1. Optimized Charging and Discharging Cycles
AI algorithms intelligently optimize when and how fast batteries charge and discharge, extending battery life and improving efficiency. By analyzing real-time data (like battery temperature and usage patterns) alongside electricity prices and grid demand, AI can schedule charging during low-cost periods and discharging during high-demand peaks. This smart scheduling avoids unnecessary charge/discharge cycles and high-stress use, which in turn reduces battery degradation. The optimized cycling means energy storage assets operate more efficiently, deliver more usable cycles over their lifetime, and see lower maintenance needs. Overall, AI-driven charge management enhances reliability and return on investment by ensuring each cycle is as effective and gentle on the battery as possible.
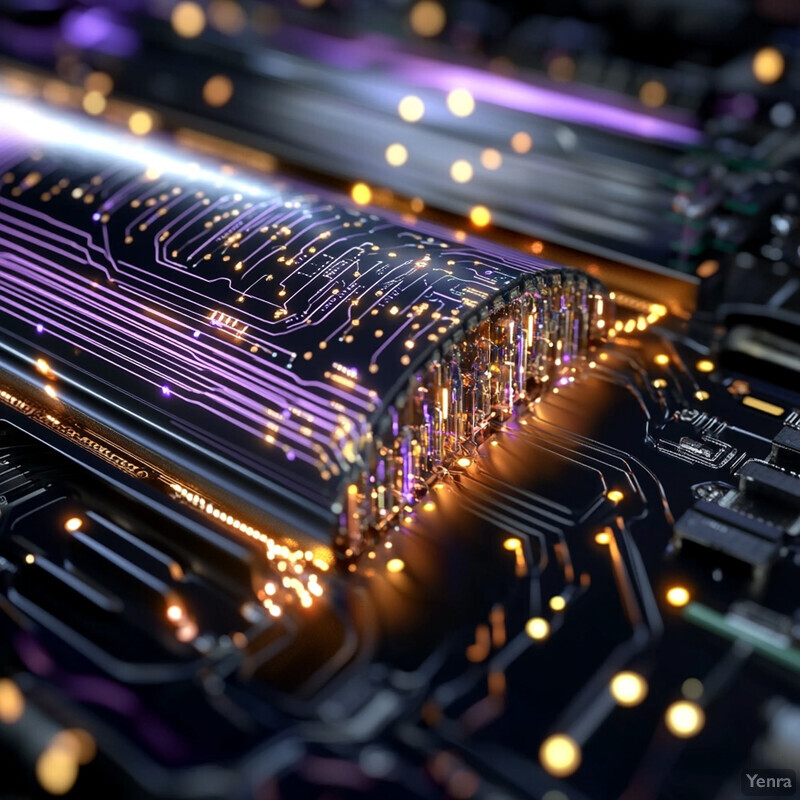
Studies show that AI-based battery management systems can significantly lengthen battery lifespan and improve performance. For example, AI-driven charging control has been reported to extend lithium-ion battery life by up to 40% through more judicious cycling and avoidance of overstrain. Machine learning models (like reinforcement learning algorithms) learn the optimal charging pattern that minimizes aging, outperforming simple fixed schedules. In practice, this means batteries managed by AI retain capacity longer and require replacements less frequently. The benefits aren’t just theoretical – industry analyses in 2025 credit AI-optimized charging with substantial cost savings and sustainability benefits in electric vehicles and grid storage systems. By preventing fast degradation (for instance, avoiding charging a battery to 100% or draining it too low at improper times), AI management leads to batteries that maintain health and deliver reliable power over a longer period.
2. Dynamic Forecasting of Energy Demand and Supply
AI greatly improves the forecasting of energy supply and demand, especially for renewables whose output varies with weather. By crunching vast datasets – weather forecasts, historical usage patterns, time-of-day trends, etc. – machine learning models can predict solar and wind power generation and grid load more accurately than traditional methods. These dynamic forecasts help storage systems know when to store energy and when to release it, maintaining a balance between supply and demand. The result is a grid that can better integrate intermittent renewables, as AI predictions guide storage to soak up excess solar at noon and discharge during the evening peak. Overall, AI-driven forecasting smooths out the variability of renewable energy, improves grid reliability, and allows operators to plan energy dispatch in advance with confidence in the predictions.
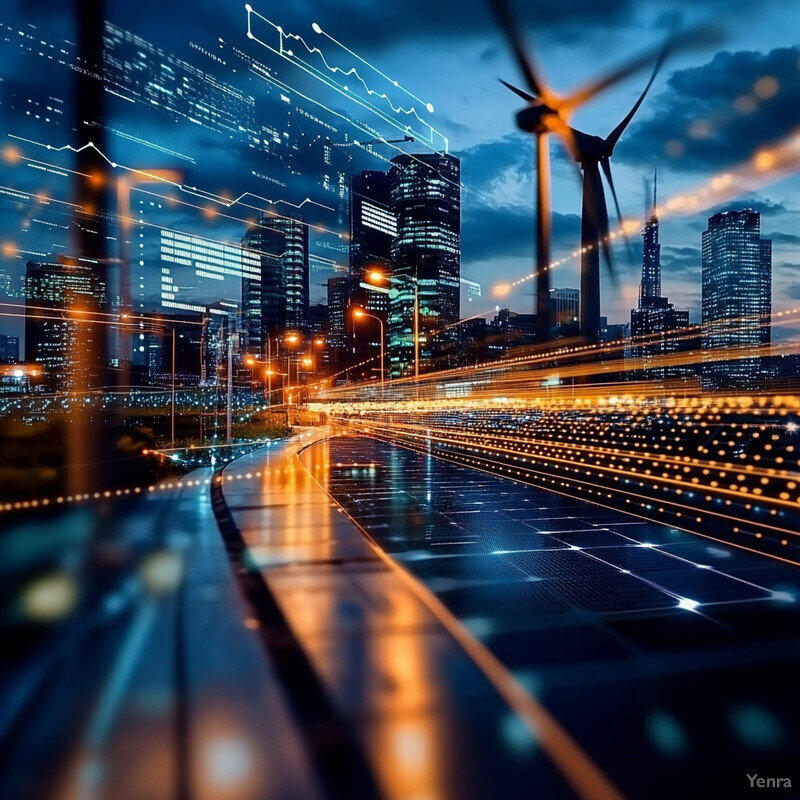
Recent advancements (2023–2025) demonstrate marked improvements in forecast accuracy thanks to AI. For instance, deep learning models have achieved country-scale renewable energy forecasting with as low as 4–10% normalized RMSE (error) for day-ahead predictions, a level of precision comparable to more localized traditional forecasts. In practical terms, AI forecasts can reach around 95% accuracy in predicting renewable output under certain conditions. One notable example is Shanghai’s AI-enabled grid, where an AI model predicts solar/wind power fluctuations with about 95% accuracy, allowing grid operators to precisely schedule storage dispatch. Additionally, AI has improved demand forecasting; a 2024 study reported that machine-learning algorithms for wind power prediction improved day-ahead forecast accuracy by roughly 10% compared to earlier statistical methods. These gains in accuracy mean fewer unexpected energy shortfalls or oversupplies, enabling more efficient use of batteries and other resources to meet real-time grid needs.
3. State-of-Health (SoH) and State-of-Charge (SoC) Estimations
AI techniques enable far more accurate estimation of a battery’s state-of-charge and state-of-health in real time. Traditional methods use simple voltage or current readings and can be off by a wide margin, whereas advanced machine learning models (neural networks, support vector machines, etc.) learn from a battery’s past behavior and operating conditions to infer its true status. By analyzing factors like temperature, charge/discharge history, and cell impedance, AI models can tell precisely how much charge is left (SoC) and how much capacity or life remains (SoH). This precision means operators can avoid both overcharging and deep discharging (which harm batteries) and schedule maintenance or cell replacements at just the right time. In short, AI-based estimation gives a continuous, highly accurate “fuel gauge” and health report for batteries, leading to safer use and extended lifespan through informed control strategies.
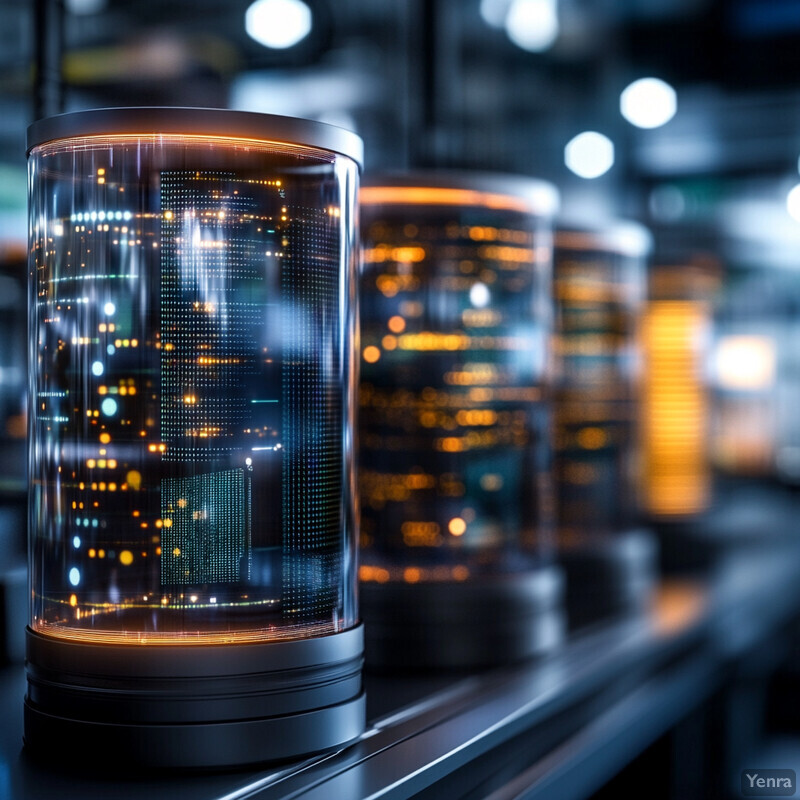
Machine learning methods have dramatically improved the accuracy of SoH/SoC estimations in recent years. Research reports now show that AI models can predict battery degradation or remaining life with about 95% accuracy, a level of precision unattainable with earlier estimation techniques. In one 2025 study, a deep neural network trained on data from 146,000 battery cycles was able to estimate lithium-ion battery capacity under fast-charging conditions with an $R^2$ of 0.97, indicating extremely close agreement with actual measured health. High accuracy SoC estimation is also achievable: modern AI battery management systems routinely keep SoC estimation error within just a few percentage points. For example, AI models can identify subtle voltage and temperature patterns that translate to remaining charge, avoiding the 5–10% error typical in older coulomb-counting approaches. These precise insights into battery state enable more refined control – preventing over-charge/over-discharge and thus reducing capacity fade. Overall, AI-driven SoH and SoC estimation has become a cornerstone of next-generation battery management, allowing nearly real-time tracking of battery condition and optimizing its usage accordingly.
