1. Automated Data Extraction and Classification
AI-driven tools can rapidly process vast amounts of financial documents, invoices, and receipts, accurately classifying and extracting relevant tax data. This reduces the reliance on manual data entry, minimizing human error and accelerating overall tax preparation.
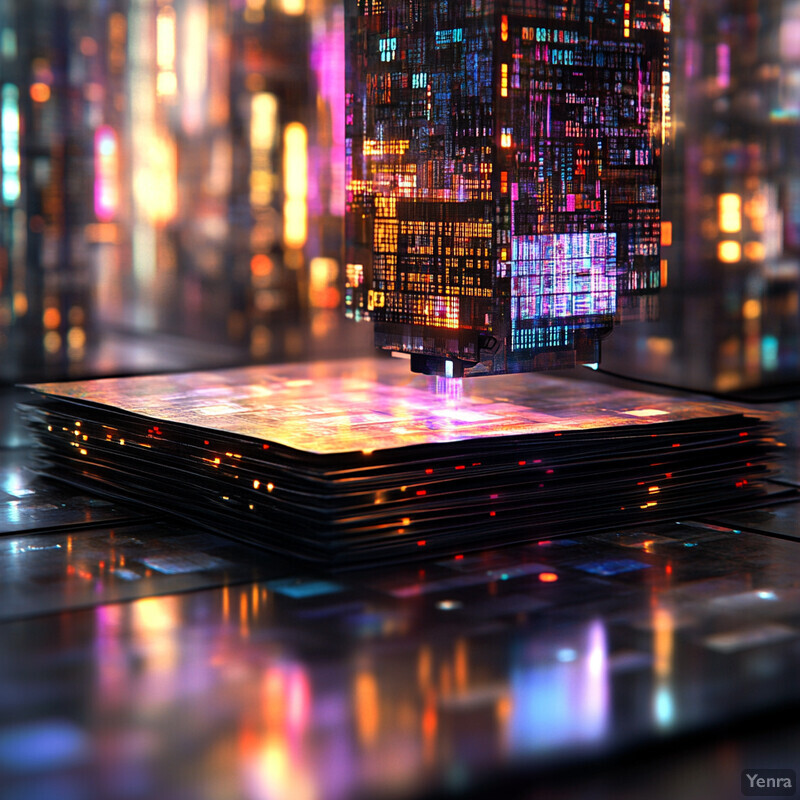
AI-driven systems leverage optical character recognition (OCR), natural language processing (NLP), and machine learning algorithms to quickly analyze a broad range of financial documents—from invoices and receipts to complex ledger entries—pulling out essential tax-relevant data with exceptional accuracy. By automating this classification process, companies reduce reliance on manual data entry, which can be laborious and error-prone. The resulting efficiency frees tax professionals to focus on strategic planning instead of data wrangling, and the consistent application of rules ensures more uniform documentation. Moreover, these systems can self-improve over time as they process more data, honing their accuracy and further streamlining the data extraction workflow.
2. Real-Time Regulatory Tracking
Tax regulations frequently change at both domestic and international levels. Machine learning models can continuously ingest official announcements, legal updates, and guidance to ensure that tax planning strategies are always aligned with the latest rules.
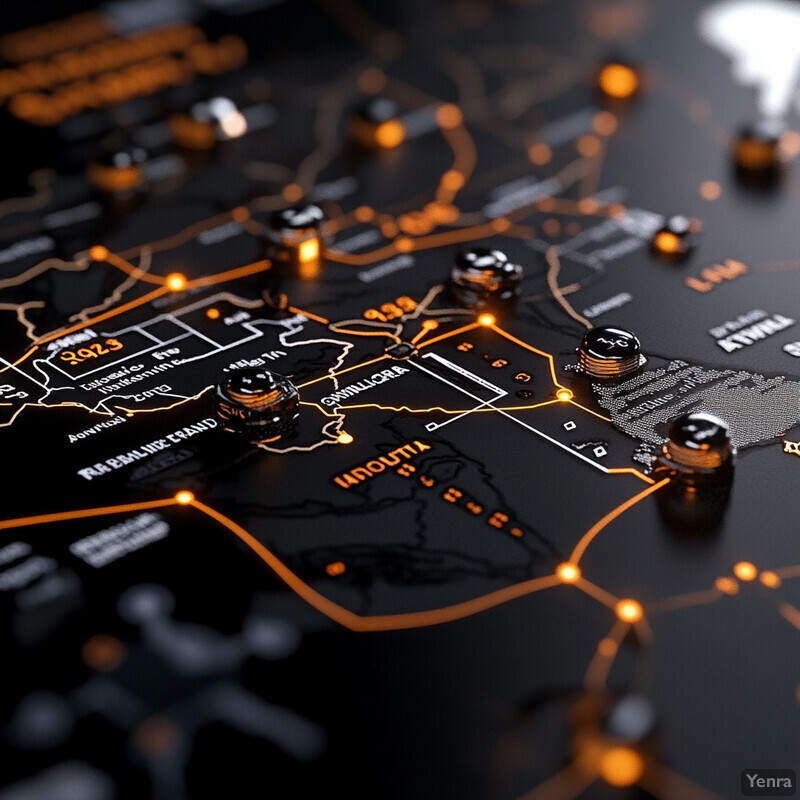
Tax laws and regulations evolve at a dizzying pace, influenced by political decisions, economic conditions, and international treaties. AI models trained to monitor legislative bodies, tax authorities, and reputable financial news sources can continuously ingest new information and flag changes that affect corporate tax obligations. This ensures that tax departments are never behind the curve on compliance requirements. Instead of reacting to changes after they’ve taken effect, companies can proactively adjust their strategies, update internal policies, and reconfigure systems. Such real-time updates help maintain compliance across jurisdictions, reduce exposure to penalties, and enable businesses to take advantage of new incentives or regulations as soon as they emerge.
3. Predictive Analytics for Tax Liability Forecasting
Advanced algorithms can forecast future tax liabilities based on historical data, current transactions, and predictive modeling, enabling companies to anticipate tax burdens and proactively adjust strategies.
