1. Automated Defect Detection via Machine Vision
AI-driven image analysis systems can identify porosity, cracks, and other welding defects in real-time, reducing reliance on human inspectors.
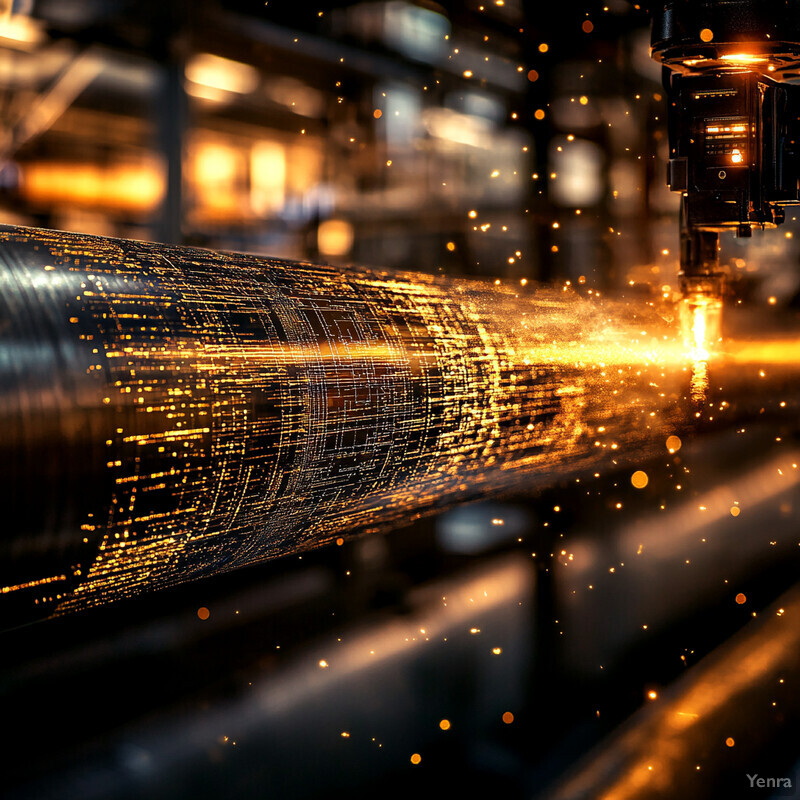
In traditional welding quality assurance, inspectors rely heavily on visual checks and manual measurements to identify defects, making the process slow, subjective, and prone to human error. With AI-powered machine vision systems, high-resolution cameras and advanced image-processing algorithms work together to detect a wide range of weld imperfections—such as cracks, porosity, undercuts, and inclusions—almost instantaneously. The AI compares each weld against established quality standards, highlighting anomalies in real-time. Because this technology can operate continuously and at high speeds, it significantly increases both the accuracy and efficiency of quality control procedures, leading to fewer defects passing through unnoticed and reduced scrap rates over time.
2. Real-Time Weld Parameter Optimization
AI models can continuously adjust welding parameters—such as voltage, current, and travel speed—to maintain optimal welding conditions, improving overall weld quality.
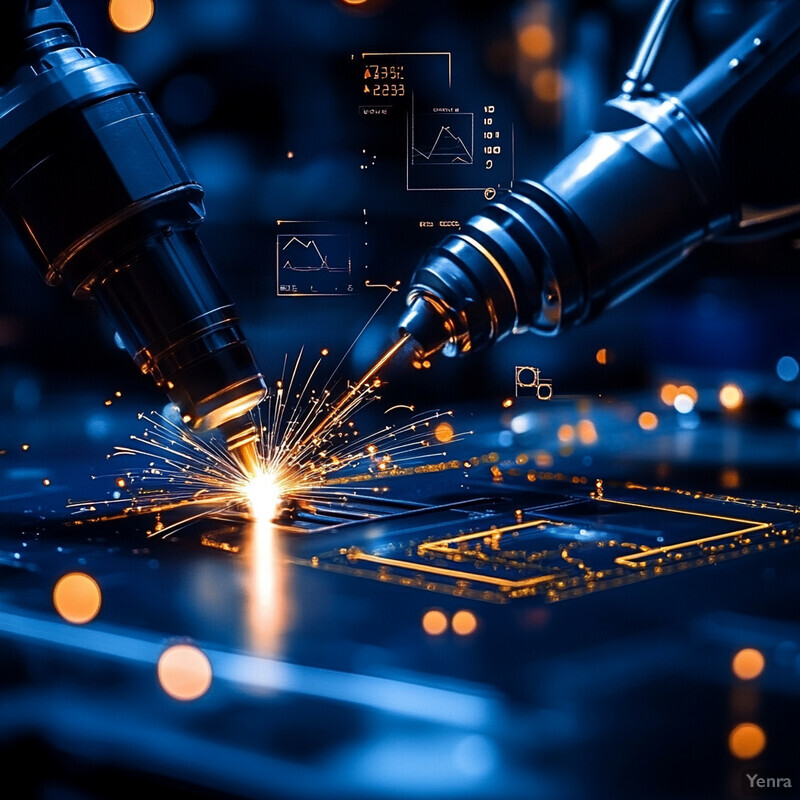
Welding processes involve a complex interplay of variables—like current, voltage, travel speed, and gas flow—that must be perfectly balanced to achieve a defect-free weld. AI-driven control systems monitor sensor data from the welding machine and the weld pool, using machine learning algorithms to recognize patterns that lead to higher-quality welds. As conditions change, the AI instantly adjusts parameters, ensuring the process remains stable and produces consistent weld quality. By automating these parameter tweaks, manufacturers reduce reliance on skilled technicians for fine-tuning, shorten production times, and improve first-pass yields.
