1. Enhanced Target Detection in Low SNR Environments
AI-driven algorithms can more effectively detect weak signals buried in noise and clutter, significantly improving radar sensitivity and range resolution.
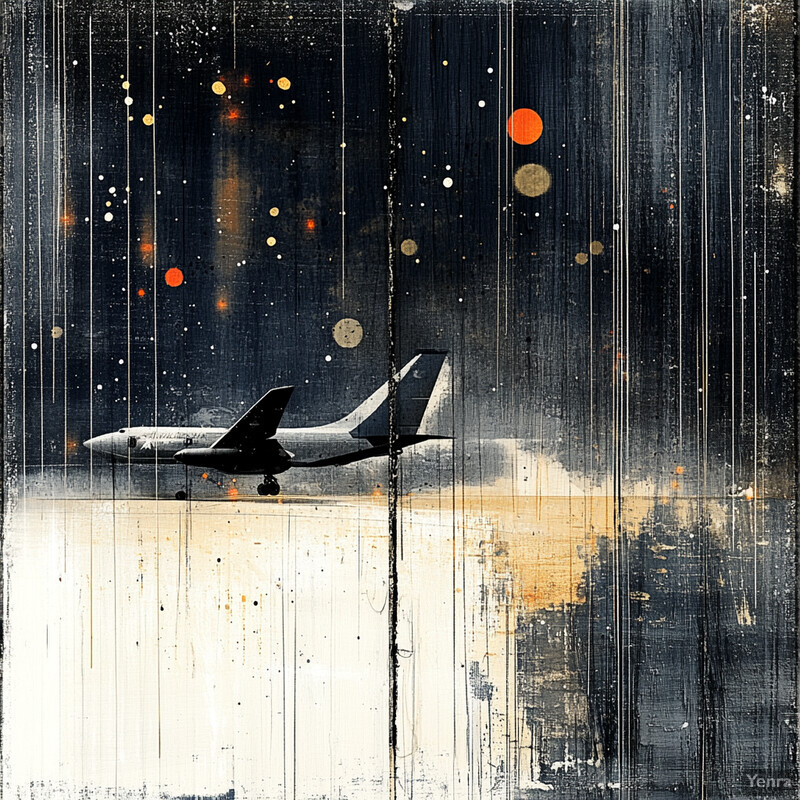
In scenarios where targets produce extremely weak echoes that are almost lost within the noise floor, AI-driven algorithms—especially those leveraging deep learning—are proving invaluable. Traditional detection methods rely heavily on linear filtering techniques and static thresholds, which can fail in complex and noisy environments. By using neural networks and adaptive models that learn patterns from training data, these AI methods can identify subtle signal features and extract targets that exhibit minimal energy. This capability allows radar systems to extend their effective detection range, ensuring that even low-visibility objects, such as stealth aircraft or small drones, are more readily identified and tracked.
2. Advanced Clutter Suppression
Deep learning models can learn complex clutter patterns—such as ground returns, weather phenomena, or sea clutter—and accurately distinguish targets from these non-target echoes.
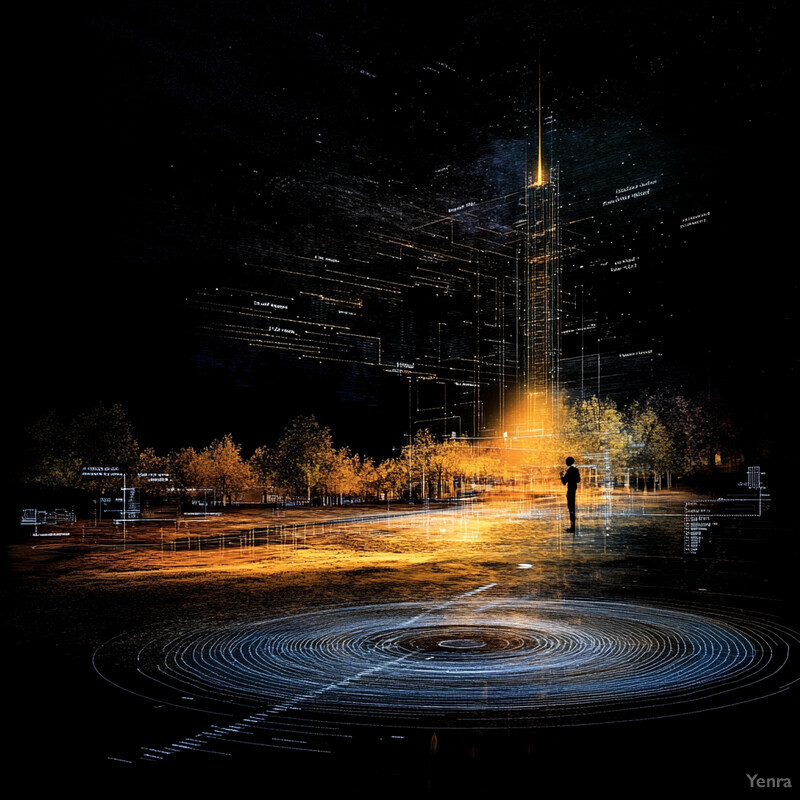
Clutter, arising from ground returns, weather phenomena, sea surface reflections, or even environmental objects, can overwhelm radar systems and obscure desired targets. AI helps here by learning the statistical profiles and temporal-spatial characteristics of clutter, enabling more nuanced classification and rejection strategies. Instead of applying fixed clutter-suppression filters, adaptive AI models continuously update their understanding of the radar scene. By differentiating between benign returns and genuine targets, these intelligent algorithms reduce false alarms, enhance overall detection performance, and enable more reliable operation in challenging environments.
3. Automated Target Classification and Recognition
Machine learning techniques can classify returns into different target categories (aircraft, UAVs, missiles) based on subtle signal signatures, streamlining intelligence and threat analysis.