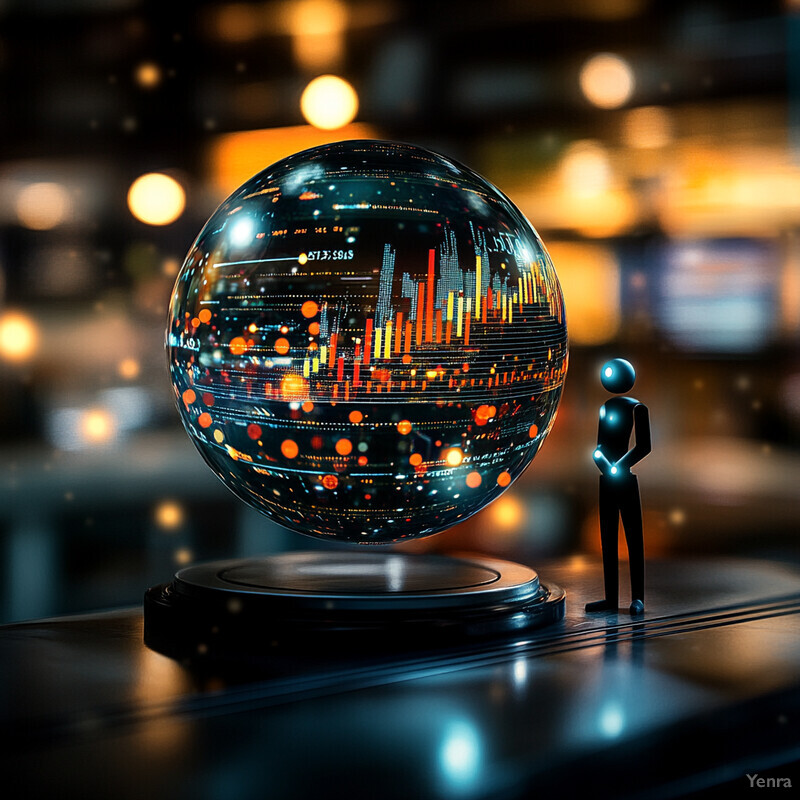
Advanced machine learning techniques can analyze historical financial data, current revenue streams, operational costs, and upcoming business activities to forecast tax liabilities with remarkable precision. By identifying patterns and trends that might not be obvious through manual analysis, AI provides forward-looking insights that allow companies to budget accurately for future tax payments. This level of predictive clarity enables more informed strategic decisions—such as timing expenses or investments—making it easier to manage cash flow, minimize unpleasant year-end surprises, and enhance overall financial stability. Over time, these predictive models become more accurate as they learn from each reporting cycle, continually improving the quality of tax forecasts.
4. Enhanced Transfer Pricing Analysis
Determining appropriate transfer prices for cross-border transactions is complex. AI systems can help analyze comparable data sets, identify anomalies, and ensure that transfer pricing methods are consistent with global standards, reducing audit risk.
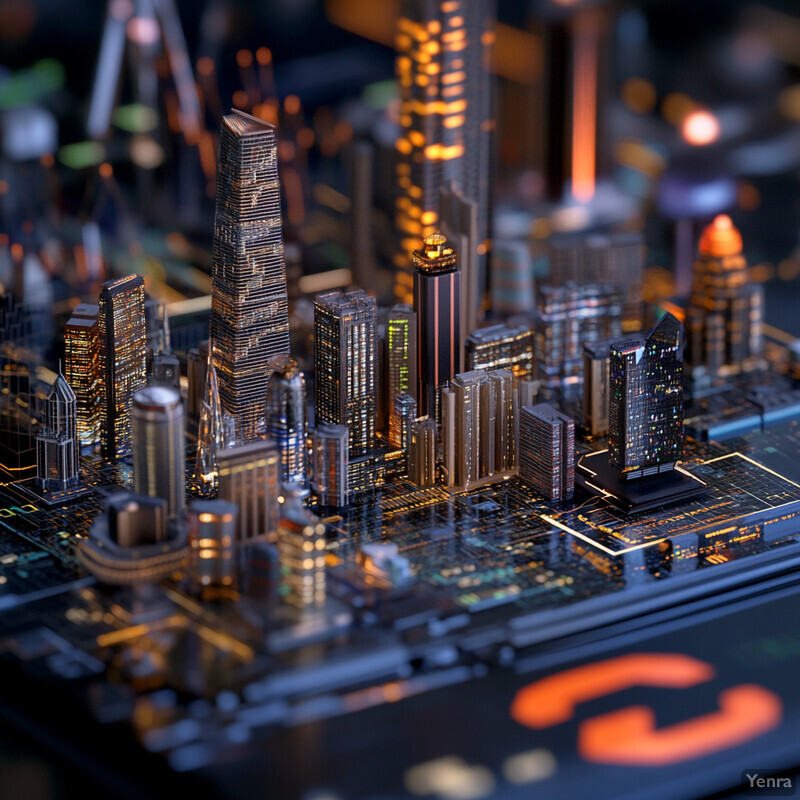
Transfer pricing involves setting prices for transactions between subsidiaries in different countries, a task complicated by varying regulations and evolving global standards. AI tools can sift through vast amounts of comparable uncontrolled price data, economic indicators, and regulatory documents to propose objective pricing that aligns with international guidelines. These systems can identify anomalies, spot outliers, and highlight discrepancies that might draw scrutiny from tax authorities. By providing a reasoned and data-driven basis for transfer pricing, AI reduces the likelihood of costly audits, helps avoid double taxation, and ensures that multinational corporations optimize their tax positioning while maintaining compliance and defending their pricing decisions with confidence.
5. Sophisticated Tax Scenarios and What-If Modeling
AI-driven simulation tools allow tax professionals to model various scenarios—changes in corporate structure, new geographic markets, shifts in supply chains—and see their potential tax implications before making critical decisions.
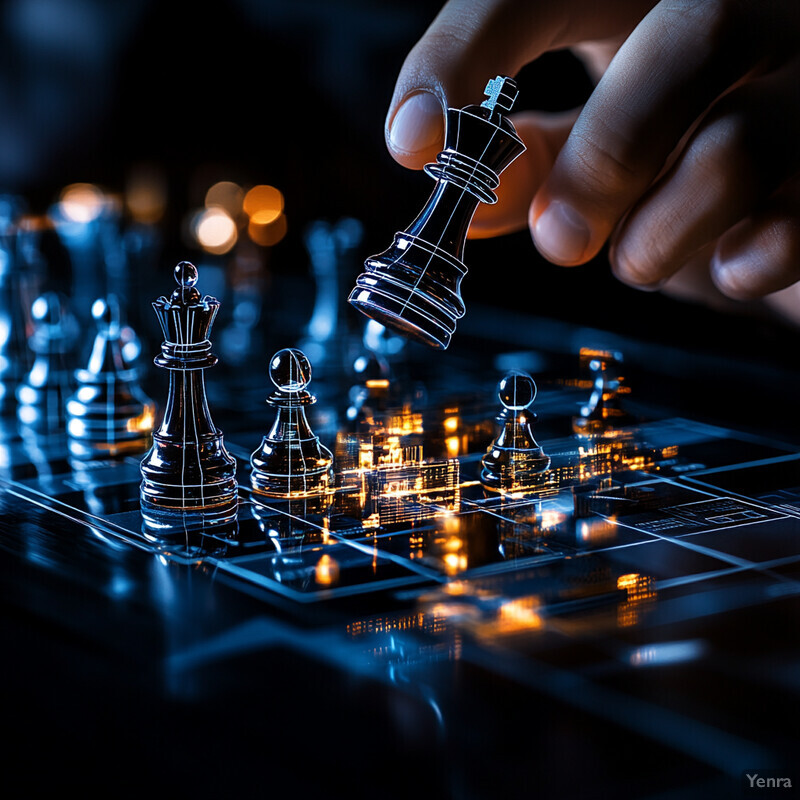
Corporate tax planning often involves navigating complex trade-offs and anticipating the tax impact of various strategic moves. AI-driven scenario modeling tools enable tax professionals to simulate a wide array of business decisions—from entering new markets and altering supply chain structures to adjusting capital investments and organizational form. By analyzing each scenario’s tax implications in detail and presenting outcomes under different regulatory environments, these systems empower decision-makers to optimize strategies before implementing them in the real world. As a result, businesses can minimize tax exposure, capitalize on opportunities, and ensure readiness for whatever external changes may arise.