3. Predictive Quality Assessment
By analyzing historical data and current sensor readings, AI can predict when welds are likely to fail quality checks, allowing operators to proactively adjust processes.
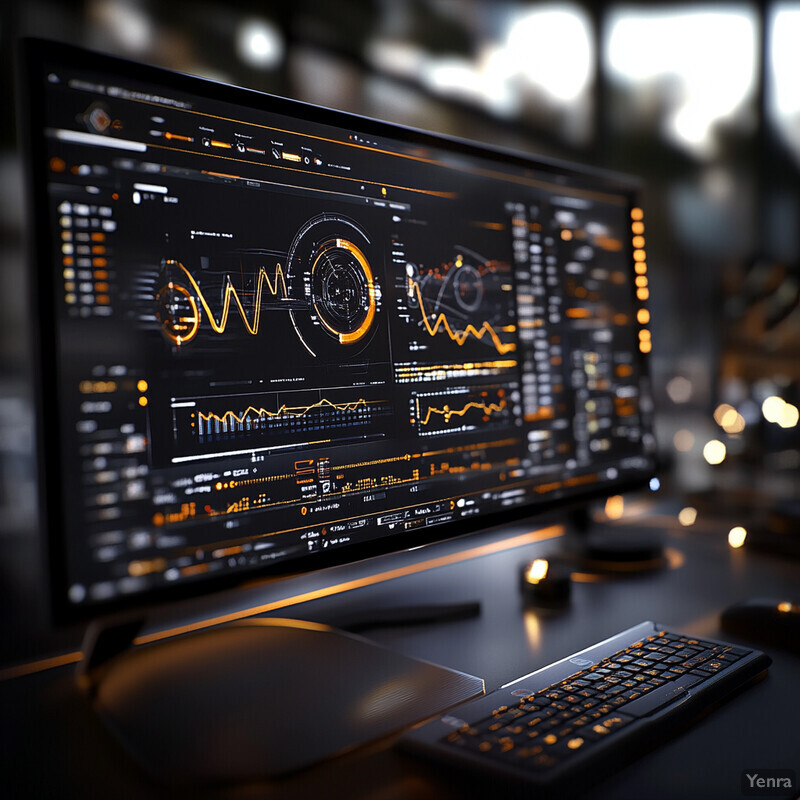
Rather than waiting until a weld is completed to determine its quality, predictive models leverage historical production data, sensor inputs, and environmental information to forecast the likelihood of defects. By running advanced analytics in the background, the AI can anticipate when a weld might fail quality standards—perhaps due to material inconsistencies, worn equipment, or unstable thermal conditions. This proactive approach allows operators to intervene before a problem occurs, adjusting parameters, changing consumables, or performing maintenance. As a result, predictive quality assessment reduces rework, minimizes downtime, and ensures more consistent output across production cycles.
4. Seam Tracking and Alignment
AI-enabled vision systems can accurately locate and track the weld seam, guiding welding robots to maintain proper alignment for consistent quality.