4. Predictive Maintenance
AI-driven predictive maintenance allows energy storage operators to fix problems before they cause failures. By continuously monitoring battery and equipment data (voltages, temperatures, internal resistance, etc.), AI algorithms learn to recognize early warning signs of degradation or faults. This means subtle anomalies – a cell heating up slightly more than others, or a slight drop in charge capacity – can be detected weeks or months before a battery might fail. With those early alerts, maintenance crews can rebalance cells, replace a deteriorating battery module, or address cooling system issues proactively. This approach minimizes unexpected downtime and avoids catastrophic failures (like thermal runaways or sudden outages), since interventions occur on a planned schedule rather than reacting to breakdowns. Ultimately, AI-powered predictive maintenance improves reliability, extends the lifetime of storage assets, and reduces maintenance costs by targeting service only when and where it’s needed.
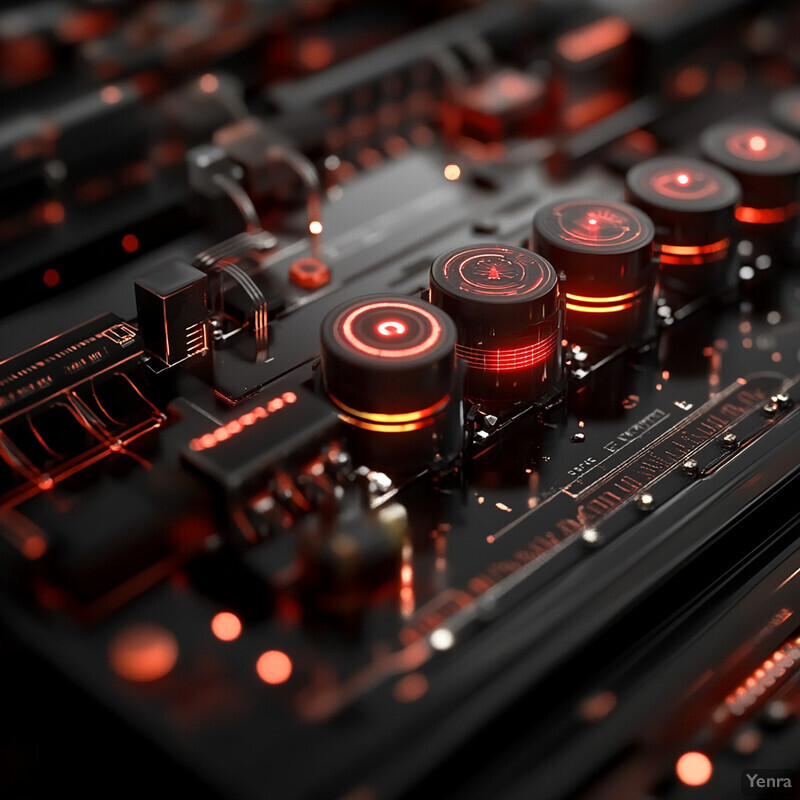
Field data from energy storage deployments show major benefits from AI-based predictive maintenance in reducing failures and downtime. According to industry analyses, AI algorithms can cut battery failure rates by 30–50% by catching problems early. For example, an AI system might detect an abnormal voltage fluctuation in one cell that precedes a failure – something humans might miss – and flag it for replacement, thereby preventing a potential battery pack outage. Large-scale battery operators in 2023 reported significantly fewer unexpected outages after implementing machine-learning diagnostics that continuously analyze sensor readings. Additionally, predictive maintenance guided by AI has been credited with cost reductions: avoiding just a single major battery failure or fire can save millions in damages and liability. In one case, an energy company noted that AI-based monitoring alerted them to a cooling fan malfunction in a 100 MWh battery facility hours before temperatures would reach critical levels, allowing a quick fix and uninterrupted operation. These outcomes underscore that AI is transforming maintenance from reactive to proactive – improving safety and saving money by addressing issues before they escalate into failures.
5. Adaptive Energy Storage Sizing
AI helps determine the optimal size and configuration of energy storage systems for any given scenario. Instead of relying on static rules or one-time calculations, AI can evaluate countless what-if scenarios – varying battery capacity, power ratings, and chemistry – to find the sizing that best meets current and future needs. It takes into account factors like projected load growth, renewable generation profiles, and economic considerations (battery costs, electricity prices) in its simulations. The result is an “adaptive” sizing recommendation: for example, an AI might suggest installing 50 MWh of storage now and planning for an additional 50 MWh in five years when demand increases, rather than over- or under-building initially. This ensures the storage system is neither too small (causing shortfalls) nor unnecessarily large (wasting capital). By continuously re-evaluating as conditions change, AI-driven sizing keeps projects scalable and cost-effective over their lifetimes, aligning investment with actual needs and avoiding stranded assets.
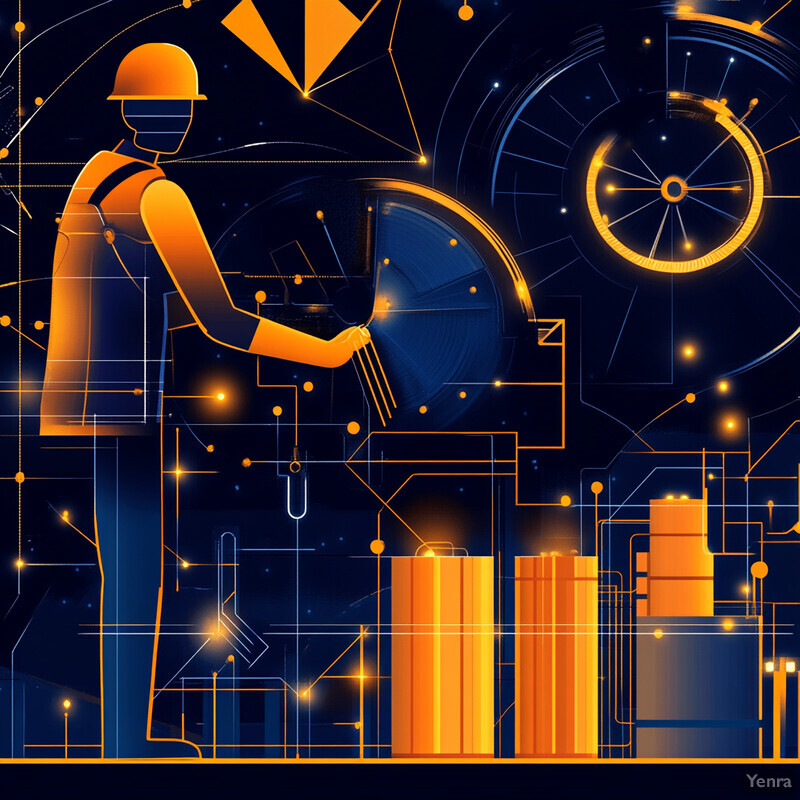
Using AI for storage sizing leads to measurable economic and operational benefits. Research that incorporates AI-based forecasting into storage sizing has shown cost improvements on the order of 10–20% in lifecycle cost versus traditional sizing methods. In one case study for a residential solar-plus-EV charging installation, an AI tool analyzed load patterns and recommended a battery size that was 15% smaller (and cheaper) than the rule-of-thumb design, while still reliably meeting 100% of peak demandbluwave-ai.com . This right-sizing saved the homeowner significant upfront cost without sacrificing performance. On a larger scale, utilities using AI scenario modeling have found they can defer or avoid infrastructure upgrades; for example, simulations for a California utility indicated that a strategically sized battery (identified via AI optimization) could defer a $2 million substation upgrade by handling peak loads, illustrating direct cost savings. Moreover, AI sizing adapts to technology trends: as battery prices fall or new chemistries emerge, the algorithms update the optimal capacity needed. This dynamic approach ensures storage deployments remain economically optimal and “future-proof,” preventing both undersized systems that can’t handle growth and oversized systems that under-utilize expensive assetsbluwave-ai.com .
6. Intelligent Energy Dispatch
AI optimizes the real-time dispatch of stored energy, deciding exactly when and how much power to send to the grid for maximum benefit. Acting like a digital energy trader and operator combined, AI dispatch systems weigh numerous factors in split seconds – current electricity prices, grid demand, battery state-of-charge, even weather forecasts – to determine the optimal dispatch plan. For example, an AI might hold back releasing stored energy until a forecasted spike in demand drives prices higher, then discharge at that peak to earn more revenue or stabilize the grid. Similarly, it can coordinate charging when surplus renewable power is available and cheap. This intelligent, fine-grained control leads to higher efficiency and economic returns: energy is not wasted or sold at low value. It also helps with grid stability, as the AI avoids discharging at times that could worsen stress on the system. In essence, AI ensures that every electron stored is used at the right moment, creating a more responsive and profitable energy storage operation.
