1. Algorithmic Trading Enhancements
AI-driven trading algorithms can process market data in real-time, identify trading opportunities, and execute trades faster and more accurately than human traders, improving both profitability and execution efficiency.
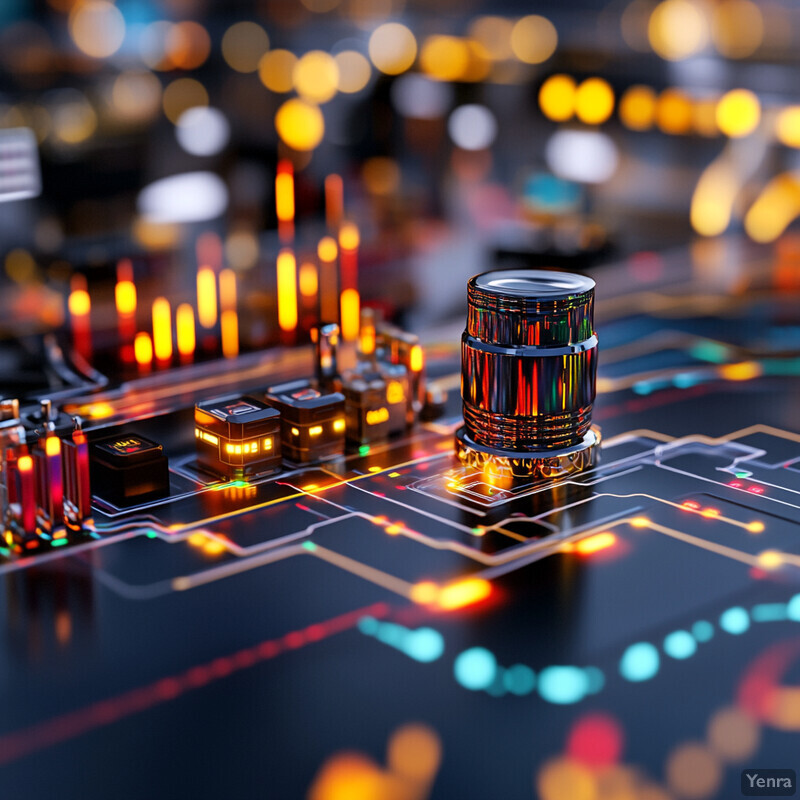
AI-driven algorithmic trading systems leverage complex machine learning models to process high-frequency market data, news feeds, and order book dynamics in real time. Rather than relying solely on traditional, rule-based strategies, these systems continuously learn from evolving market conditions. This results in more accurate trade signals, improved timing, and optimal order execution to minimize slippage and transaction costs. By monitoring multiple data streams simultaneously and adapting to new patterns, AI-based trading algorithms can detect subtle market inefficiencies and capitalize on them before competitors, leading to stronger returns and reduced risk exposure.
2. Predictive Modeling for Asset Price Movements
Advanced machine learning models can sift through massive amounts of historical and real-time data to predict future price movements of stocks, bonds, commodities, or currencies, enabling more informed investment decisions.
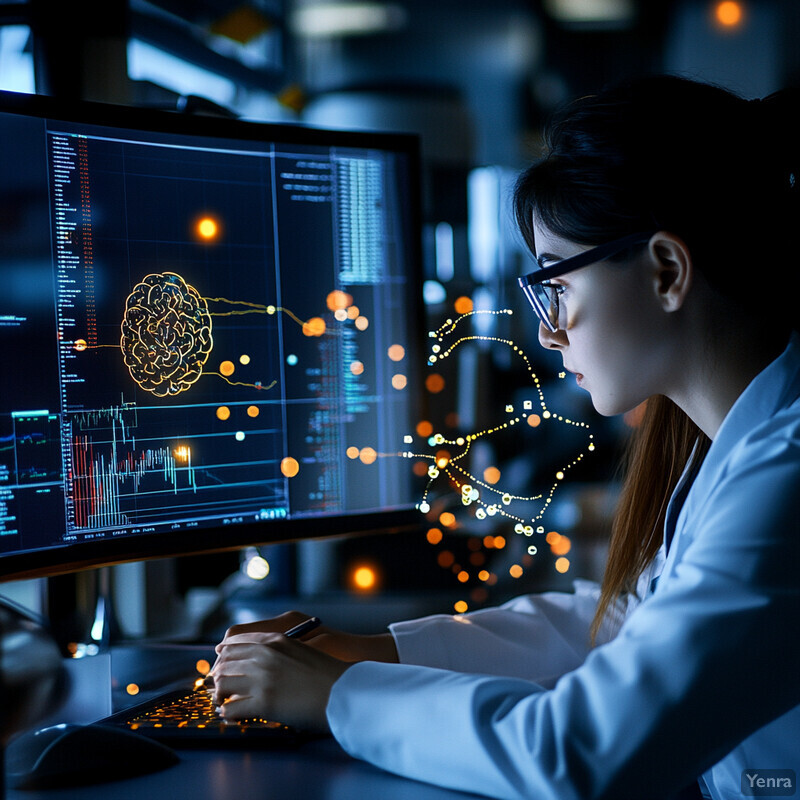
Traditional financial models often struggle to incorporate the breadth and complexity of modern market data. AI-powered predictive models, on the other hand, integrate signals from price histories, macroeconomic indicators, alternative data sources, and geopolitical developments. By applying advanced techniques such as deep learning, these models can identify patterns and relationships that humans might miss. As a result, investors gain more accurate forecasts of asset price trajectories. Over time, these forecasts can inform buy, sell, or hold decisions with greater confidence, thereby enhancing the overall precision and profitability of investment strategies.
3. Automated Portfolio Rebalancing
AI-based tools can continuously monitor portfolio compositions, automatically adjusting holdings to maintain target allocations, risk levels, and return objectives without manual intervention.