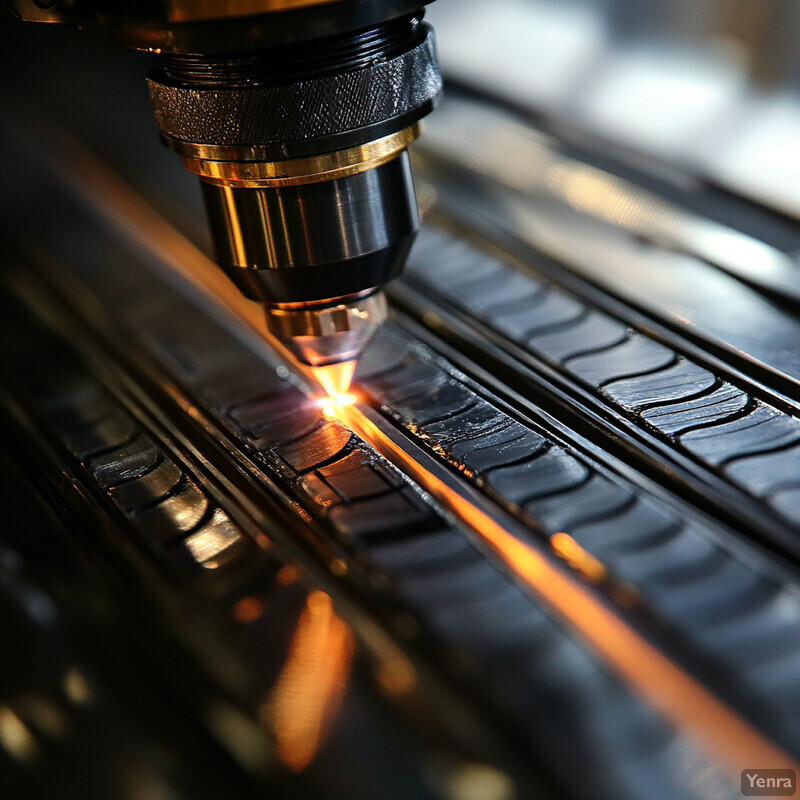
Maintaining proper alignment between a welding torch and the joint seam is critical for achieving uniform weld quality. AI-enhanced seam tracking systems rely on cameras, sensors, and predictive algorithms to identify the exact location, shape, and orientation of the seam, even if it’s irregular or partially obscured. The system continuously feeds this data to robotic welders, guiding their position and movement. This level of precision reduces human error, diminishes the risk of incomplete fusion or misalignment defects, and improves repeatability. Ultimately, automated seam tracking enhances throughput and leads to stronger, more uniform weld joints.
5. Arc Stability Monitoring
Advanced algorithms can detect subtle changes in arc characteristics and correct the welding process in real-time, preventing defects due to inconsistent arcs.
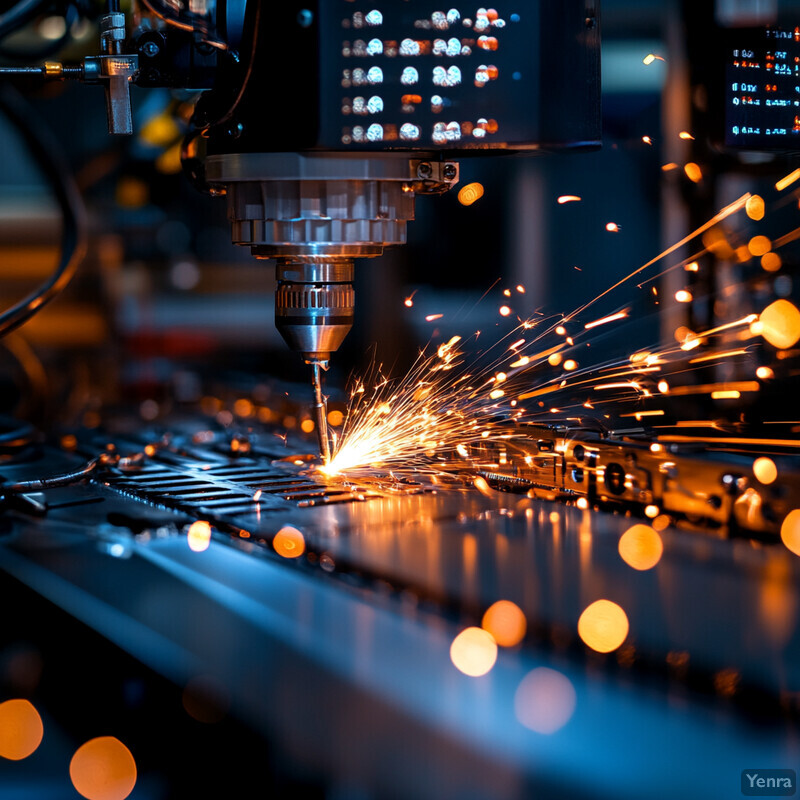
The arc’s stability during welding is a key determinant of weld penetration, bead shape, and overall quality. AI-driven analysis tools process signals from sensors measuring current, voltage fluctuations, and acoustic emissions. When instability is detected—perhaps due to contamination, gaps, or unexpected material hardness—the system can instantly adjust welding parameters or alert operators. By catching these issues in real-time, AI prevents defects from developing and ensures the weld remains uniform and structurally sound. This level of control not only improves quality but also extends the lifespan of equipment and reduces overall production costs.
6. Thermal Profile Analysis
AI-driven thermographic sensors can monitor the heat distribution across the weld and base material, ensuring proper penetration and reducing thermal distortions.
