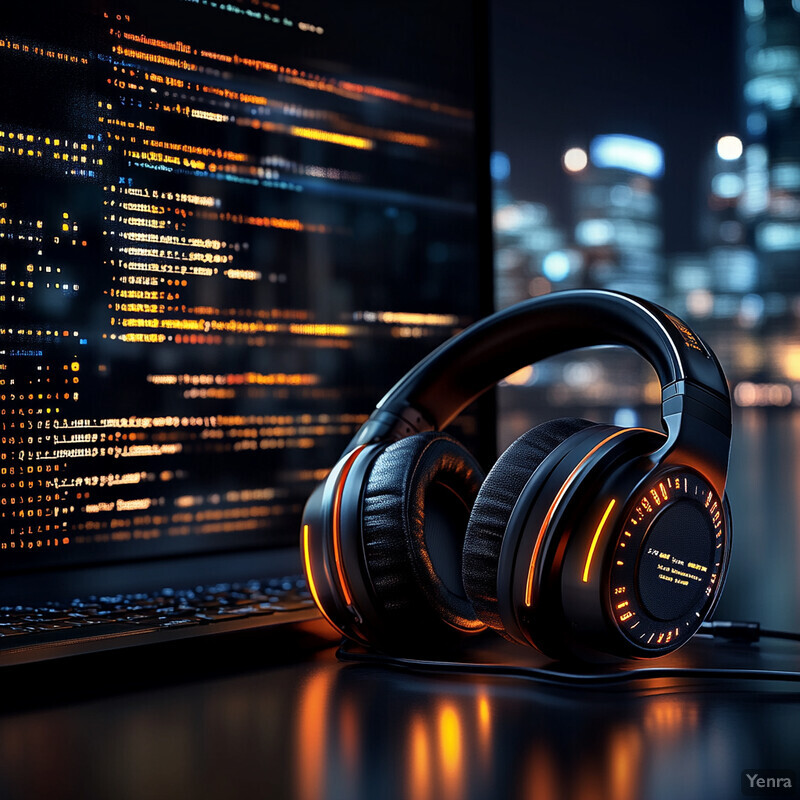
When sources are interviews, podcasts, or broadcasts, audio content often needs to be transcribed before verification can begin. AI-powered speech-to-text conversion streamlines this process, turning spoken words into searchable text. Fact-checkers can quickly scan transcripts for claims, and NLP tools can then analyze these statements for accuracy. This integration drastically reduces the time and human effort required, especially when dealing with lengthy recordings, interviews featuring multiple speakers, or real-time coverage of events such as political debates and press conferences.
11. Pattern Recognition in Disinformation Campaigns
Machine learning algorithms can identify recurring patterns, actors, and networks of influence behind coordinated misinformation efforts, guiding reporters toward the root of false claims.
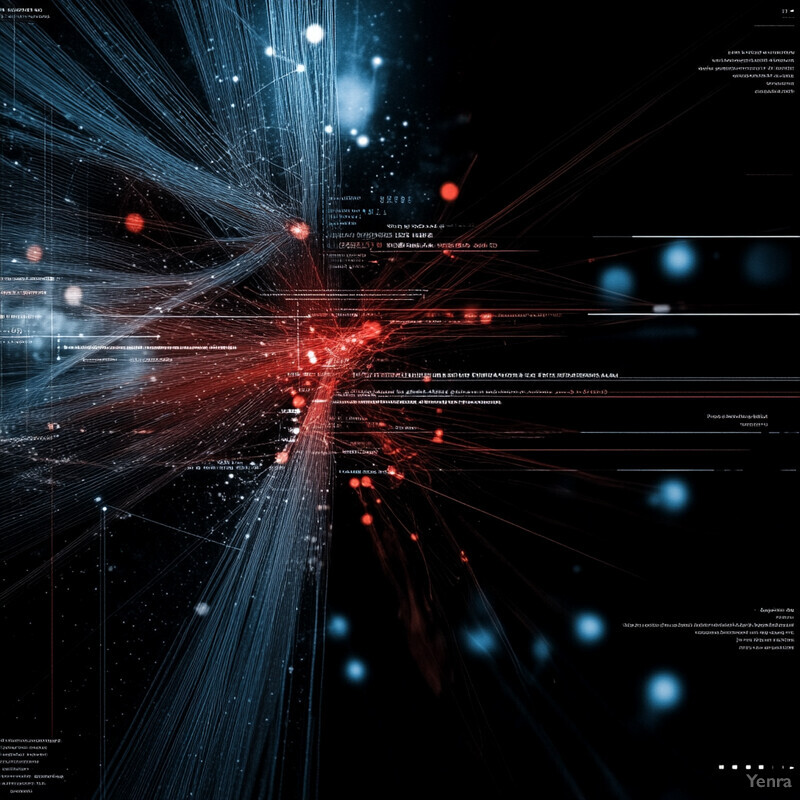
AI can analyze vast quantities of data to identify patterns underlying organized misinformation networks. By mapping out the distribution channels, influencers, recurring talking points, and cross-references, these algorithms help journalists understand the origins and impact of coordinated efforts to shape public narratives. Recognizing such patterns allows media organizations to expose hidden agendas, highlight the methods used to spread false claims, and hold responsible parties accountable, ultimately making the information ecosystem more transparent and resistant to manipulation.
