1. Computer Vision-Based Sorting
AI-driven computer vision systems use cameras and machine learning models to identify and classify recyclables by material type (e.g., plastic, paper, metal) or color and shape. These systems are trained on large image datasets of waste items, enabling them to recognize objects (bottles, cans, etc.) automatically. In practice, computer vision sorters run at high speed (often real-time or near-real-time) and can operate continuously without fatigue, increasing overall throughput. They improve sorting consistency by reducing reliance on human visual identification, which can be error-prone. By quickly directing items to the correct conveyor or bin, vision-based sorting boosts purity and yield of sorted materials. Many recycling facilities worldwide already deploy vision AI to augment traditional sorting lines.
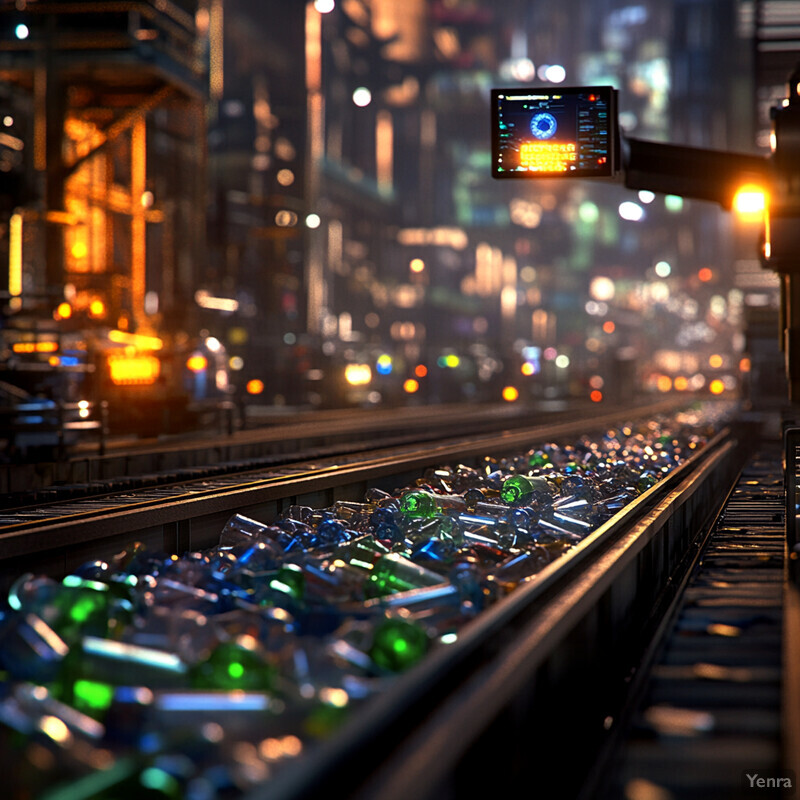
Recent studies demonstrate that deep learning models can achieve high accuracy in waste classification. For example, Sayem et al. (2025) trained convolutional neural networks on 28 recyclable categories and achieved about 83.1% classification accuracy (and 63% detection mean average precision). In commercial systems, AI vision devices have been reported to identify on the order of 90 different material types in under 60 milliseconds per item. A comprehensive review notes that AI-enabled sorting can reach identification accuracies from roughly 72.8% up to 99.9%, depending on the waste stream and method used. In one year, a deployment of AI vision analyzers processed roughly 40 billion waste objects worldwide, gathering data on millions of tons of recyclables. By analyzing visual features (color, shape, logos, etc.), these systems help ensure that mixed recyclables are correctly separated, thereby reducing cross-contamination and improving the overall efficiency of recycling operations.
2. AI-Guided Robotic Arms
Robotic arms equipped with AI perception can automatically pick, sort, and place waste items on conveyor lines. Guided by vision systems, these robots learn to grasp objects of varying shapes and weights. They can handle tasks like removing incorrectly sorted items (quality control) or transferring materials to different lines. The arms use deep learning to adapt to different waste streams and can be retrained as the composition of recyclables changes. By automating repetitive picking tasks, AI-robotic systems reduce manual labor needs and minimize exposure to hazards. They work faster and more consistently than humans in many cases, and can be redeployed to new tasks with software updates.
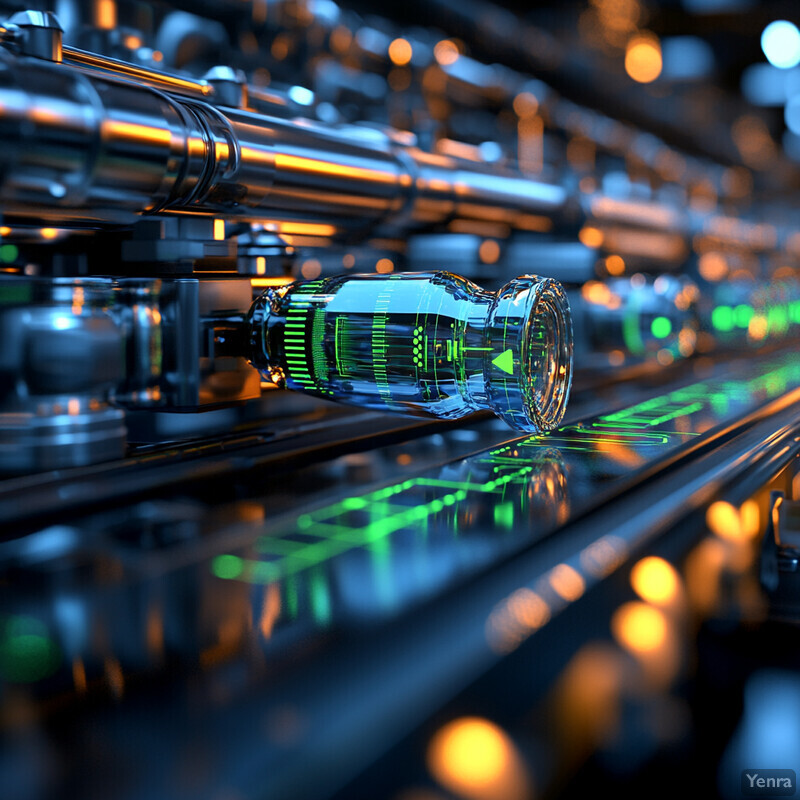
Research and industry reports show that AI-controlled robots can significantly improve sorting performance. For instance, a 2023 review found that robotic waste-sorting systems “increase the speed and accuracy of sorting while reducing the need for manual labor”. These systems also “significantly increase waste management effectiveness” and reduce labor costs by automating heavy lifting and repetitive tasks. In practice, one AI vision system can interface with robotic arms via networked controllers: the vision module identifies items and the robots pick them with millimeter precision. Experiments have demonstrated remarkably high recognition rates: for example, an AI-enabled sorting robot achieved 99% correct identification of plastic bottle types in lab tests. In simulated or pilot deployments, robotic arms with suction-grippers, coupled with AI vision, have sorted containers (PET, glass, aluminum) at high throughput and near-human speed, enabling facilities to replace or augment manual quality-control lines.
3. Advanced Object Recognition for Plastics
AI systems are being developed to distinguish between many types of plastics beyond just “plastic” vs. “metal.” By analyzing color, shape, and even surface texture (often with specialized sensors), these models can separate, for example, PET bottles from HDPE jugs or PP containers from LDPE film. This fine-grained recognition improves the quality of sorted plastic streams, allowing each resin type to be recycled more effectively. Such systems may incorporate hyperspectral or near-infrared sensing data for chemical identification when visual cues are insufficient. The result is more uniform plastic bales and higher purity recyclate, which in turn makes the recycled plastic more valuable.