6. Dynamic Tax Compliance Monitoring
Automated checks powered by AI can continuously monitor transactions and accounting systems to identify areas of non-compliance, underreporting, or missed deductions, providing alerts in real-time.
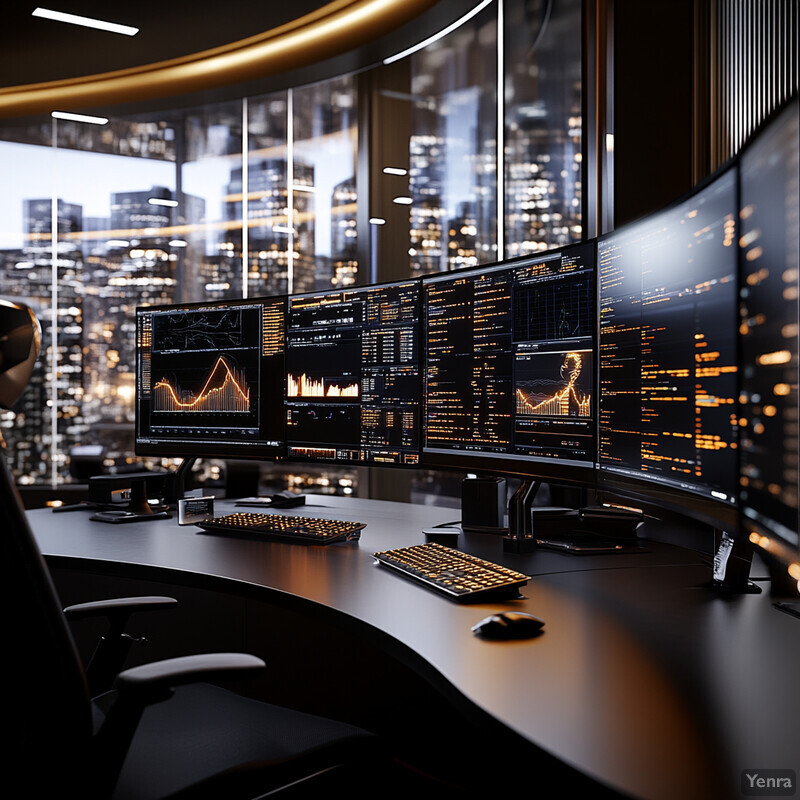
Instead of waiting until the end of a reporting period to catch tax compliance issues, AI-powered monitoring solutions run continuously in the background. These systems interface directly with accounting software, ERP systems, and other financial tools to ensure that every recorded transaction aligns with relevant tax laws. By instantly flagging potential discrepancies—such as missed deductions, inconsistent data entries, or out-of-bound expenses—AI alerts tax professionals to problems while they can still be corrected. This proactive approach mitigates the risk of penalties or interest charges, supports more accurate reporting, and fosters a culture of ongoing compliance rather than frantic year-end reconciliations.
7. Natural Language Processing for Tax Code Interpretation
NLP models can interpret and summarize complex and voluminous tax codes, regulations, and rulings, making it easier for corporate tax teams to understand obligations and plan intelligently without having to wade through dense legal texts.
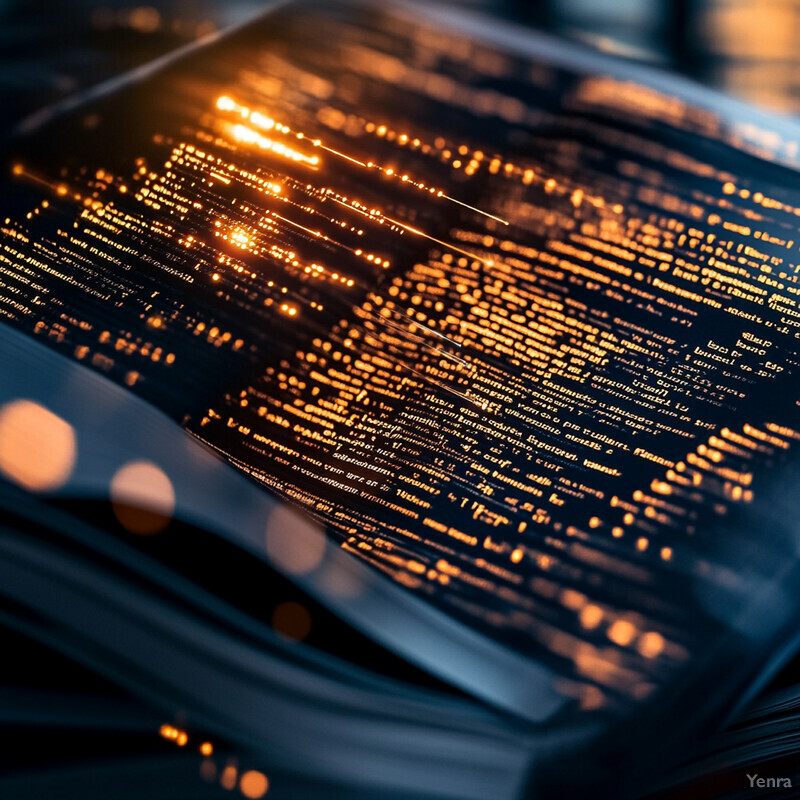
Tax codes, regulations, and official guidance are typically written in dense legal language that can be difficult to parse. NLP-powered AI models help distill this complexity by reading, interpreting, and summarizing these documents, making intricate legal rules more accessible to corporate tax teams. These tools can also highlight relevant clauses, cross-reference related guidelines, and offer explanations in plain language. By doing so, they save time and reduce the risk of misunderstanding critical provisions. Ultimately, NLP capabilities help tax departments remain well-informed, confident, and agile in applying the law to their business operations.
8. Risk Scoring and Audit Readiness
AI can assign risk scores to various tax positions and identify areas most likely to draw regulatory scrutiny. Companies can then shore up documentation and ready themselves for potential audits, reducing the likelihood of costly surprises.