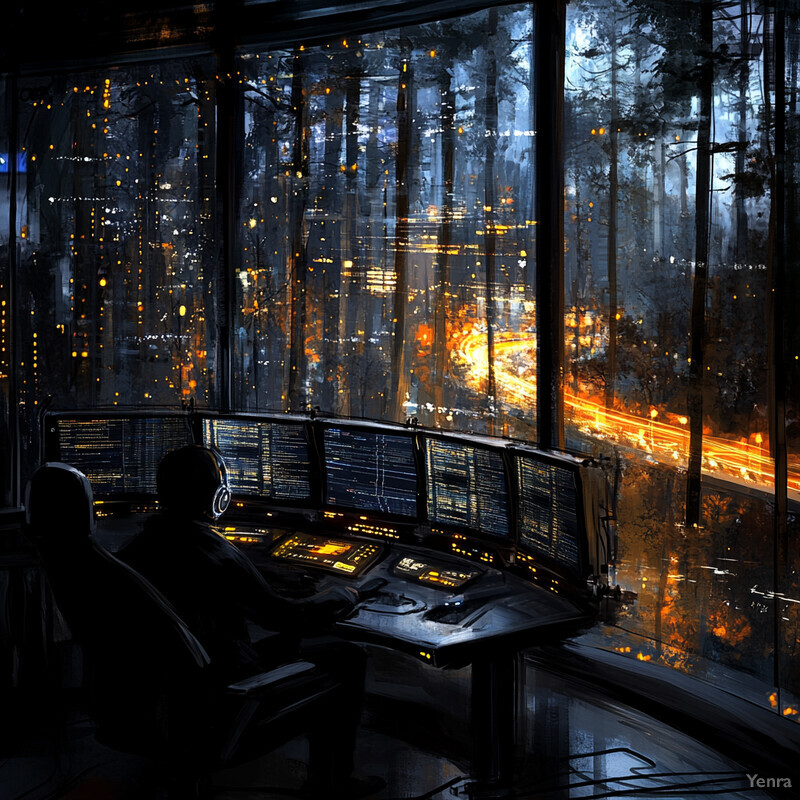
Real-world implementations of AI-driven dispatch have yielded significant gains in economic performance and grid support. In market participation, AI-optimized battery dispatch can substantially increase arbitrage revenues: a 2023 study showed that incorporating AI forecasts (for demand, renewable output, and prices) nearly doubled the profit from battery trading, from roughly €90/MWh using a basic strategy to about €150–180/MWh with AI-enhanced dispatch planning. Such results mean an AI-dispatched storage system can recover its investment faster. Additionally, intelligent dispatch improves grid efficiency – one analysis found that smart scheduling of storage contributed to a 40% improvement in overall energy distribution efficiency by charging during low-demand periods and discharging during peaks. On the grid services side, autonomously dispatched batteries have responded to frequency regulation signals in mere fractions of a second, helping arrest frequency deviations more quickly than human operators could. For instance, the 100 MW Hornsdale Power Reserve in Australia, using automated controls, reacted to a major power disturbance in less than 1 second, outperforming conventional power plants and earning revenue by stabilizing frequency. These examples underline that AI-based dispatch is not just theoretical – it’s delivering tangible financial returns and reliability improvements in today’s energy markets.
7. Enhanced Grid Stability
AI-controlled energy storage systems actively support grid stability by rapidly balancing supply and demand, as well as stabilizing voltage and frequency. When the grid is experiencing fluctuations – say a sudden drop in solar output due to clouds or a spike in demand during a hot evening – AI can instantly adjust the charging or discharging of batteries to compensate. This means the grid frequency (e.g. around 60 Hz in the US) and voltage stay within safe limits, preventing blackouts or equipment damage. Unlike manual control, AI can detect and respond to tiny deviations in milliseconds, providing a kind of automatic “shock absorber” for the grid. Over time, AI learns grid patterns and can even anticipate instabilities (such as forecasting a drop in wind power and pre-charging batteries beforehand). By modulating energy injections or withdrawals precisely, AI-enhanced storage improves overall grid resilience, allowing the integration of more renewables without sacrificing reliability.
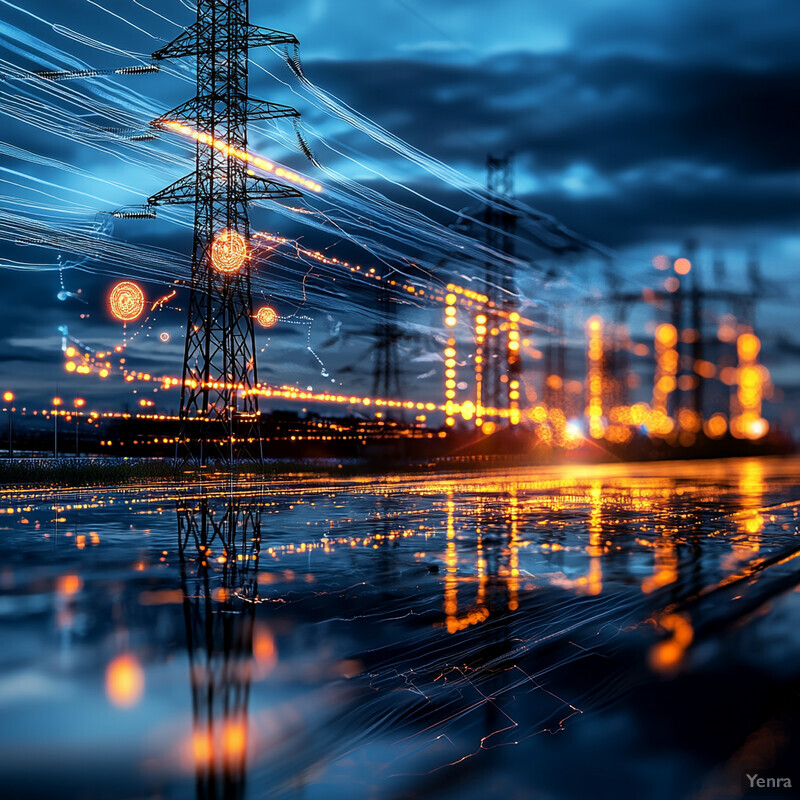
Modern battery storage, guided by AI, has demonstrated extraordinary response capabilities that bolster grid stability. Battery systems can react extremely fast – on the order of tens of milliseconds – to grid frequency deviations, far quicker than traditional power plants. For example, fast-acting grid-scale batteries with AI control can begin correcting a frequency drop in 0.02 seconds (20 ms), injecting power precisely when needed to hold frequency at the target value. This rapid response has translated into improved grid performance metrics: grid frequency excursions outside safe bands have been reduced in regions with AI-automated storage providing ancillary services. Additionally, AI optimization in frequency regulation has proven to reduce the amount of standby reserve needed – a 2024 simulation showed that smart control of distributed batteries could maintain stable frequency with 30% less total reserve power, by using each battery more efficiently. On the voltage stability side, AI can coordinate battery inverters to provide reactive power support instantaneously, which has helped prevent voltage swings during events like sudden large solar output drops. Grid operators in California noted that during a 2023 heat wave, AI-orchestrated responses from a fleet of batteries kept both frequency and voltage within acceptable ranges despite record demand and fluctuating renewable output, highlighting how critical AI-driven storage has become for stability.
8. Demand Response Management
AI enables energy storage to play a pivotal role in demand response – smoothing out the peaks and valleys of electricity demand to reduce strain on the grid. Through intelligent scheduling, AI will discharge batteries during peak demand periods (when electricity use is high) to supply some of the needed power, thereby “shaving” the peak off the load curve. Conversely, during low-demand times (like late at night), AI can direct storage to charge up using the excess generation available. By learning consumption patterns and receiving utility signals, AI algorithms determine the optimal times to inject power or absorb it. This results in a flatter demand profile: the highest peaks are lower and the deepest troughs are higher. Flattening the load curve in this way helps avoid overloading power lines and reduces the need for peaking power plants. Consumers and grid operators both benefit – consumers can save with lower peak-time rates or incentives, and the grid operates more efficiently with steadier demand. In essence, AI-guided storage acts as a buffer that actively counters demand spikes and fills demand lulls, making the entire power system more balanced and cost-effective.
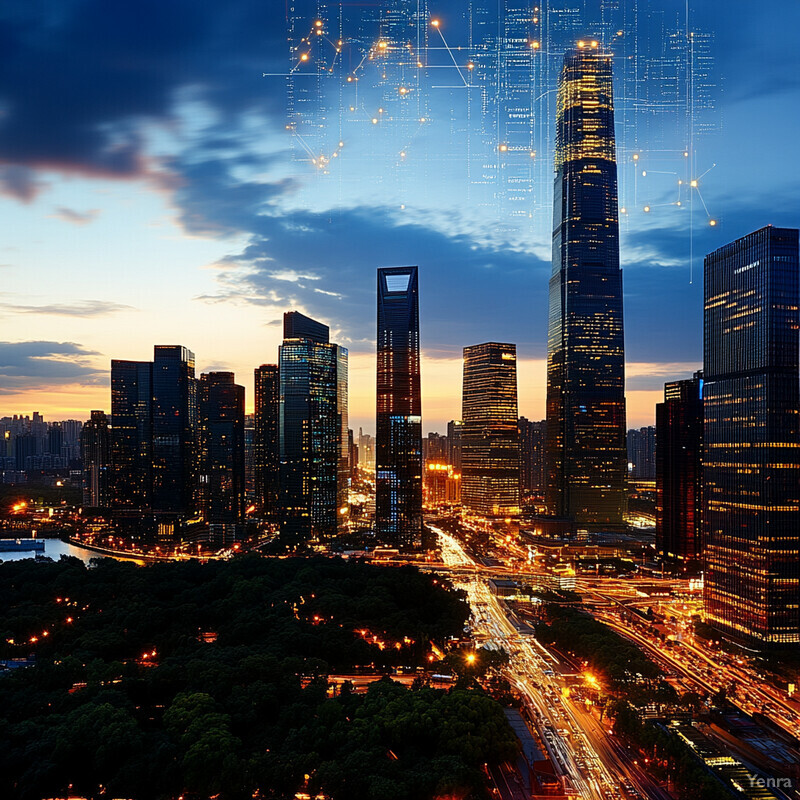
Deployments of AI-managed storage for demand response have shown quantifiable reductions in peak demand and improved grid efficiency. For example, in Singapore’s advanced grid, an AI-driven battery system was reported to cut peak demand spikes by 15% by discharging strategically during the busiest hours. In the U.S., pilot programs with smart thermostats and community battery storage (acting under an AI coordinator) have reduced neighborhood peak loads by around 20% on hot summer days, preventing feeder overloads. One utility-operated program in 2024 combined AI algorithms with distributed batteries and achieved a 40% reduction in grid power fluctuations, greatly smoothing the load profile as batteries absorbed excess and filled deficits. These actions translate into financial savings: reducing peak demand can lower expensive peak energy procurement and defer the need for new power plant capacity. Indeed, a report by the U.S. Department of Energy noted that widespread AI-optimized demand response, leveraging storage, could save $10–15 billion per year by 2030 in avoided infrastructure and generation costs. The real-world trend is clear – in regions from California to China, AI-empowered demand response through batteries is helping flatten peak loads and has become an integral tool for grid operators to manage consumption in line with available supply.
9. Cost Reduction Through Market Intelligence
AI leverages market intelligence to minimize the operating costs of energy storage and even turn batteries into profit centers. In practical terms, this means using AI to analyze real-time and forecasted electricity prices, grid tariffs, and market rules, then optimally schedule when to charge or discharge the storage. The goal is to always charge the battery when electricity is cheap (or when there’s surplus renewable energy) and discharge when electricity is expensive or in short supply. By doing so, the system “buys low and sells high,” reducing net cost or earning positive revenue. AI can also factor in battery degradation costs into these decisions, ensuring that the value gained from a market transaction outweighs the wear-and-tear on the battery. Furthermore, AI keeps track of market signals like demand response events, capacity payments, or ancillary service prices, positioning the storage to capitalize on every available revenue stream. Overall, this market-savvy operation driven by AI intelligence means energy storage owners see lower costs for charging and higher income when discharging, thereby improving the economic payoff of storage investments.