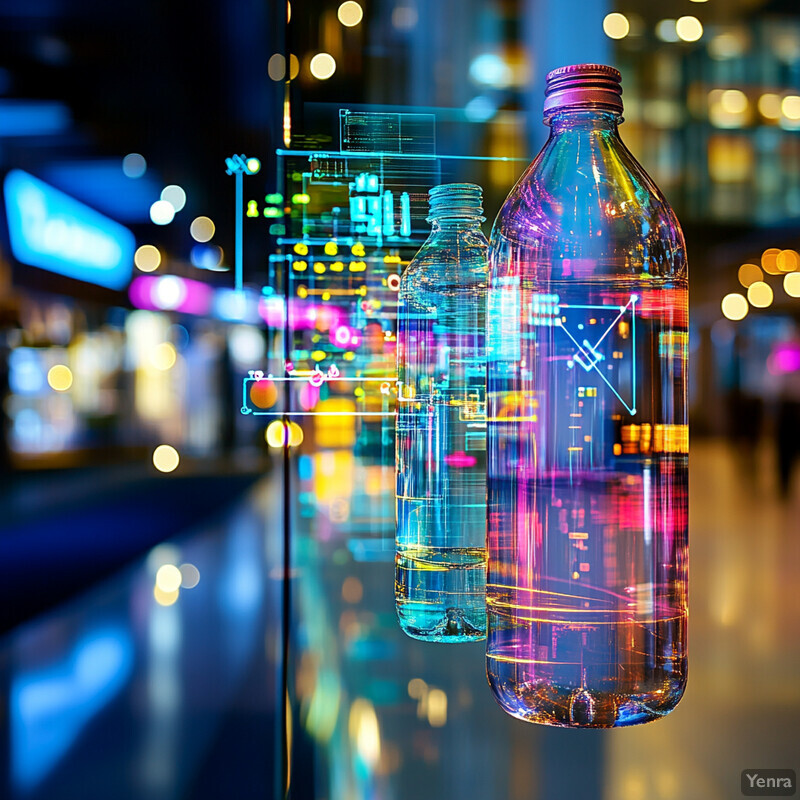
Studies and reviews indicate that recognizing specific resin types is challenging but improving with AI. For instance, one 2025 analysis notes that many MRFs now combine optical AI detection with near-infrared sensing: companies (like AMP Robotics and Greyparrot) use NIR to detect resin codes (e.g., distinguishing PET versus PVC) after vision identifies an object’s outline. However, purely vision-based methods can struggle with black or transparent plastics: Maheshkar et al. (2025) report that optical recognition alone has “limited success” on real-world plastics because color and shape often overlap between materials. To address this, researchers have applied hyperspectral imaging and deep learning, achieving high accuracy in lab conditions. One case study using image and spectral features reported classification accuracies on the order of 90% or higher for common plastics like PET, HDPE, and PP (though performance can drop for soiled or dark plastics). In practice, facilities often blend AI methods: items first pass under a camera system, and ambiguous items (e.g. dark plastics) are sent through NIR scanners. This hybrid approach is reported to yield the best results for modern recycling operations.
4. Detecting Contamination in Real-Time
AI-enabled sensors can spot contaminants (like food waste, non-recyclable items, or moisture) in the recycling stream as it moves along a conveyor. By analyzing spectral signatures, image patterns, or other sensor data in real time, these systems flag items that don’t belong in a given recyclable category. For example, a vision sensor might detect a greasy smear on paper or a wet bag in plastic. When contamination is identified, robotic blowers or arms can eject the item or send it to a landfill stream. Real-time contamination detection helps keep sorted materials clean and reduces the need for re-sorting later. It also supports quality control: managers get alerts about high-contamination loads and can adjust upstream processes or public outreach.
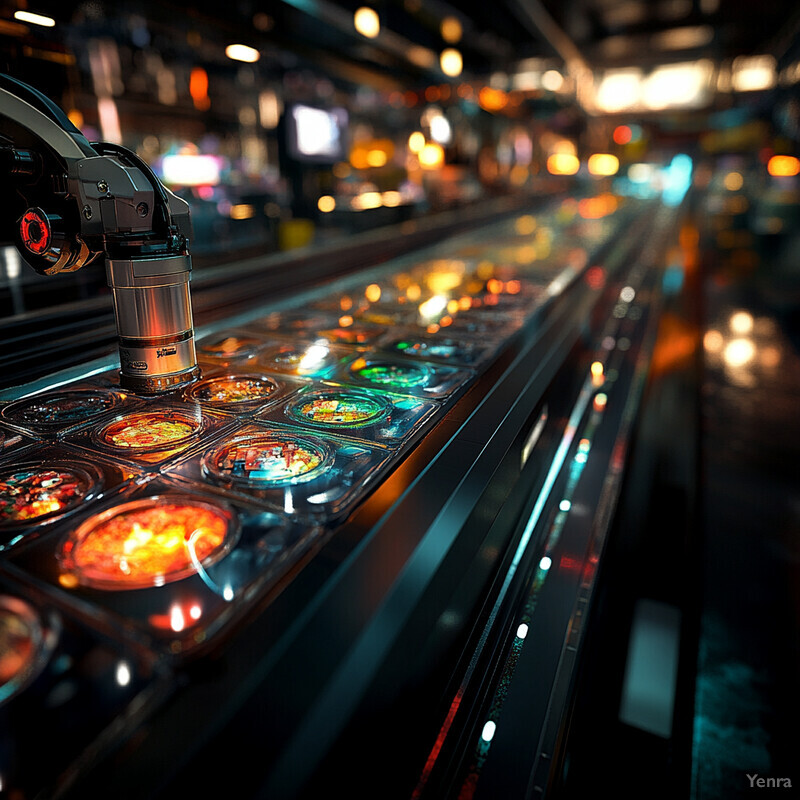
Recent research shows hyperspectral AI can detect very small levels of contamination with high precision. For example, Taneepanichskul et al. (2025) used hyperspectral imaging and machine learning to identify food-contaminated packaging. They report identification accuracies up to 99% for distinguishing plastics with and without food residue. This means an AI camera could virtually spot a bit of peanut butter on a plastic film as it passes by. Moreover, reviews of intelligent sorting note that “intelligent garbage sorting can improve recycling efficiency and reduce contamination” compared to manual sorting. In practical terms, operators using AI have observed that contaminant detection allows faster removal of problem items: an industry report notes AI systems can “spot contaminants early” and flag hazardous or non-conforming materials for removal before they derail the process. By minimizing contaminated loads (e.g. wet fibers, hazardous debris), these AI tools help maintain high-grade output and regulatory compliance.
5. Predictive Maintenance of Sorting Equipment
AI-driven predictive maintenance analyzes sensor data from conveyors, screens, motors, and other equipment to forecast when maintenance or repairs will be needed. For example, vibration and power sensors can feed machine-learning models that detect unusual patterns indicating wear or impending failure. By predicting issues before breakdowns occur, these systems allow scheduled servicing during planned downtime, avoiding costly unplanned stoppages. This keeps sorting lines running continuously at high capacity. Predictive maintenance extends equipment life and reduces emergency repair costs. Many AI platforms now offer analytics dashboards that alert operators to specific parts likely to fail soon, allowing inventory of replacement parts to be managed proactively.
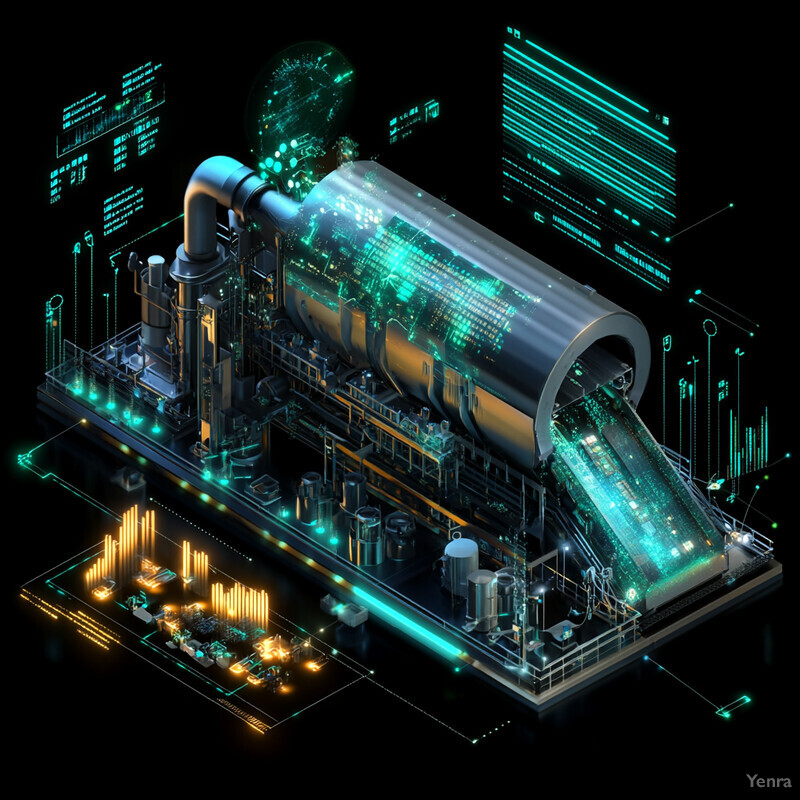
Both research and industry reports underline the efficiency gains from AI maintenance. For instance, one review highlights that AI can be used “to anticipate when waste-sorting equipment will require maintenance, reducing downtime and extending equipment lifespans”. In real-world recycling facilities, AI algorithms monitoring motors and conveyors have indeed led to fewer unexpected shutdowns. An industry case study notes that with AI-based monitoring, unscheduled equipment shutdowns were significantly reduced, keeping operations near full capacity. Similarly, a corporate white paper reports that AI maintenance systems have “emerged as a game-changer” for Material Recovery Facilities, enabling operators to prevent “costly downtime” by identifying faults early. Overall, companies find that condition-based predictions (powered by AI) cut maintenance costs and prevent throughput losses, which is critical for 24/7 recycling plants.