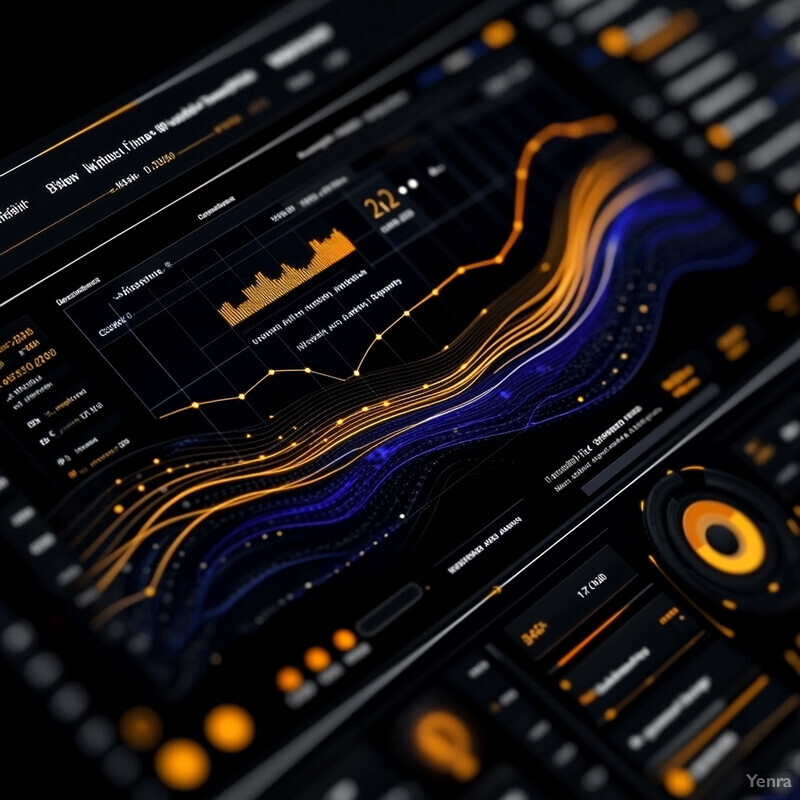
AI engines can assess the overall risk profile of a corporation’s tax positions by analyzing financial histories, transaction patterns, and known red flags that attract regulatory attention. They assign risk scores to various areas—such as transfer pricing, R&D credits, or depreciation strategies—indicating where the company may face heightened scrutiny. Armed with this insight, tax professionals can strengthen documentation, refine their arguments, or consider alternative approaches to reduce exposure. By doing so, they not only lower the likelihood of audits but also ensure that if an audit does occur, the company is well-prepared to defend its positions swiftly and successfully.
9. Automated Tax Return Preparation
Machine learning algorithms can assemble and prepare corporate tax returns by accurately mapping data from ERP systems to the correct forms and schedules, leaving tax professionals to focus on oversight and strategic planning.
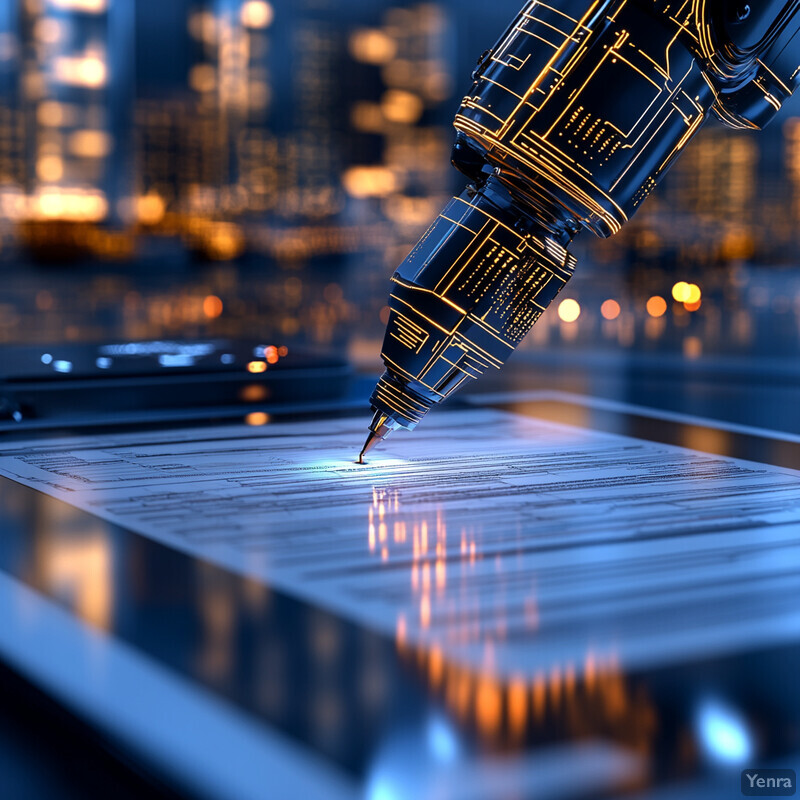
Preparing corporate tax returns can be an exhausting and detail-intensive process prone to human error. AI-driven solutions connect to internal financial databases and systems, mapping each data point to the correct field on the relevant tax forms. The technology checks calculations, ensures that all required attachments are included, and even suggests where additional documentation might strengthen a claim. As a result, tax professionals spend less time on mechanical tasks and can devote more attention to strategic analysis and review. The streamlined accuracy reduces the risk of costly filing mistakes, accelerates the filing process, and leads to more reliable and defensible returns.
10. Hyper-Personalized Tax Optimization Strategies
Through pattern recognition, AI can tailor tax strategies to the unique characteristics and needs of individual business units or subsidiaries, ensuring optimal deductions, credits, and incentives are utilized.
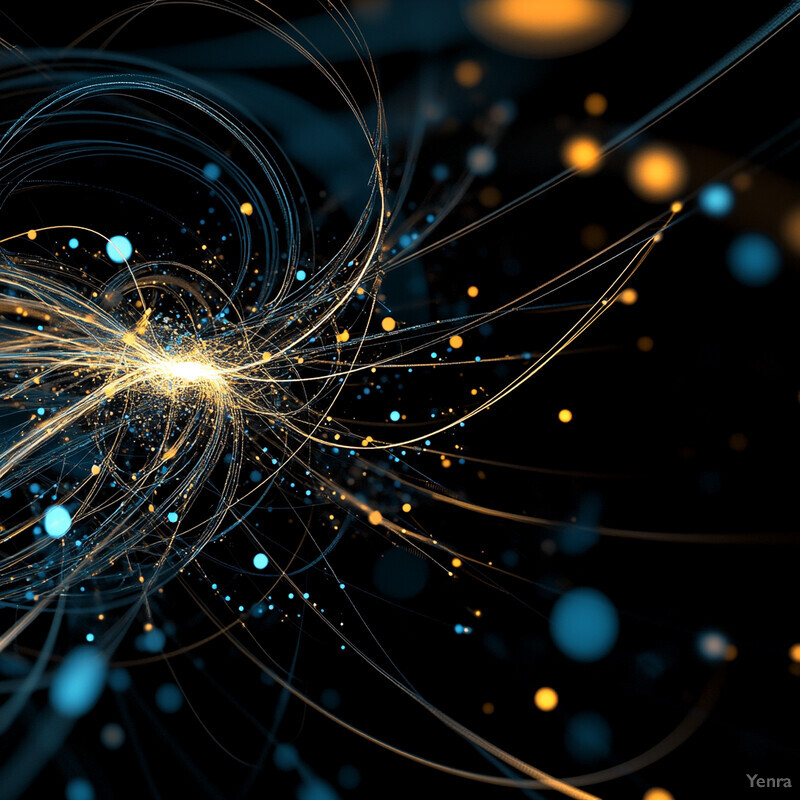
A multinational enterprise can encompass various subsidiaries, divisions, or product lines, each with unique financial dynamics and regulatory environments. AI-driven segmentation allows companies to move beyond one-size-fits-all tax strategies. By analyzing each unit’s financials, market conditions, and jurisdictional rules, AI tools generate tailored recommendations that optimize credits, deductions, and incentive programs at a granular level. This personalization ensures that tax planning aligns with local nuances and operational realities, ultimately reducing the global tax burden and freeing resources for investment and growth.