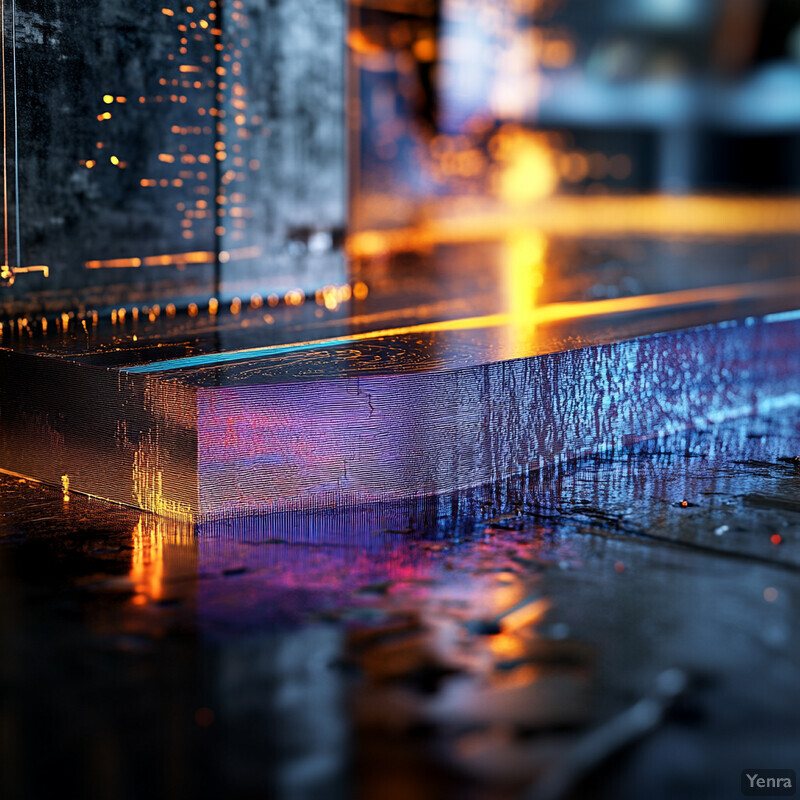
Controlling heat input is crucial to achieving the correct weld penetration and metallurgical properties. AI-based thermal imaging and sensor fusion techniques monitor the temperature distribution across the weld pool and the surrounding material. Using advanced models, the AI interprets these thermal profiles to determine if adjustments are needed to maintain the desired weld properties. If a certain area is too hot or cool, the system modifies parameters on the fly. This ensures consistent penetration depth, prevents deformation or warping, and enhances the mechanical properties of the finished weld. Over time, this leads to more predictable outcomes and a higher degree of confidence in weld integrity.
7. Adaptive Filler Material Control
Machine learning can predict when more filler wire or a different composition is needed based on real-time weld pool characteristics.
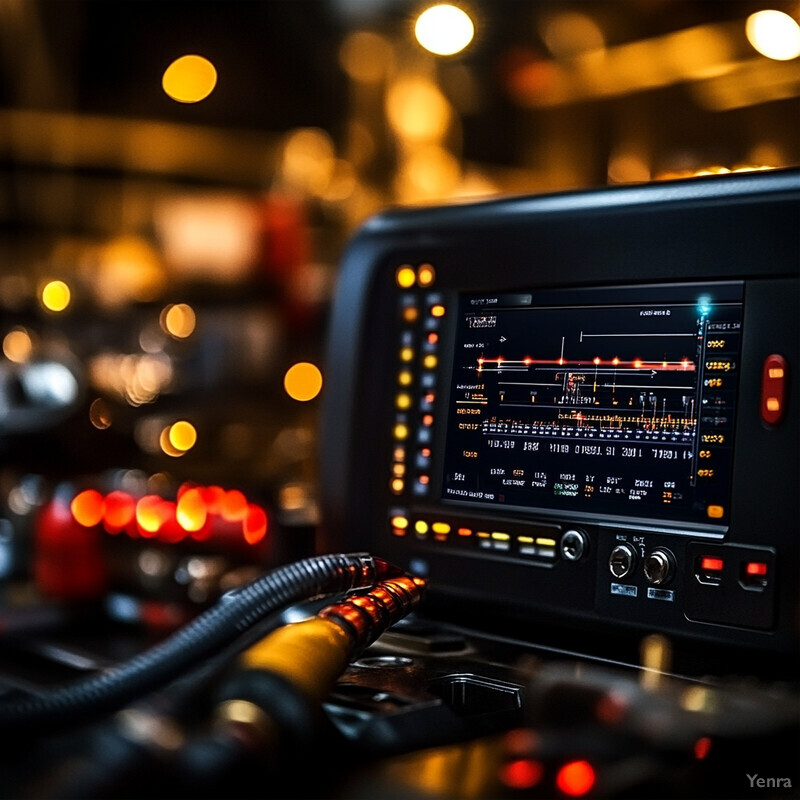
The proper delivery of filler wire and shielding gas is fundamental to producing a sound weld. AI systems can monitor the weld pool’s appearance, sound, and thermal characteristics to deduce when more or less filler material is required. For example, if the weld pool is shrinking or the penetration is insufficient, the system increases filler feed rate; if the bead is too large or the weld is overheating, it reduces the supply. By autonomously balancing these elements, the AI ensures that each weld meets the required specifications while minimizing material waste and operator intervention.