12. Sentiment Analysis to Detect Polarizing Claims
By analyzing the emotional tone of content, AI tools can help flag potentially contentious or sensational claims that warrant extra scrutiny.
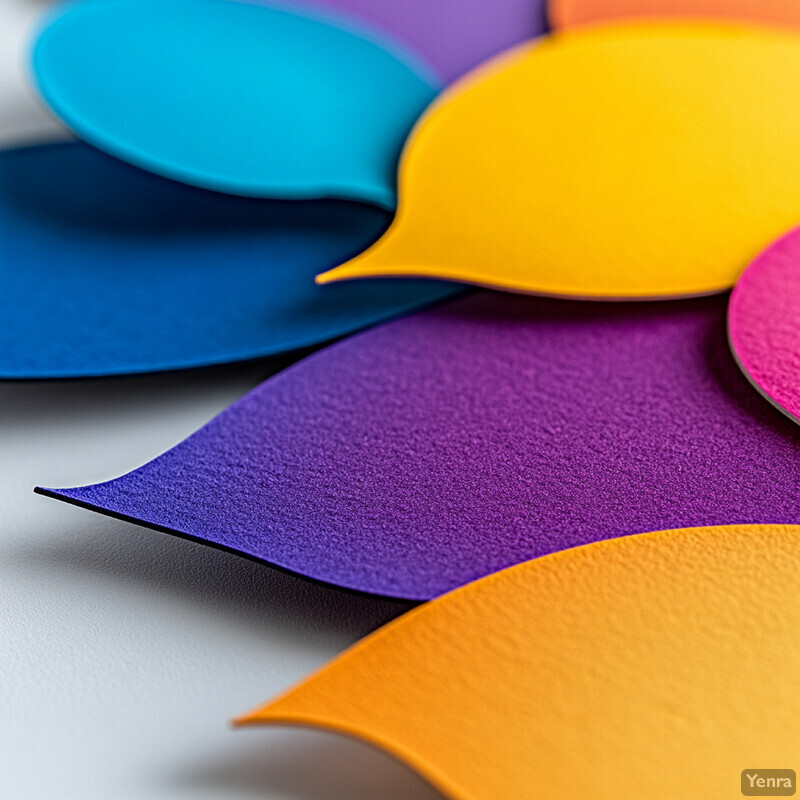
AI-driven sentiment analysis interprets emotional tone, stance, and intensity within content. By identifying claims that provoke strong emotional responses—such as anger, fear, or outrage—fact-checkers can prioritize verifying these statements. High-emotion claims are often more likely to go viral, influencing public perception rapidly. By focusing on these high-impact assertions first, journalists can mitigate potential harm by quickly providing accurate, verified information that neutralizes emotionally charged falsehoods before they become deeply ingrained in the public discourse.
13. Temporal Fact-Checking
AI can verify whether an event referenced in a claim actually took place at a given time, crucial for historical fact-checking or debunking out-of-context citations.