11. Identification of Tax Incentives and Credits
AI systems can scan legislation, industry reports, and government programs globally to pinpoint relevant incentives, credits, and rebates that a company may qualify for—often uncovering opportunities that might be missed by manual methods.
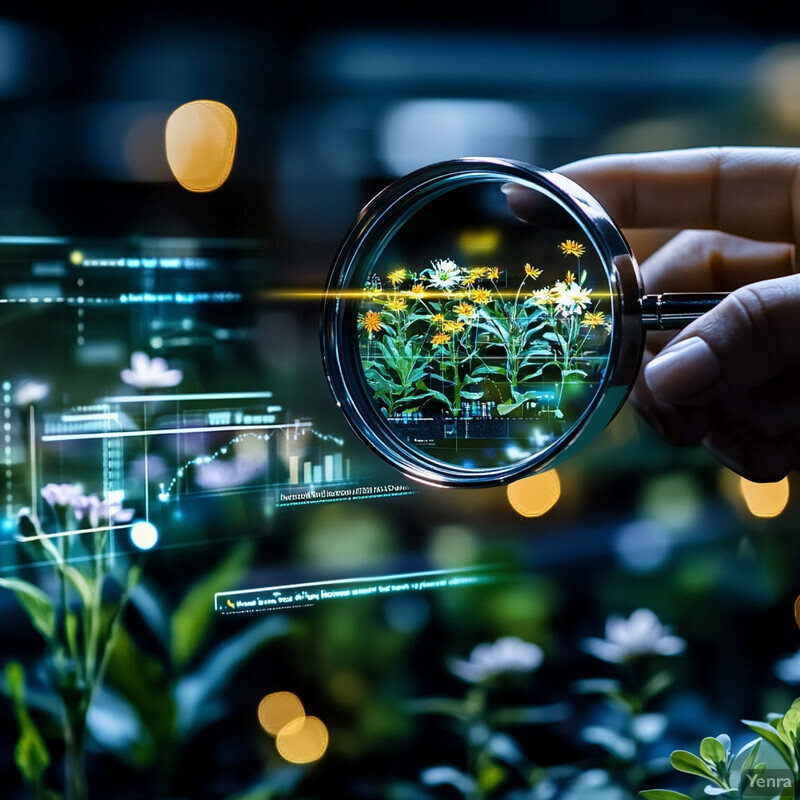
Keeping track of all possible tax incentives—from R&D credits to green energy rebates—across multiple jurisdictions can overwhelm even large tax departments. AI systems equipped with web scraping, NLP, and database integration capabilities can comb through legislation, regulatory updates, and government websites to pinpoint relevant incentives that a company can claim. By mapping these opportunities to the company’s activities and expenses, AI provides a ready list of beneficial programs. This proactive identification helps businesses maximize available credits, reduce effective tax rates, and gain a competitive edge without missing valuable incentives hidden in fine print.
12. Improved Interdepartmental Integration
AI-driven tax solutions can integrate seamlessly with a company’s accounting, finance, and procurement systems, ensuring tax considerations are embedded in operational workflows and making tax planning a continuous process rather than an end-of-year scramble.
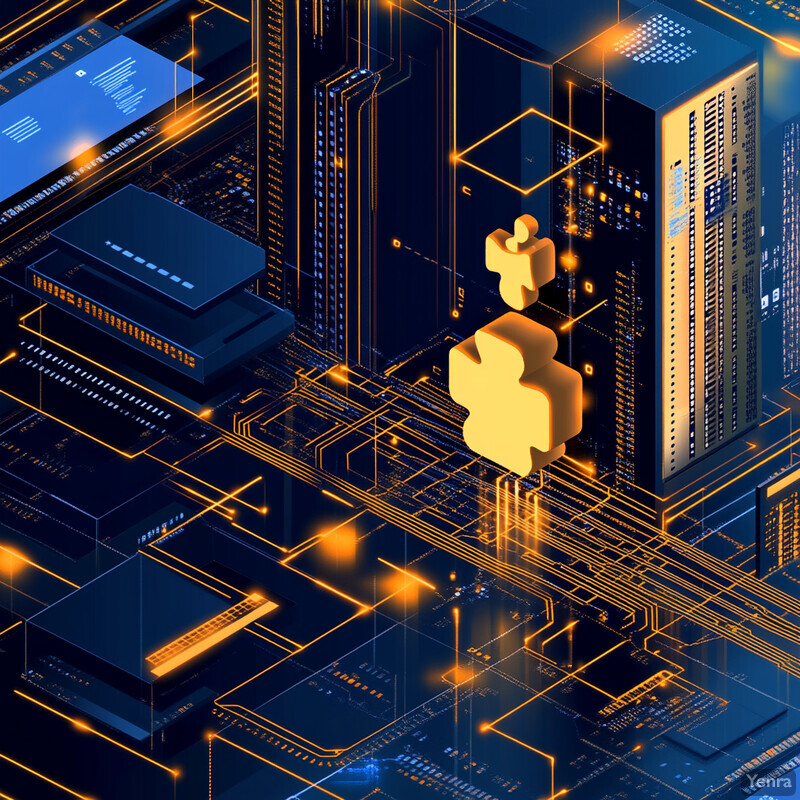
Tax planning does not exist in a silo; it intersects with accounting, procurement, finance, and supply chain decisions. AI-powered integration solutions ensure that tax considerations are woven into everyday operations. For instance, changes in supplier pricing, adjustments to inventory levels, or new capital expenditures are instantly analyzed through a tax lens. By automating data flow and ensuring that all departments operate with a tax-aware perspective, businesses can prevent last-minute scrambling, improve internal communication, and better align corporate strategies with holistic tax objectives.
13. Enhanced Data Integrity and Validation
By cross-referencing multiple data sources, machine learning can detect discrepancies and validate the consistency of tax-related information, reducing the chance of misstatements and erroneous filings.