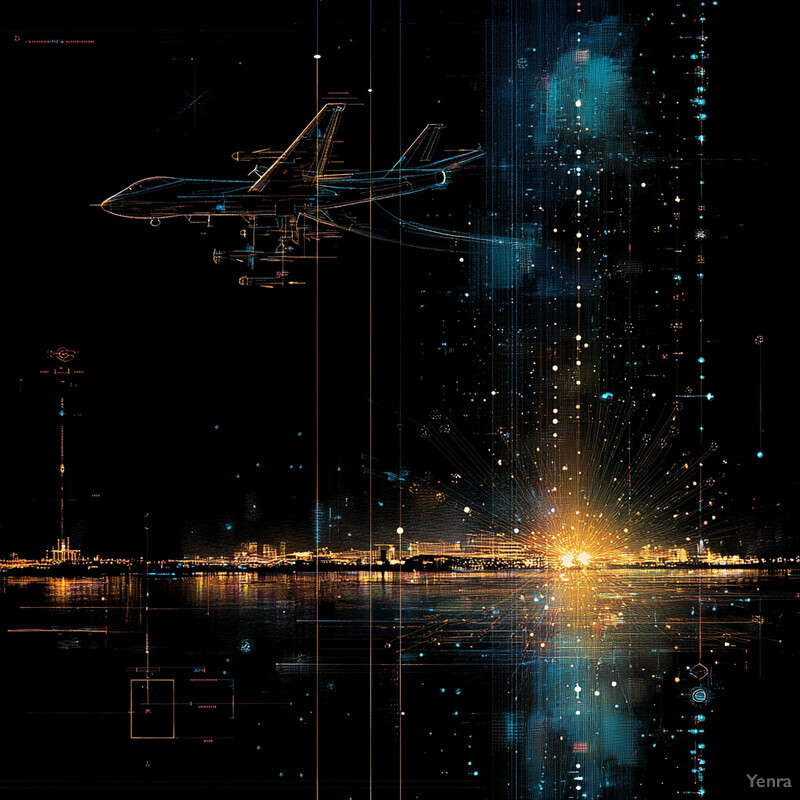
Identifying what type of object a radar return corresponds to—whether it’s an aircraft, an unmanned aerial vehicle, or a missile—is a crucial aspect of situational awareness. AI-driven classification models draw upon large datasets of radar signatures to learn distinct features associated with different object classes. Using convolutional neural networks and other advanced architectures, these systems can match real-time returns to known profiles in microseconds. The result is faster, more accurate target identification and improved decision-making support, which is especially valuable for defense and security scenarios where response time and target discrimination can mean the difference between mission success and failure.
4. Intelligent Waveform Design and Adaptation
Reinforcement learning methods can dynamically select or design radar waveforms on-the-fly to optimize performance under varying environmental conditions and mission objectives.
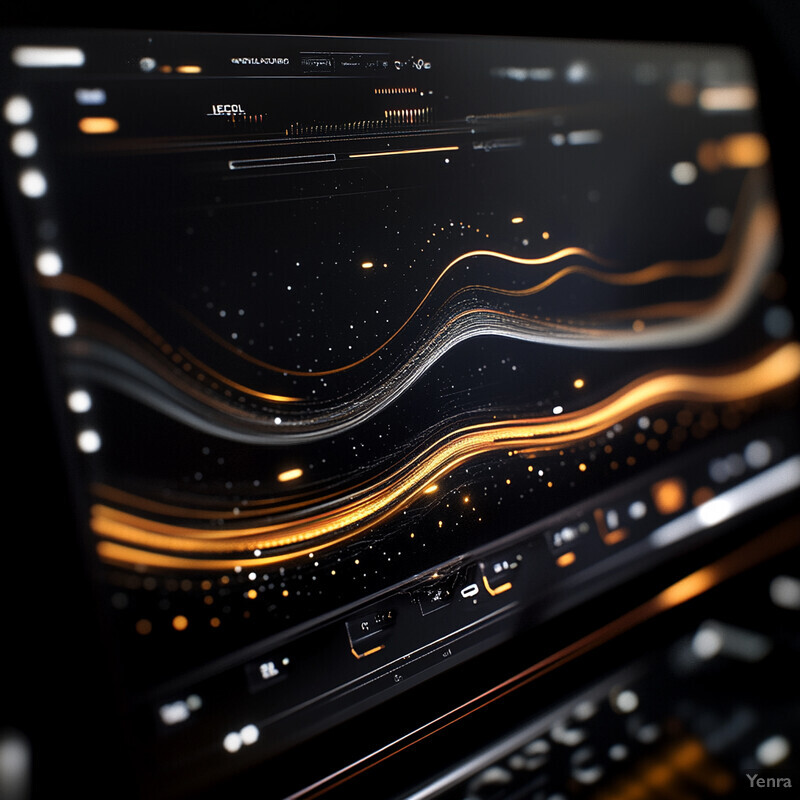
Radar performance hinges on selecting or designing optimal waveforms that balance resolution, range, and interference resistance. AI, through reinforcement learning or evolutionary algorithms, can autonomously choose or modify waveforms to adapt to changing conditions. For example, if the environment becomes more cluttered or jamming is detected, the radar can switch to a more robust waveform. Over time, these algorithms can learn patterns in the operational environment, predict adversarial tactics, and evolve waveforms that optimize detection probability. This ability translates to a more resilient and efficient radar system capable of maintaining performance across a broad spectrum of scenarios.
5. Adaptive Beamforming and Antenna Array Optimization
Neural networks can rapidly optimize antenna beam patterns, improving target localization accuracy and suppressing interference in real time.
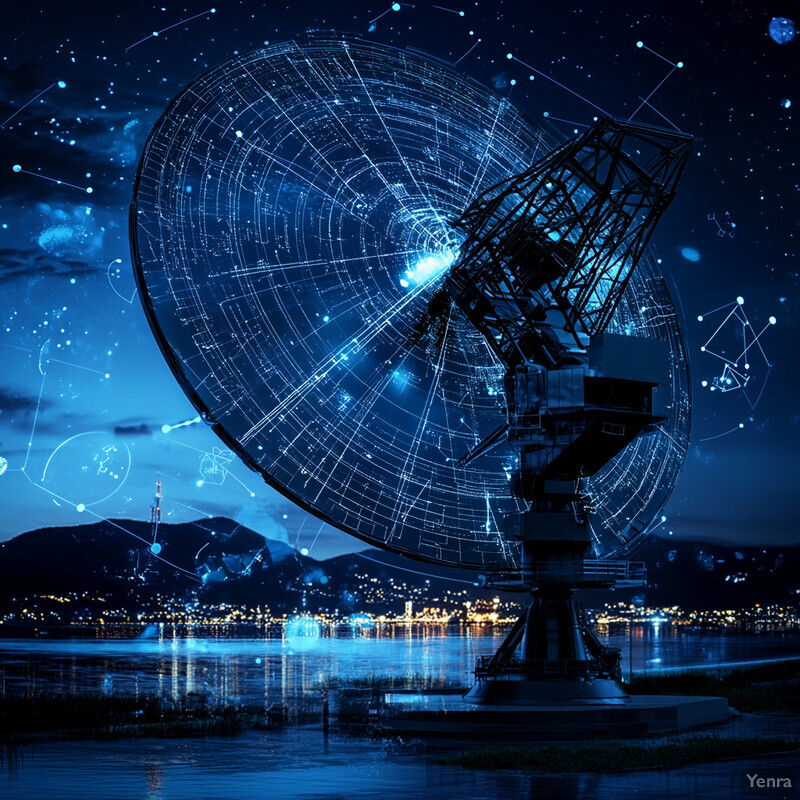
Beamforming involves shaping the radar antenna’s radiation pattern to enhance signals arriving from a particular direction while suppressing interference and clutter from others. Traditionally, beamforming solutions rely on established algorithms like MVDR or linear constraints. AI takes this a step further by dynamically learning the best weighting strategy for each antenna element in real-time. This adaptation can account for unknown interference sources, evolving target trajectories, and complex multipath reflections, leading to improved localization accuracy, more stable target tracking, and an overall increase in radar system effectiveness.
