1. Predictive Analytics for Demand Forecasting
AI-driven models use historical weather data, occupancy patterns, and energy usage trends to accurately predict heating and cooling demand, allowing HVAC systems to proactively adjust settings before conditions change.
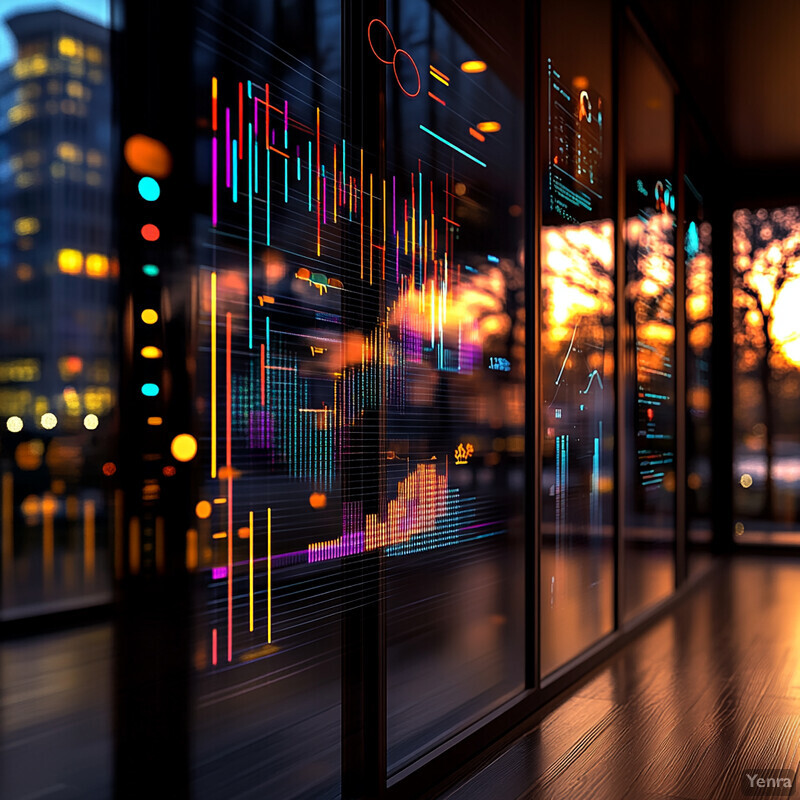
AI-driven predictive analytics leverage historical building and environmental data to forecast heating and cooling requirements well in advance. By examining patterns from past energy usage, occupancy rates, and local weather conditions, intelligent models can anticipate future load demands. This empowers HVAC systems to preemptively adjust operations, starting necessary equipment earlier or delaying activation until the most energy-efficient moment. Consequently, the system reduces the likelihood of sudden spikes in energy consumption and ensures indoor comfort remains consistent, even amid fluctuations in occupancy or external climate changes. Over time, these forecasts grow more accurate, thanks to continuous learning and refined data inputs.
2. Dynamic Setpoint Optimization
Intelligent algorithms continuously optimize temperature setpoints to balance comfort, energy efficiency, and system longevity, using inputs like real-time building occupancy, humidity levels, and utility rate structures.
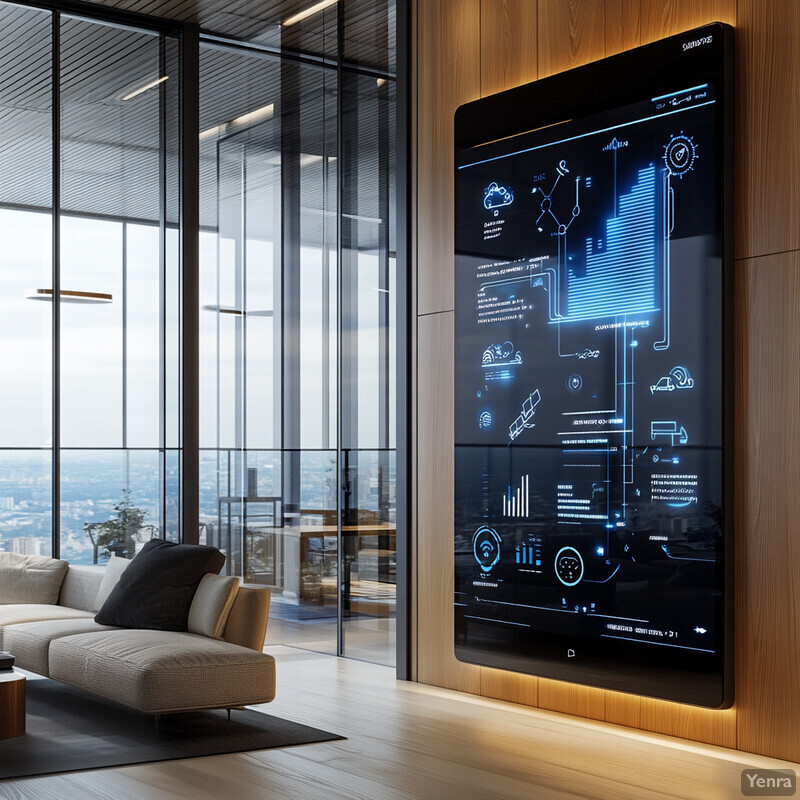
Dynamic setpoint optimization uses AI algorithms to refine the temperature and humidity targets within a building’s HVAC system on a near-continuous basis. Instead of relying on static, one-size-fits-all thresholds, the system analyzes live data such as indoor air quality, occupant feedback, outside weather forecasts, and current utility rates. By dynamically tweaking setpoints, the HVAC system consistently balances energy efficiency and user comfort. This might mean allowing slightly higher temperatures during peak energy cost periods if occupants remain comfortable, or pre-cooling spaces before a heat wave hits. The outcome is a more responsive and cost-effective HVAC strategy.
3. Advanced Fault Detection and Diagnostics FDD
Machine learning models analyze sensor data to identify subtle performance degradations or anomalies in HVAC components, flagging potential issues before they grow into costly failures.