6. Waste Composition Analysis
AI systems can analyze the material composition of incoming waste streams or residual reject piles. By running cameras or spectrometers over moving loads of waste, they quantify what percentage is paper, plastic, metal, glass, etc. This real-time analysis helps facilities understand exactly what they are receiving and can guide sorting priorities. For example, if AI analysis shows a surprisingly high metal content in residue, operators might divert that stream to scrap metal recovery. Over time, tracking this data helps managers optimize the entire plant – from adjusting sort rules to estimating future supply of each material. Composition data can also inform municipal programs (e.g. adjusting curbside campaigns if cardboard drops) and supply procurement for recycling markets.
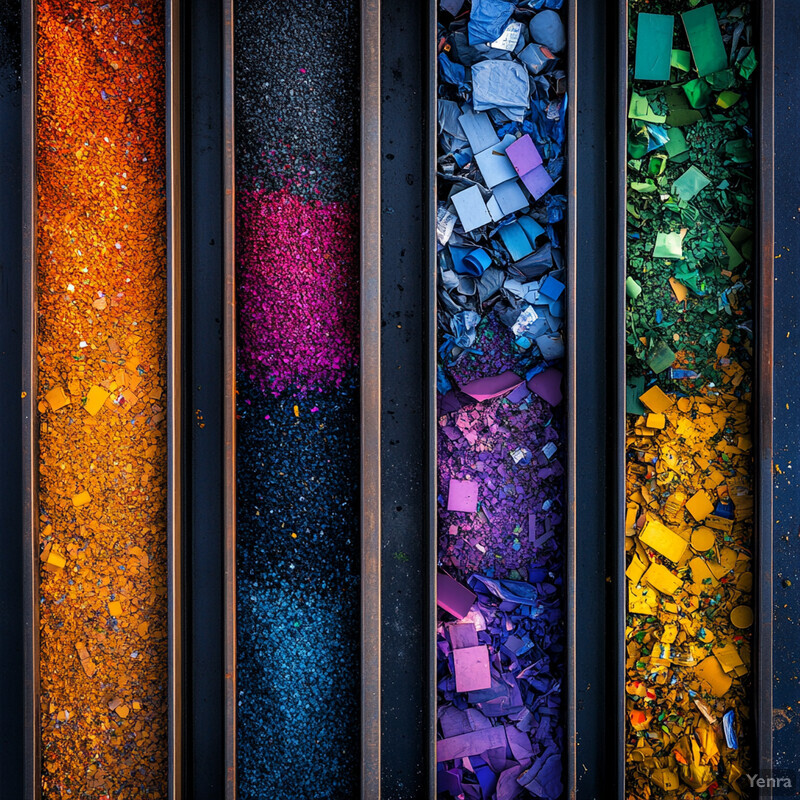
Industry data illustrates how AI delivers detailed composition insights. For instance, a large waste intelligence deployment analyzed residual “reject” lines from multiple facilities and quantified global trends: their AI identified over 3.1 billion PET bottles, 3.3 billion aluminum cans, and 7.4 billion paper/cardboard objects processed in one year. In that analysis, the average residue composition was about 37% paper/cardboard, 26% recyclable plastic, and 3% metal. Facilities use such analytics to find hidden value: one example report notes customers used analyzer data to “guide infeed blends” (i.e. the mix of incoming materials) and to adjust machinery and processes to improve yield. In practice, plants applying AI composition sensing gain a kind of “omniscience” about their input streams, which leads to incremental recovery of otherwise-overlooked recyclables and informs decisions like when to haul additional batches of a particular commodity.
7. Optimized Routing for Collection Vehicles
AI-powered routing software plans the best pick-up schedules and routes for garbage and recycling trucks. By using historical and real-time data on fill levels, traffic, and weather, AI optimizes which containers to empty and when. This can mean dynamically re-routing trucks to avoid congestion or to handle priority bins. The system also balances vehicle workloads and can incorporate variable-bin strategies (e.g. more frequent pick-ups in high-demand areas). The result is minimized total distance traveled and more consistent service frequency. When bins have IoT sensors, AI uses those signals to fill trucks only when needed, further improving efficiency.
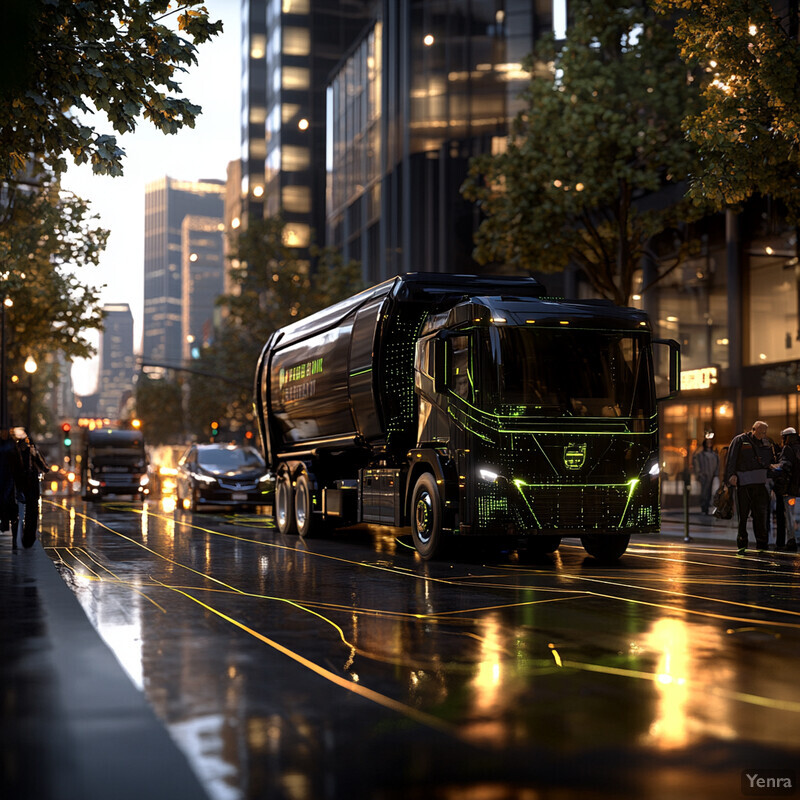
AI-enhanced routing has been shown to cut operational costs significantly. For example, a case study in Switzerland used adaptive AI algorithms to re-plan collection routes daily. Compared to static (pre-set) routes, the AI plans reduced travel distances by about 24% in one city and 38% in another, which correlates to similar reductions in fuel use and CO₂ emissions. In many cities, simulation and pilot projects report that AI route optimization lowers fuel and maintenance costs while still meeting collection targets. One technical review notes that AI-based route planning can incorporate factors such as “traffic, weather, and population density” to improve overall waste collection efficiency. Municipalities adopting these tools report saving drivers’ time and reducing truck wear. For instance, in one deployment, vehicles needed far fewer trips through residential areas, cutting labor and vehicle hours by over 30% (proportional to the distance saved).