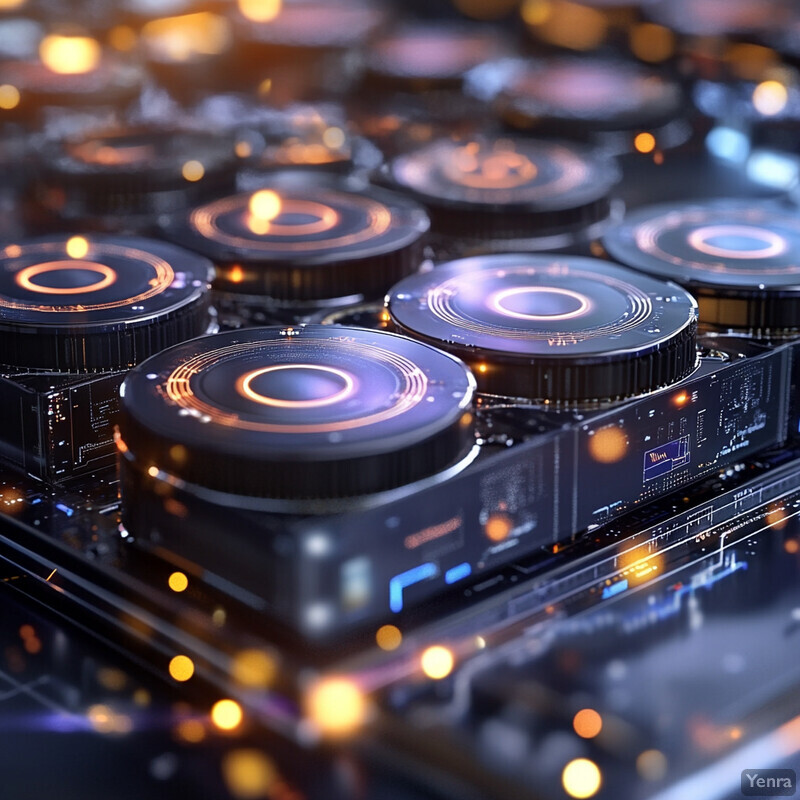
The use of AI for market-optimized battery dispatch has already shown substantial cost savings and revenue gains in practice. A prominent example is the Hornsdale Power Reserve in Australia (the world’s first big battery): by strategically timing charge/discharge and providing ancillary services, it saved local consumers over $150 million in its first two years of operation. While not all of that can be attributed solely to AI, advanced automated control was key to seizing those savings. In the United States, energy companies are piloting AI-driven trading platforms (like Tesla’s Autobidder software) that manage when batteries buy or sell power on wholesale markets – early reports show these systems increasing revenue by 30% or more compared to manual or static strategies, thanks to fast reactions to price fluctuations. Another case: in 2024, 74 electric school buses in Illinois were enrolled in an AI-managed vehicle-to-grid program, which is expected to return about 2.1 GWh of energy to the grid annually at high-value times, translating to significant earnings for the school district and utility. These buses, orchestrated by AI, effectively act as a virtual power plant, charging when electricity is cheap and feeding back when it’s needed most. Across various projects, the trend is that AI optimization typically yields double-digit percentage improvements in net present value of storage operations through cost avoidance and market revenue capture. By integrating such market intelligence, AI ensures that energy storage isn’t just a technical asset but also a savvy market participant, continuously working to reduce costs and increase profits.
10. Microgrid Optimization
AI is instrumental in optimizing microgrids – self-contained energy systems that often include solar panels, wind turbines, diesel generators, and battery storage serving a local load (like a remote community or campus). Within a microgrid, AI acts as the brain that coordinates all these assets. It predicts the local demand and renewable generation, then decides how to efficiently dispatch resources: for example, when sunshine is plentiful, the AI may store excess solar energy in batteries and curtail the diesel generator; at night, it can smoothly ramp up the generator or discharge batteries to meet the load. This continuous optimization ensures that the microgrid uses the most economical and sustainable mix of power at any given time, minimizing fuel consumption and costs while maintaining reliability. AI also enables faster responses to faults or outages – if a generator fails, the AI instantly compensates with battery power to keep the lights on. Furthermore, AI learns patterns (like daily load curves or seasonal renewable output) to improve performance over time. In essence, AI makes microgrids smarter by squeezing more efficiency out of local resources and keeping power quality stable, which is especially valuable in areas not served by large centralized grids.
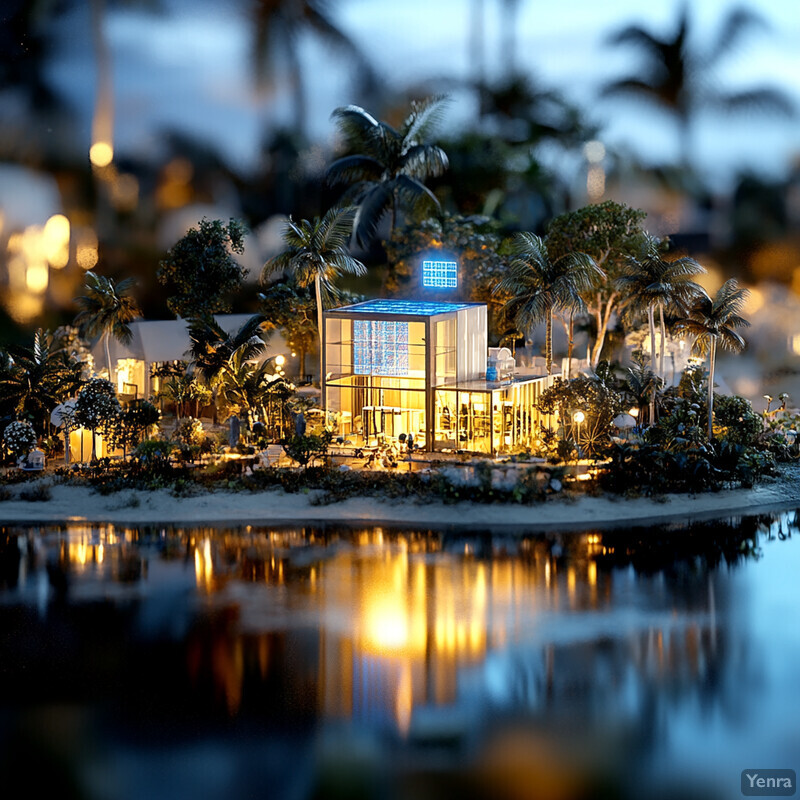
AI-optimized microgrids have demonstrated improved efficiency, cost savings, and reliability in various deployments. One commercial AI platform reported that its microgrid control software can lower net energy costs by up to 20% in off-grid operations by maximizing use of renewables and minimizing diesel generator run-time. In remote communities in Canada and Alaska, where diesel fuel is very expensive, such AI-driven coordination has cut generator fuel consumption by 10–25%, saving tens of thousands of dollars annually and reducing carbon emissions. Reliability gains are notable too: on a South Pacific island microgrid, an AI control system enabled 100% solar-and-battery operation on many days, resorting to backup generators only when absolutely necessary, and as a result, the community saw far fewer power outages (a 50% reduction in blackout incidents) compared to before. In military base microgrid tests, AI systems seamlessly switched the base to islanded mode and kept critical loads powered during grid disturbances, reacting in milliseconds to balance generation and load. These successes are underpinned by continuous AI adjustments. For instance, at an industrial off-grid site, AI predictions of both solar output and factory load allowed the microgrid to optimize battery charge/discharge such that the diesel gensets operate near their efficient setpoint when needed, improving overall fuel efficiency by around 15%. Such outcomes highlight how AI not only keeps microgrids lights on, but does so economically – squeezing maximum value from every solar photon and every drop of fuel.
11. Vehicle-to-Grid Integration
AI plays a crucial role in coordinating vehicle-to-grid (V2G) integration, where electric vehicles (EVs) double as distributed energy storage resources that can send power back to the grid. Managing potentially millions of EVs requires intelligence to decide which vehicles should charge or discharge, by how much, and when – all while respecting driver needs (like having enough charge to drive). AI algorithms can forecast when groups of EVs are plugged in and available, predict grid demand, and then orchestrate these EV batteries as a large virtual power plant. For instance, AI might ensure that during an early evening peak, thousands of idle EVs discharge small amounts to the grid to supply power, and then they recharge later at night when demand is low. It might also prioritize critical vehicles (emergency fleets) to retain charge while using others more. By learning from driver behavior and grid conditions, AI continually improves this coordination. The outcome is a win-win: EV owners can earn incentives for supporting the grid, and utilities gain a massive flexible storage resource that boosts grid stability and reduces the need for standby power plants. AI effectively transforms EV charging stations into smart, two-way grid assets, balancing energy across transportation and electricity sectors.