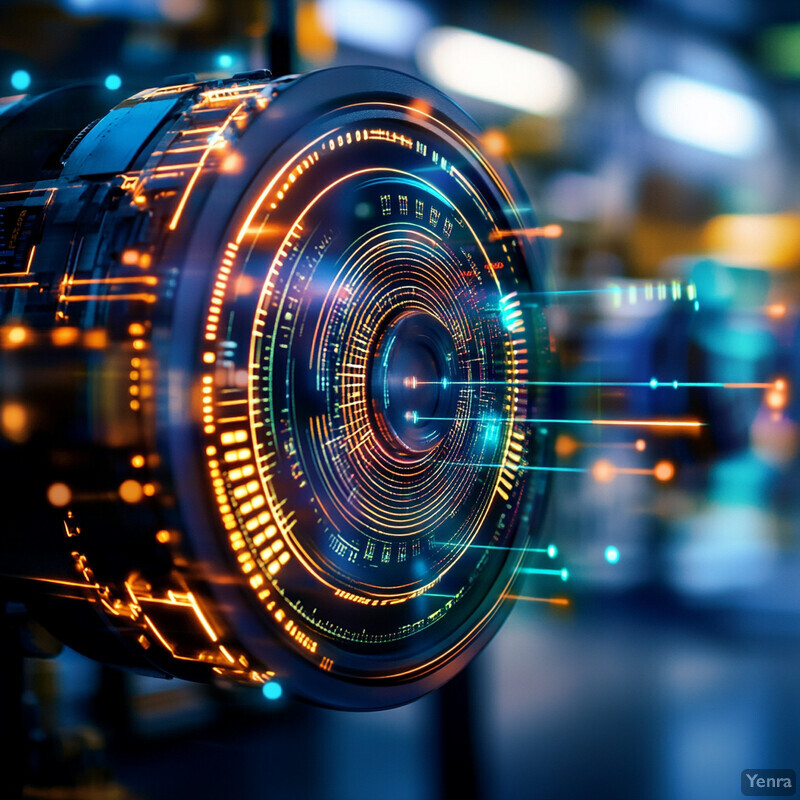
AI-driven fault detection and diagnostics involve continuously analyzing sensor feeds from HVAC components—motors, compressors, fans, filters, and valves—to identify subtle performance anomalies. Advanced machine learning models can recognize when equipment performance starts drifting from normal parameters, even before human operators notice. By catching issues early, the system can notify maintenance teams, helping them address mechanical problems proactively rather than waiting for costly breakdowns. Over time, these diagnostics also improve capital planning, as building managers gain insights into common failure modes and the expected lifespan of components, ultimately reducing downtime and maintenance costs.
4. Adaptive Control Strategies
AI algorithms can learn from past system performance and user feedback to develop adaptive control policies that automatically refine HVAC operations over time, improving both occupant comfort and energy efficiency.
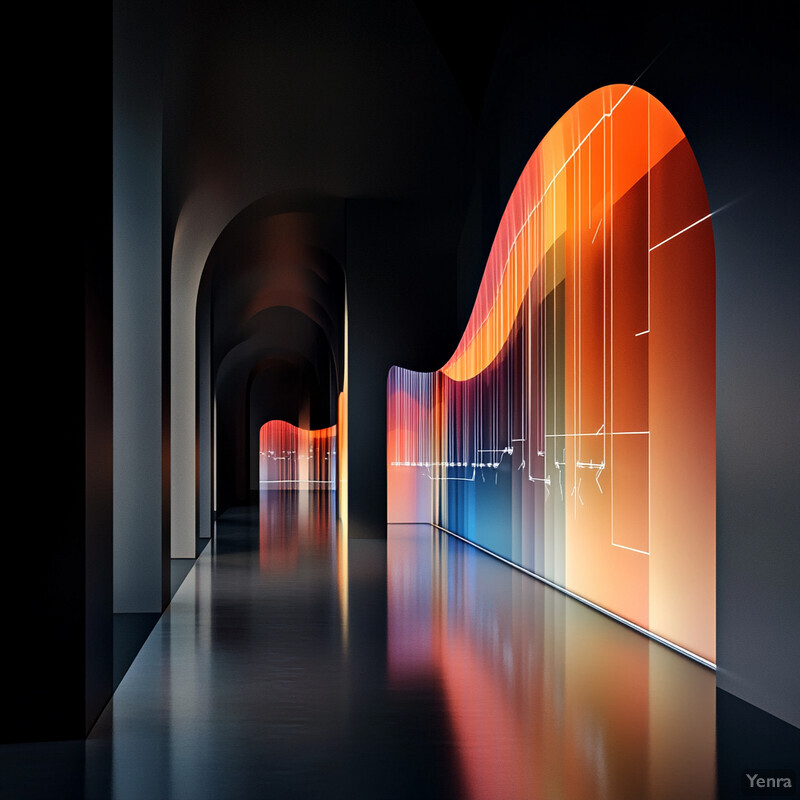
Adaptive control strategies rely on AI’s capacity to learn from past performance data, user interactions, and changing environmental conditions to refine HVAC operations. Instead of running on fixed schedules or simplistic rules, adaptive controllers evolve their decision-making policies over time. For example, if certain temperature adjustments yield better occupant comfort without significantly increasing energy use, the algorithm will prioritize these strategies moving forward. As a result, HVAC systems become more personalized, efficient, and responsive, improving occupant satisfaction and cutting down on wasted energy through constant optimization.
5. Occupant Behavior Modeling
By integrating data from smart building sensors and occupant calendars, AI can predict when and where people will be in the building, optimizing HVAC operations accordingly.
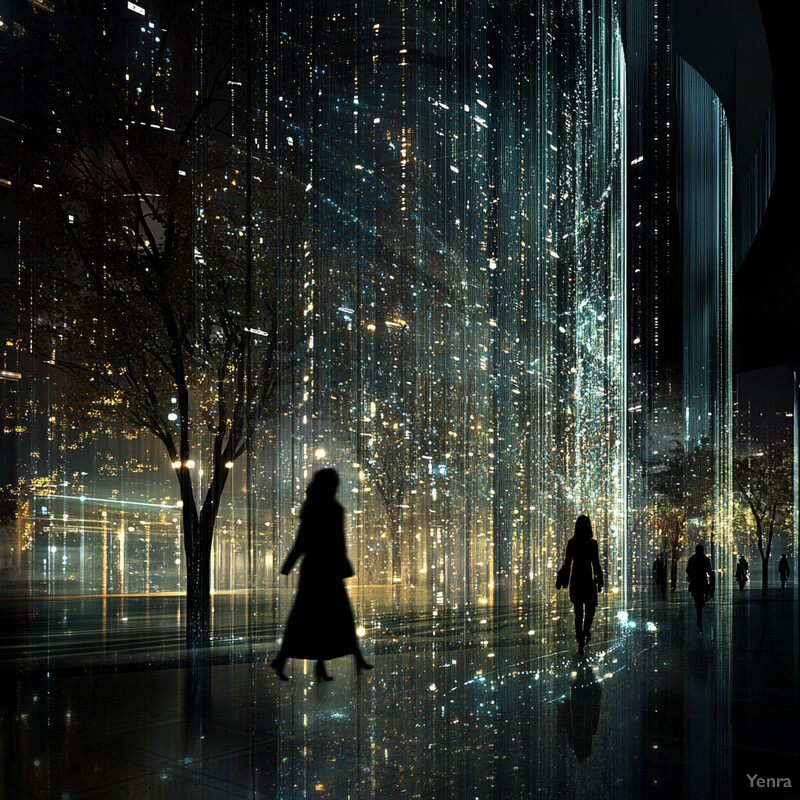
By incorporating occupant behavior modeling, AI-enabled HVAC systems learn from patterns in how people use and move through a building. Data from occupancy sensors, room reservation systems, and security access logs can be integrated to predict when different zones will be populated. The HVAC system then pre-conditions these areas before occupants arrive and reduces conditioning when spaces are empty. Over time, this behavior-driven approach ensures that energy is not spent heating or cooling unoccupied rooms while guaranteeing that frequently used areas are always comfortable exactly when needed.