8. Automated Inventory Management
AI tools can predict and manage the inventory of recyclable materials inside a facility. For example, by analyzing trends in inbound loads and outgoing baled material, AI forecasts how much of each commodity (like aluminum cans or PET bottles) will be stored or shipped. This helps recycling plant managers plan sales of sorted material and schedule shipments to mills. It also applies to reordering consumables or spare parts: if AI sees that fiber throughput is dropping, it might indicate a wrapper machine fault or a downstream jam. On a higher level, when multiple facilities share data, AI can balance supply and demand regionally – for instance, sending extra bottles from one plant to another that is short on PET inventory. Overall, AI-driven inventory analytics ensure that operations are balanced and materials are not left idle in storage for too long.
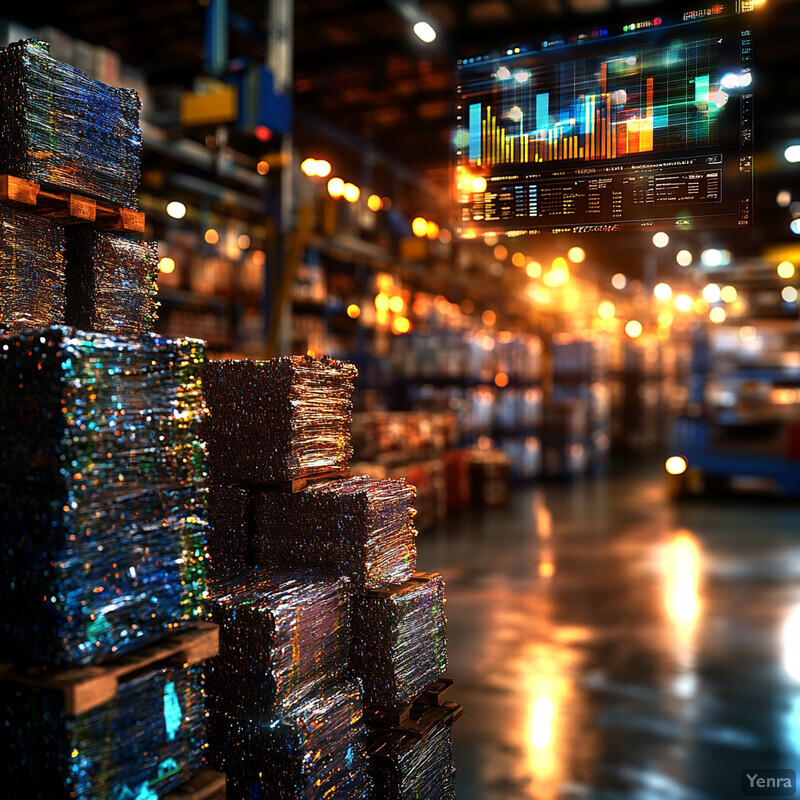
Emerging waste intelligence platforms now capture 100% of material movements entering and exiting facilities, enabling visibility into inventory flows. One industry blog reports that plants using AI “identify every object entering and exiting” so that managers can track cumulative totals of each material by day or month. This data is then used for operational adjustments: for instance, customers have used AI analytics to “guide everything from infeed blends to machinery adjustments”, which by extension includes inventory planning. Although academic literature on AI forecasting of recyclables inventory is sparse, these AI tools effectively create a real-time inventory report for recyclables. They can suggest, for example, when to hold or release certain bales. One report from a recycling technology firm noted that producers can use sensor data to fulfill reporting requirements for recovery rates, implying coordinated tracking of recycled volumes across facilities.
9. Quality Control and Sorting Line Adjustments
AI provides continuous feedback on sort-line performance and allows quick adjustments. For example, if an AI sensor detects an unexpected type of material passing through (say, more glass than usual), it can automatically tweak a robotic picker’s target. Facilities can retrain vision models or reassign robot arms when the waste mix changes. AI also monitors the sorted output quality: it can measure the purity of sorted bales and compare it to targets. When quality drifts, the system alerts operators to adjust conveyor speeds, air nozzle timing, or conveyor divisions. In short, AI acts like an automated quality inspector: it “looks at” every item in real time and tunes the sort process to maintain high output standards.
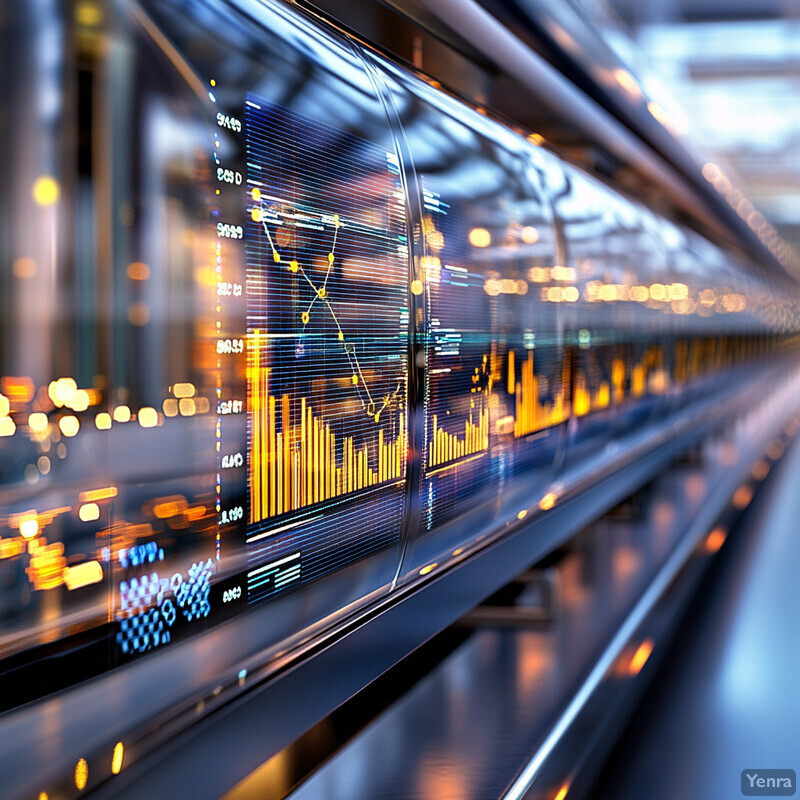
Case studies show AI aiding line control. In one modern Material Recovery Facility, AI cameras on “last chance” quality-control belts sorted up to 15 different categories automatically, flagging contaminants that sensors missed earlier. The system is highly flexible: for example, a recycling plant reported retraining its vision models when the incoming mix shifted, doubling the capture of aluminum cans by simply swapping neural network weights and redefining robot pick targets. Importantly, AI sorting provides full visibility: the vision system scans 100% of items and outputs near-real-time reports of material composition and equipment performance. This means plant managers can see, for instance, that on a given day the conveyor is yielding 90% plastics, 7% metals, etc., and identify any bottleneck. Such instant feedback lets engineers fine-tune sorting diverts or clean out jammed sections quickly, preventing buildup of errors down the line.
10. Dynamic Pricing Recommendations
AI can help recyclers set dynamic prices for inbound materials or sales of sorted commodities by analyzing market and quality data. By tracking real-time commodity prices (e.g. daily index of recycled aluminum or PET), the AI can suggest price adjustments for materials offered by customers or bids for materials. It also factors in the actual quality and composition of loads: for example, a mostly-clean bale of glass might warrant a higher price than a contaminated one. These recommendation engines enable facilities to react quickly to volatile scrap markets and to offer incentives (e.g. bonuses for cleaner material) to generators. Over time, this aligns the economics of recycling with recovery goals.