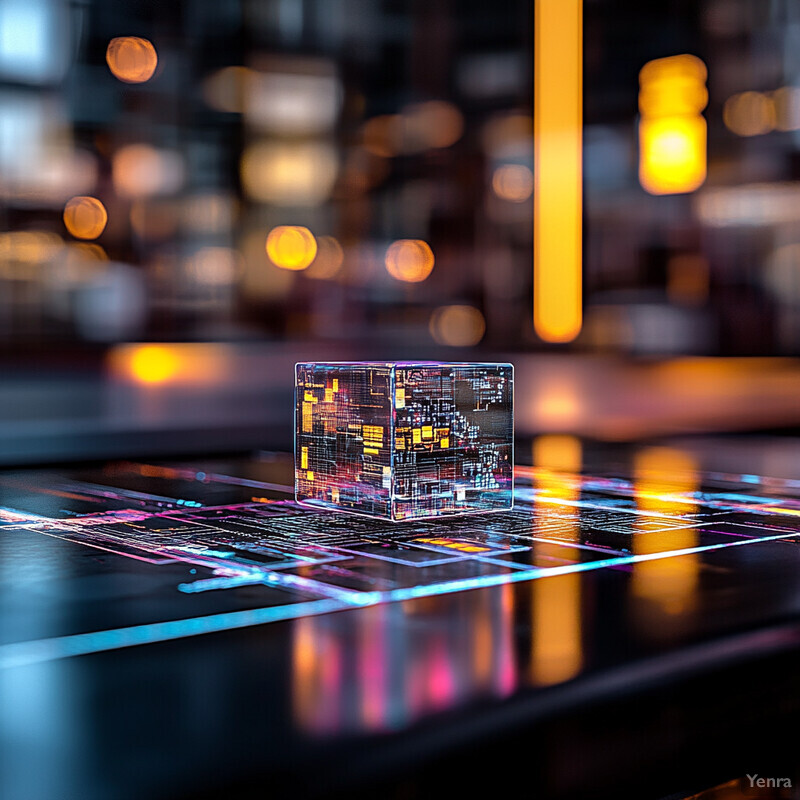
Maintaining an optimal portfolio composition is crucial for achieving desired risk-return profiles, but doing so manually can be time-consuming and prone to error. AI-powered rebalancing tools continuously monitor a portfolio’s positions and market conditions. When certain assets drift from their target allocations due to price fluctuations or newly identified risk factors, the system automatically rebalances holdings. This process ensures that the portfolio remains aligned with the investor’s strategic goals and risk tolerance. By automating these adjustments, asset managers improve efficiency, reduce operational overhead, and respond more quickly to changing market dynamics.
4. Enhanced Risk Management and Stress Testing
Using AI, investment managers can model a wide range of market scenarios—including rare or extreme events—more accurately.
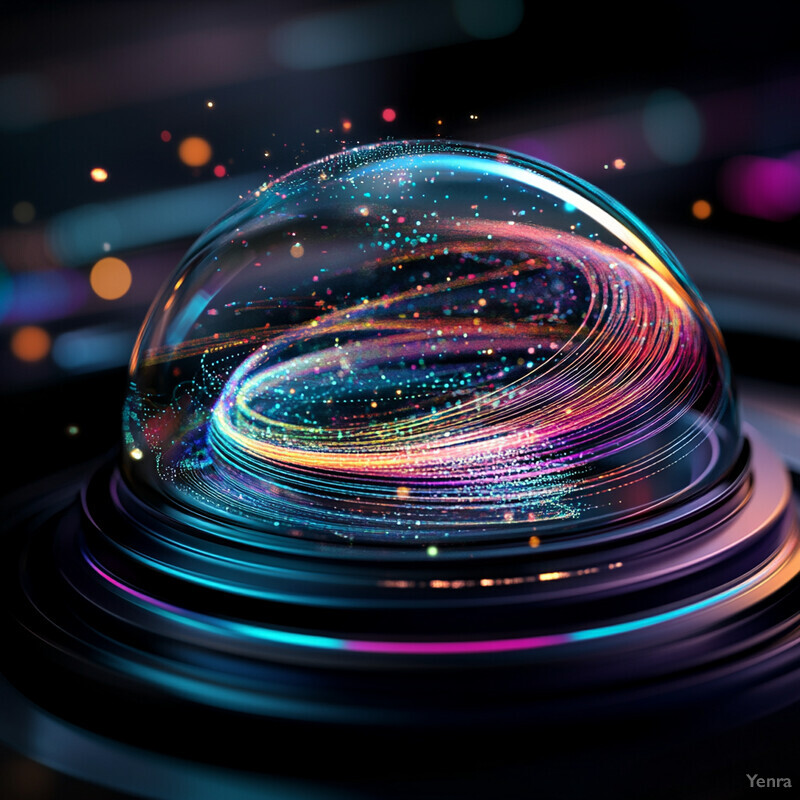
Understanding the potential impact of extreme market events and evolving risk factors is vital for robust investment management. AI-driven risk models can simulate thousands of market scenarios, including historically rare conditions, using machine learning to refine their accuracy over time. These simulations help identify vulnerabilities in the portfolio, measure potential drawdowns, and gauge the resilience of different strategies under crisis conditions. With richer scenario analysis and more nuanced stress testing, asset managers can implement proactive risk mitigation techniques—such as dynamic hedging or liquidity buffers—thereby bolstering the portfolio’s long-term stability.
5. Sentiment Analysis from Unstructured Data
Natural Language Processing (NLP) tools can parse social media posts, news articles, earnings call transcripts, and other unstructured text to gauge market sentiment and incorporate it into investment decisions.
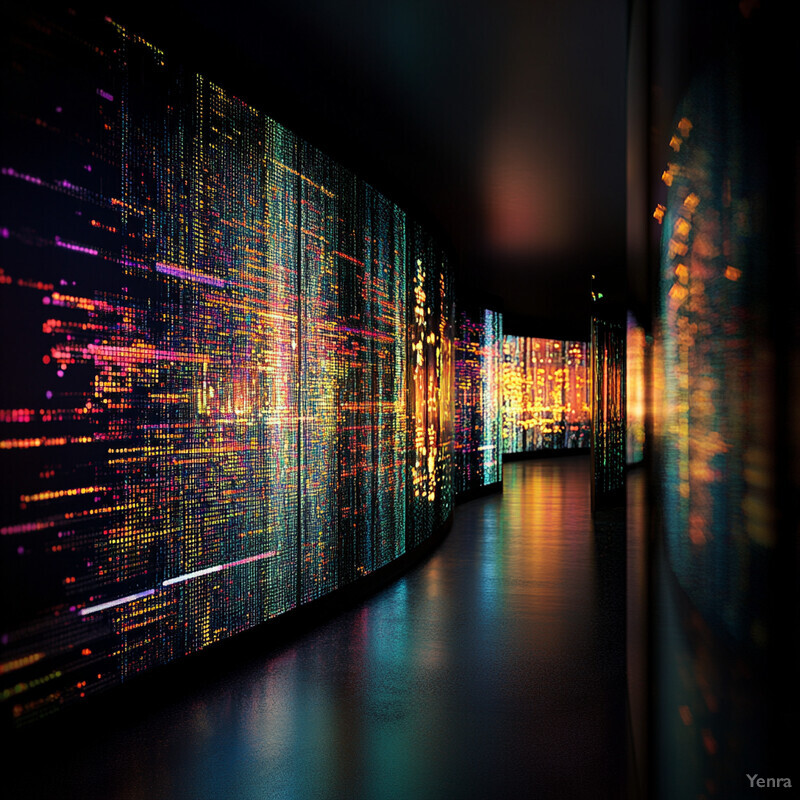
Market sentiment often moves faster than formal financial reporting. AI-powered Natural Language Processing (NLP) techniques enable managers to parse high volumes of unstructured data, including social media chatter, news articles, company blogs, and earnings call transcripts. By extracting entities, topics, and sentiment polarity, these models provide insights into public perceptions, investor confidence, and emerging market narratives. In turn, sentiment analysis can inform timing and selection decisions, alerting managers to developing trends, potential market reversals, or shifts in consumer preference that may not yet be reflected in traditional financial metrics.