6. Weather-Adaptive Adjustments
Advanced forecasting models ingest local climate predictions and dynamically tune HVAC schedules and setpoints, ensuring systems ramp up or down optimally in response to shifting outdoor conditions.
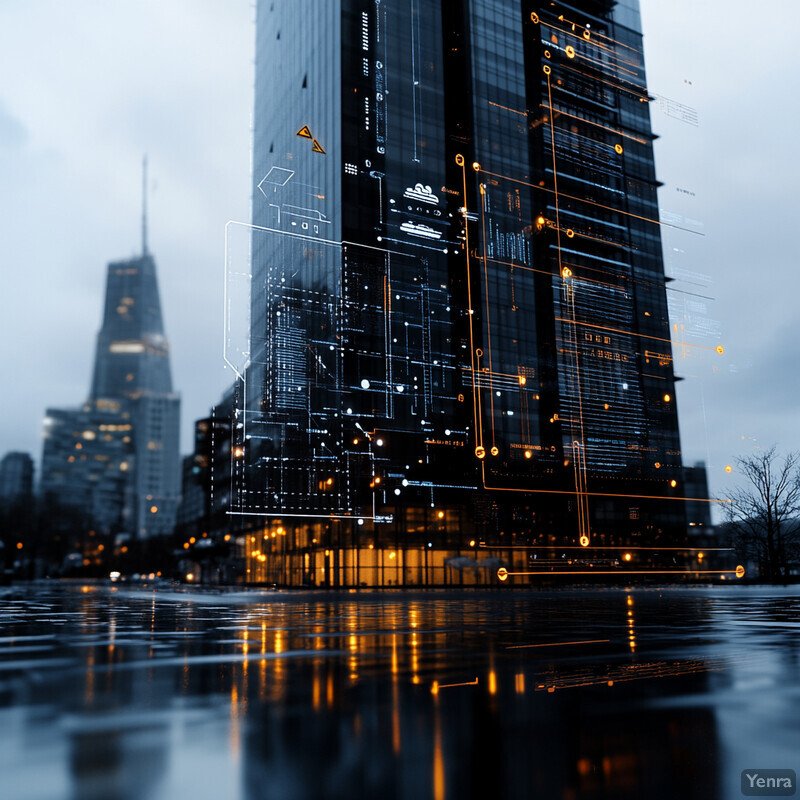
Weather-adaptive adjustments empower HVAC systems to prepare for environmental changes well before they occur. Advanced AI models ingest short- and long-term forecasts, analyzing trends in temperature, humidity, and even solar radiation. By anticipating a cold front or a sudden heat wave, the system can gently adjust conditioning in advance to avoid sudden spikes in energy consumption. Additionally, leveraging renewable energy inputs (e.g., solar generation forecasts) can help the system operate more sustainably and cost-effectively. This forward-looking approach ensures consistent occupant comfort and optimized energy usage regardless of the weather.
7. Learning-Based Scheduling
Reinforcement learning approaches let HVAC controllers experiment with different operational strategies, learning which produce the best outcomes for comfort and cost savings.
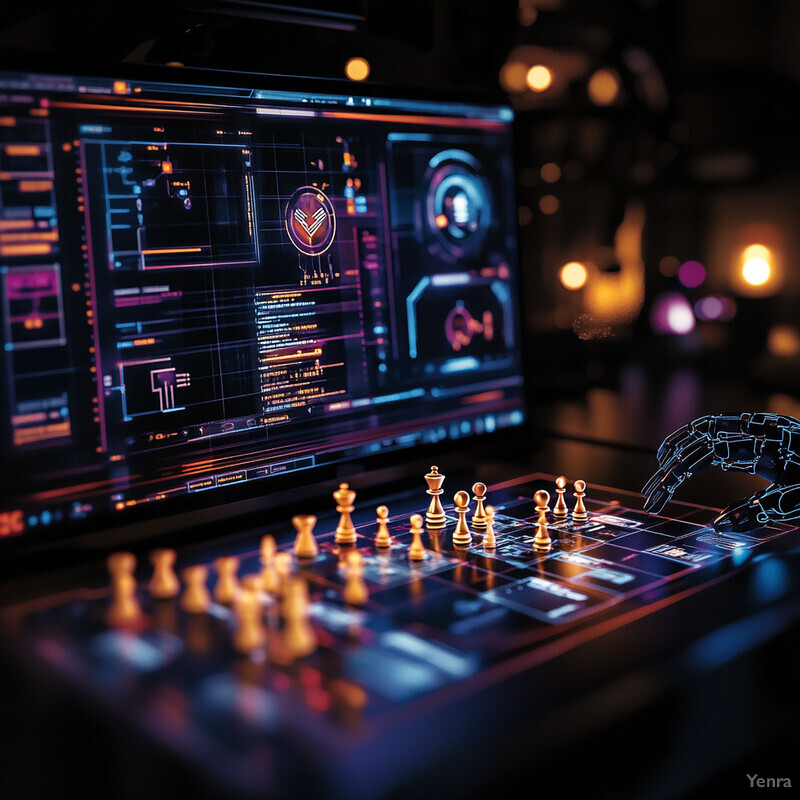
Reinforcement learning (RL) approaches treat HVAC control as a dynamic game, where the system “tries out” various operational decisions and receives feedback through energy consumption costs and occupant comfort metrics. Over time, the RL agent learns which sequences of actions lead to the best outcomes. For example, if pre-cooling an office at a certain time leads to higher satisfaction and lower costs, the agent will adopt that strategy more frequently. This iterative learning process can adapt to changing building conditions or energy pricing structures, making HVAC control more robust, efficient, and aligned with evolving goals.
8. Load-Shifting and Demand Response
AI-enabled HVAC systems can respond to grid signals, shifting heating and cooling loads to off-peak times or participating in demand-response programs to reduce costs and aid grid stability.