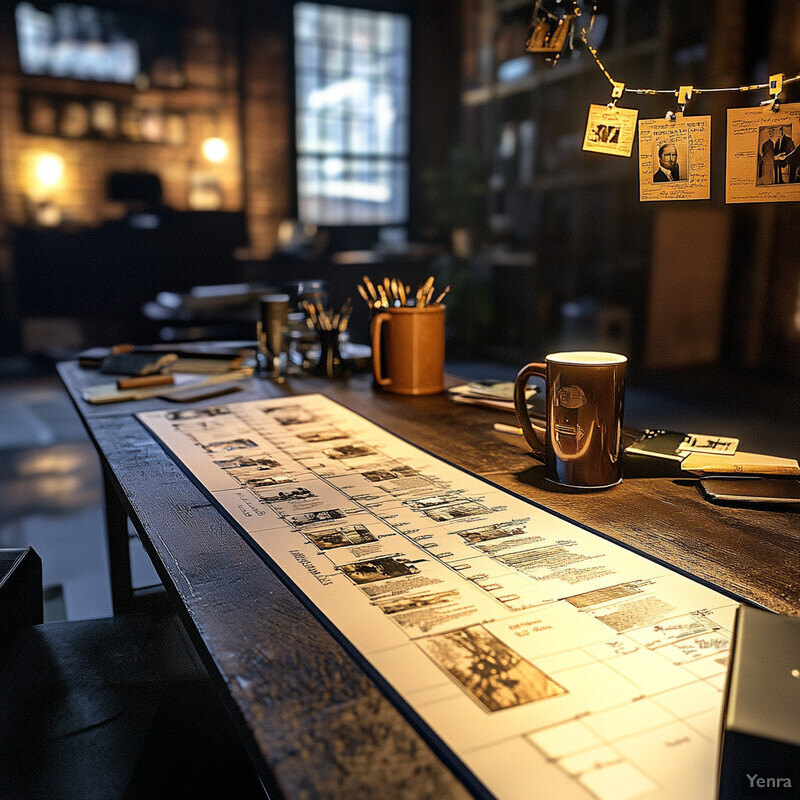
Many misleading claims rely on manipulating timelines—either presenting events out of chronological order or associating them with the wrong historical context. AI tools designed for temporal fact-checking compare referenced events to verified timelines, checking their authenticity and relevance. This helps journalists confirm whether a claimed incident happened when alleged, or if it’s being taken out of its original time frame to distort meaning. Ensuring temporal accuracy is vital for maintaining historical integrity and preventing the rewriting of past events to fit present narratives.
14. Automated Linking to Reputable Fact-Checking Organizations
Tools can automatically direct journalists to relevant fact-check articles from established organizations like PolitiFact, Snopes, or FactCheck.org, speeding up verification.
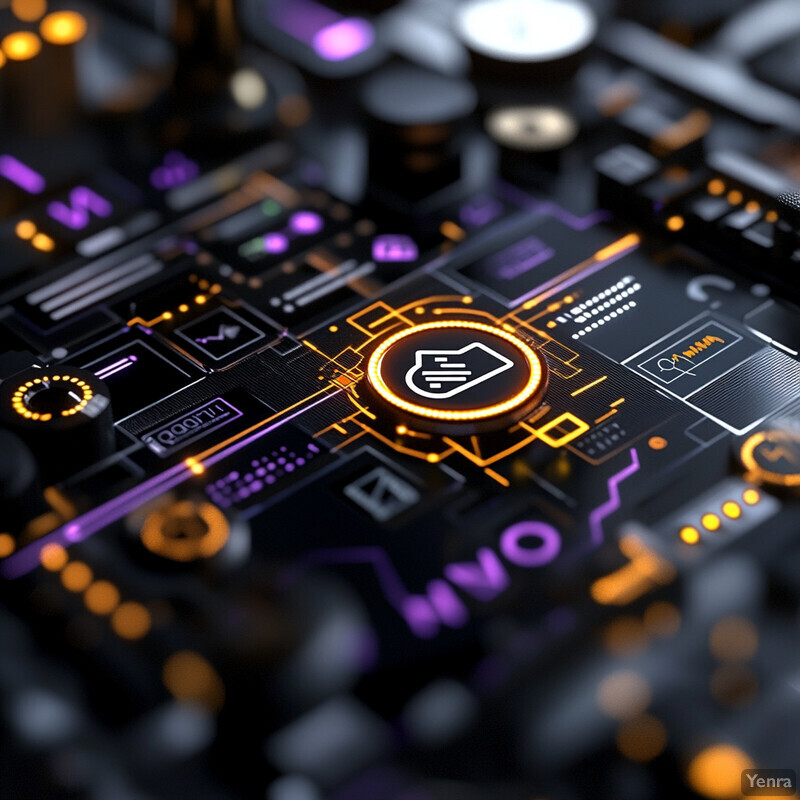
Fact-checking is often a collaborative, global effort. AI can streamline the process by automatically linking dubious claims to existing verifications from trusted entities like PolitiFact, Snopes, or FactCheck.org. Journalists can instantly access reputable assessments, reducing the time and effort needed to substantiate or refute statements. In turn, readers benefit from consistent, authoritative coverage, as the inclusion of such links reinforces credibility and transparency while enhancing the overall informational value of journalistic content.