6. Predictive Maintenance and Fault Diagnostics
AI-based predictive models can monitor radar hardware health, detect anomalies, and schedule maintenance before critical failures occur, reducing downtime and lifecycle costs.
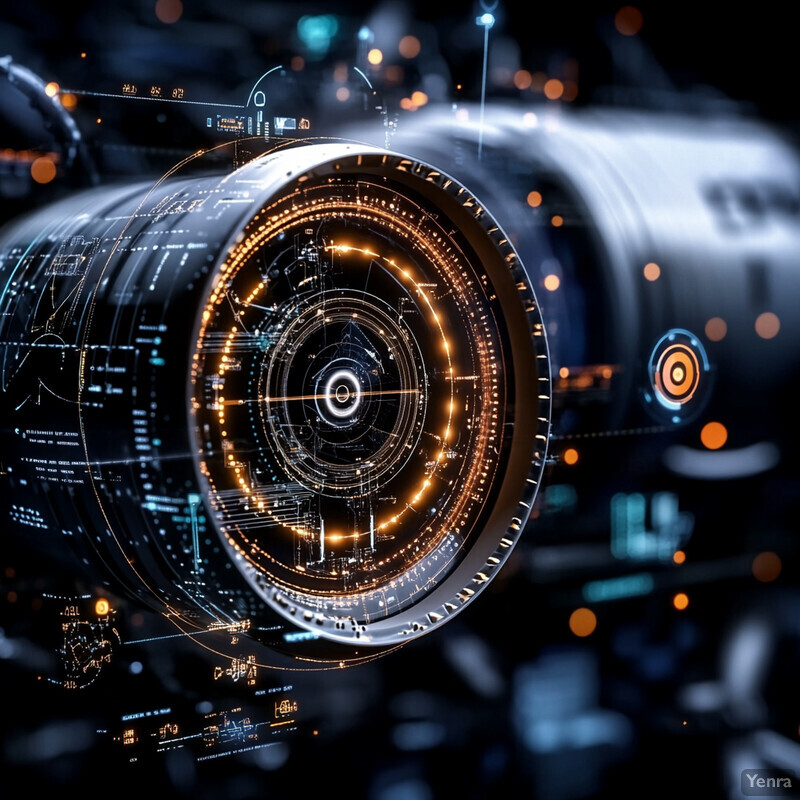
Beyond signal processing, AI is helping radar operators ensure system reliability and reduce downtime. Machine learning models can continuously monitor radar components—transmitters, receivers, processors—and analyze patterns in system health metrics and environmental data. By identifying subtle signs of wear or impending failure, predictive maintenance systems can recommend proactive repairs or replacements. This approach prevents catastrophic downtime during critical operations, extends hardware longevity, and lowers operating costs. It transforms maintenance from a reactive, time-based process into a proactive, condition-based strategy.
7. Robust Parameter Estimation (Range, Doppler, Angle)
Deep learning models can more accurately estimate target parameters, even in the presence of heavy interference, leading to finer velocity and position measurements.
-2.jpg)
Accurate estimation of a target’s range, velocity (Doppler shift), and angle is fundamental to radar operation. Traditional estimation methods sometimes struggle in low SNR or high interference conditions. AI-driven approaches employ neural networks and data-driven modeling to directly map received signals to parameter estimates, bypassing many classical assumptions. Such models can handle nonlinearities and complex noise statistics more naturally. This improves measurement accuracy and stability, ensuring that trackers receive more reliable input data and that subsequent decision-making—such as engagement control or collision avoidance—is based on sound, refined intelligence.
8. Improved Multi-Target Tracking and Data Association
Advanced ML techniques can handle complex multi-target scenarios, more accurately associating successive detections with individual targets, thus improving track stability and continuity.
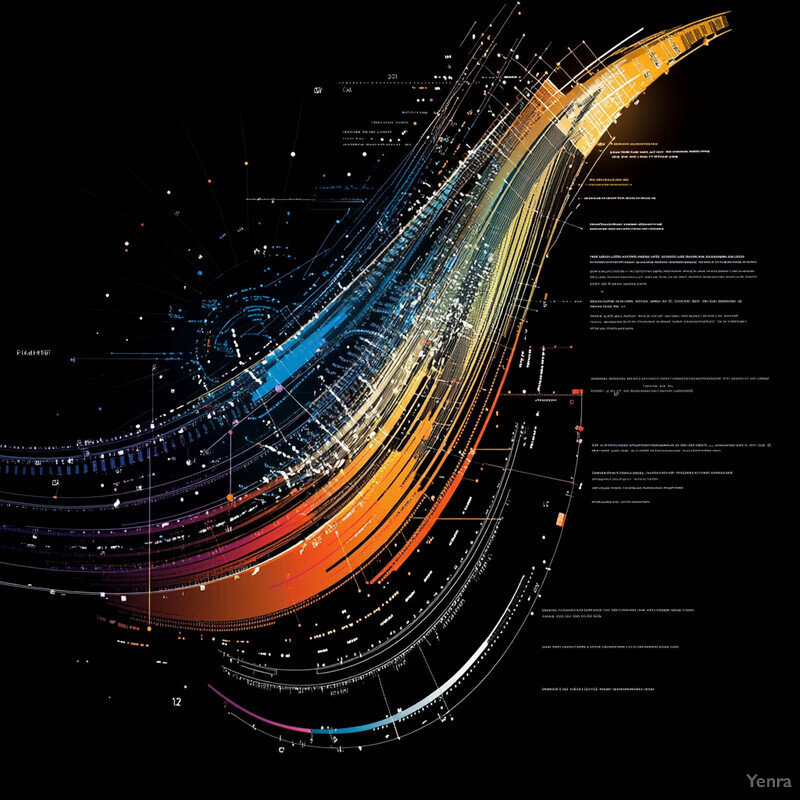
In dense target scenarios, the radar must not only detect multiple objects but also maintain distinct tracks on each. Classical multi-target trackers can get confused when targets cross paths, interfere with each other, or exhibit nonlinear motion. AI-driven data association techniques—like those involving recurrent neural networks—help associate consecutive measurements with the correct targets more reliably. This improved association reduces the risk of 'target swapping' and lost tracks, leading to more continuous and confident target trajectories. This capability is especially important for crowded skies and complex battlefield scenarios where multiple objects simultaneously vie for attention.