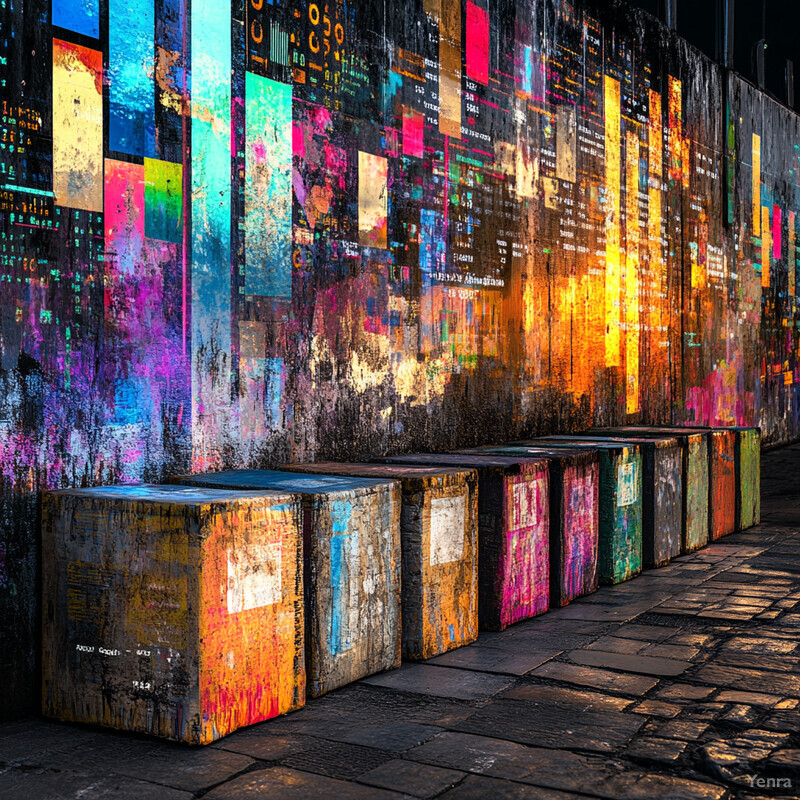
Industry sources indicate that advanced analytics tools are already being used to guide pricing strategies. For instance, a materials consultancy notes that next-generation valuation platforms allow recyclers to “optimize pricing strategies based on precise material composition data”. In other words, if sensor analysis shows a bale is exceptionally pure PET, the AI system might recommend a premium price. Although scholarly studies on AI pricing in recycling are limited, similar concepts are well-established in commodity markets. Modern systems ingest large amounts of scrap-market data (price, demand forecasts, futures curves) alongside quality measures to update prices frequently. One scrap industry report explains that AI tools can dynamically adjust offers or bids to help businesses remain profitable amid metal price swings. In practice, these AI recommendations are used by traders and brokers to time sales; for example, selling a batch of cardboard when pulp prices spike. This dynamic approach helps ensure recyclables are sold at the best time and price, increasing revenue for the recycling system.
11. Brand and Logo Recognition
AI vision can recognize brand logos or packaging patterns on waste items. This enables tracking of specific manufacturers’ packaging or verifying that products are recycled properly under extended producer responsibility (EPR) programs. For example, AI cameras might read a soda company’s logo on bottles, counting how many of that brand are entering the recycling stream. Knowing the brand mix helps companies report on their own packaging waste and target improvements. In a related approach, some products carry invisible digital watermarks (QR codes or UV patterns) that AI scanners read; this tells systems exactly which batch or type of item it is. Overall, logo recognition adds a layer of traceability: it can link waste back to brands, enabling feedback loops from recyclers to producers.
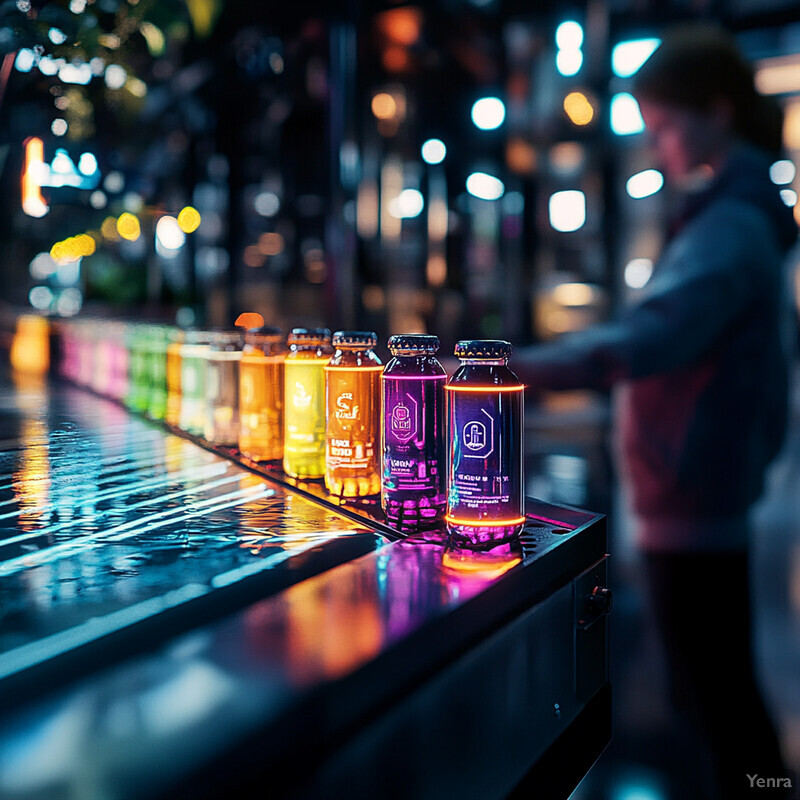
Research projects and industry pilots show this is feasible. For instance, a browser extension project named “Litter Log” collected images of litter and trained an AI model to classify brand logos in those images. Its AI successfully identified brands from crowdsourced litter photos and produced pollution data for those brands. On the commercial side, some retailers (e.g., a UK supermarket chain) are piloting code-based tracking: the company Polytag developed a UV-visible code printed on packaging that an AI camera can detect and read. In trials, this system enabled the retailer to count exactly how many of its own wrapped products were successfully collected for recycling. Thus, AI-driven logo or watermark recognition is being used to create accountability: producers get precise recycling yields for their own items. These systems not only improve data accuracy for recycling rates, but also support targeted design changes in packaging by revealing which branded items remain as litter or contaminants.
12. Micro-Contaminant Detection
AI vision systems can be trained to detect very small contaminants, such as shards of glass, bits of plastic, or even microscopic metal fragments, within waste streams. These tiny objects can damage equipment or lower product quality, so catching them is important. AI algorithms process high-resolution images or sensor signals to spot shapes or spectral signatures of micro-contaminants. For example, underwater cameras with AI have been developed to find microplastics in flowing water. In processing lines, such detection can trigger automatic removal actions (e.g. blowing out the tiny piece). This capability ensures that even minute fragments are identified, improving safety and purity of recycled materials.
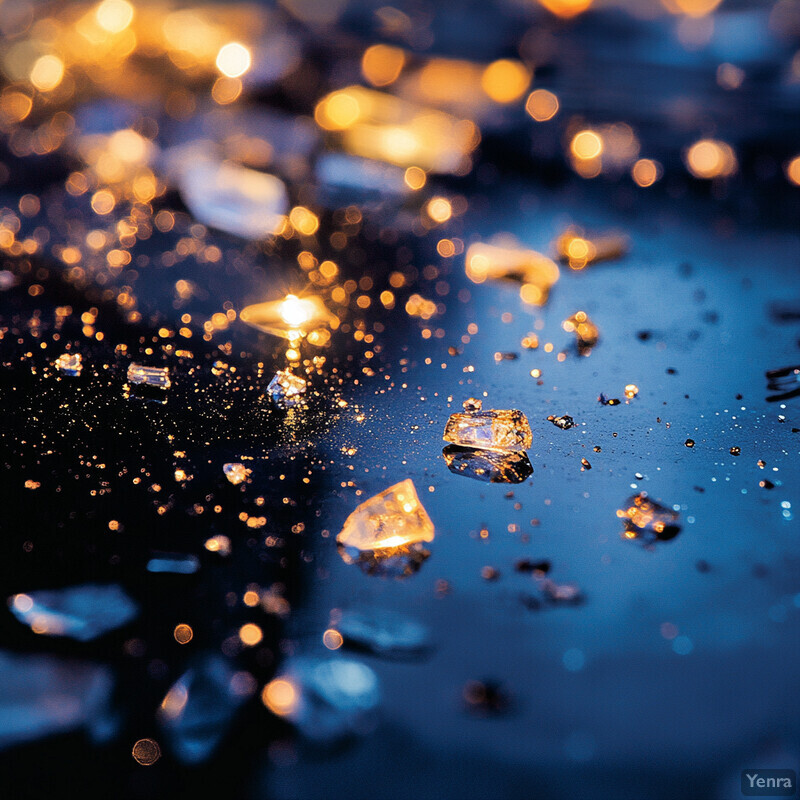
A 2024 study demonstrates AI’s effectiveness at this scale. Sarker et al. developed a specialized camera system and YOLOv5 neural network to detect microplastic particles (≤5 mm) in moving water. In controlled tests, their AI detected particles with 97% precision in the lab and 96% precision in a river environment. In other words, the AI correctly counted microplastics almost all the time in real time. While that study focused on water monitoring, it shows the principle applies to sorting plants as well: AI models can detect small bits amidst debris with very high accuracy. By extension, similar AI vision setups are used on conveyor lines to catch fragments (e.g. glass or metals) that human operators would miss. These high detection rates suggest AI can serve as an automated micro-inspector on the line, preventing tiny dangerous objects from damaging equipment or contaminating final bales.