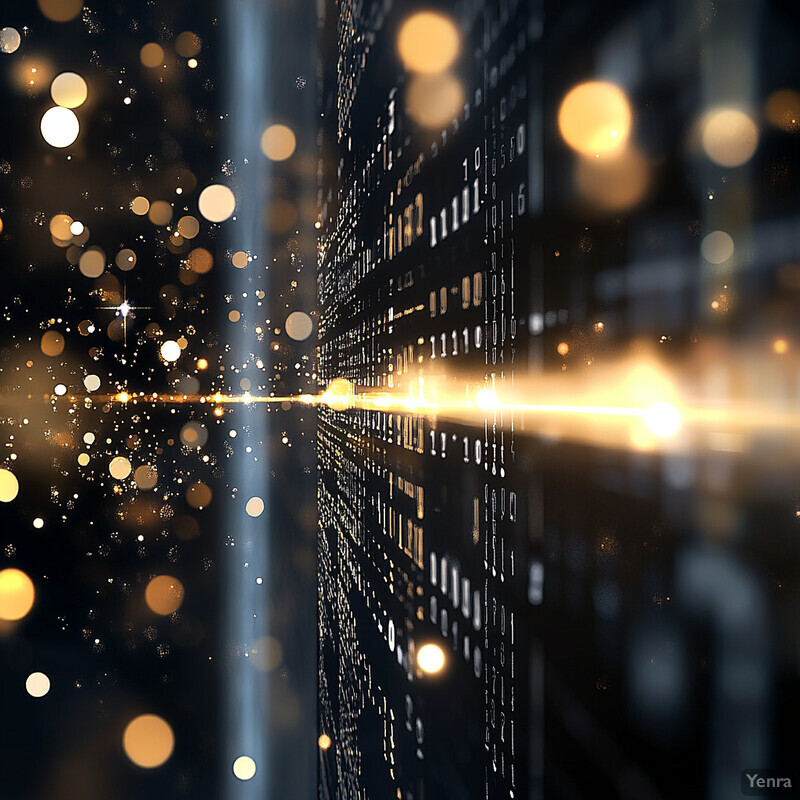
Inconsistent or inaccurate data can lead to flawed tax planning, filing errors, and compliance breaches. AI-driven validation systems cross-check financial information against multiple sources, looking for inconsistencies like mismatched supplier names, irregular expense claims, or revenue figures that deviate from historical patterns. By catching these issues early, companies can correct errors before they balloon into larger problems. A strong foundation of accurate, reliable data underpins every facet of tax planning, enabling more confident decision-making and reducing the risk of fines, audits, and reputational damage.
14. Continuous Learning from Outcomes
As AI models receive feedback from audits, regulatory reviews, and finalized returns, they continuously improve. Over time, these systems become more adept at identifying subtle patterns and implementing more effective tax planning strategies.
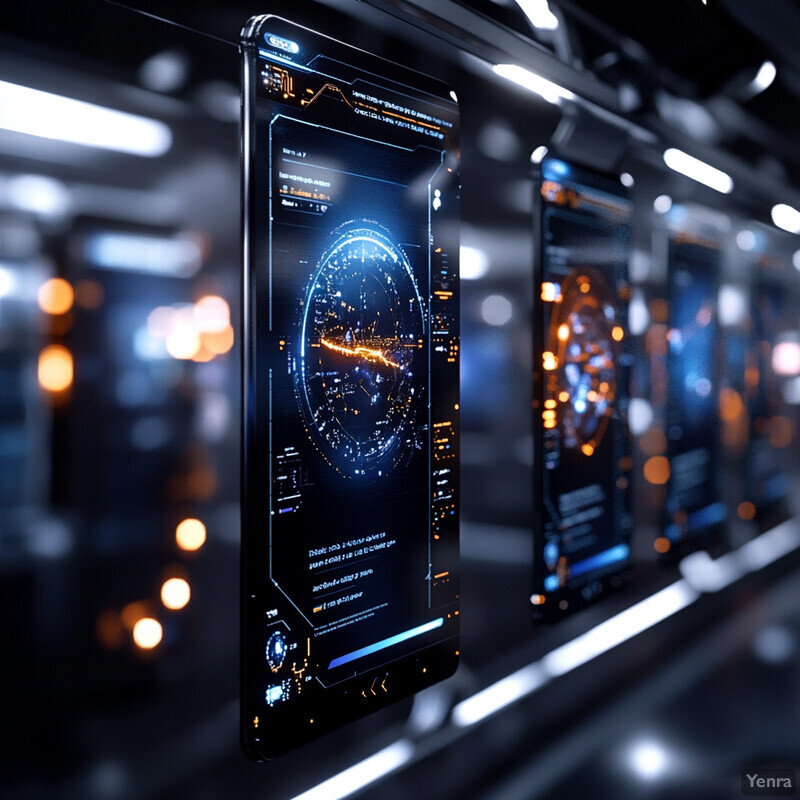
AI thrives on feedback loops. Over time, these systems learn from each round of filings, audits, and regulatory reviews. They identify which tax strategies survived scrutiny, which data sources proved most reliable, and which regulatory interpretations were upheld. As the model refines its understanding, it becomes better equipped to recommend strategies that stand the test of regulatory oversight. This continuous improvement cycle helps companies stay ahead of evolving tax environments, ensuring that their tax planning is always informed by the most recent successes and failures, both internally and across the broader corporate landscape.
15. Intelligent Benchmarking
AI-driven benchmarking tools can compare a corporation’s tax positions against industry peers, identifying where the company stands competitively and suggesting strategic adjustments to better align with best practices.
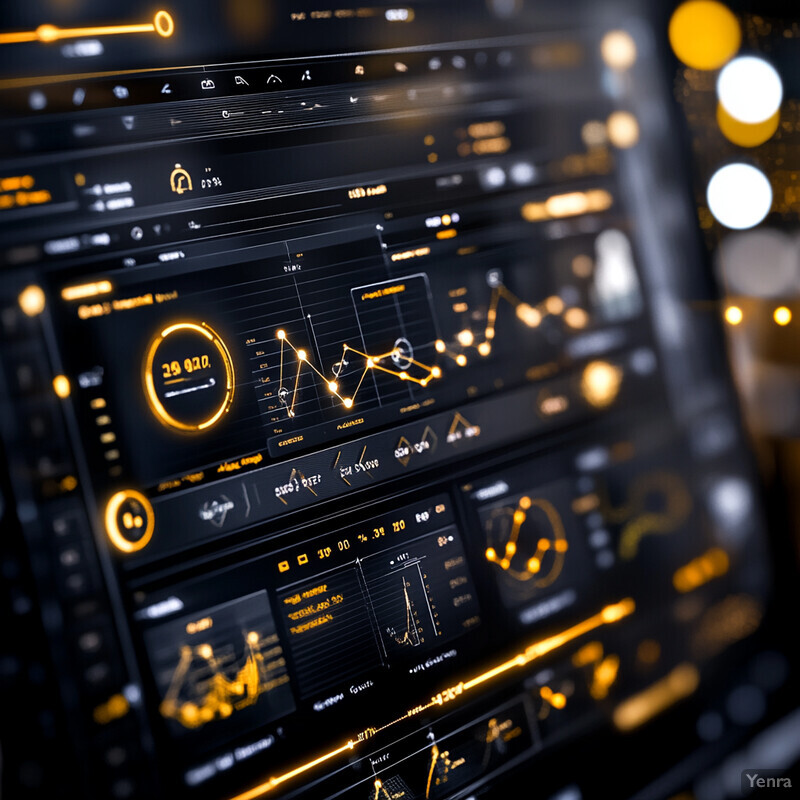
Understanding how a company’s tax positions compare to those of industry peers can provide critical insights into competitiveness and regulatory risk. AI tools can aggregate data from public filings, industry reports, and anonymized databases to benchmark a company’s effective tax rate, credit utilization, and compliance posture. By illuminating where the company stands relative to its competitors, AI allows managers to identify areas for improvement. Perhaps there is a particular tax credit that industry leaders leverage more effectively, or a compliance practice that is now an industry norm. Benchmarking thus informs strategic adjustments that help the company remain at the forefront of best practices.