9. Sensor Fusion and Multi-Radar Integration
AI algorithms can fuse data from multiple radars and other sensors (e.g., EO/IR) to create a comprehensive and coherent situational picture, increasing confidence in target identification.
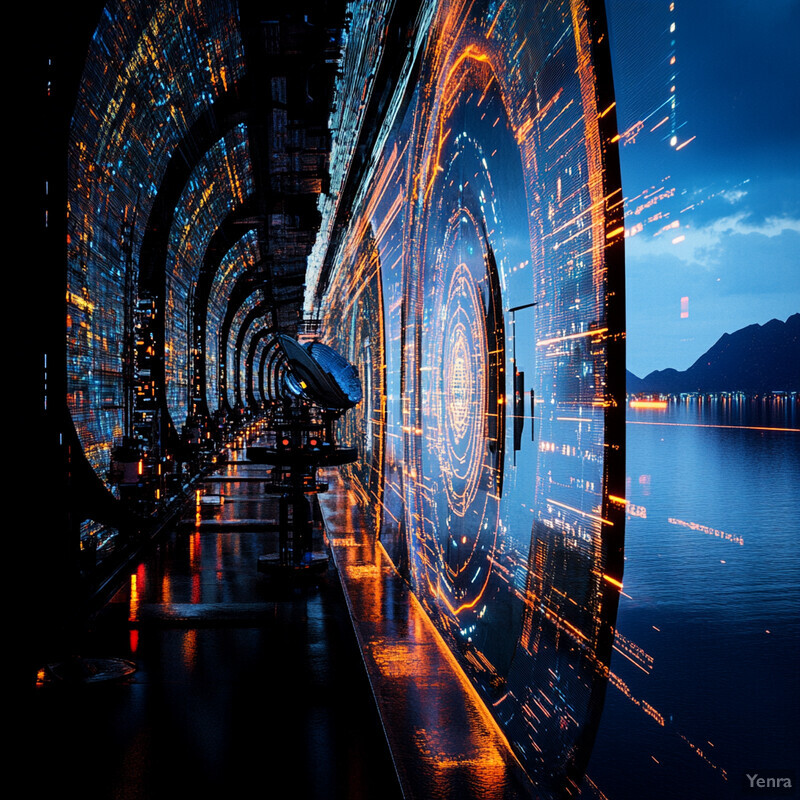
Modern operational environments increasingly rely on networks of multiple radar stations and other complementary sensors, such as electro-optical or infrared systems. AI enables seamless data fusion, correlating detections from different sensors to build a more complete and accurate representation of the environment. By integrating data of varying fidelity and modalities, AI can fill in gaps left by individual sensors and improve the overall situational picture. This comprehensive understanding leads to stronger decision-making and more robust target identification, as conflicting or ambiguous measurements are resolved through intelligent inference.
10. Adaptive ECCM (Electronic Counter-Countermeasures)
AI can rapidly detect and classify jamming or deceptive signals, then adapt the radar’s parameters to counteract these threats, maintaining target tracking amidst hostile ECM.
-1.jpg)
Radar systems face sophisticated adversaries that use electronic countermeasures (ECM) to deceive or jam signals. AI enhances ECCM by quickly detecting, classifying, and responding to these hostile tactics. Real-time inference allows the radar to adjust parameters—such as frequency hopping patterns, pulse repetition intervals, or beam steering angles—to mitigate jamming. Additionally, AI can predict adversary behavior over time, refining its responses with each encounter. This adaptability means that the radar remains operational and effective even when under continuous electronic attack.
11. Unsupervised Anomaly Detection
Unsupervised learning can identify unexpected or never-before-seen signal patterns, potentially uncovering stealth targets, low-observable threats, or new signal types without prior labeling.
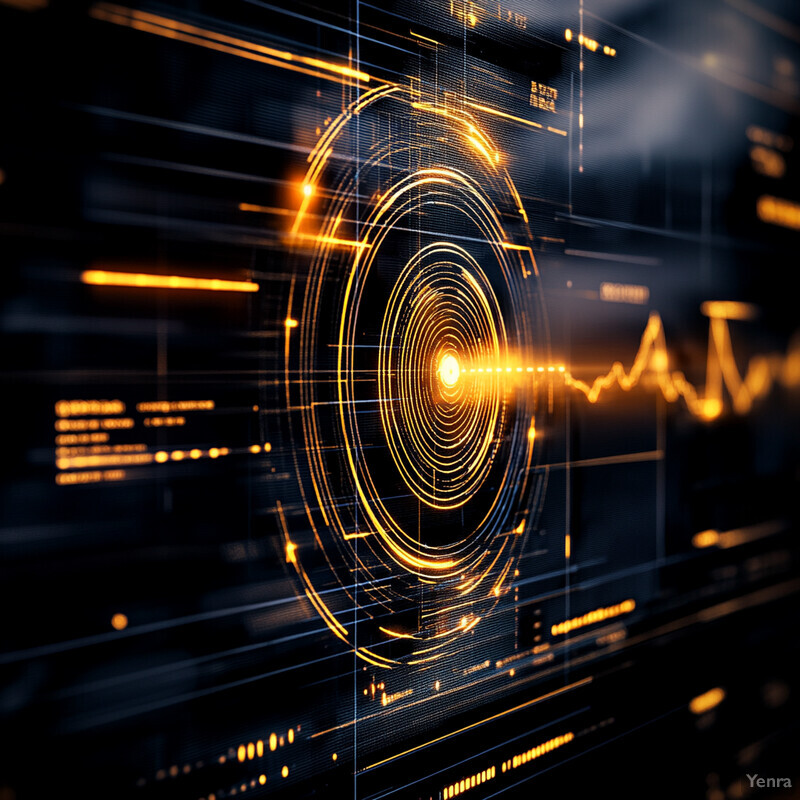
Not all signal patterns fit neatly into known categories. Unsupervised machine learning methods, like clustering and autoencoders, excel at detecting unusual or anomalous returns without prior labeling. These algorithms learn the 'normal' baseline of radar scene dynamics and highlight deviations that might indicate stealthy intruders, unusual weather conditions, or equipment malfunctions. By doing so, the radar can raise alerts for previously unseen threats, expand its operational capabilities, and evolve with changing landscapes and adversarial techniques.