13. Enhanced Worker Training Tools
Augmented reality (AR) and virtual reality (VR) applications use AI to create interactive training for recycling workers. For example, an AR app can visually guide a user in sorting waste correctly by overlaying prompts on a camera view of real objects. This provides immediate feedback – if a trainee points a device at a plastic bottle, the app can show the correct bin or flag an error. Such tools can simulate complex sorting scenarios that new hires might not frequently see. The visual and dynamic nature of AR/VR makes learning faster and more engaging. These intelligent training aids help improve worker accuracy and speed before they join a live production line.
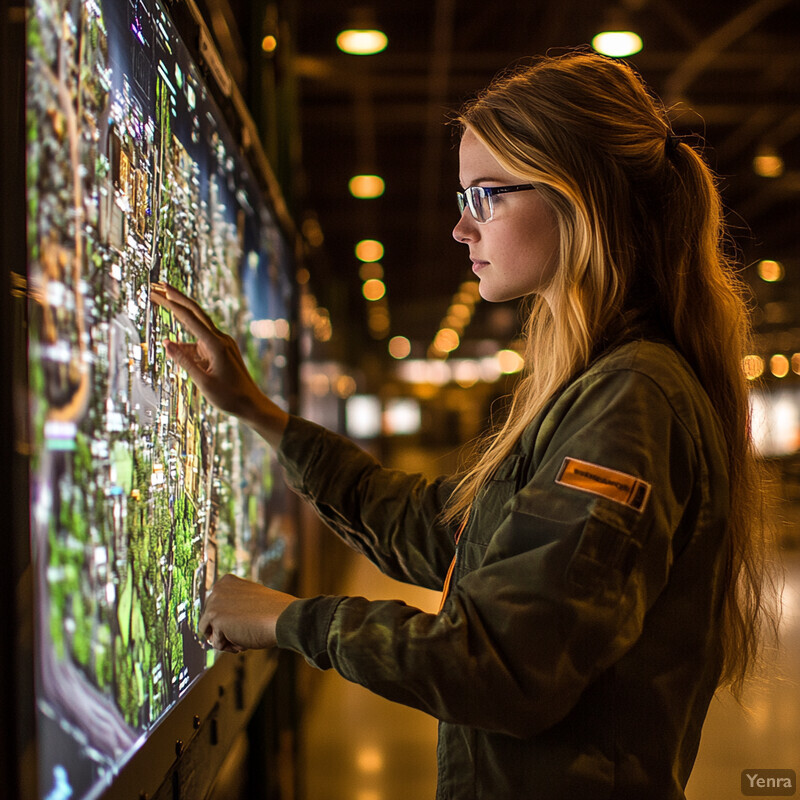
Trials of AI-driven training aids show significant improvements in sorting skills. For instance, Sun et al. (2023) developed an Augmented Reality app (Educational Recycling Assistant) that used object detection to assist users in sorting household waste. In a user study, participants using the AR tool achieved significantly higher waste-bin accuracy than a control group – meaning they threw items into the correct bin more often. The study found that after using the AR assistant, users’ understanding of complex waste categorization improved substantially. Although targeted at home users, this indicates the power of AI-guided visuals to teach correct sorting. Industrial training programs apply similar ideas: VR headsets can simulate factory floors, while AI avatars quiz trainees on contamination scenarios. Overall, data from pilot programs suggest that AI-enhanced training reduces error rates and shortens the time needed for new workers to reach competency, although comprehensive field studies in MRFs remain limited.
14. Automated Compliance Monitoring
AI can continuously ensure recycling outputs meet regulatory and quality standards. For example, sensors and vision systems check that the composition of each bale meets legal requirements (such as no prohibited materials or moisture limits). If a deviation is detected (e.g. an illegal hazardous waste piece or too much organic residue), the system instantly alerts operators or rejects the batch. AI also logs compliance data automatically, simplifying reporting to regulators. This automated monitoring covers both purity (e.g. verifying PVC isn’t in PET bales) and environmental thresholds (e.g. detecting off-spec emissions from machinery). Overall, AI compliance tools act as a virtual inspector, flagging any out-of-spec conditions in real time.
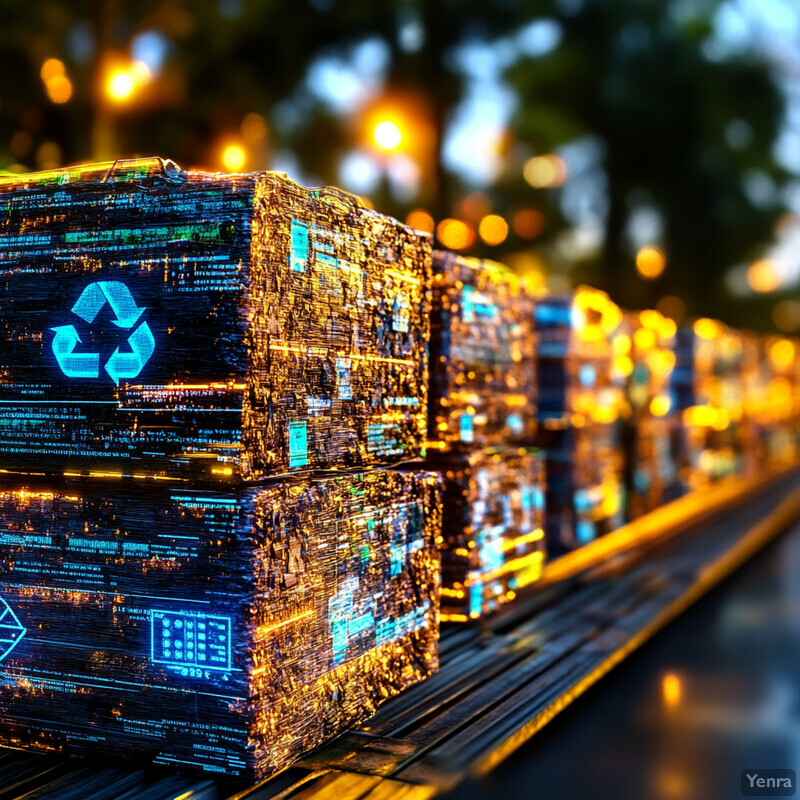
Literature on AI compliance in recycling is emerging. A review of smart sorting notes that intelligent systems can “improve recycling efficiency and reduce contamination,” which is a key step toward compliance. In practice, AI systems are explicitly used to flag forbidden substances: one industry source describes how AI-powered sorters “spot contaminants early” and identify “hazardous contaminants” (such as battery fragments) to remove them promptly. By extension, this capability ensures the final recyclables meet safety and regulatory criteria. For instance, if a stream is supposed to be all aluminum, the AI will alert the operator if any non-aluminum item (e.g. a paint can) appears. Companies report that having AI continuously monitor composition allows them to adjust the process before full loads are off-spec, thus avoiding fines and ensuring each bale complies with quality standards.
15. Resource Use Optimization
AI helps recycling facilities use resources (energy, water, fuel) more efficiently. For example, AI can control equipment power modes or conveyor speeds to minimize electricity consumption during low loads. It can also optimize use of compressed air, water (for wash lines), and other utilities based on real-time needs. In operations, AI algorithms might schedule energy-intensive tasks (like baler compaction) at off-peak hours when electricity costs are lower. When integrated with routing (section 7), AI reduces fuel use for collection vehicles. By holistically balancing inputs and outputs, AI ensures that resource usage is tailored to demand, reducing waste of energy and materials.