6. Credit Scoring and Fixed-Income Analysis
AI can provide more nuanced credit risk assessments by analyzing large sets of borrower data and macroeconomic indicators.
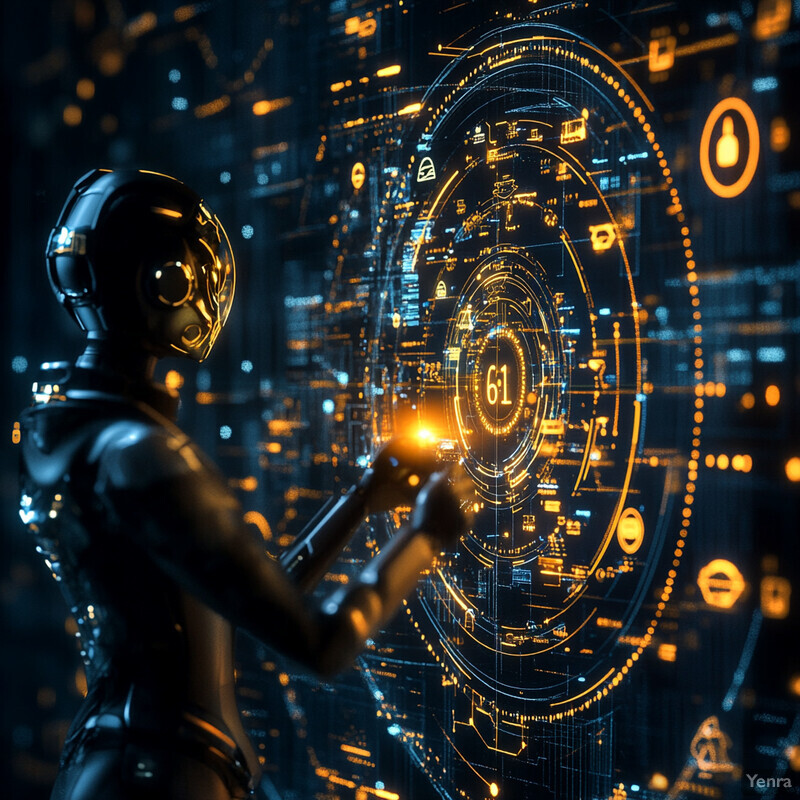
Credit markets rely on accurate assessments of default risk and borrower quality. AI models enhance this evaluation by tapping into a vast range of data points—from corporate financial statements and macroeconomic indicators to alternative datasets like social signals or supply chain networks. By identifying subtle patterns in these data sets, AI can offer more granular credit scoring and continuous monitoring of credit spreads. This level of precision helps fixed-income investors price bonds more accurately, select high-quality issuers, and anticipate credit events, thus improving yield and maintaining portfolio stability.
7. Identifying Hidden Relationships Among Assets
Machine learning algorithms can uncover non-obvious correlations between different asset classes or securities that may not be apparent through traditional quantitative methods, informing diversification and hedging strategies.
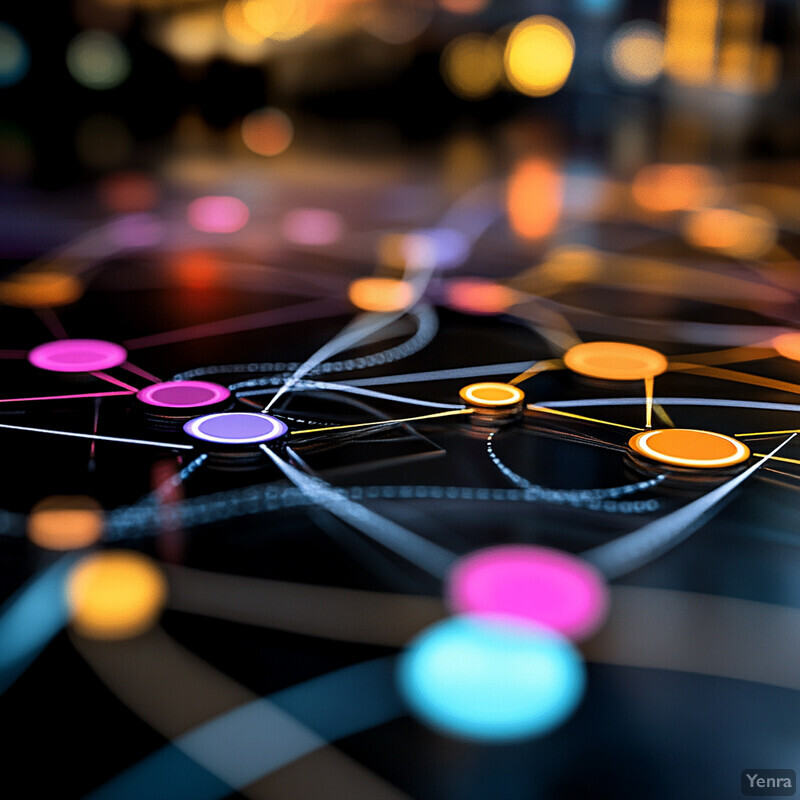
Financial markets exhibit complex interdependencies that can defy simple correlation analysis. Machine learning algorithms can uncover intricate, nonlinear relationships between various asset classes, industries, and regions. By detecting these hidden linkages, asset managers can devise more effective diversification strategies, pairing assets that respond differently to market shocks. This understanding also helps in building more robust hedging techniques that anticipate how certain holdings will behave under different conditions. The result is a richer understanding of portfolio dynamics and more resilient investment structures.
8. Faster and More Accurate Research
AI-powered research assistants can rapidly review vast amounts of financial documents, reports, and market data, summarizing insights and accelerating the research process, allowing analysts to focus on higher-level strategic thinking.