12. Nonlinear Signal Processing for Overlapping Targets
Neural networks can separate and recover overlapped signals with nonlinear techniques, improving the extraction of meaningful information from challenging radar returns.
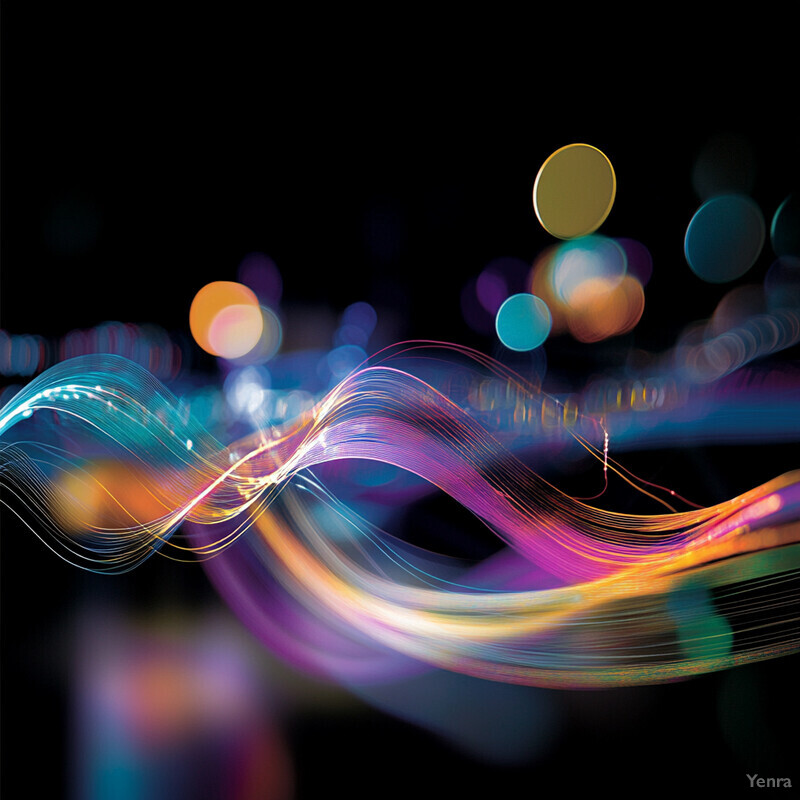
In busy or contested airspace, multiple targets may produce overlapping or interfering signals, making it hard to separate and identify each one using classical linear filters. AI approaches can exploit sophisticated nonlinear transformations, enabling more effective signal separation. By learning patterns in the time-frequency domain or employing deep source separation networks, the radar can disentangle complex mixtures of signals. The result is enhanced clarity, ensuring that critical targets do not get lost in the blend and that the radar can handle high target densities without performance degradation.
13. Deep Learning-Based Doppler Compensation
AI methods can correct Doppler shifts and phase errors more accurately and quickly, improving range-Doppler imaging and velocity estimation.
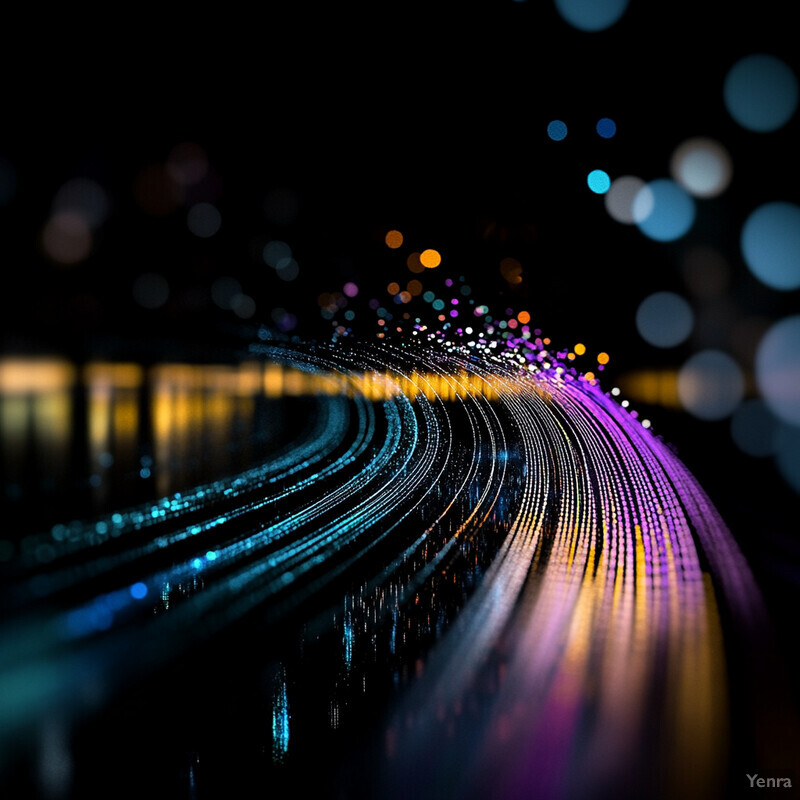
Doppler shifts induced by target motion can distort signal frequency and degrade detection and imaging quality. Deep learning models can predict and correct these shifts automatically, reducing computational overhead and increasing accuracy. By mapping raw radar returns to corrected Doppler parameters, the radar system can produce sharper range-Doppler profiles, improve velocity estimation, and support more effective target recognition. This technique ensures that even fast-moving or maneuvering targets remain trackable, strengthening the radar’s situational awareness.