15. Source Clustering and Influence Mapping
Using network analysis, AI can group related sources or stories, helping journalists understand who’s influencing a particular narrative and whether a claim is part of a coordinated misinformation push.
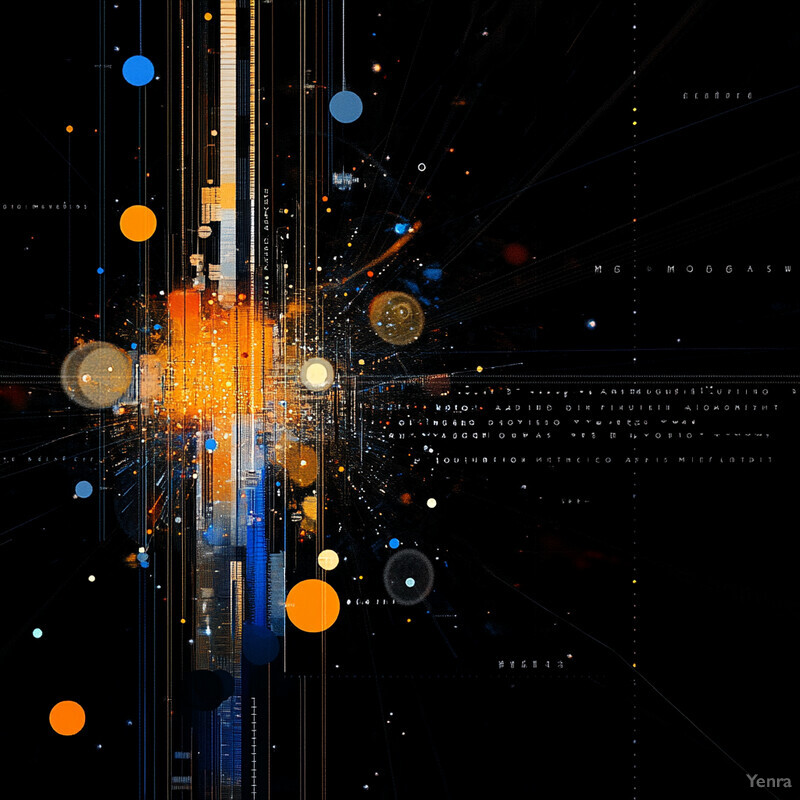
By deploying network analysis and clustering algorithms, AI can group related articles, social media posts, and websites around a claim or topic. This technique reveals which sources are amplifying certain narratives and how they interconnect. Journalists gain insights into the complexity and reach of a disinformation ecosystem—who the key players are, where influence originates, and how particular claims gain traction. With this knowledge, they can better target their verification efforts, exposing the roots of disinformation and offering readers a clearer picture of how falsehoods spread.
16. Anomaly Detection for Out-of-Pattern Claims
AI can flag news stories or statements that deviate significantly from known facts or reliable reporting patterns, prompting closer human examination.