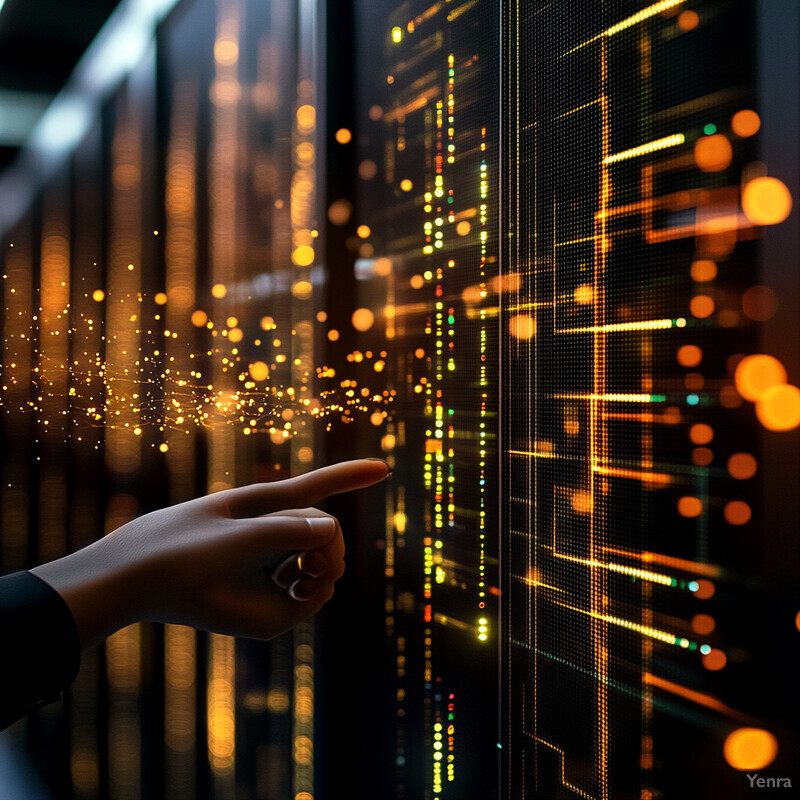
Traditionally, analysts spend countless hours sifting through lengthy research reports, earnings data, and market studies. AI-powered research assistants expedite this process by crawling through large information sets, synthesizing key insights, and summarizing critical points. These systems can highlight emerging trends, forecast industry shifts, and spot discrepancies in financial statements. With the heavy lifting automated, human analysts can devote more time to high-level strategy formulation and qualitative judgment. This efficient division of labor increases the throughput and quality of the firm’s investment research activities.
9. Enhanced Asset Allocation Strategies
By incorporating advanced predictive analytics, AI systems can optimize asset allocation models in response to shifting economic indicators, volatility regimes, and investor objectives.
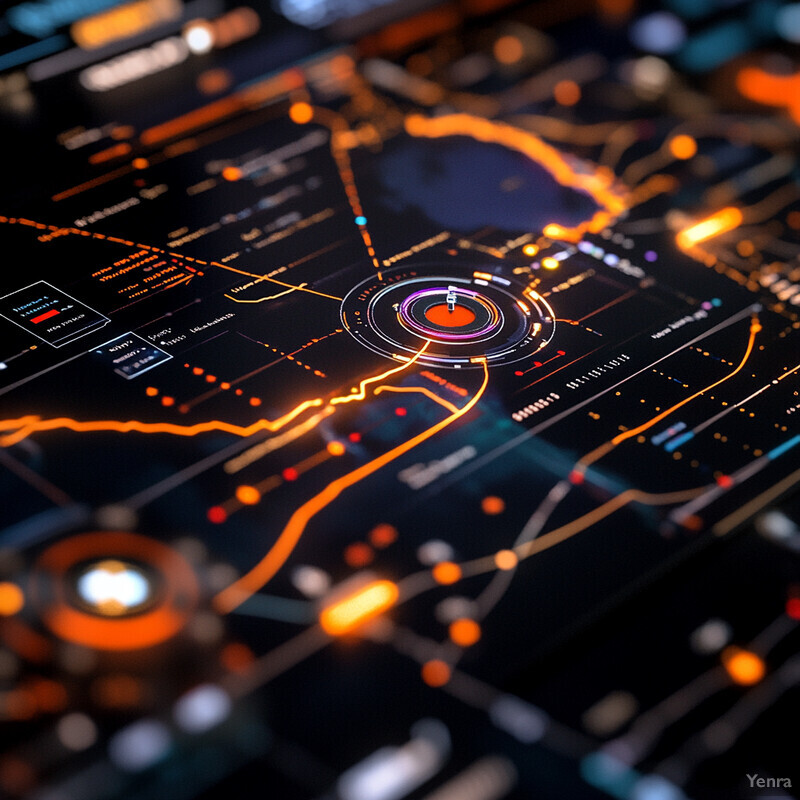
Deciding how to allocate capital across different asset classes is a fundamental challenge in portfolio management. AI-driven asset allocation models incorporate large volumes of historical data, forward-looking indicators, and scenario simulations. They dynamically adjust allocations in response to changing market conditions, investor objectives, and risk constraints. By doing so, these models help manage volatility, improve long-term risk-adjusted returns, and capture new opportunities as economic cycles evolve. As a result, asset managers can rely on a more responsive and data-rich framework for their allocation decisions.
10. Dynamic Pricing and Valuation Models
AI tools can update valuation models in near real-time using a continuous flow of data (e.g., trade volumes, analyst reports, global macro trends), providing more accurate and timely inputs for investment decisions.
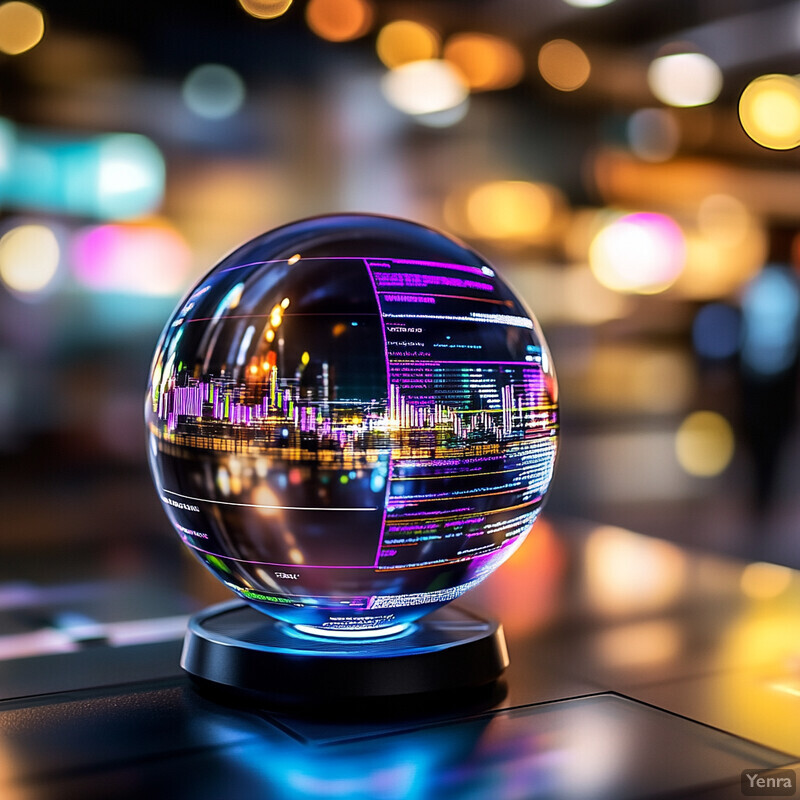
Valuing assets accurately is essential for making informed buy or sell decisions. Traditional valuation often depends on static inputs and backward-looking assumptions. AI-driven valuation engines continuously integrate real-time pricing data, macroeconomic updates, sector-specific catalysts, and sentiment indicators. This approach yields more precise, current estimates of an asset’s intrinsic worth, allowing managers to identify mispricings more effectively. Over time, these dynamic models guide more timely investment decisions, helping to exploit emerging value opportunities and avoid overpriced securities.