14. Smart Clutter Classification for Environmental Monitoring
ML techniques can differentiate between man-made and natural clutter, leading to advanced environmental characterization and improved false alarm control.
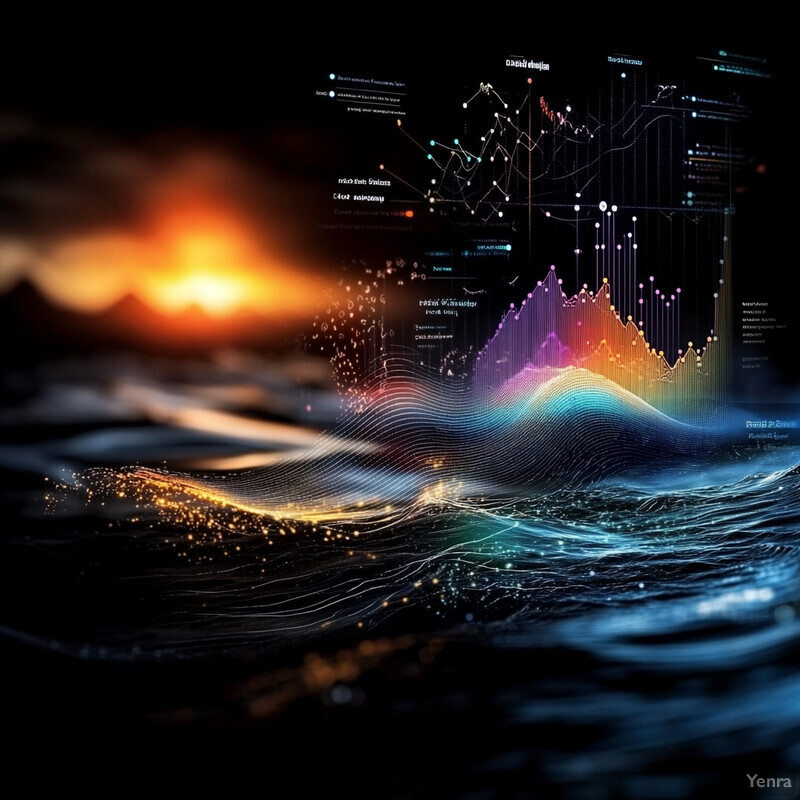
Not all returns need to be interpreted as threats or targets. Some echoes are from environmental sources—like trees, birds, or waves—that can provide valuable context or need to be filtered out. Intelligent algorithms can classify these clutter sources, not just as 'noise' but as meaningful categories that enrich the radar’s understanding of its surroundings. By doing so, radar operators can adapt detection thresholds to reduce nuisance alarms, track environmental changes, or even use radar data for ecological and meteorological studies, thus expanding the utility of radar beyond pure target detection.
15. Compressive Sensing with Learned Dictionaries
AI-driven compressive sensing can optimize how radar data is sampled, compressing large datasets while still retaining critical target information for rapid downstream processing.
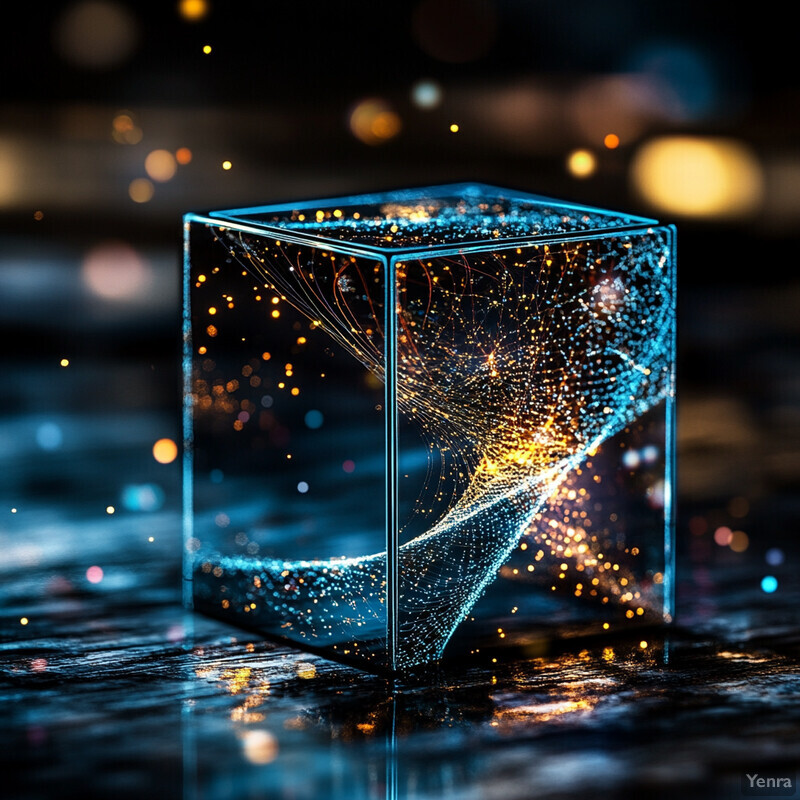
Radar data can be massive, necessitating efficient sampling and storage. AI-driven compressive sensing methods intelligently sample fewer data points while preserving essential information. Learned dictionaries and sparse coding techniques identify the most critical signal components for representing targets. By training on representative data, these models know which features to retain. This reduces bandwidth requirements and processing load while maintaining—or even improving—detection and estimation performance. As a result, radar systems become more resource-efficient and responsive.
16. Adaptive Thresholding and CFAR Optimization
Intelligent algorithms can dynamically adjust detection thresholds (e.g., Constant False Alarm Rate parameters) based on learned patterns, reducing false alarms and missed targets.