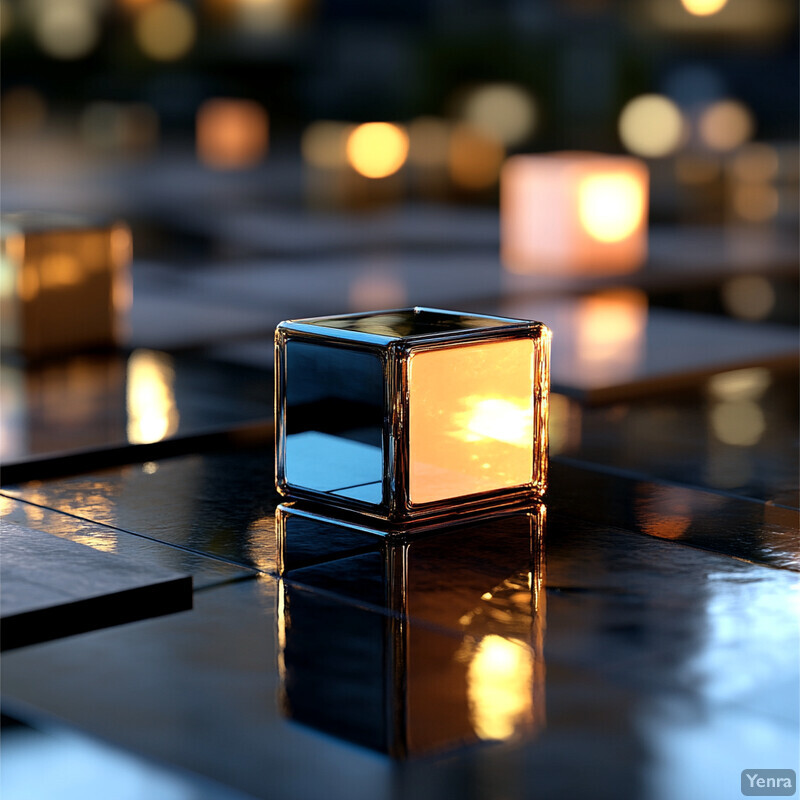
Sometimes misinformation does not match established patterns. AI can flag anomalies—statements that differ starkly from known data, widely accepted facts, or the typical editorial stance of a source. By focusing on these unusual claims, journalists can isolate potential misinformation before it becomes a trend. This proactive approach ensures that fact-checking doesn’t only respond to popular viral falsehoods, but also anticipates and preempts new misinformation narratives as they emerge, reinforcing the integrity of the information ecosystem.
17. Historical Record Validation
For long-lead investigative journalism, AI can compare a claim against historical data sets, archives, and academic research to ensure factual consistency over time.
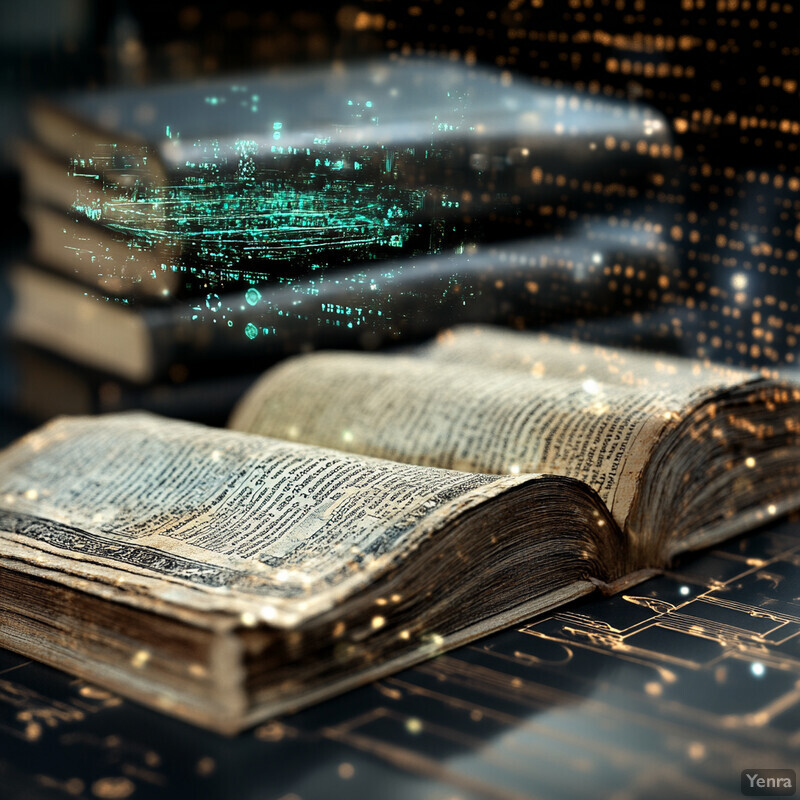
Checking older claims often involves delving into archives, academic journals, old newspapers, and historical datasets. AI expedites this process by swiftly retrieving relevant historical information, comparing past assertions to current events, and ensuring consistency. Journalists can quickly verify whether today’s claims align with established historical records, exposing attempts to rewrite or misrepresent the past. This capability supports long-form investigative pieces and fact-checks that rely heavily on robust historical context, thereby maintaining the factual continuity of public discourse.