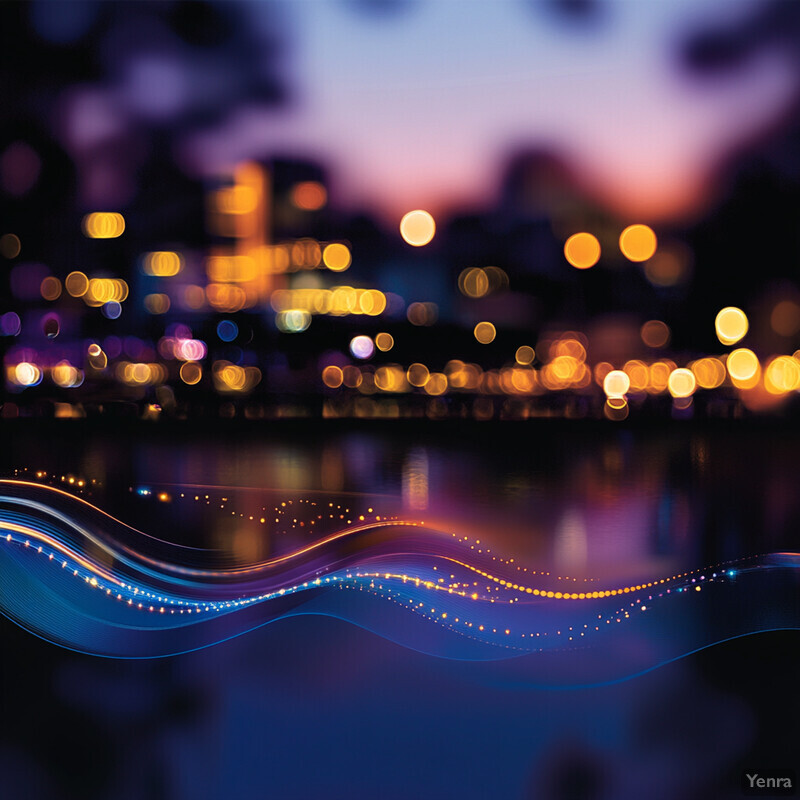
AI-driven load-shifting and demand response strategies allow HVAC systems to participate in grid-level energy management. By analyzing utility pricing signals and peak demand events, an intelligent HVAC controller can shift heating and cooling loads to off-peak hours or modulate demand during critical periods. This approach not only helps building owners reduce costs by avoiding premium energy rates but also supports grid stability and sustainability. For instance, a system might pre-cool a building at night when electricity is cheaper, lowering the need for costly energy usage during the daytime peak period.
9. Multi-Zone Coordination
Intelligent optimization routines analyze conditions across multiple zones, coordinating HVAC output so that energy is distributed efficiently where it’s needed most.
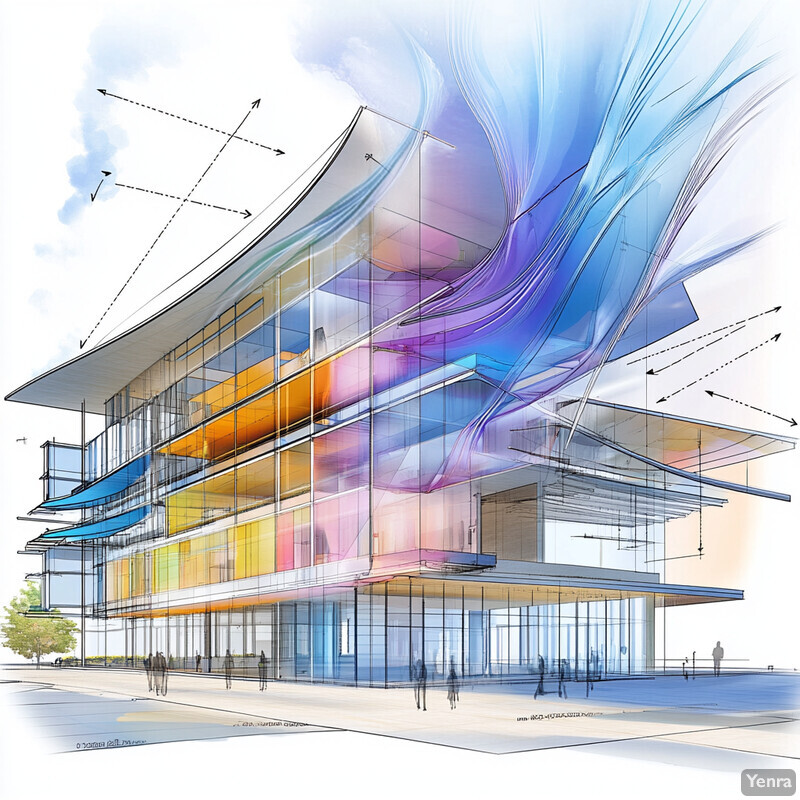
Large buildings often have multiple zones with distinct usage patterns and thermal properties. AI-based multi-zone coordination harmonizes these areas by analyzing each zone’s conditions and requirements in real-time. The system identifies which zones need immediate attention, which can be delayed, and how to allocate energy resources optimally. This ensures that all areas receive just the right amount of conditioning at the right time. By preventing over-conditioning in one area while another is neglected, multi-zone coordination reduces energy wastage, enhances overall comfort, and extends equipment life through balanced usage.
10. Integration with Building Management Systems (BMS)
AI-based HVAC tuning solutions can interface seamlessly with broader building management platforms, orchestrating HVAC, lighting, and other subsystems for holistic energy savings.
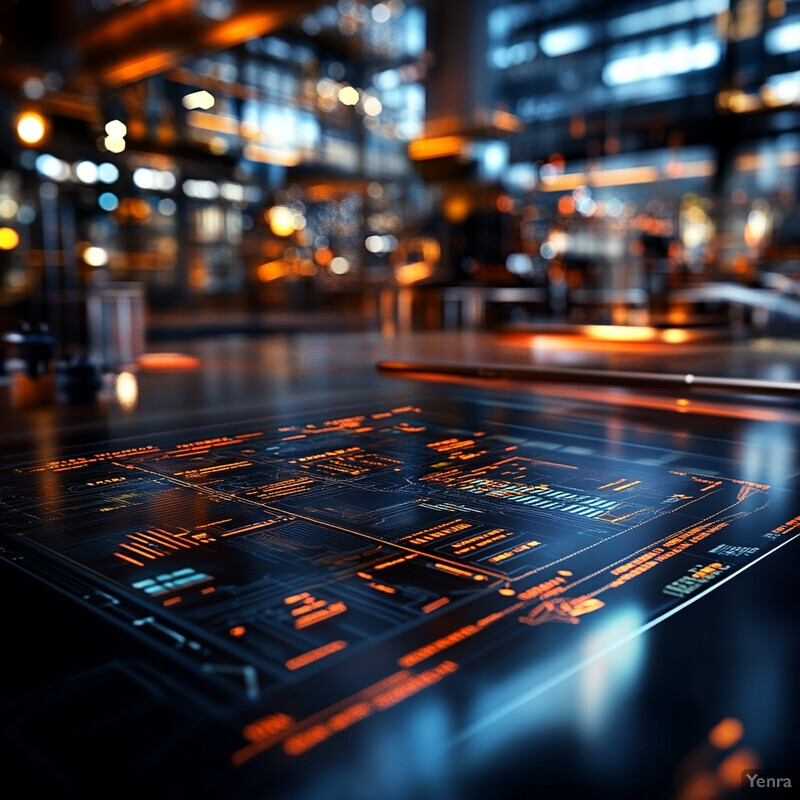
When integrated with broader building management systems, AI-driven HVAC solutions synchronize with lighting controls, access systems, and security platforms. By sharing data and control signals, the HVAC system can make more informed decisions. For example, if the BMS detects that a large conference room is scheduled for a meeting, the HVAC system can pre-heat or pre-cool that space. Simultaneously, if access logs show many employees working from home on a certain day, unnecessary conditioning can be minimized. This holistic approach results in a fully orchestrated, energy-efficient environment.