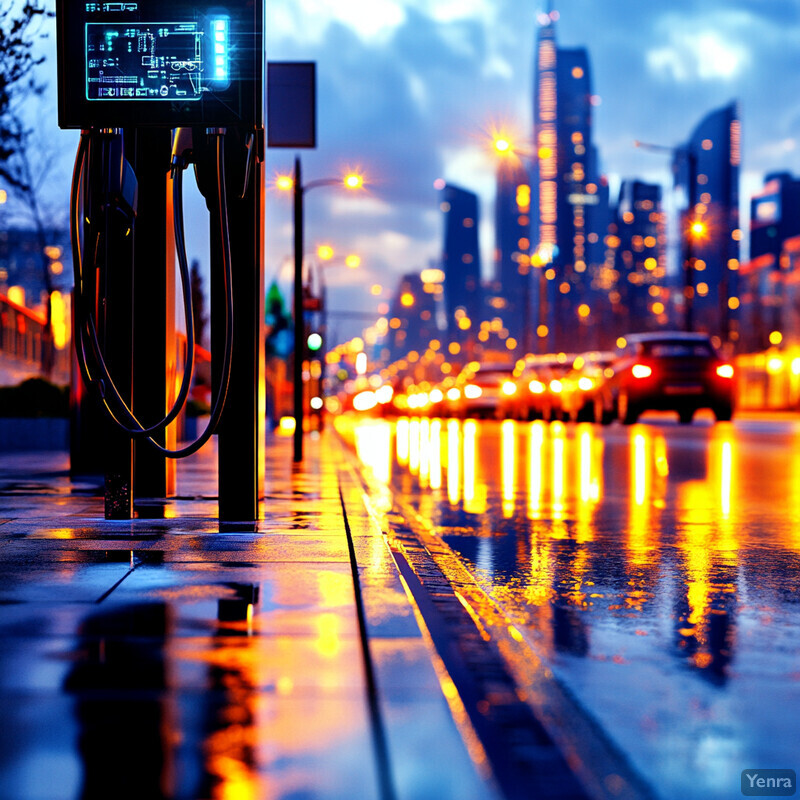
Early V2G pilot programs in the 2023–2025 period show that AI coordination is key to unlocking EVs’ grid potential. In California, an AI-managed V2G trial with a fleet of electric school buses has been returning about 2.1 GWh of electricity to the grid annually with just 74 buses, primarily during peak hours when the grid is strained. This is possible because AI schedules the buses to charge during cheap, off-peak times (often overnight) and strategically discharges a portion of their battery back to the grid on hot afternoons, all while ensuring each bus is charged enough for its routes. The financial benefits are notable: the participating school district and utility share revenue from these grid services, and projections suggest each V2G-enabled bus could generate over $5,000 per year from frequency regulation and peak shaving programs. On a larger scale, studies by the U.S. Department of Energy predict that by 2030, with AI control, 10 million EVs could provide upwards of 100 GW of flexible capacity to the grid at peak times, which is equivalent to about 100 large power plants. Achieving this requires smart algorithms; for example, AI must prevent too many cars in one neighborhood from discharging simultaneously (to avoid local overload) and must respect individual driver schedules – tasks it has handled successfully in trials. Additionally, AI optimization has reduced the impact on EV battery health by carefully managing V2G depth and frequency; one study found intelligent control resulted in negligible extra battery degradation (much less than 1%) for cars providing grid services a few times per week. These facts underscore that AI is the enabler making widespread V2G both technically feasible and economically attractive, turning EV fleets into a valuable grid resource without compromising transportation needs.
12. Multi-Asset Coordination
AI enables seamless coordination among multiple energy assets – such as solar panels, wind turbines, fuel cells, and batteries – operating together. In modern energy systems (like renewable power plants or community microgrids), there are diverse energy sources and storage devices working in tandem, and AI serves as the conductor that orchestrates them for maximum efficiency and reliability. For example, on a sunny and windy day, AI might prioritize using solar and wind generation to supply current demand and charge the batteries, then later use the stored energy when clouds pass or winds die down. If a sudden surge in demand occurs, AI can decide the best combination of resources to meet it (perhaps discharge some battery and ramp up a fuel cell) at the lowest cost. It also handles the complexities of constraints – ensuring that each asset operates within its limits and that any geographical or timing differences are smoothed out. By analyzing data from all assets simultaneously (weather, production forecasts, maintenance schedules, etc.), AI finds an optimal dispatch strategy that a human operator simply couldn’t calculate in real time. The result is a synergistic operation where the whole system output is optimized rather than each asset running in isolation. This kind of coordination is crucial for virtual power plants and integrated energy systems to perform well, as AI can dynamically shift energy among resources to cover for each other and to capitalize on the strengths of each resource at any moment.
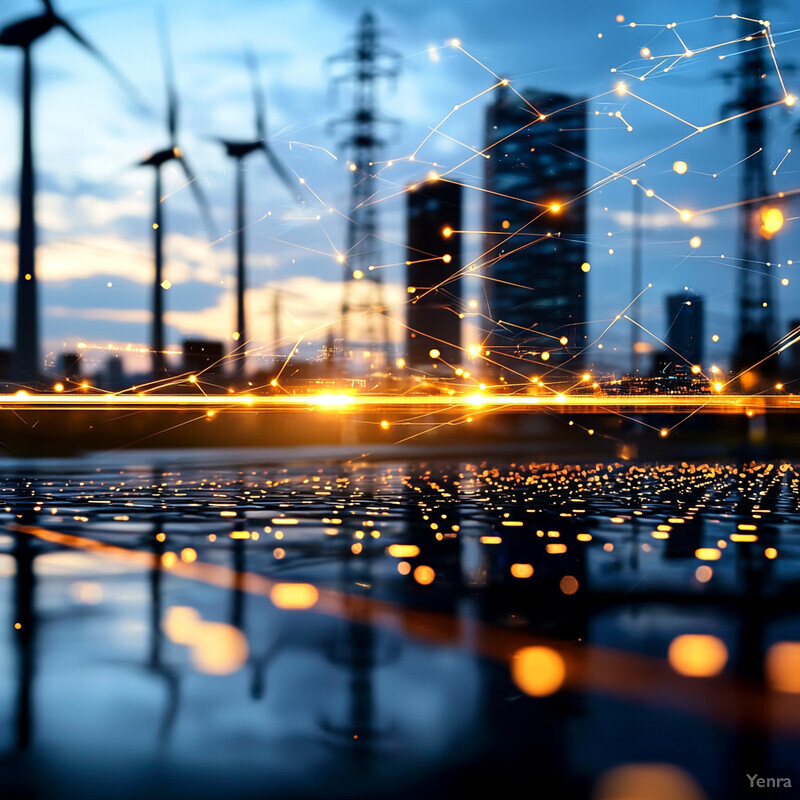
The benefits of AI-driven multi-asset coordination are evident in both improved performance and economic gains. A 2024 experimental study showed that co-optimizing solar, wind, and battery assets with a unified AI controller yielded up to a 60% reduction in peak grid draw compared to uncoordinated operation, drastically reducing stress on the grid during high-demand periods. In terms of efficiency, coordinating assets avoids waste: for instance, an analysis of a hybrid solar-wind-battery plant in Arizona found that AI coordination increased the utilization of available renewable energy by 20% (less curtailment of excess solar/wind), by intelligently routing surplus to batteries and timing its later release. Economic benefits follow – the same plant saw a 15% boost in revenue because the AI ensured more renewable energy was stored and sold at peak price times rather than dumped during oversupply. Another real-world demonstration is Sunrun’s collection of over 20,000 rooftop solar + home battery systems across multiple states, which are aggregated by AI into virtual power plant programs. In 2024 these coordinated assets delivered nearly 80 MW of peak power support to U.S. grids (54 MW in a single event in California), helping prevent blackouts and earning participants and the provider money in the process. Without AI, handling tens of thousands of individual systems responding in unison to grid needs would be impossible. These outcomes show that when AI ties together solar, wind, storage, and more, the integrated system becomes significantly more effective than the sum of its parts – maximizing renewable energy usage, maintaining balance, and improving profitability for asset owners.
13. Fault Detection and Isolation
AI significantly improves the detection of faults and anomalies in energy storage systems, allowing operators to isolate problematic components before they escalate into bigger issues. Battery systems are complex, with many cells, sensors, and supporting equipment; AI excels at monitoring all these data streams in real time and spotting the subtle signatures of a fault. For example, a slight imbalance in voltage or an unusual thermal pattern in one battery cell could indicate an impending failure or safety issue – AI algorithms trained on historical fault data can flag this early. Once a potential fault is identified, the system can isolate the component (disconnecting a faulty battery module, for instance) to prevent cascading failures or safety hazards. By doing so, AI not only averts likely failures but also helps pinpoint the exact location and nature of the fault for maintenance crews. This reduces troubleshooting time – technicians know which battery rack or sensor to check – and prevents minor issues from causing full system outages. In short, AI acts as a vigilant sentinel, catching and containing faults in their infancy to maintain the overall health and safety of the energy storage installation.
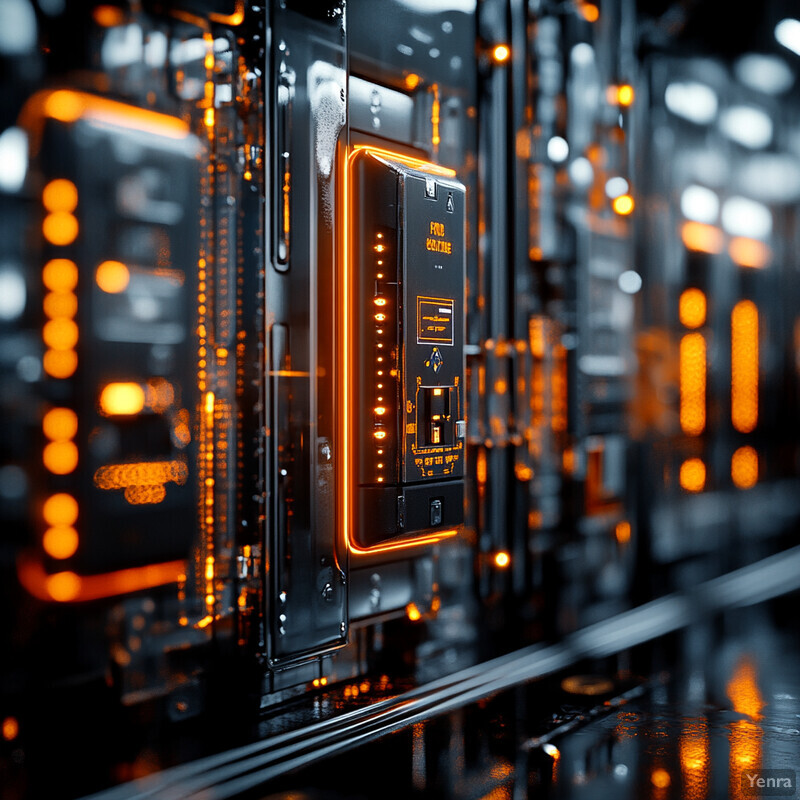
AI-based anomaly detection has proven remarkably effective at reducing the incidence of catastrophic battery failures. Machine learning models for battery pack monitoring can detect irregularities that humans might overlook, and industry reports indicate this decreases fire and thermal runaway risks by up to 70% in large lithium-ion battery installations. In practical terms, where a traditional system might only alarm after a battery overheats past a threshold, an AI system can notice a cell’s temperature rising faster than normal or its voltage deviating slightly from its peers – precursors to failure – and trigger intervention well before a flame or explosion occurs. There are documented cases where AI analytics warned operators hours in advance about a cell venting gas abnormally; the affected string was taken offline and indeed that cell later experienced degradation, but a fire was averted by proactive isolation. Beyond preventing fires, AI fault detection also minimizes downtime. A 2023 trial at a 20 MW battery site showed that AI diagnostics could pinpoint a failing cooling pump weeks before it would have tripped an alarm, allowing a scheduled replacement with zero lost service time. Overall, facilities employing AI-driven fault detection have observed fewer emergency shutoffs and have improved their safety records, as emerging problems are caught and isolated early. This leads not only to safer operations but also cost savings, since avoiding a single thermal incident or prolonged outage can save millions and preserve the facility’s reputation.