18. User-Generated Content Verification
AI tools can help authenticate or refute claims made in social media posts, comment sections, or online forums, where disinformation often thrives.
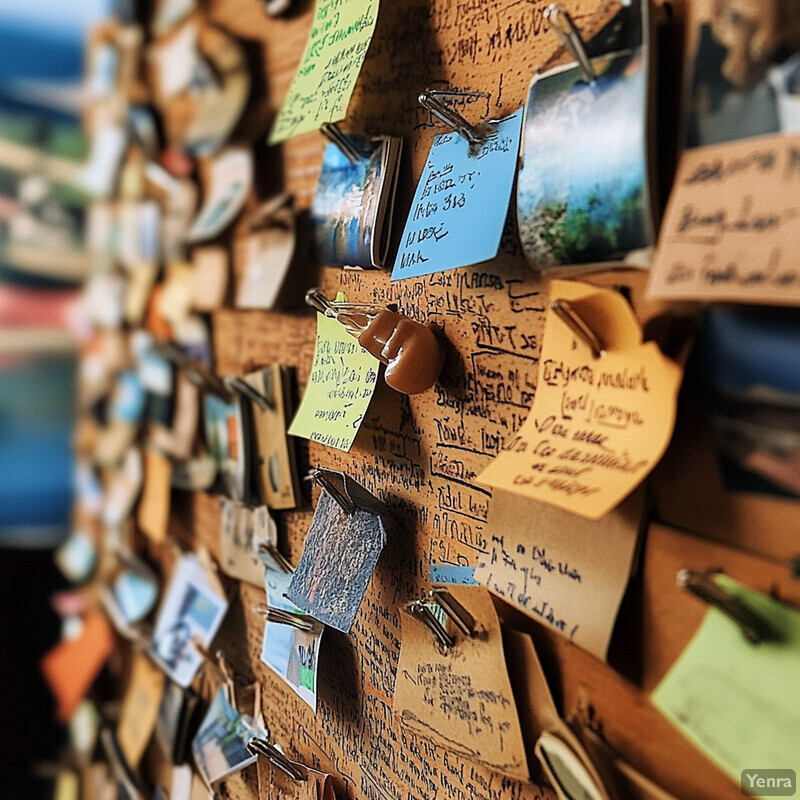
With the rise of social media and citizen journalism, large quantities of user-generated content appear daily. AI tools help fact-checkers sort through this content by analyzing source credibility, checking metadata for authenticity, and cross-referencing user claims against reputable databases. By quickly filtering out fabricated or misleading posts, journalists can devote more time to verifying authentic stories or footage. This not only helps counter disinformation but also ensures credible user contributions receive the attention and verification they deserve.
19. Adaptive Learning from Human Fact-Checkers
Machine learning systems can improve their accuracy over time by learning from the corrections and inputs of trained fact-checkers, progressively refining their ability to spot falsehoods.