11. Automated System Calibration
AI models can verify that HVAC sensors, actuators, and controllers are functioning correctly and calibrate them as needed, ensuring consistent, accurate performance.
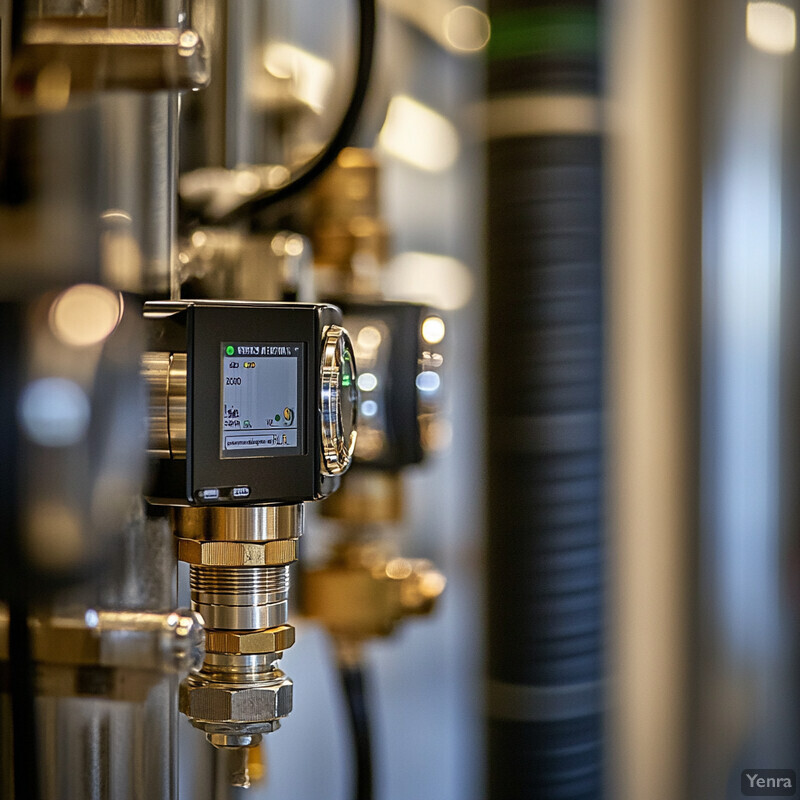
Over time, sensors drift from their original accuracy and actuators may not respond as reliably. AI-driven automated calibration tools monitor sensor readings and control outputs, comparing them against expected baselines. If differences arise, the system can either recalibrate devices automatically or alert technicians. This continuous validation ensures that HVAC equipment always runs at peak accuracy, so decisions based on those sensor readings remain trustworthy. Reduced reliance on manual calibrations frees up maintenance resources and guarantees better system reliability and performance.
12. Virtual Sensors and Soft Sensing
Machine learning can infer missing data from correlated variables, effectively creating 'virtual sensors' that estimate conditions without extra hardware.
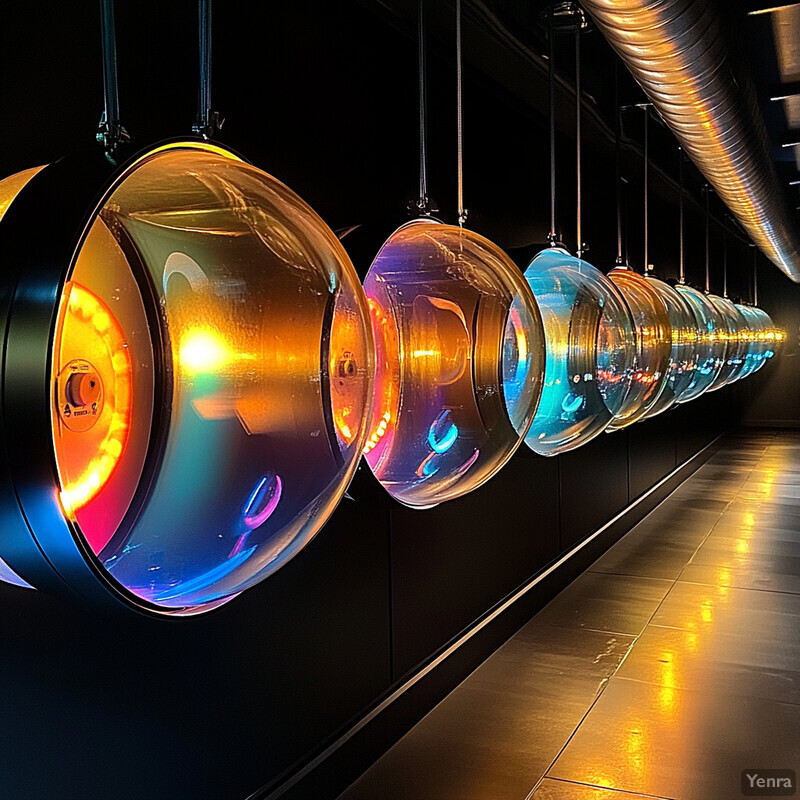
Virtual sensors—also known as soft sensors—use AI models to estimate conditions that are not directly measured by physical sensors. By correlating known data points (e.g., temperature, humidity, occupancy) with desired but unmeasured parameters (e.g., localized CO2 levels or specific zone air quality), the system can infer additional insights without expensive sensor installations. These virtual measurements provide richer situational awareness, enabling more nuanced HVAC control and guiding maintenance decisions. They fill critical data gaps and empower more precise, data-driven optimization strategies across the building.
13. Performance Benchmarking
AI continuously compares HVAC performance against historical baselines or peer buildings, revealing improvement opportunities and verifying energy savings.