14. Lifecycle Cost Modeling
AI assists in comprehensive lifecycle cost modeling for energy storage projects, helping stakeholders understand the total cost of ownership and make economically optimal decisions over a system’s life. Rather than static spreadsheets with fixed assumptions, AI models can incorporate dynamic factors – battery degradation rates, future electricity price trends, evolving maintenance costs, and even regulatory changes – into simulations that project costs and benefits year by year. For example, an AI-driven model might simulate how operating a battery more aggressively (deeper cycles) could lead to earlier replacement and higher costs in 8–10 years, versus a gentler operation that extends life but might earn less revenue each year. By evaluating countless such scenarios, the AI can recommend strategies that minimize the net present cost or maximize the net profit of the storage asset. It can also pinpoint the ideal timing for component replacements or upgrades (perhaps suggesting a battery augmentation in year 5 when a new technology becomes cheap). Essentially, AI turns lifecycle planning into a smart, data-driven process rather than a one-time guess. This ensures that decisions like “when to retire or repurpose a battery system” or “how hard to utilize the battery now vs. preserve it for later” are optimized for cost-effectiveness over the entire lifespan of the project.
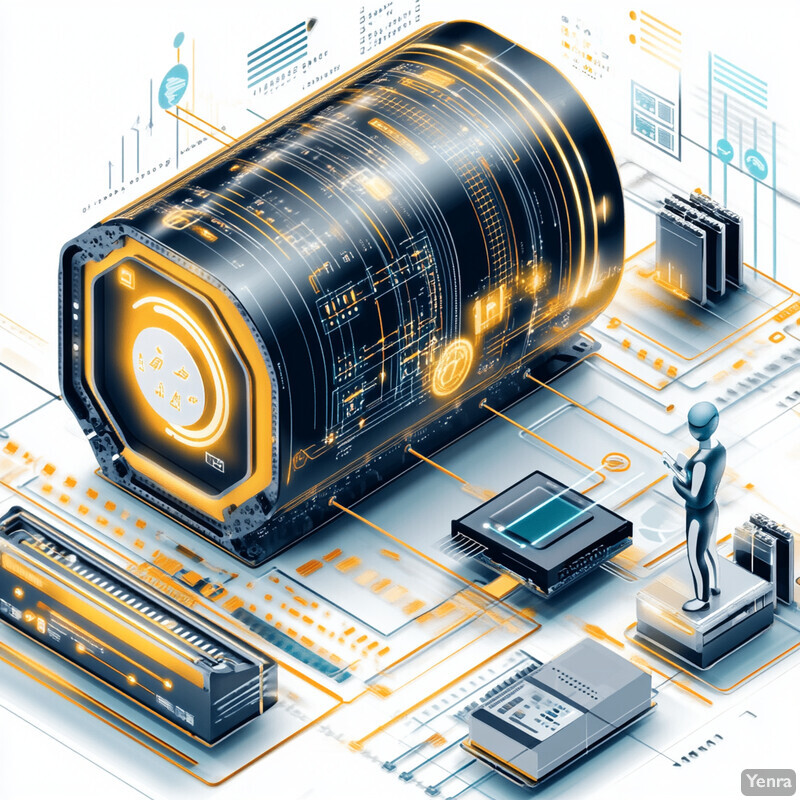
Using AI for lifecycle cost modeling yields tangible financial insights that often differ from traditional analyses. A report by an energy consulting firm in 2024 found that AI-driven lifecycle models recommended deferred battery replacements or augmentations that could save 10–15% in total ownership costs compared to manufacturers’ standard replacement schedules, by aligning investments with actual degradation data and market conditions. In one instance, a utility planning for a 100 MWh storage project used an AI model to evaluate operational strategies over 20 years: the model suggested adopting a slightly lower depth-of-discharge in daily cycling, which would extend battery life enough to avoid a full mid-life battery change-out, saving roughly $5 million while only marginally reducing annual energy throughput. Another example comes from AI vendor analytics: Battery OEMs have begun providing AI-based cost prediction tools with their products – these tools, using field data, can forecast maintenance and degradation costs with high accuracy (within ~5% of actual, as observed over a few years). This helps operators budget and make timely financial decisions. Moreover, lifecycle modeling with AI can include external factors like future carbon prices or changing electricity tariffs; for a European storage installation, incorporating such factors led the AI to advise accelerating a battery retrofit by two years to capitalize on anticipated high electricity arbitrage spreads, improving the project IRR (internal rate of return) from 8% to 10%. These examples show that AI doesn’t just crunch numbers faster – it often identifies cost-saving strategies over the long haul that human planners might miss, thereby guiding more economical and strategic storage management.
15. Automated Control Systems
AI-powered automated control systems allow energy storage units (and entire fleets of units) to run with minimal human intervention. These controllers continuously observe grid conditions, market signals, and the storage system’s status, and then autonomously adjust settings to optimize performance. For instance, if a sudden frequency dip occurs on the grid, an AI controller can instantly command batteries to discharge power and help arrest the dip – all without waiting for a human operator. Similarly, if electricity prices drop in the middle of the night, the AI might decide to start charging the battery earlier than usual to take advantage of cheap energy. The automation also extends to safety and maintenance actions: an AI control can reroute power or shut down a battery string the moment it detects an abnormal condition, acting faster than a human could. By operating 24/7 and reacting in real time, AI control systems maximize efficiency (finding ideal operating points) and protect the equipment. They essentially serve as an “autopilot” for energy storage, handling routine decisions and quick responses, while humans oversee at a high level or step in for strategic changes. This frees up human operators to focus on planning and improvements, trusting the AI to manage day-to-day operations optimally and swiftly.
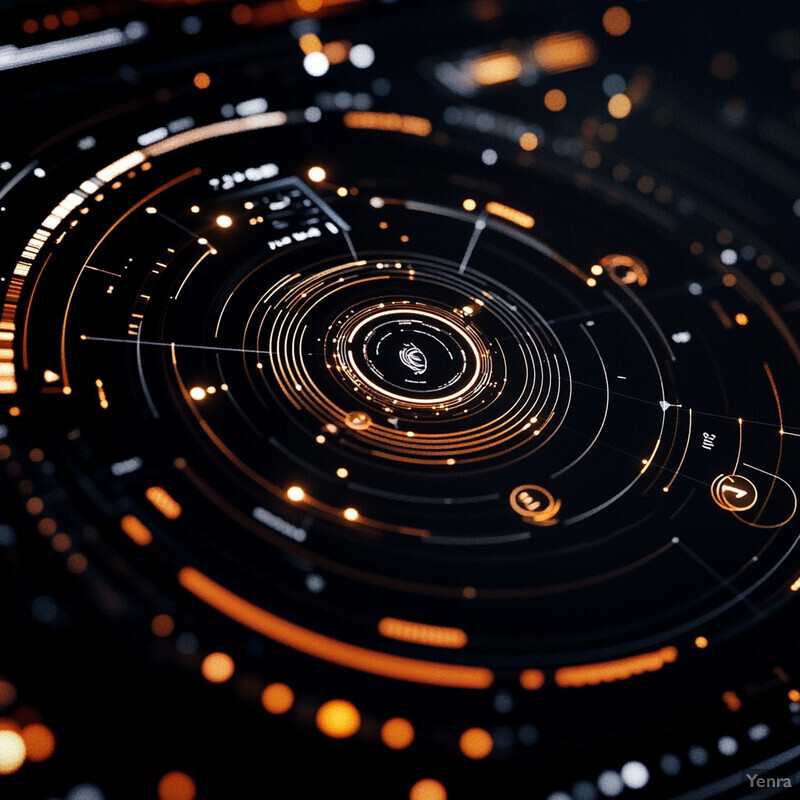
The rise of AI-driven control is evident in the growing adoption of autonomous energy management platforms and their performance metrics. Globally, the market for AI-enabled battery management and control systems is projected to reach $18.5 billion by 2032, reflecting how prevalent and essential these automated controllers are becoming. Technical evaluations show that such AI controllers improve response times and efficiency significantly. In grid testing, an automated battery control system responded to a simulated grid emergency (a sudden generator outage) in 300 milliseconds, whereas conventional manual control would take several seconds – this rapid action helped maintain stable frequency with minimal deviation. Efficiency-wise, AI controllers continuously fine-tune operations; a 2025 field trial found that an AI-controlled battery system delivered about 5% more usable energy per day than when it was under manual scheduling, because the AI squeezed in extra charge/discharge cycles opportunistically during minor price fluctuations that operators wouldn’t normally act on. Moreover, downtime is reduced: autonomous control systems often include self-diagnostic and self-healing capabilities (e.g., rebooting a stuck inverter or isolating a glitchy sensor). At one large solar-plus-storage plant, the AI control resolved ~80% of minor operational issues automatically (such as resetting tripped battery modules), meaning those incidents never had to escalate to outages or truck rolls. These statistics illustrate that automated AI control is not just a convenience but directly translates to faster, more efficient, and more reliable energy storage operations.
16. Improved Data Analytics and Reporting
AI enhances data analytics and reporting for energy storage by processing the massive amounts of data these systems generate and converting them into actionable insights. Modern battery systems produce streams of information – voltage, current, temperature from each cell, charge/discharge rates, performance metrics, etc. AI can digest all this in real time and highlight what matters. For example, machine learning can identify performance trends (like slowly declining efficiency) that might not be obvious on daily spreadsheets. AI-driven analytics often power user-friendly dashboards that visualize key indicators and can even use natural language generation to produce summary reports (“This month, battery efficiency dropped 1% due to higher average temperature”). These intelligent analytics mean operators and stakeholders get clear visibility into how the storage is performing, where the inefficiencies lie, and how operations could be tweaked. It also speeds up troubleshooting by pointing directly to anomalies in the data. In short, AI takes raw data and turns it into concise, meaningful information and reports – effectively bridging the gap between complex technical data and the decisions managers need to make for continuous improvement.