8. Expert System Integration
AI-based expert systems can combine human domain knowledge with real-time data to recommend solutions for complex welding challenges, improving decision-making.
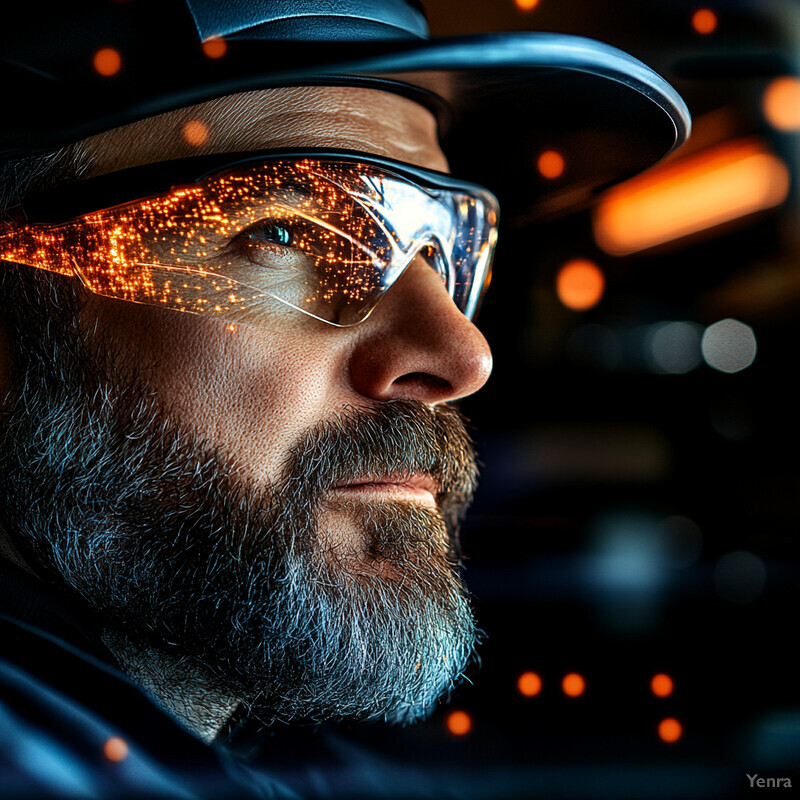
Traditional QA processes rely on human expertise that can vary widely from operator to operator. AI-driven expert systems combine vast amounts of historical welding data with coded domain knowledge from experienced welders, engineers, and metallurgists. When confronted with a new set of conditions—like welding a novel alloy or working in a constrained geometry—the system draws on its “knowledge” to suggest optimal parameters and best practices. This codification and application of expertise helps standardize quality levels, reduce training time for new personnel, and maintain a consistently high level of welding performance throughout the production environment.
9. Root Cause Analysis for Defects
Advanced analytics can identify patterns leading to defects and provide insights into underlying causes, facilitating targeted improvements.
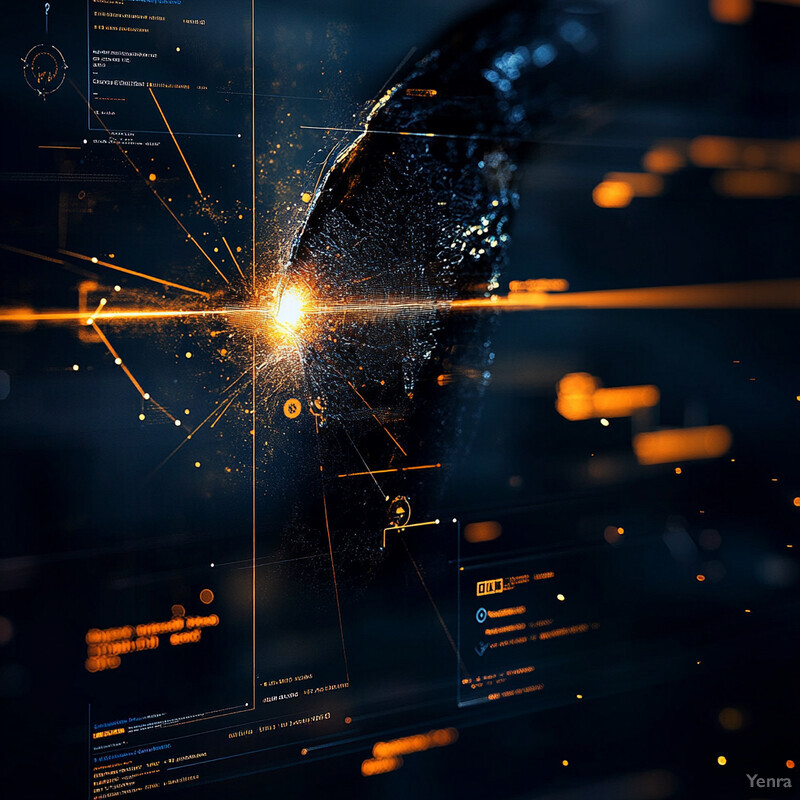
When defects occur, pinpointing the cause can be challenging. AI-driven analytics tools continuously collect and store data from sensors, logs, and previous defect occurrences. By applying machine learning and statistical techniques, the AI identifies patterns and correlations that may not be obvious to human inspectors. For instance, a recurring porosity problem might be linked to slight variations in shielding gas purity or subtle temperature shifts in the work environment. Identifying these hidden relationships enables targeted corrective actions, such as adjusting ventilation or improving gas filtration, thereby reducing the frequency of future defects.