16. Accelerated Cross-Border Compliance
For multinational corporations, AI can handle complex, multi-jurisdictional tax laws and detect conflicts, ensuring global compliance and recommending country-specific optimizations while maintaining a cohesive global tax strategy.
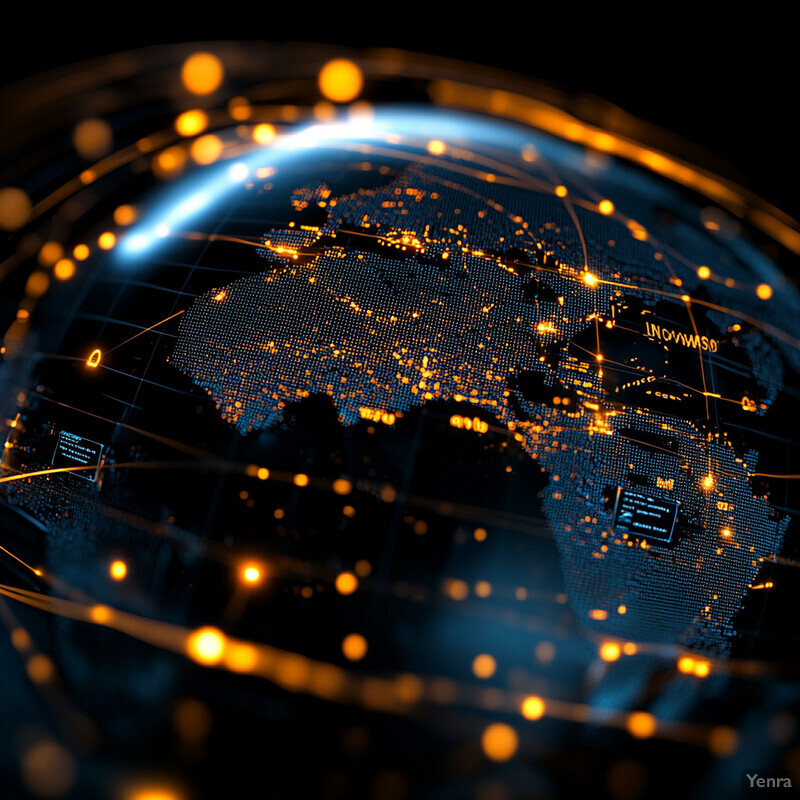
Operating across multiple countries entails grappling with different tax laws, filing deadlines, documentation requirements, and risk profiles. AI-driven platforms can centralize these disparate regulations, providing a unified view of multinational tax obligations. They can automate complex calculations for VAT, GST, withholding taxes, or custom duties, ensuring that nothing slips through the cracks. With real-time updates, the system quickly adapts to new treaties, rulings, and rates. This acceleration not only prevents compliance lapses but also helps CFOs and tax directors shape a cohesive global tax strategy that balances local optimization with global coherence.
17. Real-Time Transfer of Knowledge
Corporate tax teams often rely on institutional knowledge. AI-based knowledge management systems ensure that insights, past analyses, and best practices remain accessible as team members change roles, retire, or leave the organization.
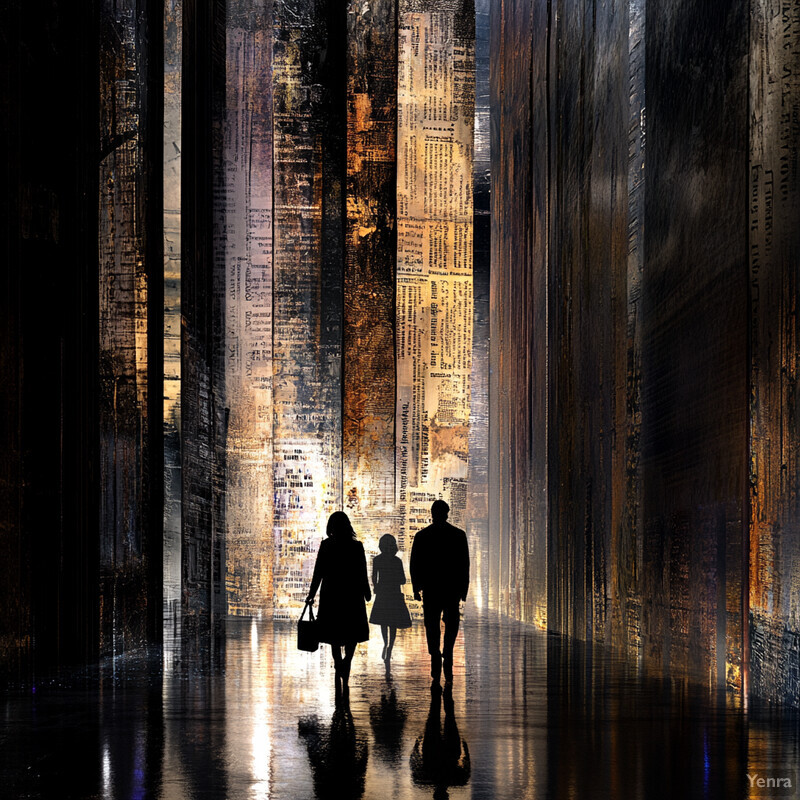
Corporate tax departments often rely on the expertise of seasoned professionals who have accumulated years of institutional knowledge. When team members retire or move on, organizations risk losing valuable insights. AI-based knowledge management systems capture and codify best practices, historical decisions, and regulatory interpretations. These systems then serve as a repository that new team members can tap into, reducing onboarding times and ensuring continuity of expertise. By preserving and disseminating institutional memory, companies build resilience into their tax planning capabilities and avoid reinventing the wheel with each personnel change.
18. Smart Collaboration Tools
With AI-enhanced platforms, tax professionals can collaborate more effectively across departments and geographies. These systems can manage version control, highlight key issues, and propose solutions based on similar past cases.
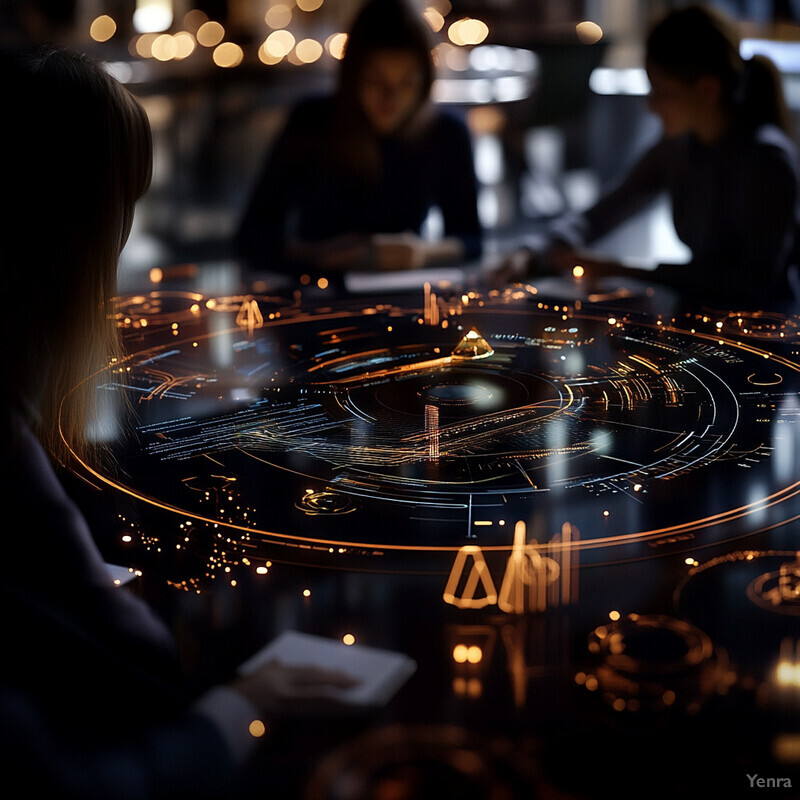
Today’s tax functions are often dispersed across various regions and time zones. AI-enhanced collaboration platforms streamline communication, version control, and workflow management. They can suggest whom to involve in a particular discussion, highlight sections of documents needing immediate attention, and track revisions to ensure that everyone works from the most current data. By maintaining centralized, intelligent oversight over projects, these tools help prevent miscommunication, duplication of efforts, and last-minute chaos. The result is a more cohesive, productive, and responsive tax team that can effectively handle global complexity.