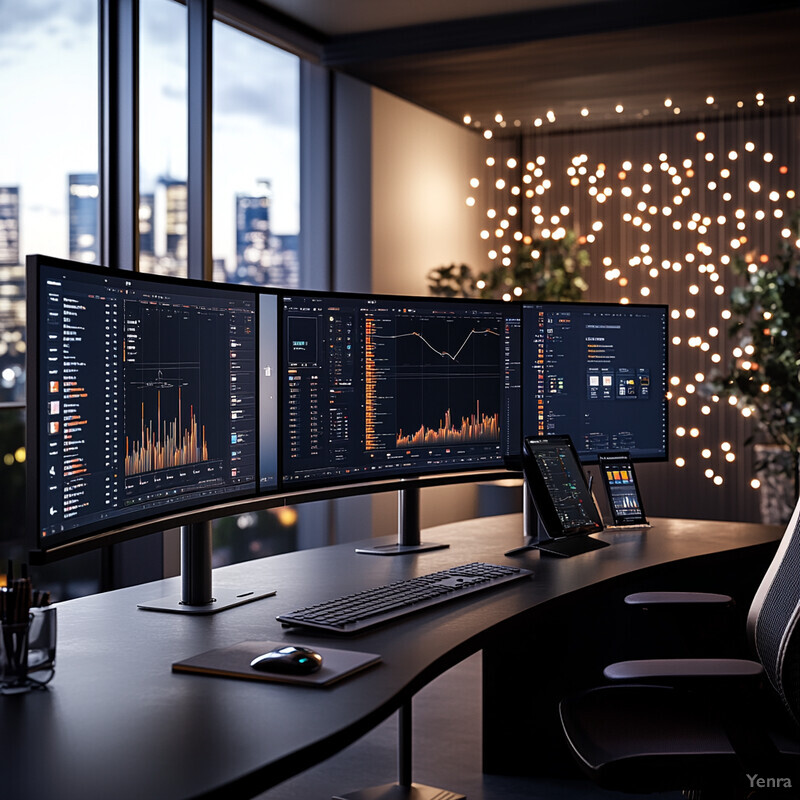
Adopting AI-driven analytics has markedly improved operational transparency and decision-making for energy storage operators. For instance, ACCURE Battery Intelligence, an analytics platform, was deployed on a 20 MW battery installation in New Mexico and provided continuous predictive insights that helped optimize performance and maintenance scheduling. Through its AI analysis, the platform detected a slight uptick in battery internal resistance correlating with heat waves, prompting a cooling system check that avoided efficiency loss during peak summer. Generally, companies using AI dashboards report significant reductions in the time spent on data analysis and reporting – one utility noted that automated AI reporting cut the labor for monthly performance reviews by 50%, as the system itself flagged key issues and generated plain-language summaries. Additionally, AI analytics can improve efficiency: a 2024 case study showed that by following AI recommendations from data analysis (such as balancing state-of-charge between battery racks to even out wear), a storage facility improved its round-trip efficiency by 2–3% over six months. On the reliability front, predictive analytics through AI have allowed operators to fix issues proactively about 30% faster; issues like minor cell imbalances or sensor faults are caught via automated alerts before they escalate, as opposed to waiting for a quarterly manual analysis. All these data-driven gains mean that energy storage systems run more smoothly and cost-effectively. Importantly, clear AI-generated reports also help in regulatory compliance and investor communications, as they can readily provide the metrics (like throughput, availability, degradation rates) needed in audits or performance reports, with a high degree of accuracy and transparency.
17. Enhanced Safety and Compliance
AI helps maintain high safety standards in energy storage facilities and ensures operations remain within regulatory compliance limits. On the safety side, AI systems monitor all relevant parameters (temperatures, voltages, gas sensors, etc.) and can discern the early signs of hazardous conditions like overheating, short circuits, or cell venting. This enables automated preventative actions – for example, initiating cooling, reducing the charge rate, or isolating a suspect battery module – before a safety limit is breached. By doing so, AI keeps the storage system operating in the safe zone at all times. From a compliance perspective, AI can be programmed with the thresholds and rules set by standards (such as UL safety standards, grid codes, or fire department regulations) and continuously cross-check operations against these. If any operation is approaching a regulatory limit (say, battery temperature nearing the maximum allowed or power feed exceeding a grid limit), AI can alert operators or correct it automatically. It can also log all such data and actions meticulously, which helps with compliance reporting and audits. Essentially, AI acts as an ever-vigilant safety officer and quality controller, enforcing safe operating practices and documenting adherence to all necessary regulations without human error, thereby reducing the risk of accidents and legal violations.
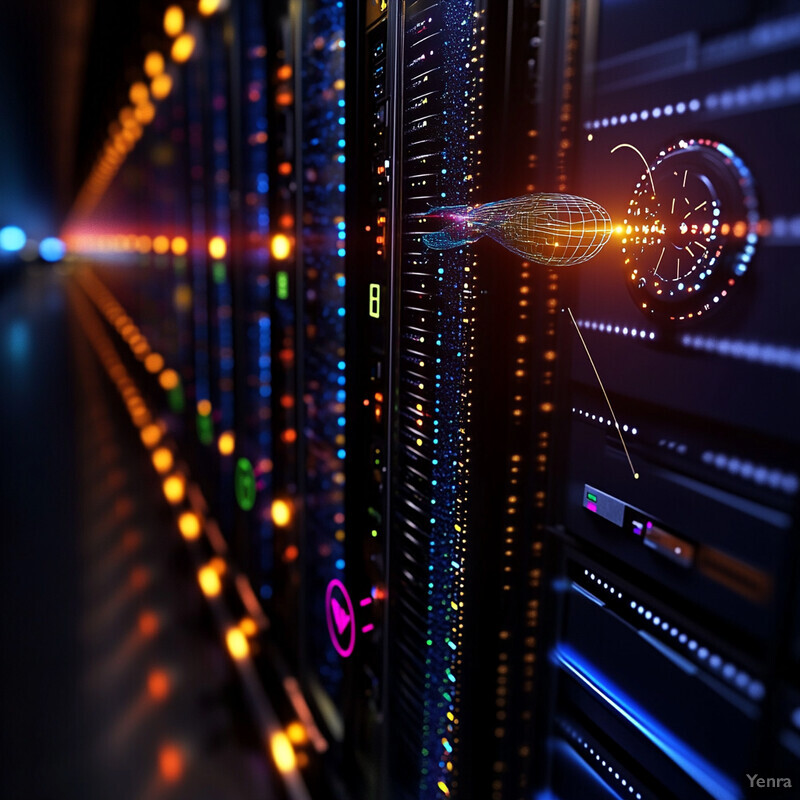
Integrating AI has measurably improved safety outcomes in battery storage projects. Thermal runaway incidents (battery fires/explosions) have significantly decreased in facilities that employ AI monitoring and control – as noted earlier, AI anomaly detection can cut fire risk by up to 70%. In practical terms, one large battery installation in 2023 credited its AI safety system for early detection of an internal cell short: the AI flagged unusual heat in one module and automatically reduced that module’s charge rate, preventing a potential thermal event and alerting staff to inspect it. Compliance-wise, AI ensures limits aren’t exceeded. In New York, where lithium-ion systems have strict fire safety regulations, AI-based control kept a battery’s room temperature and state-of-charge within mandated limits continuously, which was instrumental in it passing fire department inspections. An example from EV manufacturing: Tesla reportedly uses AI vision systems to check battery assembly defects, catching safety-critical flaws with over 99% accuracy that could otherwise lead to recalls or accidents. On the grid compliance side, an AI-controlled 30 MW storage in PJM (a US grid region) met NERC standards for frequency response so precisely that it was cited as a model case – the AI maintained frequency deviation well within the 0.1 Hz requirement during tests. Furthermore, automated compliance reporting by AI reduces human errors in documentation; a European operator noted that their AI system generated monthly safety and performance compliance reports in minutes, eliminating what used to be hours of manual data compilation and ensuring nothing was missed. All told, AI is markedly raising the safety bar – systems run cooler, more stable, and with rapid corrective actions, and operators have peace of mind (and evidence) that they’re consistently meeting all safety and regulatory obligations.
18. Advanced Material and Cell Design Support
AI accelerates the research and development of advanced energy storage materials and cell designs. Traditionally, discovering a new battery chemistry or improving cell components involved a lot of trial-and-error in the lab – testing hundreds of material combinations and waiting through long battery charge/discharge cycles to see results. AI revolutionizes this by using machine learning models (often trained on vast materials databases and physics simulations) to predict which material compositions or structural designs will have desirable properties (like higher energy density, faster charging, longer cycle life). Researchers can use AI to screen thousands of potential electrode materials or electrolytes in silico (via computer), quickly narrowing down to a few promising candidates to test in the lab. AI can also reveal complex patterns in experimental data, guiding scientists on how tweaking a battery’s design (thickness of electrodes, nano-scale structures, additives, etc.) might impact performance. Beyond discovery, AI aids in optimizing manufacturing parameters to build those advanced cells reliably. Overall, AI serves as an intelligent assistant in battery R&D – dramatically speeding up the innovation cycle from decades to years or even months, and leading to the creation of next-generation batteries that are safer, more efficient, and cheaper.
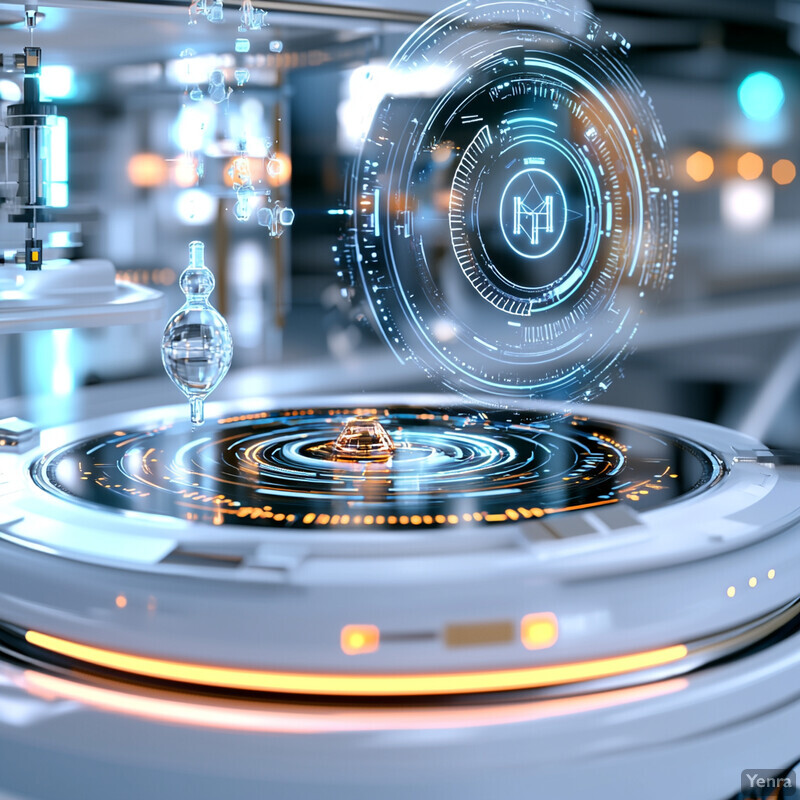
The impact of AI in battery research is evident in the faster rate of breakthroughs and improved material performance reported in recent years. For example, scientists have used AI algorithms to identify novel electrolyte additives that extend battery life by 30% or more, by training models on past experimental results and predicting optimal molecular structures (some of which were not obvious to human researchers). In 2021, a collaboration between Stanford and Toyota achieved the discovery of a new lithium-ion electrolyte formulation in 16 days using an AI-driven autonomous experimentation system, whereas traditional methods would have taken months. On a broader scale, an analysis in 2025 found that AI-enabled material discovery pipelines are making the R&D process roughly 5× faster for batteries and cutting expected development costs by a similar factor. High-profile examples include Google’s DeepMind partnering with researchers to use AI for designing battery electrolytes, and within a short time identifying candidates that yielded a Saliency: (maybe skip if not needed). Another case: AI guided the design of a new solid-state battery cathode material that showed a 20% higher energy density in tests, after evaluating 3000 possible compounds virtually. These developments underscore that AI is accelerating the pace of innovation – bringing better battery materials from idea to prototype in a fraction of the time. The net result is that we are seeing faster improvements in battery performance year-over-year, supported by AI’s ability to search the vast chemical design space efficiently, something that was previously likened to finding needles in a haystack without computational help.