11. Fraud Detection and Compliance Checks
Sophisticated anomaly detection algorithms can help asset managers identify fraudulent activities, market manipulation, or compliance breaches, ensuring regulatory adherence and reducing reputational risk.
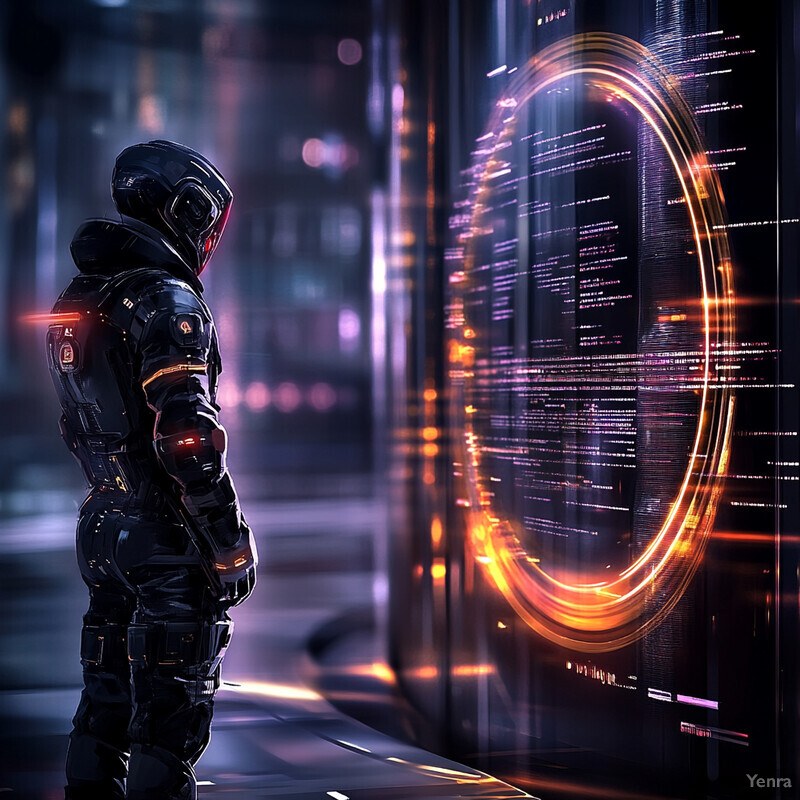
Regulatory compliance and fraud prevention are critical considerations in investment management. AI-driven anomaly detection systems can identify irregular trading patterns, suspicious transactions, and potential market manipulation. By processing transaction records, communications, and third-party data sources, these systems detect deviations from established norms. When questionable activity arises, the AI flags it for human review, ensuring timely intervention. This proactive vigilance helps safeguard the firm’s reputation, maintain investor trust, and adhere to stringent regulatory standards in a volatile global landscape.
12. Customization of Investment Products
AI enables the creation of personalized investment solutions—such as robo-advisors offering individually tailored portfolios—based on investor profiles, risk tolerance, goals, and preferences at scale.
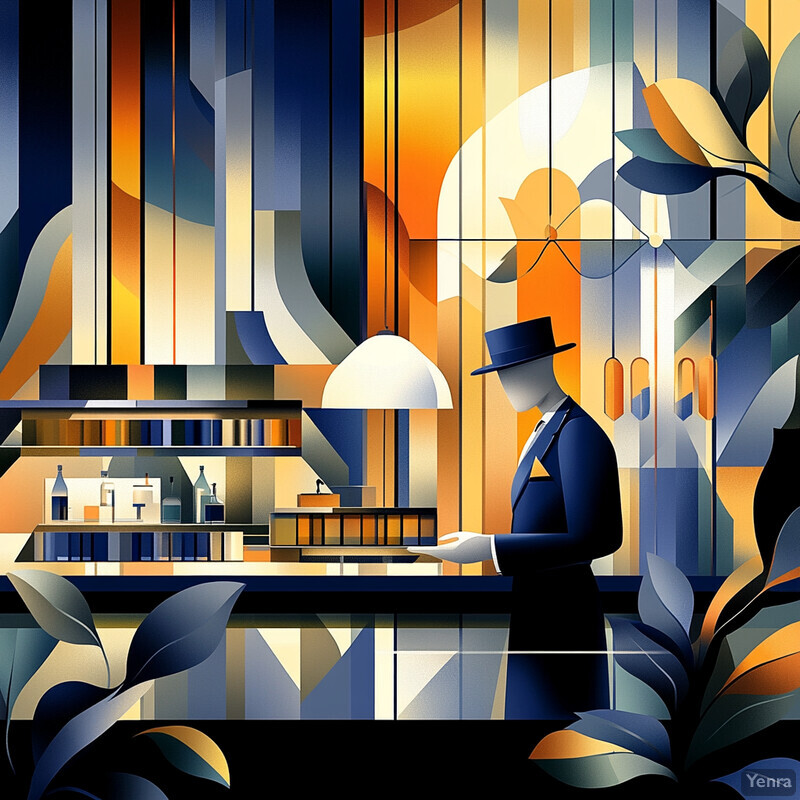
Investors increasingly demand personalized solutions that align with their unique goals, risk appetites, and values. AI-powered customization tools allow asset managers to create tailored portfolios and advisory recommendations at scale. By analyzing an investor’s historical behavior, stated objectives, and life events, these systems can propose targeted asset mixes, thematic investments (e.g., green energy), or tax-optimized portfolios. As a result, clients receive better-aligned portfolios that reflect their preferences, while managers benefit from scalable personalization that was previously difficult to achieve with traditional methods.