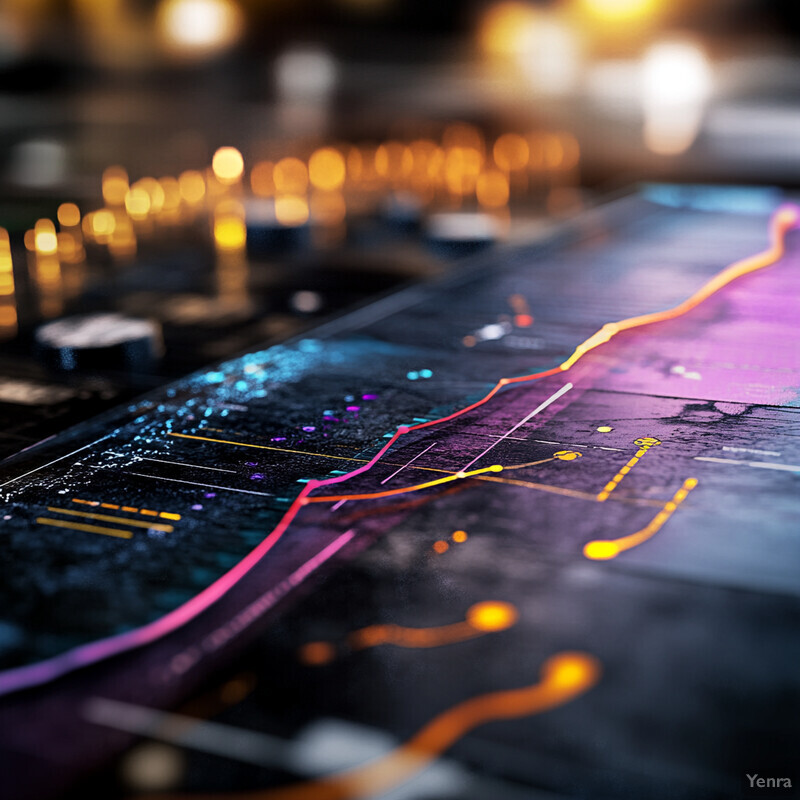
Constant False Alarm Rate (CFAR) detectors traditionally rely on fixed rules for setting detection thresholds. AI enables dynamic thresholding by analyzing signal distributions, clutter statistics, and environmental conditions in real-time. By continually adjusting the threshold to local conditions, the radar can lower false alarms when the environment is stable or become more sensitive when threats may be present. This fine-grained control balances the need for high probability of detection with a manageable false alarm rate, ultimately optimizing radar performance on the fly.
17. Transfer Learning Across Missions and Platforms
Models trained on one radar system or environment can be refined and quickly adapted to new platforms or scenarios, shortening development cycles and deployment times.
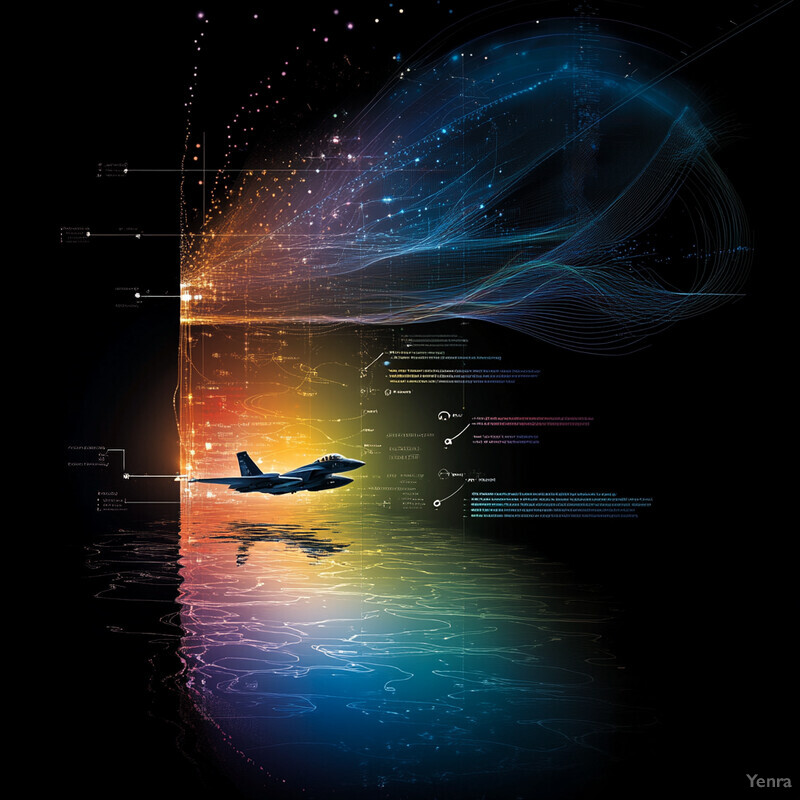
Developing new radar signal processing models from scratch for each mission or platform is time-consuming. Transfer learning leverages pre-trained models from one setting and tailors them to another, drastically reducing training data requirements and accelerating deployment. For instance, a model trained on one type of radar operating in maritime conditions can be adapted to a different radar system on land with minimal effort. This approach fosters rapid technological adoption and flexible system configuration, ensuring that AI-enhanced radar capabilities can be scaled and repurposed efficiently.
18. Cognitive Radar Capabilities
AI-enabled “cognitive radars” can learn from their environment, predict changes, and autonomously adjust operational parameters to achieve optimal performance in real time.
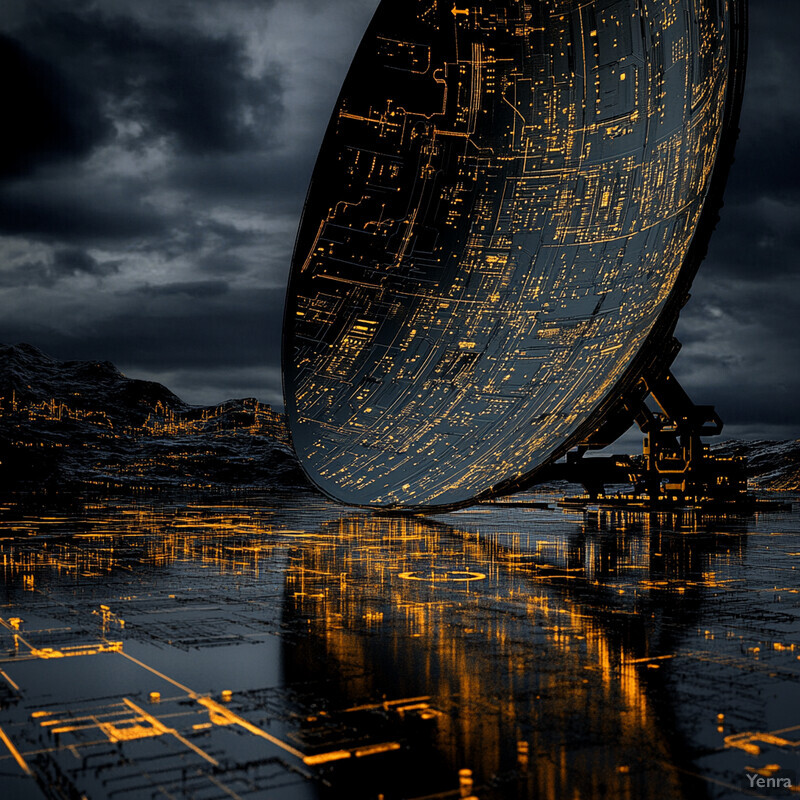
Cognitive radars are AI-driven systems that observe their environment, learn from their experiences, and refine their operational strategies. By continually evaluating performance indicators—like detection probability, false alarm rates, and track continuity—cognitive radars identify when their strategies need to change. They can autonomously select new waveforms, revisit beam scheduling, or refine clutter models. This closed-loop feedback makes the radar system more resilient, adaptive, and capable of optimizing its behavior to evolving challenges, embodying the next generation of intelligent sensing.