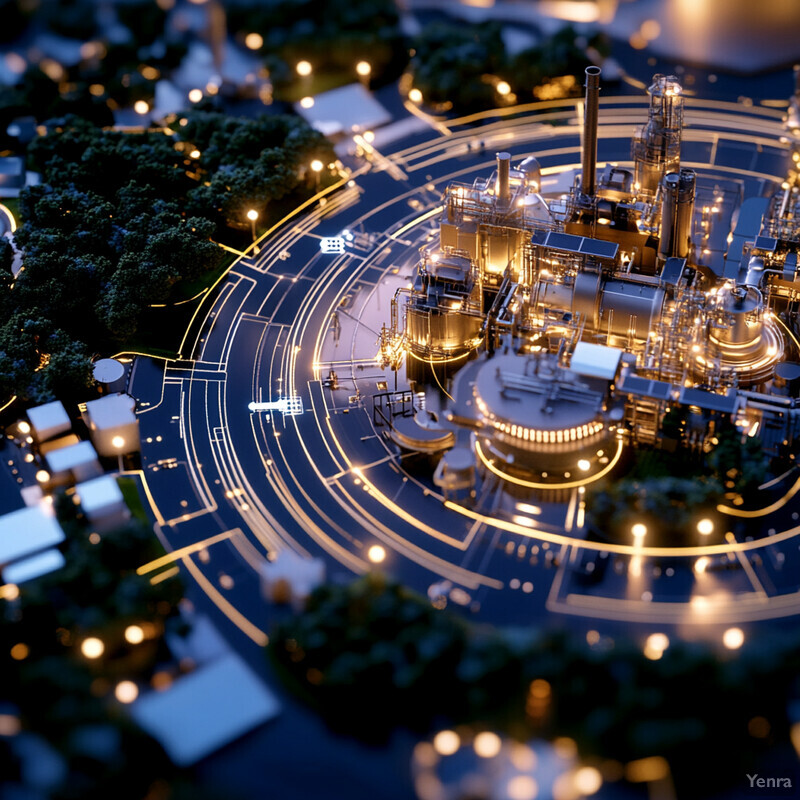
Studies in related industrial settings show significant resource savings. For example, AI-based control of cooling systems in a power plant (a related heavy-use scenario) reduced water withdrawals by about 27% while maintaining performance. Though direct studies on recycling plants are limited, similar principles apply: dynamic control can cut pump or blower usage by double-digit percentages. In waste collection, optimized routes driven by AI have a direct resource impact: one case reduced collection distance by 24–38%, implying a comparable cut in fuel consumption. In resource-intensive sorting centers, predictive scheduling of equipment based on load forecasts can trim electricity peaks. Overall, industry experience suggests that AI-driven resource optimization (coupled with sensors and IoT) can cut operational costs by 10–30% depending on the process and facility, though exact figures vary.
16. IoT Integration for Smart Bins
Internet-of-Things (IoT) sensors in waste and recycling bins allow AI to know when and where collection is needed. Fill-level sensors (ultrasonic or weight-based) send data on how full each bin is. AI platforms collect these signals citywide and trigger pickups only for bins above a threshold, avoiding emptying near-empty containers. Some smart bins even compact waste when sensors detect high fill, under AI control. The sensors also record usage patterns (time-of-day disposal trends) which AI analyzes to forecast demand. By integrating IoT data with routing software, cities optimize collection schedules and eliminate unnecessary truck trips.
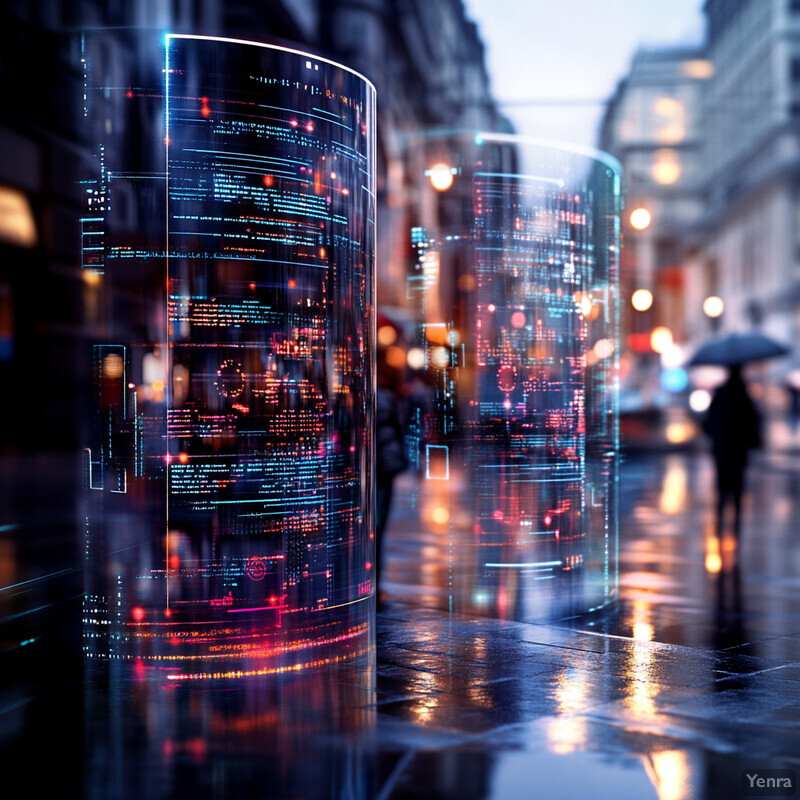
Smart bins with AI-driven monitoring have been demonstrated to greatly improve efficiency. For example, sensors that alert sanitation when a bin is full allow crews to plan fewer stops. One report states that in a pilot project, equipping bins with fill-level sensors enabled routing optimizations that reduced garbage truck trips by 80% within six months in one city. In general, studies of smart waste management note that such IoT data collection “helps analyze waste trends and improve recycling” and reduces labor, fuel, and machine wear by preventing both under- and over-filling. Empirical results include fewer overflow incidents (improving hygiene) and substantial cost savings from fewer vehicle miles driven. AI algorithms use these bin signals continuously to adapt future schedules; one estimate showed that smart bin monitoring systems can cut service mileage by up to one-third, dramatically lowering both CO₂ emissions and collection costs.
17. Landfill Diversion Predictions
AI models can predict how much waste will be diverted from landfills through recycling under various scenarios. By analyzing historical recycling rates, seasonal patterns, and policy changes, these tools forecast future diversion percentages. For example, if a new recycling program is introduced, AI can estimate the increase in diversion based on similar past rollouts. These predictions help planners set realistic goals and adapt strategies (e.g. targeting education campaigns in low-diversion areas). On an operational level, AI uses composition data (from earlier sections) to estimate how much of a given load will be recoverable, thereby predicting the portion that would otherwise go to landfill. These forecasts inform capacity planning for both recyclables processing and landfill space.
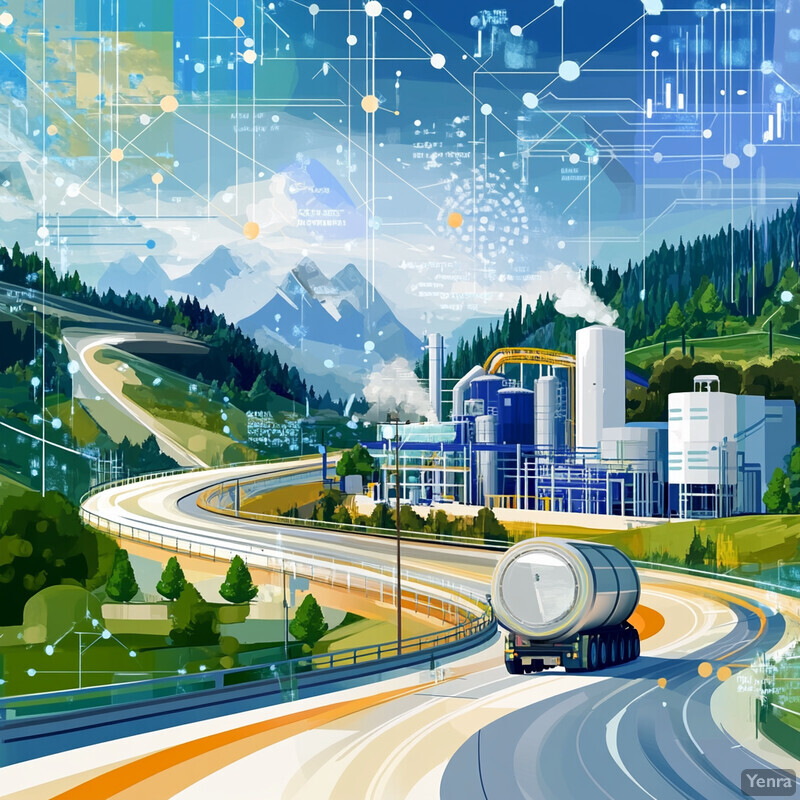
While academic studies on AI forecasting landfill diversion are still emerging, smart-sorting reviews imply significant gains. A comprehensive review notes that intelligent sorting “improves recycling efficiency and reduces contamination”. Though not a direct prediction, increased efficiency directly translates to higher diversion. In industry, one estimate (Forbes, 2024) was that AI could greatly help “divert recyclable materials away from landfills,” though specific figures depend on local factors. For example, an AI-driven sort line that boosts recovery rates from 50% to 80% effectively predicts a large uplift in diversion. Additionally, datasets collected by AI can be used to train statistical models: regions with AI-enabled MRFs have used their data to project that waste diversion could increase by several percent annually under current trends. In practice, many recycling agencies now use data analytics to refine forecasts of landfill-bound waste, often citing machine learning as the method improving their estimates.