13. Liquidity Forecasting and Management
Machine learning models can predict liquidity conditions in various markets, helping managers adjust positions, anticipate bottlenecks, and minimize the cost of large trades.
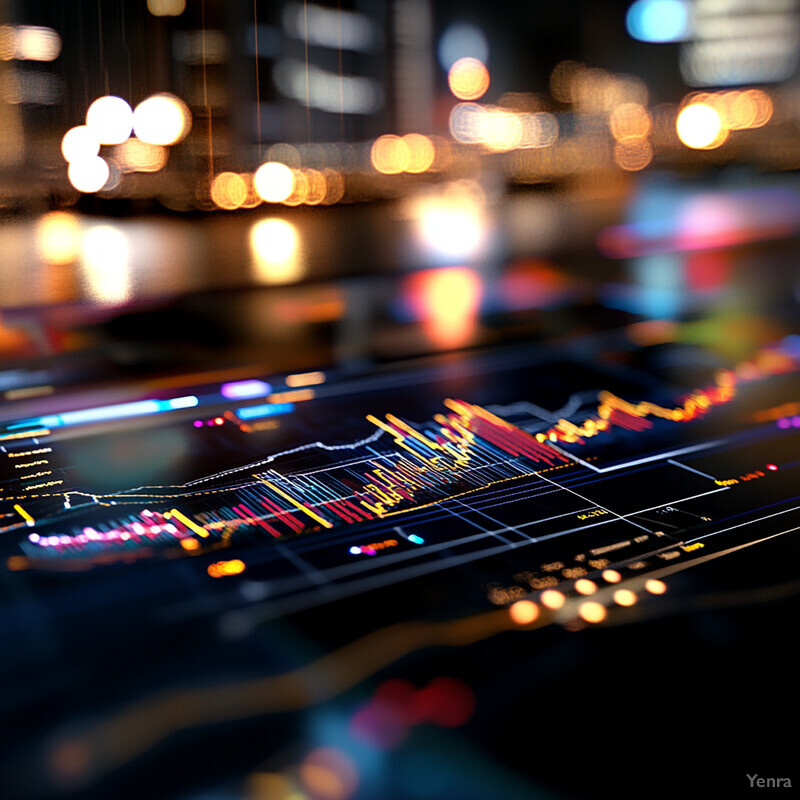
Liquidity is the lifeblood of trading. Accurately forecasting liquidity conditions is essential, especially in volatile or thinly traded markets. AI models incorporate historical trading volumes, order book data, and cross-asset correlations to predict market liquidity availability and cost. With clearer insight into when liquidity might dry up or when large trades could incur price impact, asset managers can plan their execution strategies accordingly. This foresight reduces transaction costs, prevents forced sales at inopportune times, and contributes to more stable portfolio performance.
14. Transaction Cost Analysis (TCA) Improvements
AI-driven TCA tools analyze vast market microstructure data to identify optimal execution strategies, thereby reducing transaction costs and improving overall returns.

Controlling transaction costs is a direct way to improve net returns. AI-enhanced TCA tools assess market microstructure, historical execution quality, and trading venue efficiency to recommend the best routes and times to execute trades. They can adapt to changing conditions, such as volatility spikes or widening bid-ask spreads, and adjust execution tactics (e.g., dark pool routing, algorithm selection) on the fly. By reducing slippage, commissions, and market impact, these tools help investors retain more of their investment gains and maintain a competitive edge.
15. Scenario Analysis and Economic Forecasting
AI systems integrate massive streams of macroeconomic, demographic, and geopolitical data to produce more accurate market outlooks and scenario analyses, guiding long-term strategic decisions.
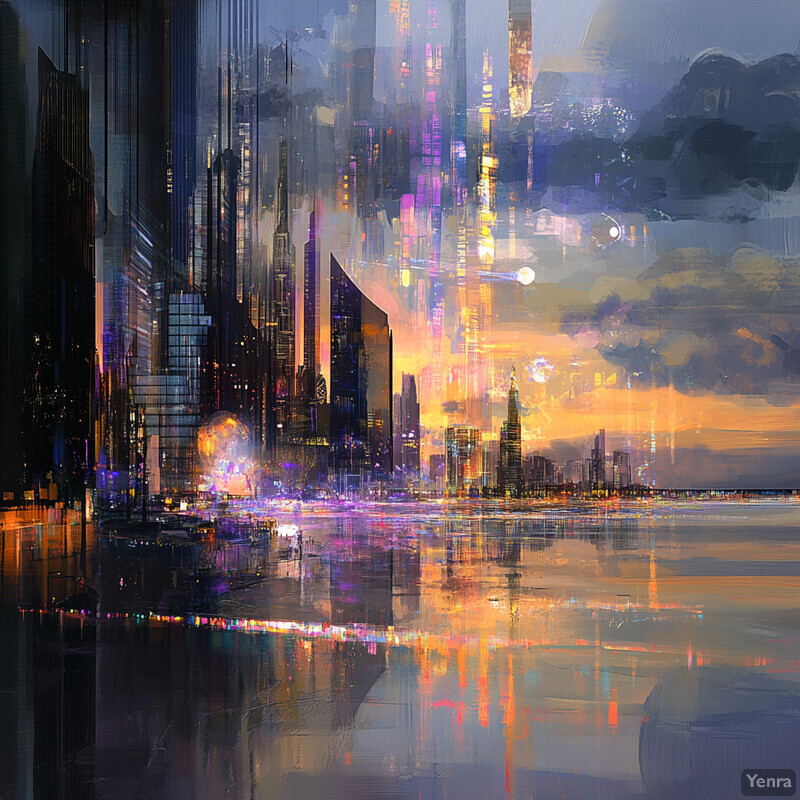
Global markets are influenced by a complex web of economic, political, and social factors. AI-driven scenario analysis tools aggregate massive data sets—ranging from macroeconomic indicators and central bank policies to geopolitical tensions and climate risks—to provide a more holistic view of potential market trajectories. These systems simulate how portfolios would perform under various hypothetical situations, helping managers anticipate challenges and reposition assets proactively. By improving foresight and strategic planning, AI empowers investment teams to navigate uncertainty with greater confidence.
16. Adaptive Strategies in Evolving Markets
AI models can continuously learn and adapt to shifting market dynamics.