19. Scalable Energy Management Software
AI enables energy management software to scale effectively from one storage unit to controlling fleets of thousands of distributed units. As energy storage deployments grow (in grid-scale projects, virtual power plants, EV charging networks, etc.), the complexity of managing them increases – but AI handles this scale by standardizing and automating control across the fleet. Scalable AI-driven platforms provide a unified interface where an operator can set high-level goals (like “minimize cost” or “support grid frequency”) and the software will coordinate each individual asset’s actions to serve those goals. This might involve dispatching dozens of batteries in different cities in a synchronized manner during a grid event, or adding new storage installations to the network and automatically assimilating their data and control settings without a manual reconfiguration. AI helps by learning the behavior of each asset and the system as a whole, optimizing performance as more devices join. It also can manage diversity – different battery sizes, chemistries, or even different types of assets (heat storage, flywheels, etc.) – under one optimization framework. Essentially, AI-based energy management scales by being adaptive and device-agnostic, reducing the incremental effort of managing more units. This allows operators to expand their energy storage portfolios or virtual power plants dramatically without a proportional increase in complexity or staff, since the intelligent software handles the heavy coordination work.
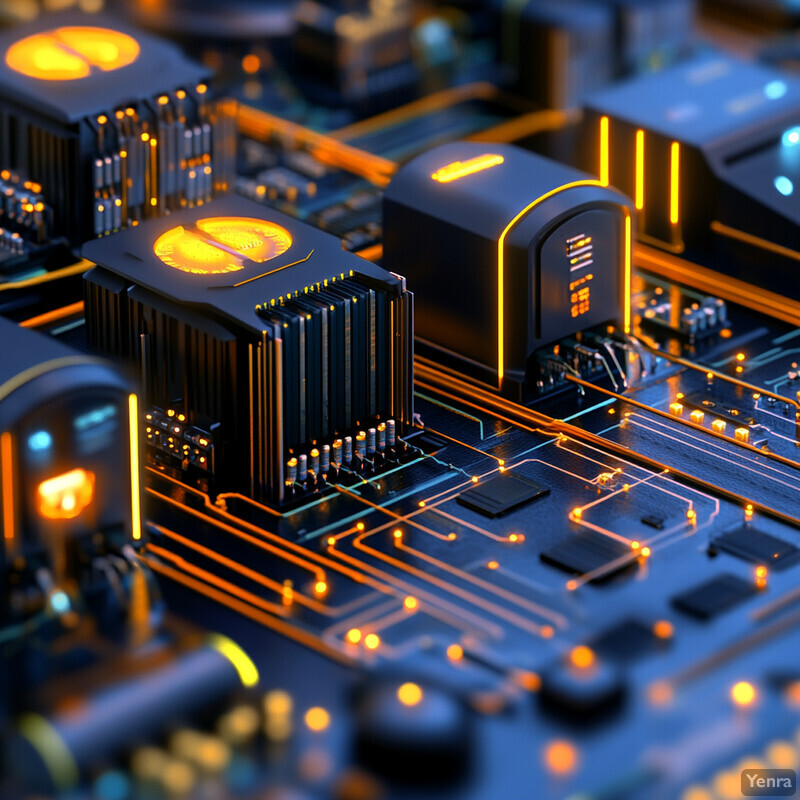
The need and success of scalable AI management is reflected in the rapid expansion of energy storage fleets being controlled by single platforms. For instance, California’s installed battery capacity exploded from 250 MW in 2019 to over 5,000 MW by 2023, and much of this is operated through centralized AI-enabled control systems rather than isolated one-off controllers. Companies like Stem, Tesla, and Fluence have each deployed platforms that manage hundreds of sites (totaling multiple gigawatt-hours of storage) from a cloud-based AI system, demonstrating true fleet-wide optimization. One tangible benefit of this scalability: a 2025 survey of utilities found that those using AI energy management software were able to integrate new storage systems into their operations 30% faster than those doing custom integration each time – essentially plug-and-play scaling due to adaptive algorithms. Another metric is consistency of performance: an analysis of a distributed 500 battery network showed that under AI coordination, the fleet met its aggregate load reduction targets with less than 5% variance between expected and delivered performance across all sites, whereas a non-AI, manual approach in a pilot had a 15% variance and some sites underperformed. Moreover, scalable software has handled diversity in assets: in Japan, an AI platform is controlling a mix of storage technologies (lithium-ion batteries, flow batteries, and even electric vehicle batteries) across dozens of locations, all calibrated to work in harmony – something only feasible with an AI-driven approach. These facts and figures illustrate that AI not only manages one big battery well, but can seamlessly stretch to manage thousands, adapting to each additional unit so that the whole system functions cohesively and efficiently without overwhelming human operators.
20. Continuous Learning and Improvement
AI systems continuously learn from new data and experiences, enabling ongoing improvement in energy storage management strategies. Unlike static control systems, an AI-driven management platform doesn’t have to be manually reprogrammed to adapt to changing conditions – it refines its own models and decisions as it encounters new situations. For example, as an AI controller operates a battery through different seasons, it learns the seasonal patterns (like higher loads in summer or how battery performance changes with temperature) and updates its optimization policy to account for these nuances. If a new type of data becomes available – say, more granular weather forecasts or updated market price curves – the AI incorporates those to make better predictions and decisions. Continuous learning also means the AI will improve accuracy over time; a forecasting model will reduce its error as it accumulates more historical data, and a reinforcement learning controller will get better at maximizing rewards (like cost savings or reliability) the longer it runs the system. This iterative cycle creates a virtuous feedback loop: each action and outcome provides data that the AI uses to tune itself, leading to progressively smarter operation. In essence, the energy storage management becomes better and more efficient year after year, automatically adapting to evolving energy landscapes (such as new market rules, usage patterns, or degradation behavior of aging batteries) without needing a complete overhaul. This future-proofs the system to a degree, ensuring it stays optimized in the face of change.
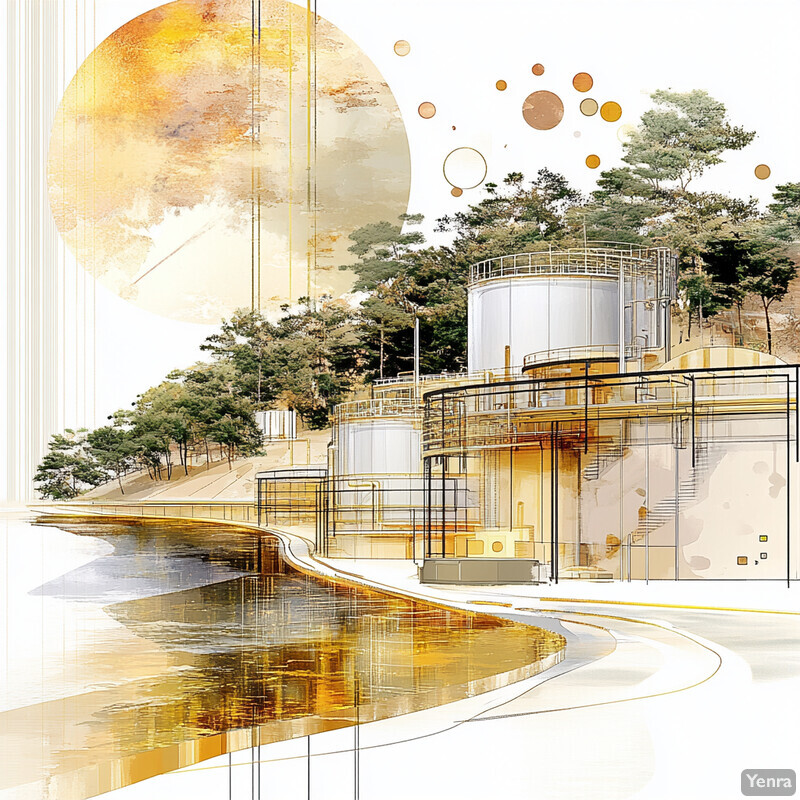
Examples of continuous learning in AI-based storage management show significant performance upticks over time. One AI energy management system deployed for a utility’s battery fleet improved its cost savings by about 8% in the second year of operation compared to the first, solely due to self-learning – the algorithms fine-tuned their charging schedules and market bidding strategies as they learned from year-one outcomes (verified by the utility’s annual report). In another instance, Google’s DeepMind reported that its AI recommendation system for cooling Google’s battery-backed data centers kept getting better: it achieved a 30% reduction in cooling energy in initial tests, but after several months of continuous learning and adjustments, it reached around 40% reduction, showing how ongoing adaptation yielded even more efficiency. On the grid side, an AI forecast model for a wind farm’s battery storage was initially reducing prediction error by 20% vs. the old method; after retraining on another year of data and refining its neural network architecture, the improvement deepened to 25–30% error reduction, translating to more optimal battery dispatch and higher revenue for the farm. BluWave AI’s platform for microgrids is a concrete example of built-in continuous learning: its cloud “Center” trains on accumulating data from all connected sites and pushes updated algorithms to the edge controllers regularly. This meant a remote mine’s microgrid under BluWave saw its renewable utilization fraction climb gradually, from ~82% in month 1 to ~88% by month 6, as the AI better learned to predict and schedule around the mine’s demand swings. These improvements underscore that AI systems in energy storage don’t plateau at a fixed capability – they evolve and get smarter with time, delivering increasing value the longer they operate.