19. Enhanced State Estimation and Filtering
Integrating neural networks with traditional tracking filters (e.g., Kalman or particle filters) leads to more accurate and robust state estimation under a variety of challenging conditions.
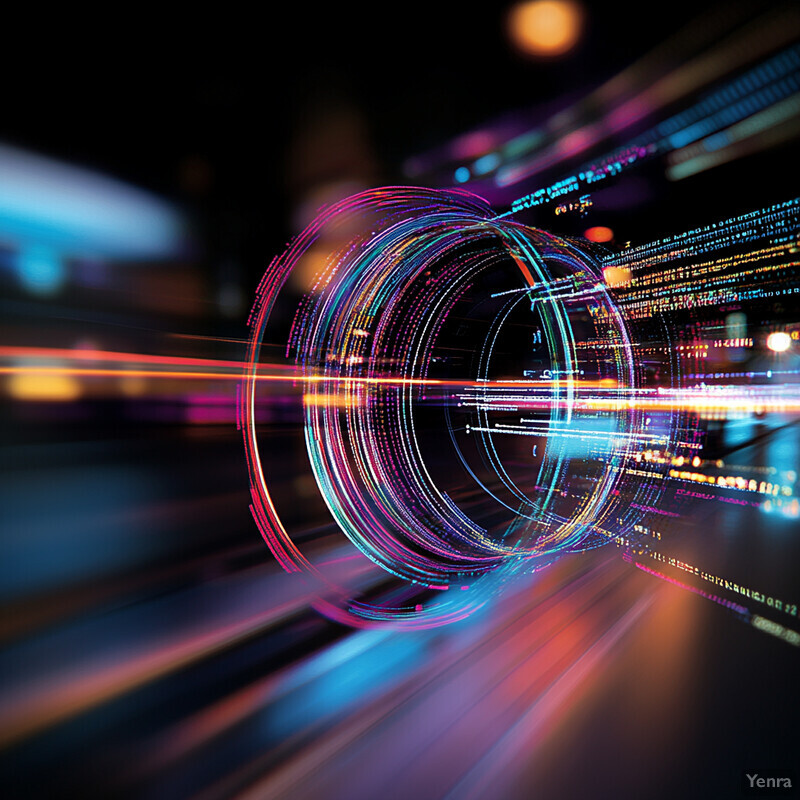
State estimation underpins tasks like target tracking and predictive guidance. Integrating AI with traditional filters (e.g., Kalman, particle filters) marries model-driven approaches with data-driven pattern recognition. Neural networks can learn to correct for nonlinearities, biases, or uncertainties not accounted for by classical estimators. This synergy results in more accurate and stable state estimates, smoother target tracks, and more reliable measurements for decision-making processes, particularly in complex, high-uncertainty environments.
20. Continual Learning for Long-Term Adaptation
Machine learning architectures can evolve over time, integrating experience from multiple missions to gradually improve detection, classification, and tracking performance as environments or threat profiles change.
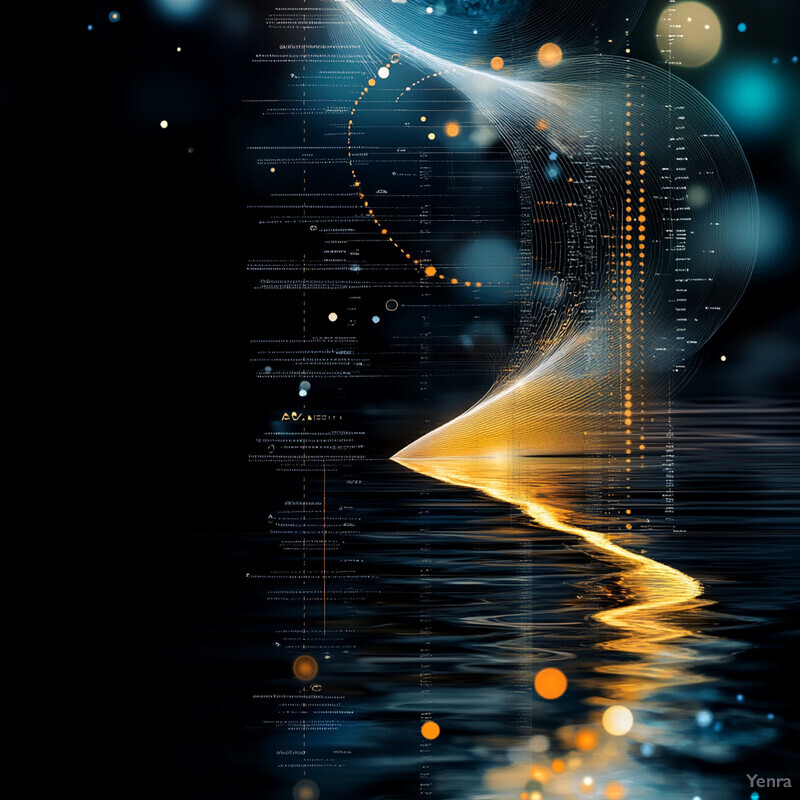
The world evolves, and so must radar systems. AI algorithms built on continual learning paradigms adapt as they encounter new signal types, environments, and threats. Instead of static models that degrade over time, these systems refine their understanding continuously, integrating new data without forgetting previously learned concepts. This approach ensures that radar capabilities improve with every deployment, maintaining cutting-edge performance, resilience, and intelligence in a rapidly changing operational landscape.