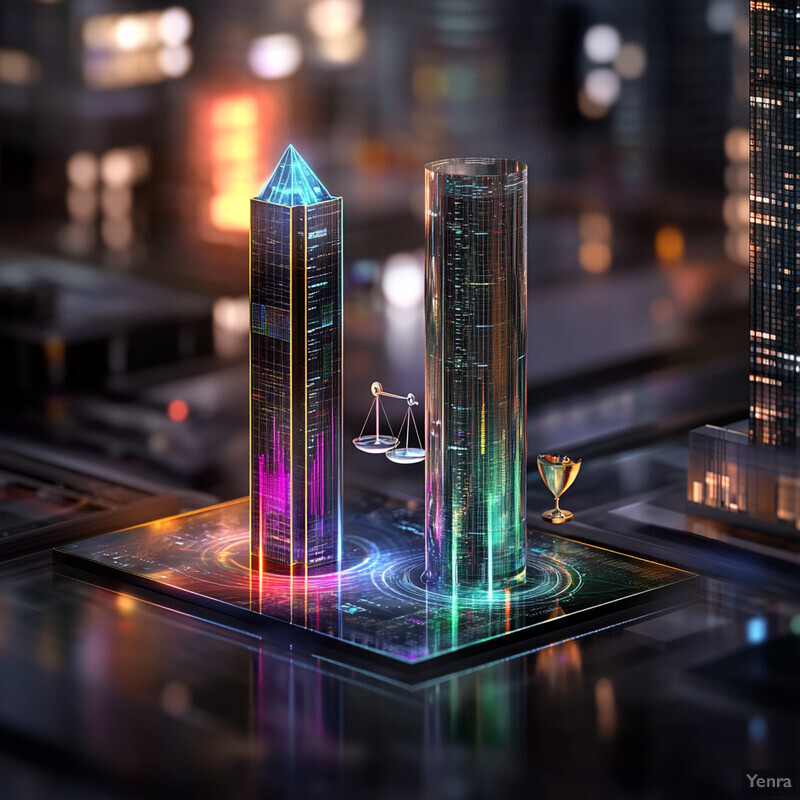
AI-enabled HVAC solutions can continuously monitor how the system is performing compared to historical baselines or similar buildings in the industry. By analyzing trends, anomalies, and energy usage patterns, it can highlight opportunities for improvement and verify the effectiveness of implemented strategies. Building managers receive actionable intelligence about how their building stacks up against benchmarks, which encourages ongoing improvement. Over time, consistent monitoring and benchmarking drive incremental refinements, gradually enhancing energy efficiency, occupant comfort, and overall HVAC system robustness.
14. Life-Cycle Cost Optimization
Through simulations and predictive analytics, AI can guide capital planning by suggesting HVAC upgrades or modifications to reduce overall life-cycle costs.
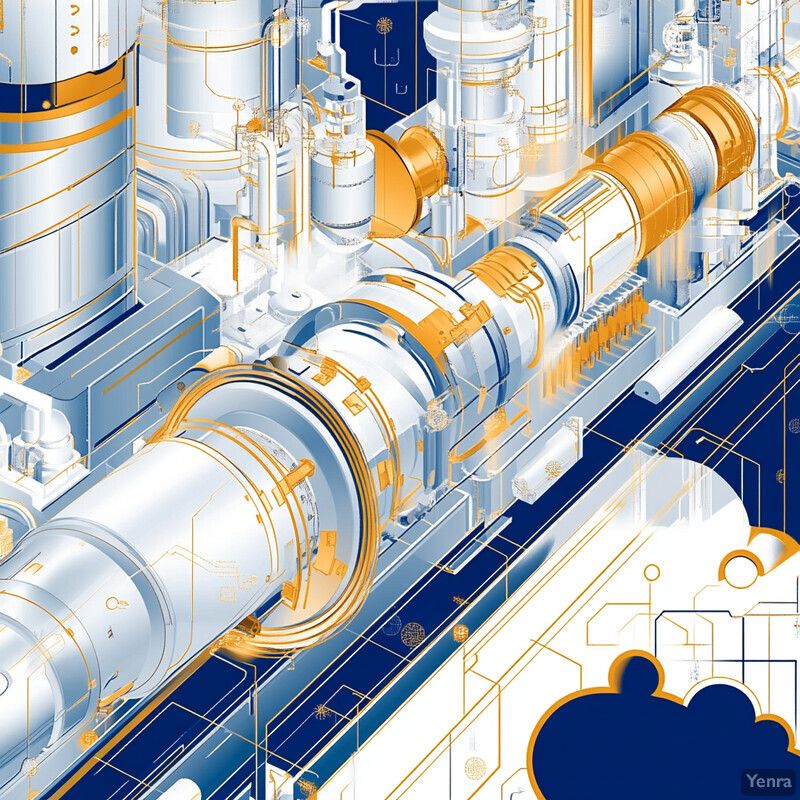
HVAC systems are long-term investments, and AI can optimize costs across their entire lifespan. By simulating different upgrade scenarios, retrofit strategies, and maintenance plans, AI tools help decision-makers choose the most cost-effective approach. For instance, replacing an aging chiller with a more efficient model might pay for itself through reduced energy bills over a known timeframe. AI algorithms balance upfront costs, operational expenses, and anticipated maintenance to guide the building’s stakeholders in achieving the best return on investment and longevity for their HVAC infrastructure.
15. Health and Comfort Analytics
Beyond temperature, AI can incorporate data on pollutants, humidity, and noise levels to ensure HVAC operations enhance overall indoor environmental quality.
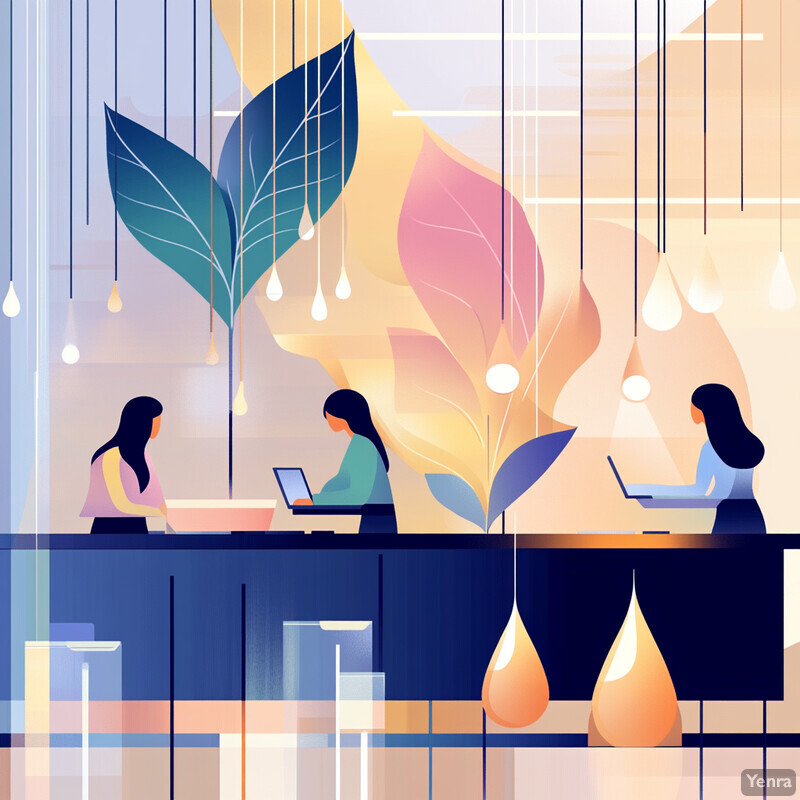
Modern buildings must maintain not just temperature comfort, but also a healthy indoor environment. AI can incorporate indoor air quality (IAQ) metrics—like particulate matter, volatile organic compounds (VOCs), and CO2 levels—into HVAC control strategies. By dynamically adjusting airflow, filter usage, and fresh air intake based on occupant density and pollutant concentrations, the system maintains a healthier indoor climate. This proactive approach can reduce sick building syndrome and improve occupant productivity, as well as ensure compliance with health and safety regulations.