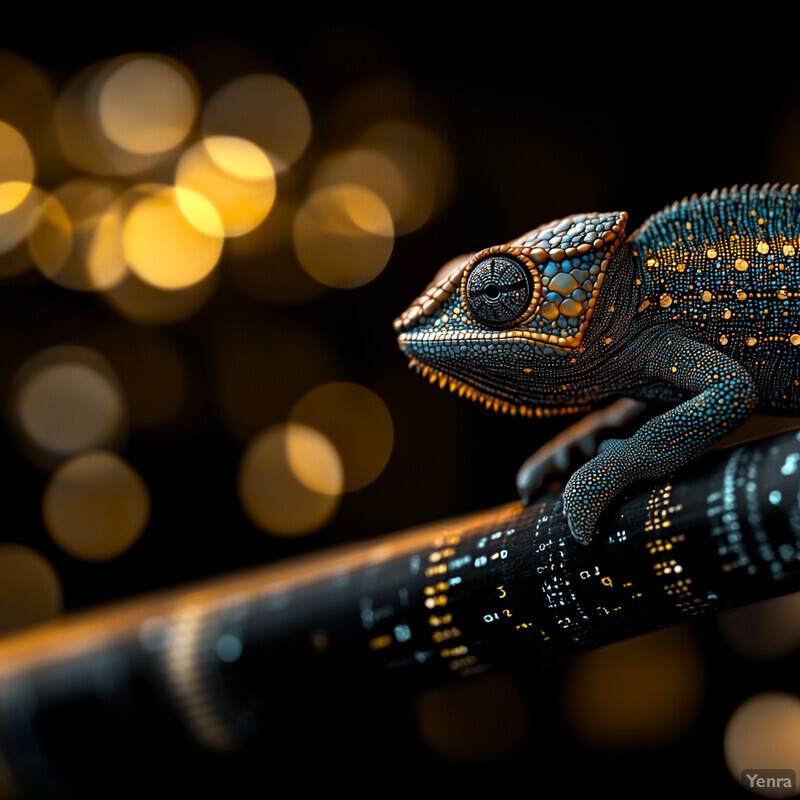
Markets are not static; the parameters that guide sound investment decisions today may not hold true tomorrow. AI models excel at continuous learning, updating their understanding as new information emerges and conditions shift. This adaptability enables strategies that evolve in tandem with the market, whether it’s adjusting to new sources of volatility, recognizing the rise of alternative asset classes, or capturing momentary arbitrage opportunities. By embracing a learning-based approach, asset managers can maintain an adaptive edge, staying relevant and competitive over the long term.
17. Improved Benchmarking and Performance Measurement
Advanced analytics can provide more granular insights into portfolio performance, including factor attribution and anomaly detection, improving benchmarking and strategy refinement.
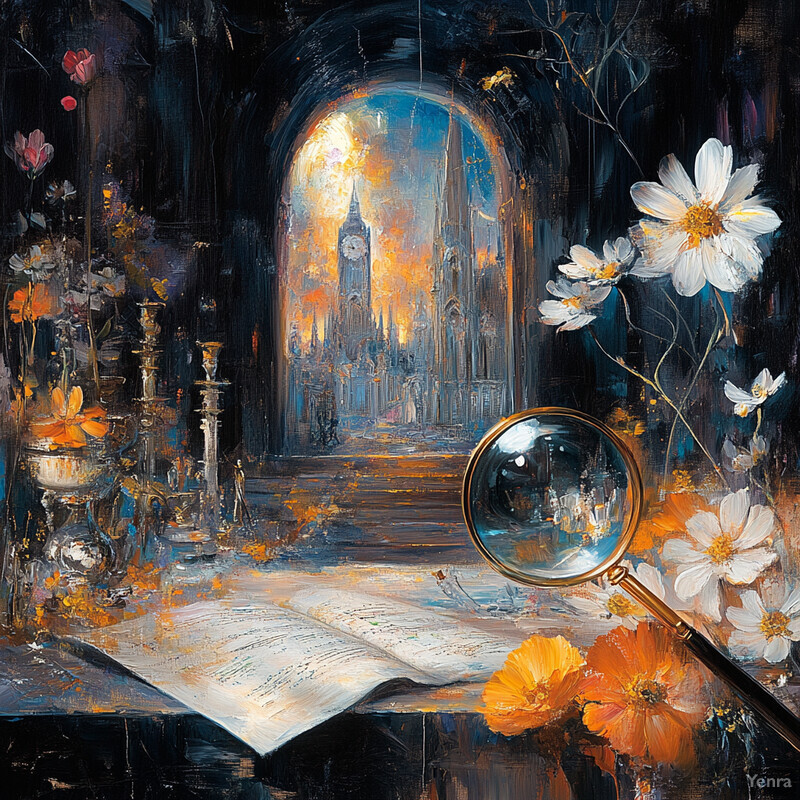
Assessing how well a portfolio performs relative to benchmarks or competitors is crucial. AI-driven analytics can parse performance data at a granular level, attributing results to specific factors, sectors, styles, or market conditions. By identifying where excess returns come from—or where shortfalls occur—managers can refine strategies more effectively. Furthermore, these insights help managers select appropriate benchmarks, understand the drivers of alpha, and communicate performance results more transparently to clients and stakeholders.
18. Enhanced ESG (Environmental, Social, Governance) Analysis
AI-based text analysis and data aggregation tools can help investors evaluate ESG factors by mining sustainability reports, regulatory filings, and media sources.
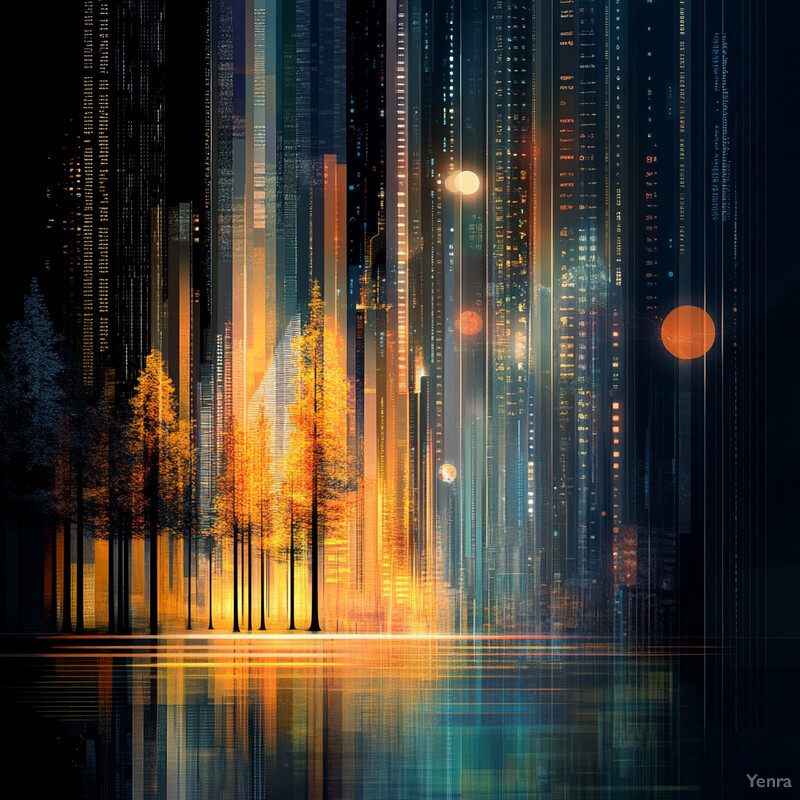
Sustainability and corporate responsibility are increasingly central to investment mandates. AI-enabled ESG analysis tools synthesize data from sustainability reports, regulatory filings, satellite imagery, and media coverage to provide a comprehensive view of a company’s ESG profile. These tools move beyond simple ESG scores and into detailed breakdowns of a firm’s environmental impact, supply chain ethics, and board independence. With richer ESG insights, managers can invest in companies that align with clients’ values, manage reputational risks better, and meet evolving regulatory and societal expectations.