19. Scenario Planning for Mergers and Acquisitions
AI can rapidly assess the tax implications of potential mergers, acquisitions, or divestitures, suggesting optimal deal structures and providing guidance on post-integration tax strategies to maximize tax efficiencies.
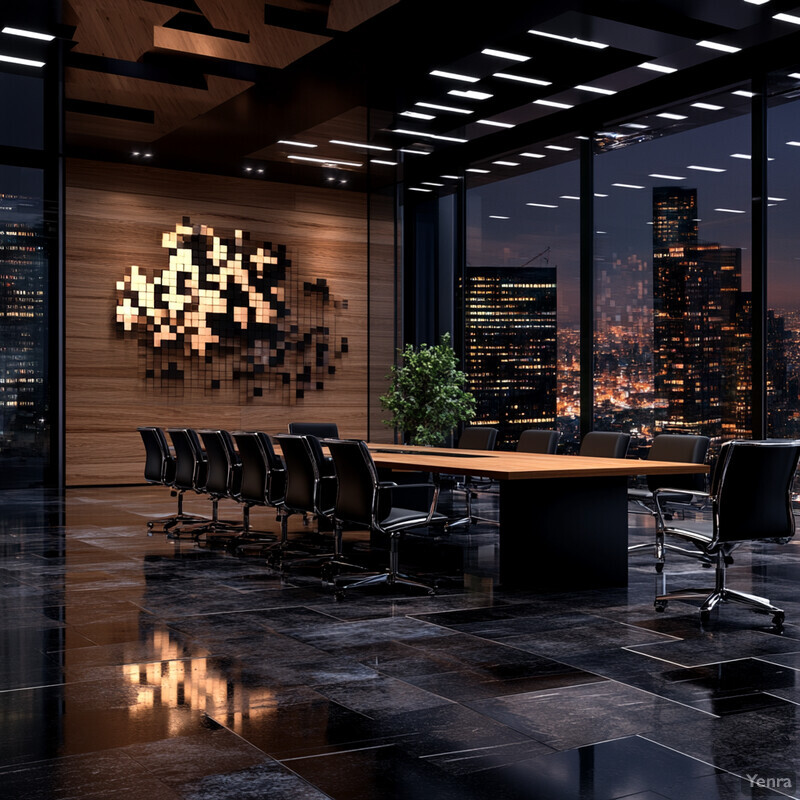
M&As can dramatically alter a company’s tax profile, introducing new jurisdictions, asset structures, and market considerations. AI-based scenario analysis tools can rapidly evaluate the tax consequences of proposed deals, testing various transaction structures—such as asset deals vs. stock deals—and mapping out subsequent steps for post-integration optimization. This forward-looking insight ensures that tax considerations are integrated early in the M&A process, preventing costly oversights that might emerge during diligence or after closing. In addition, by providing a thorough understanding of tax implications, AI allows decision-makers to choose the deal structure that best supports the company’s long-term financial goals.
20. Continuous Regulatory Liaison and Lobbying Insights
By monitoring legislative developments, policy proposals, and stakeholder input, AI can guide corporate tax teams on potential future changes. This foresight can inform strategic engagement with policymakers or preparation for upcoming reforms, providing a competitive edge.
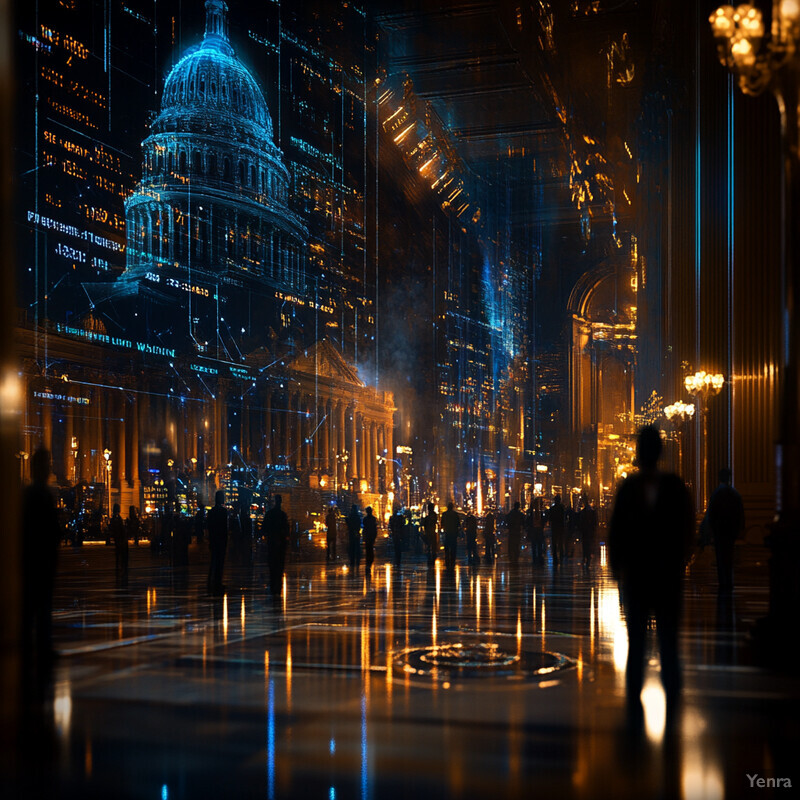
Anticipating future tax trends and regulatory shifts is essential for long-term strategic planning. AI-driven monitoring tools keep track of legislative proposals, think-tank reports, and stakeholder submissions to regulatory bodies. By identifying emerging patterns—such as momentum toward certain tax reforms or increased scrutiny of specific industries—these systems give corporate tax teams an early warning. Equipped with this foresight, companies can adjust their policies, consider different lobbying strategies, or prepare compliance frameworks for new rules likely to come into effect. Ultimately, this strategic engagement with policymakers and timely adaptation helps safeguard the company’s interests and ensures that its tax planning remains forward-thinking and resilient.