16. Real-Time Sensor Fusion
By combining data from disparate sources, AI develops a more accurate understanding of current conditions and applies more precise HVAC tuning adjustments.
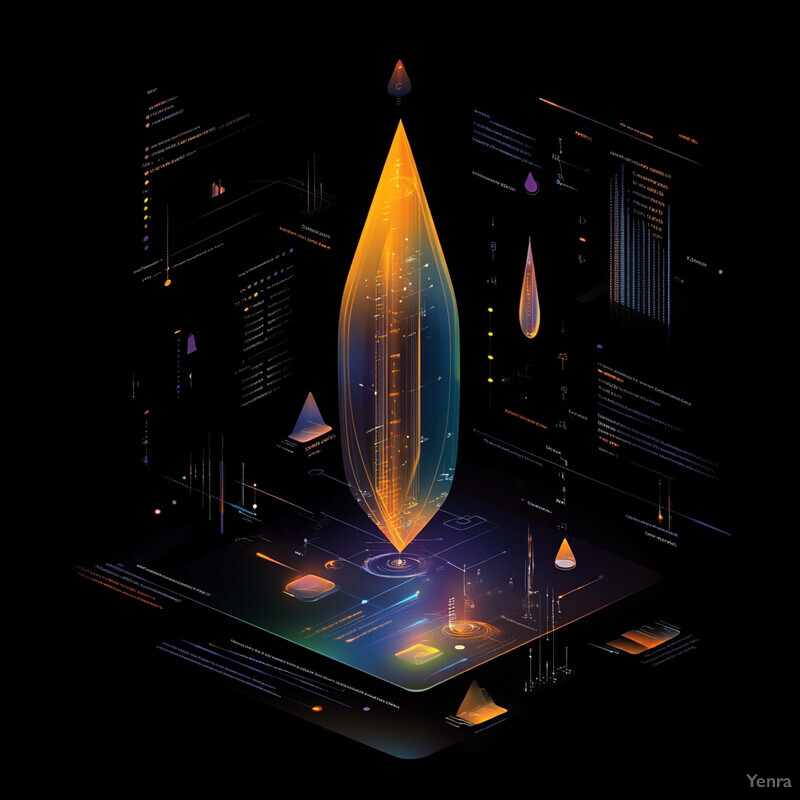
Real-time sensor fusion combines data streams from a multitude of building sensors, including temperature, humidity, occupancy counters, and air quality metrics. AI models integrate these diverse inputs to create a comprehensive picture of the building’s current conditions. By weighing each data source’s reliability and relevance, the system can make more informed decisions, fine-tune HVAC operations, and quickly adapt to any changes. This holistic understanding of the environment leads to more accurate temperature and ventilation control, ensuring stable comfort levels and optimized energy consumption.
17. Automated Commissioning and Retro-Commissioning
AI-driven tools streamline the initial commissioning of new HVAC systems and the retro-commissioning of existing ones, identifying inefficiencies and fine-tuning parameters automatically.
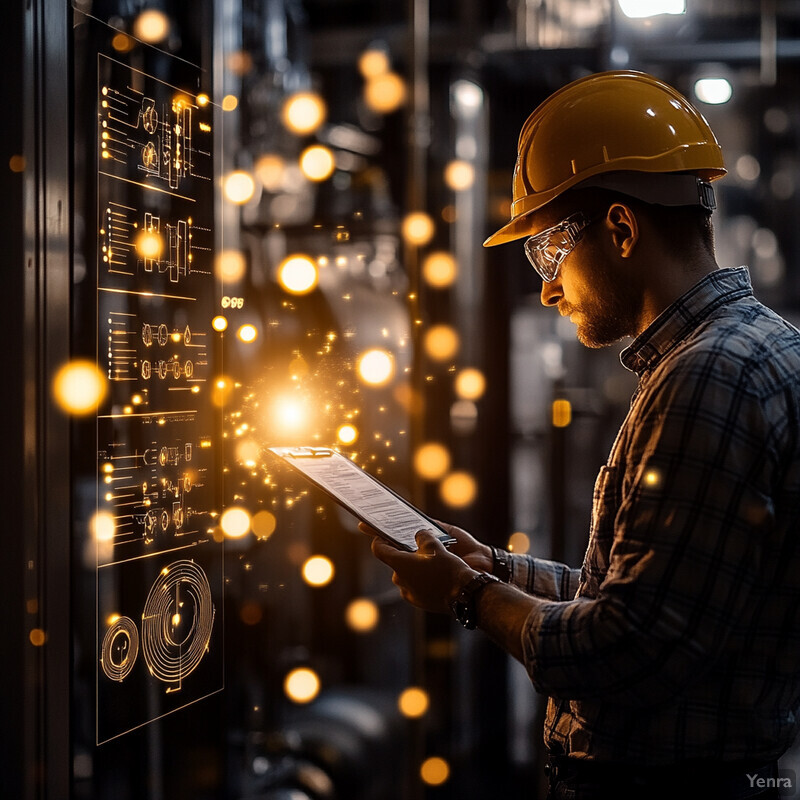
Commissioning ensures that a new HVAC system is installed and operating correctly from the start, while retro-commissioning applies similar principles to existing systems. AI-driven tools streamline these processes by rapidly analyzing performance data, identifying deviations from expected behavior, and suggesting parameter adjustments. Instead of manually testing and tuning equipment, building managers can rely on AI insights to confirm optimal operation. Over time, this reduces the effort, cost, and complexity associated with bringing systems online or fine-tuning aging infrastructure, ensuring peak performance throughout the building’s life.
18. Predictive Maintenance Scheduling
By analyzing real-time equipment data, AI can schedule maintenance before failures occur, reducing costly downtime and extending system life.