10. Wear and Tear Prediction on Equipment
AI-driven predictive maintenance models can determine when welding torches, nozzles, and other consumables will fail, ensuring timely replacements and consistent weld quality.
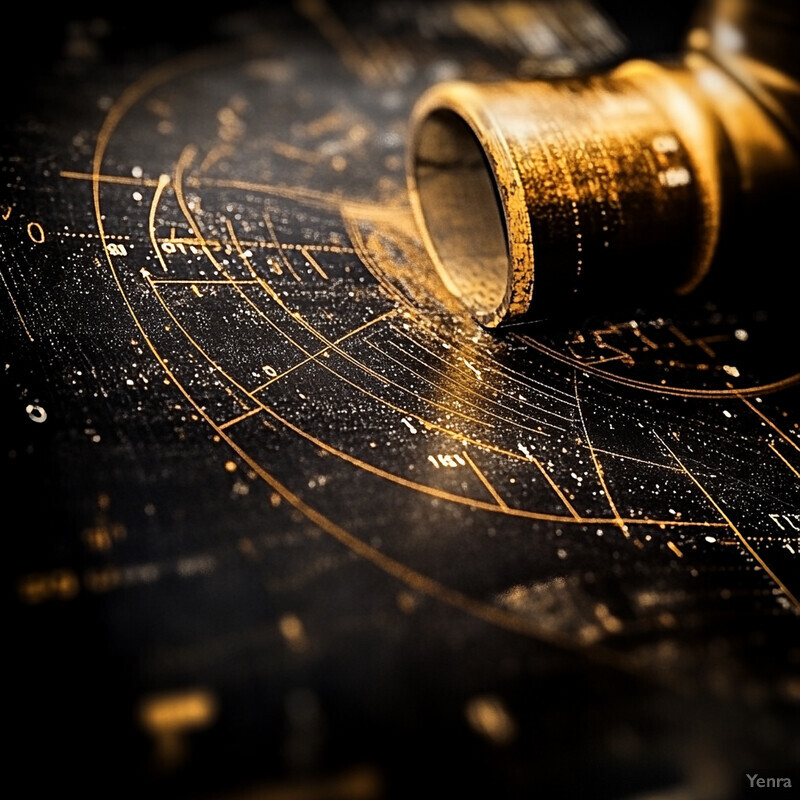
Weld quality is influenced by the condition of welding nozzles, electrodes, torches, and other consumables. Over time, these components degrade, leading to inconsistent welds and potential defects. By analyzing sensor data, operational cycles, and historical usage, AI can predict when parts are likely to fail or degrade to a point where quality might suffer. This allows maintenance teams to perform proactive replacements rather than waiting for visible failures. Such predictive maintenance not only ensures consistent weld quality but also optimizes inventory management, reduces unexpected downtimes, and extends equipment life.
11. Machine-to-Machine Communication
AI can coordinate multiple robotic welders, enabling them to share quality data and adjust collectively, improving consistency across production lines.