18. Cross-Facility Coordination
AI platforms can connect multiple recycling centers and haulers into a coordinated network. By sharing data (e.g. inbound volumes, market prices, performance metrics) across facilities, the AI can balance loads regionally. For instance, if one plant is overfull with cardboard but another nearby is underutilized, the system might redirect trucks to even out inventory. AI can also aggregate data from all sites for central planning: it can identify which locations need more capacity or outreach. In this way, a consortium of facilities can collectively optimize sorting and distribution rather than each acting in isolation. This helps meet broader goals like state-wide recycling targets or corporate sustainability commitments.
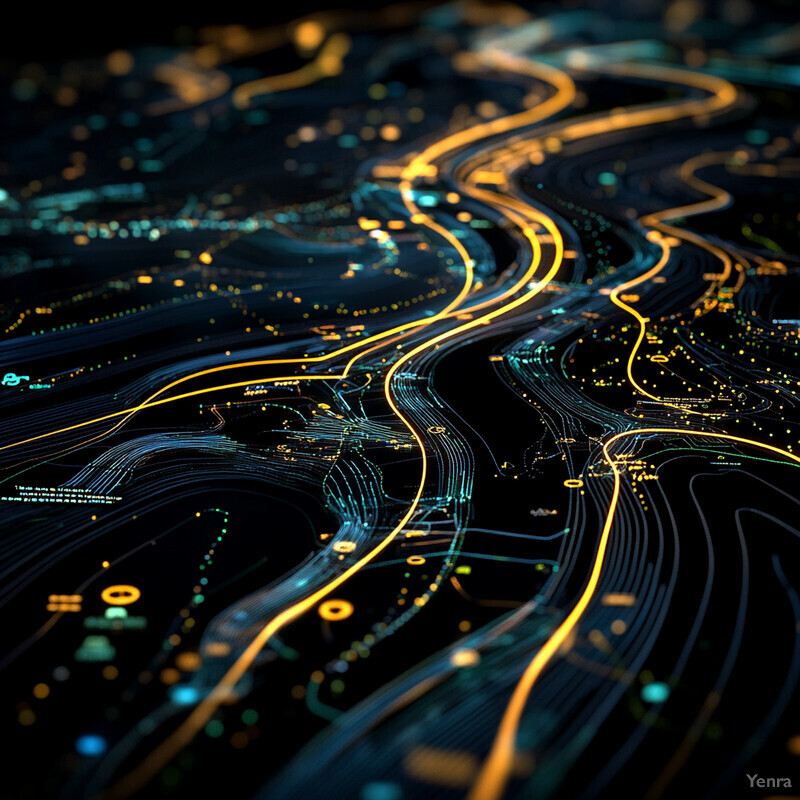
Industry experts highlight that aggregated data from AI systems now informs cross-company initiatives. For example, sensors in multiple MRFs feed data to producers’ Extended Producer Responsibility programs. One report states that growing sensor use “can help satisfy the demand for reporting recovery rates” across regions. Similarly, it notes that collecting granular data on waste flows creates a mechanism to support government landfill diversion goals and recycled-content standards. These statements imply that AI data from many facilities is being combined to track progress. In practice, recycling networks use shared dashboards: an aluminum industry association, for instance, collects monthly sorted-weight data from all member MRFs (often via AI-driven systems) to compute overall diversion rates. While concrete quantitative studies are scarce, the Waste Today industry magazine confirms that better data capture “provide[s] opportunities” for consumer goods companies and regulators to coordinate across facilities.
19. Material Innovation Feedback Loops
Data from AI-powered recycling helps drive innovation in product and material design. By revealing which packaging items are most often found as contaminants or low-quality recyclate, AI insights tell manufacturers what to redesign. For example, if vision analytics show that a certain plastic wrapper is always mis-sorted or ends up in the wrong stream, companies can phase in an easier-to-recycle alternative. Generative AI tools are also being applied directly to design: they can propose new packaging shapes or materials optimized for both function and recyclability. The feedback loop closes when data on recycled material properties is fed back into the design cycle. Over time, this iterative process (fed by AI analytics) results in products that are easier to sort and recycle, reducing waste at the source.
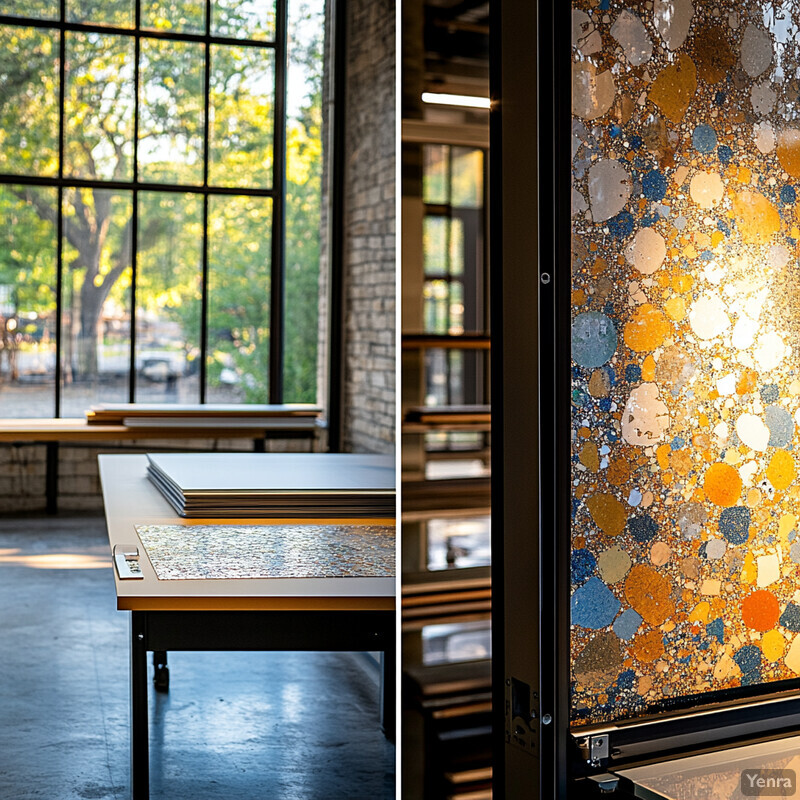
Recent industry trends illustrate this feedback. Packaging companies are now using AI-driven design platforms to explore sustainable alternatives. For example, generative AI systems can “create optimized packaging designs” and “suggest green packaging materials” that meet strength and cost requirements. These tools can run hundreds of virtual simulations to minimize waste (e.g. less excess material) before any prototype is made. Dataforest, a consulting firm, reports that with AI “packaging companies can instantly adjust designs based on available materials” and find eco-friendly solutions without sacrificing durability. This means a manufacturer could alter material composition if sensors show poor recycling performance. Although scholarly papers are few, the packaging industry cites AI as a key enabler of next-generation sustainable design. In one example, a major paperboard producer used AI optimization to reduce material use by 10% while maintaining strength, based on recycling quality data. In summary, AI-driven design tools, when combined with recycling analytics, are actively creating feedback loops that enhance material innovation in favor of circularity.