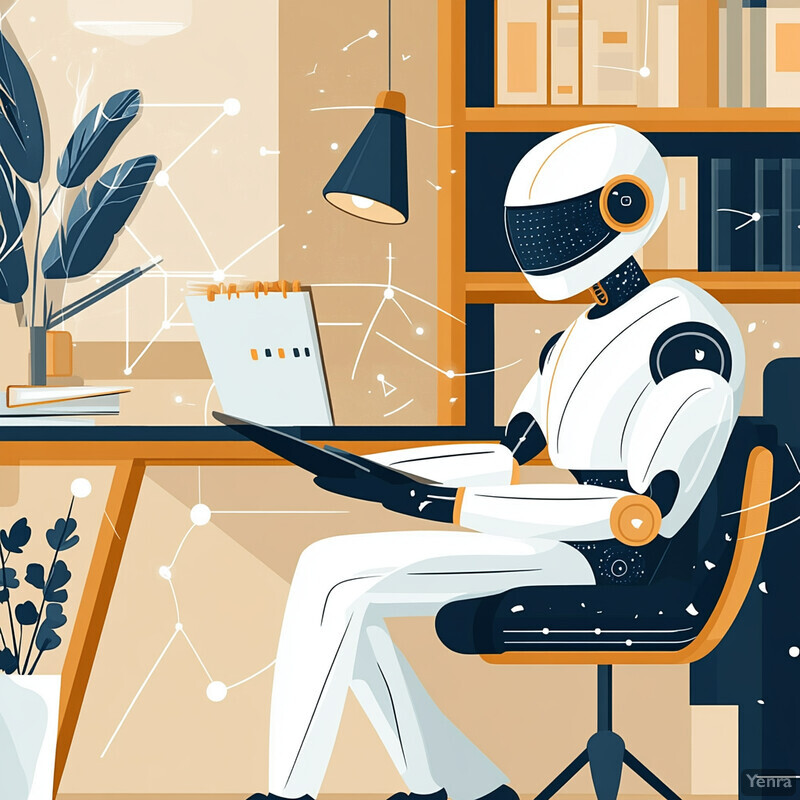
AI models continually improve through iterative feedback loops. Human fact-checkers correct and refine AI analyses, teaching the systems which patterns indicate accurate or inaccurate claims. Over time, the algorithms become increasingly precise, reducing false positives and negatives. This synergy between human expertise and machine efficiency ensures that fact-checking processes keep pace with evolving language, shifting political climates, and new forms of manipulation. As a result, both AI and journalists grow more effective, creating a virtuous cycle of continuous improvement.
20. Personalized Fact-Checking Dashboards
AI can tailor verification tools for individual journalists or newsrooms, suggesting claim-relevant evidence and displaying real-time credibility assessments to aid in the investigative process.
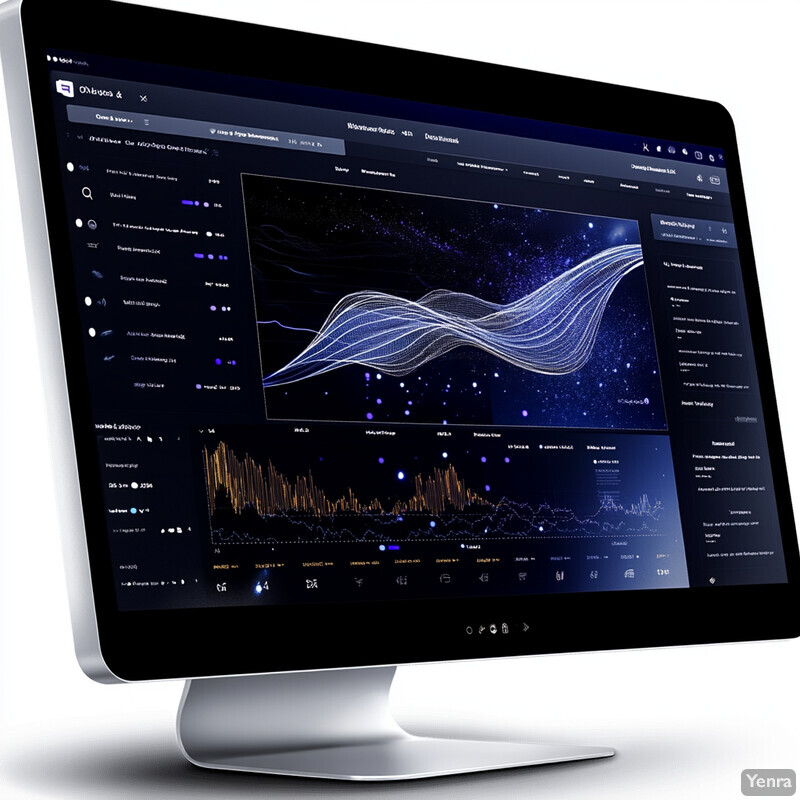
Every newsroom or journalist has distinct priorities, coverage areas, and editorial guidelines. AI-driven personalized dashboards allow fact-checkers to filter and prioritize claims based on their relevance, topic interest, or the types of sources they typically encounter. The dashboard can display real-time credibility assessments, automated cross-references, and recommended experts to consult. Customization ensures that each journalist’s workflow is optimized for their beats and editorial standards, fostering a more efficient and targeted approach to verification that enhances the overall quality and trustworthiness of news reporting.