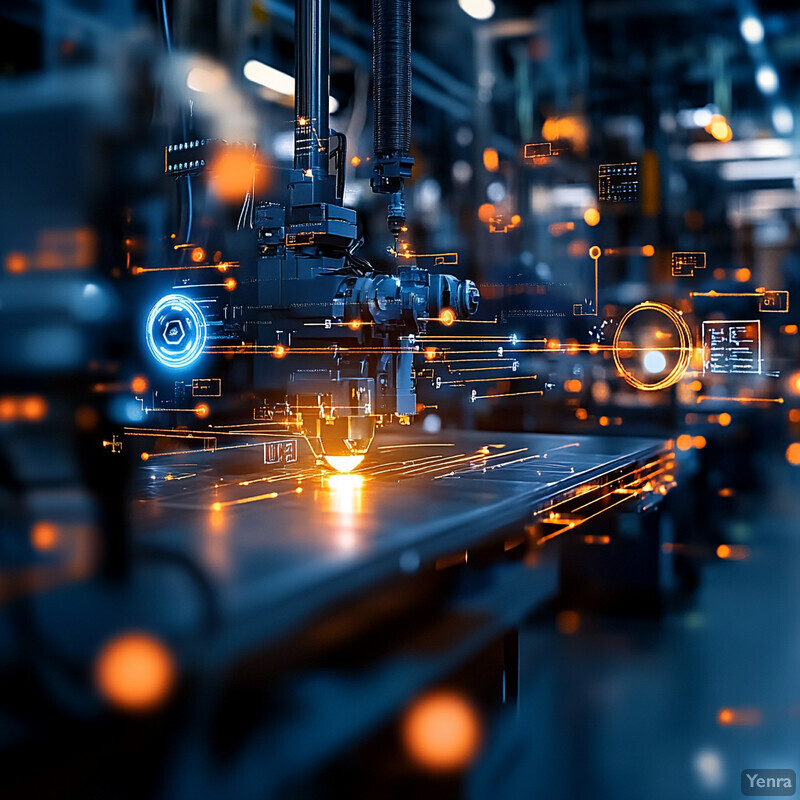
In a modern, interconnected factory environment, multiple robotic welders may work in parallel. AI can facilitate “smart” coordination among these machines, enabling them to share data about welding parameters, detected defects, and environmental changes. If one robot detects an anomaly, it can inform others, prompting them to adjust their process settings or warn operators. This collective intelligence reduces the risk of systemic errors, improves line-wide consistency, and ensures that best practices are propagated across all machines in real-time, improving overall throughput and product quality.
12. Adaptive Positioning in Complex Geometries
For intricate parts, AI can guide robotic arms to reach difficult weld joints with precision, reducing errors and scrap.
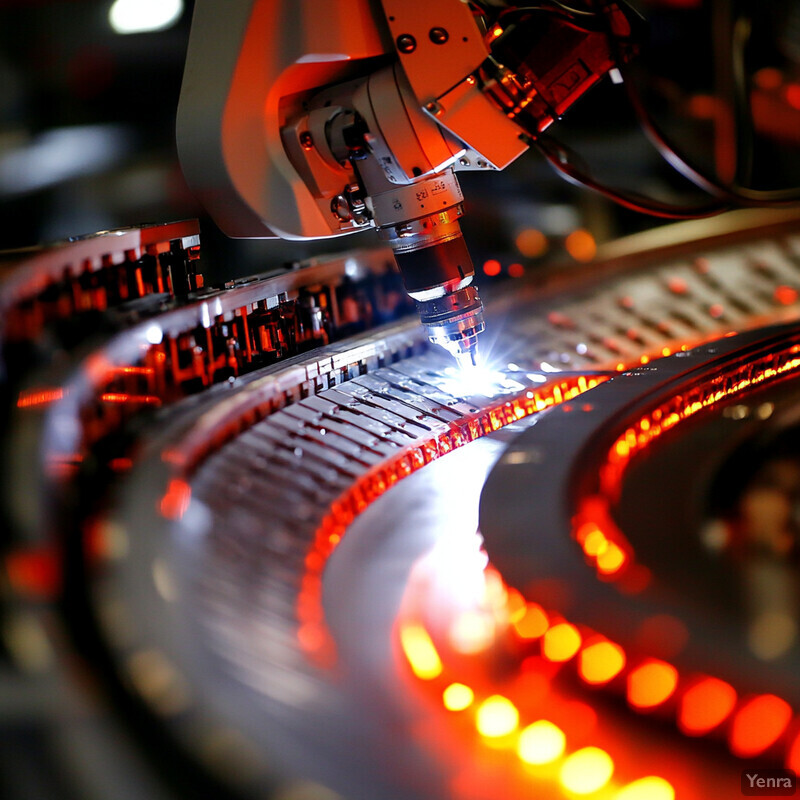
Some parts to be welded feature intricate shapes, tight corners, or variable thicknesses. AI-driven systems use advanced geometry recognition algorithms and 3D sensor data to navigate these complexities. By analyzing the shape and orientation of parts, the AI guides the welding robot to position itself at the optimal angle, reducing the likelihood of incomplete fusion, excessive spatter, or other geometric-related defects. This flexibility allows manufacturers to maintain high-quality standards even in challenging applications, reducing scrap and rework in complex component production.
13. Real-Time Weld Pool Geometry Control
AI can interpret sensor data to maintain an ideal weld pool shape, controlling penetration depth and minimizing undercut or overlap.