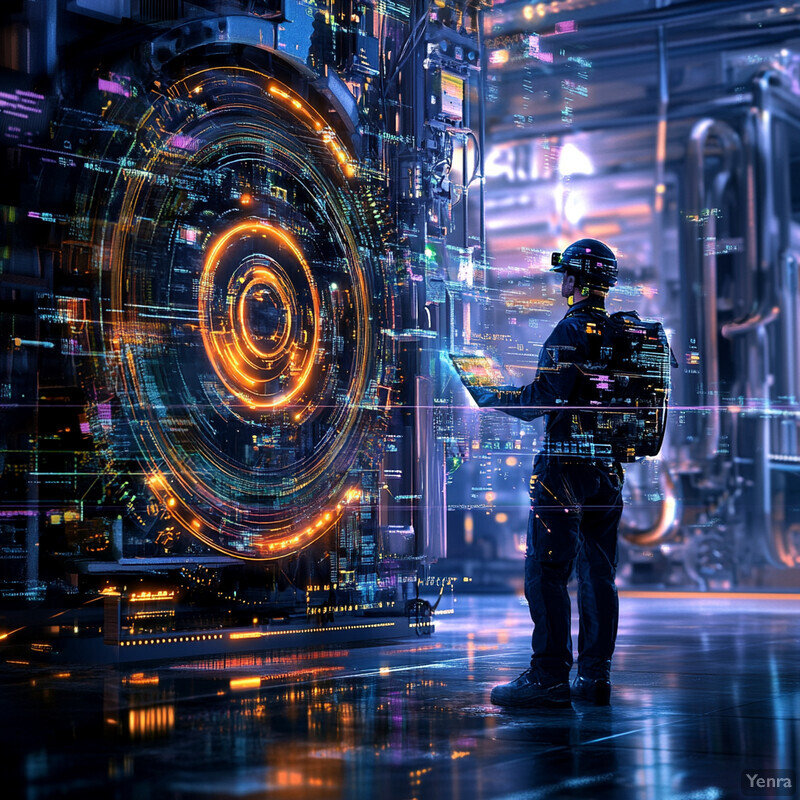
Instead of adhering to rigid maintenance timetables, AI-driven predictive maintenance solutions assess real-time data from HVAC components to anticipate when parts will need attention. By analyzing factors like motor vibration, bearing temperature, or compressor cycling patterns, the system can schedule maintenance activities before failures occur. This proactive approach reduces costly downtime, extends equipment lifespans, and ensures that the HVAC system remains reliable and efficient. Building owners can budget and plan more effectively, improving the overall economic performance of their HVAC assets.
19. User Preference Learning
Advanced AI systems can learn individual or group comfort preferences and tailor HVAC operation accordingly without manual input.
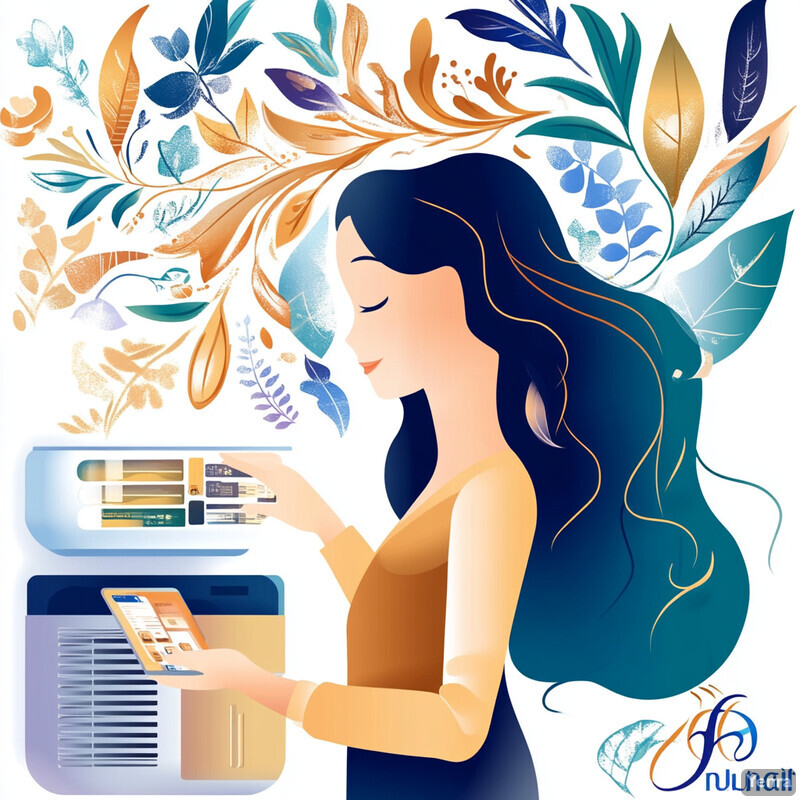
Occupant preferences may vary significantly—some may prefer slightly cooler conditions, while others enjoy a warmer environment. AI-enabled systems use occupant feedback (either explicit, such as through apps or panels, or implicit, such as detecting when people manually adjust thermostats) to learn personal comfort thresholds. Over time, the HVAC system can adapt to cater to these preferences at a group or individual level. This personalization enhances occupant satisfaction, reduces complaints, and builds trust in the building’s climate control capabilities.
20. Continuous Improvement Through Feedback Loops
AI solutions create feedback loops that monitor performance, energy usage, and occupant comfort, refining strategies over time for persistent improvements.
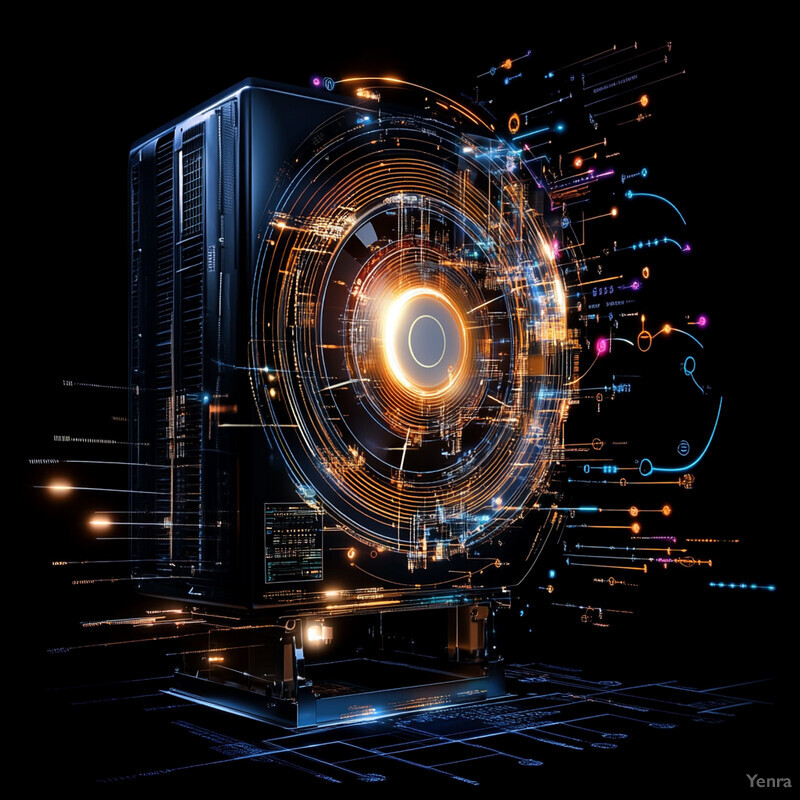
AI’s learning capabilities do not end once an initial optimization is found. Continuous improvement involves establishing feedback loops, where the HVAC system consistently monitors outcomes (energy usage, comfort levels, maintenance costs) and feeds this data back into the decision-making engine. Over time, the system refines its algorithms and strategies based on what works well and what does not. As new data comes in—changes in building usage, new equipment installations, fluctuating energy prices—the HVAC system evolves, ensuring that improvements are persistent, cumulative, and responsive to the ever-changing building environment.