19. Real-Time Strategy Execution Monitoring
Machine learning models integrated into order management and execution systems allow for continuous monitoring of strategy effectiveness.
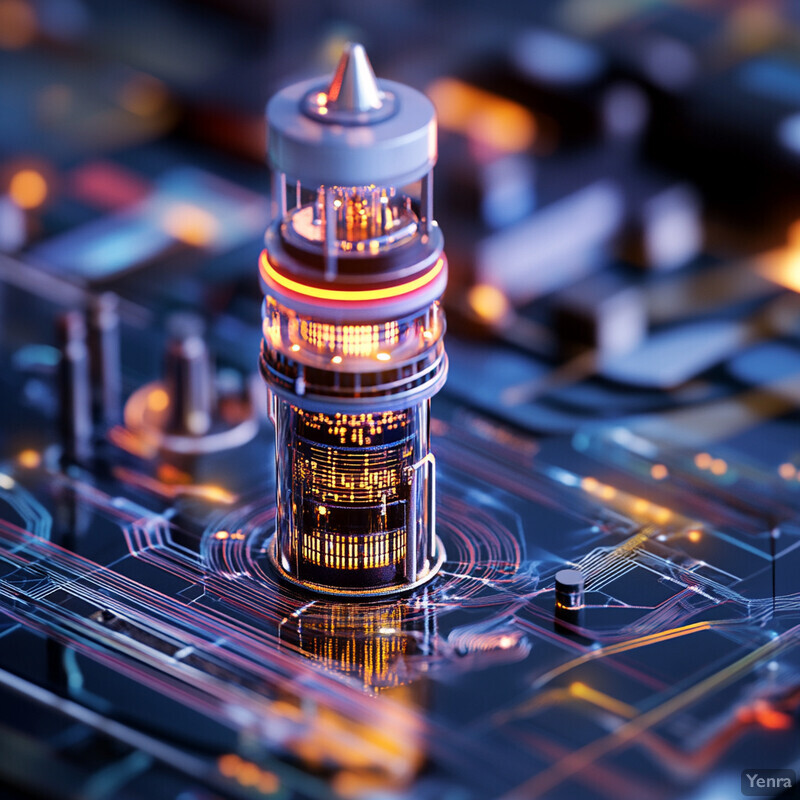
Once an investment strategy is set in motion, continuous oversight is essential to ensure it remains effective. AI systems integrated into order management and execution platforms track real-time performance metrics, market conditions, and deviation from expected models. If the system detects unusual patterns—such as sudden liquidity drying up or price movements inconsistent with historical models—it can alert managers or automatically adjust trading parameters. This level of vigilance helps maintain strategy integrity and ensures that portfolios remain aligned with desired outcomes, even in rapidly shifting markets.
20. Client Engagement and Advisory Services
Virtual assistants and chatbots powered by AI can provide instant, data-driven answers to client queries about their portfolios, market conditions, or investment strategies.
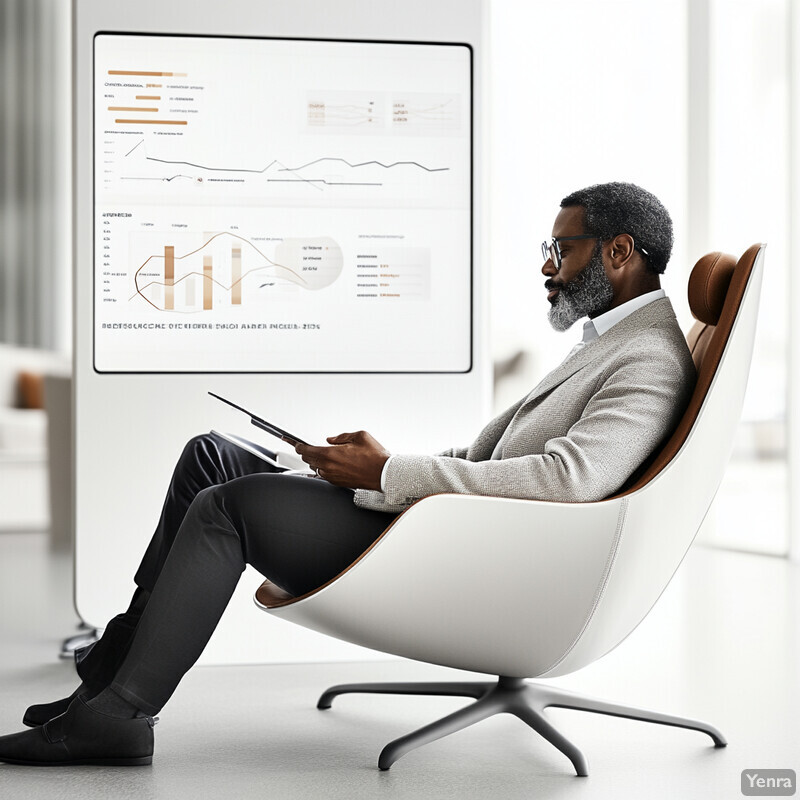
Investors increasingly demand transparent, timely, and personalized communication with their asset managers. AI-driven virtual assistants and chatbots can rapidly address client inquiries about portfolio performance, market conditions, or the rationale behind recent trades. By delivering insightful, data-backed explanations, these tools enhance the client experience and build trust. Additionally, AI can anticipate client needs—suggesting portfolio adjustments before a meeting or providing educational materials tailored to their investment profiles. The result is a more responsive and engaging advisory process that strengthens client relationships.