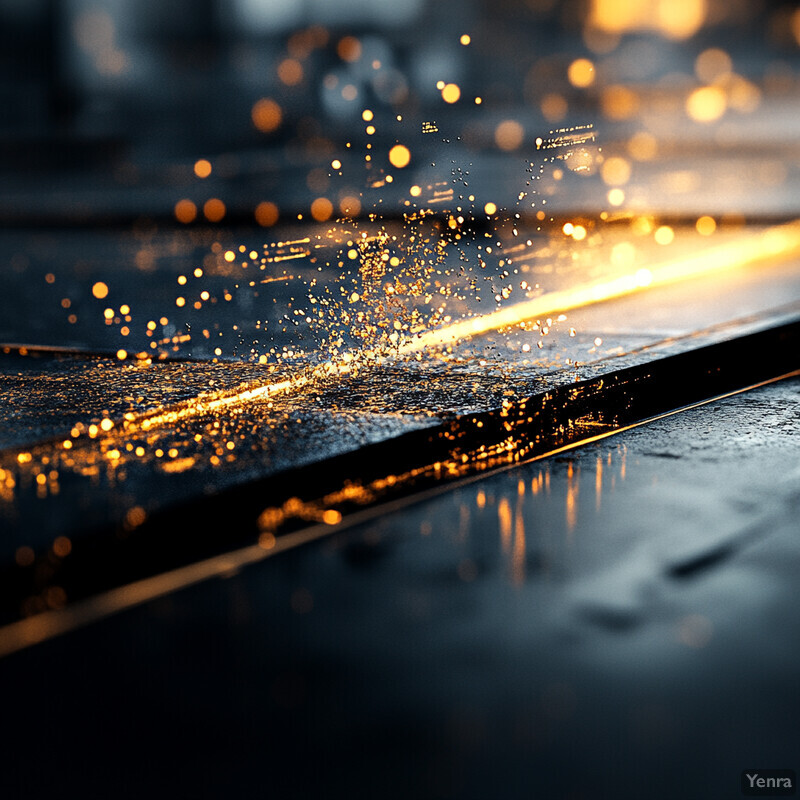
The weld pool’s shape and size directly affect the resulting weld’s mechanical properties and appearance. AI systems monitor the weld pool using cameras, infrared sensors, and other diagnostic tools, then analyze shape, fluidity, and surface tension patterns. If irregularities are detected—such as an elongated pool indicating too much heat or a narrow pool suggesting insufficient penetration—the AI adjusts parameters to restore the ideal state. This dynamic control results in uniform weld beads, minimized distortion, and predictable mechanical performance, ultimately enhancing the reliability of the welded structure.
14. Data-Driven Tool Selection
Machine learning can analyze historical outcomes to recommend the best welding wires, gases, and tooling for specific materials and joint types.
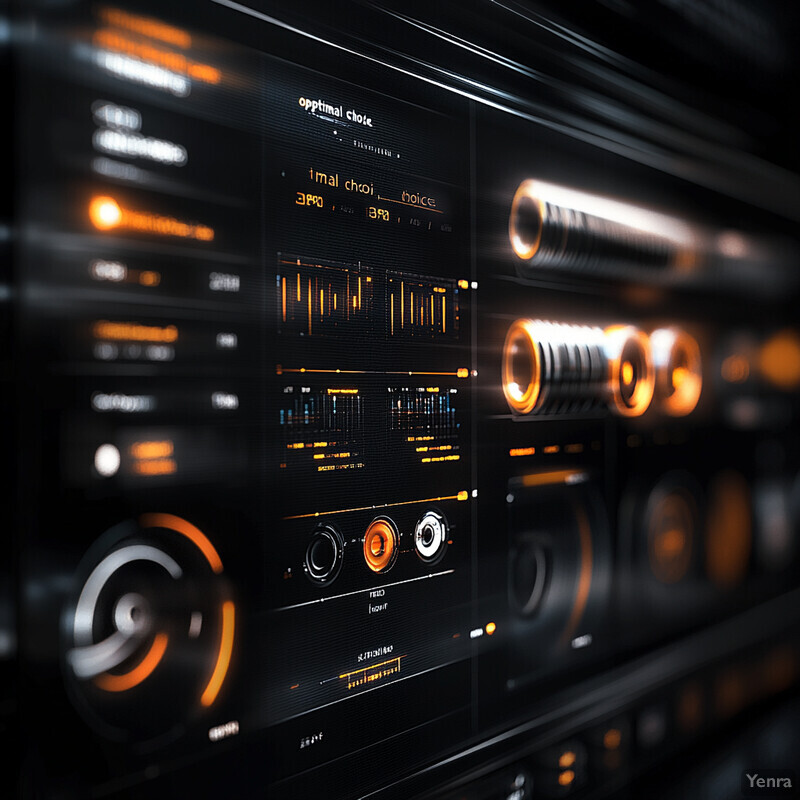
Selecting the right welding wire, electrode type, shielding gas mixture, and equipment settings can be a complex, trial-and-error process. AI shifts this paradigm by analyzing large datasets of previous weld outcomes under various conditions. By correlating materials, equipment, and parameters with achieved quality levels, the AI recommends the most appropriate consumables and tools for future jobs. This data-driven approach reduces guesswork, shortens setup times, and ensures that operators start with optimal conditions, thereby speeding the path to a consistently high-quality weld.
15. Virtual Welding Simulations
AI-powered simulators can predict outcomes of various welding parameters before live production, minimizing trial-and-error and improving first-pass yield.
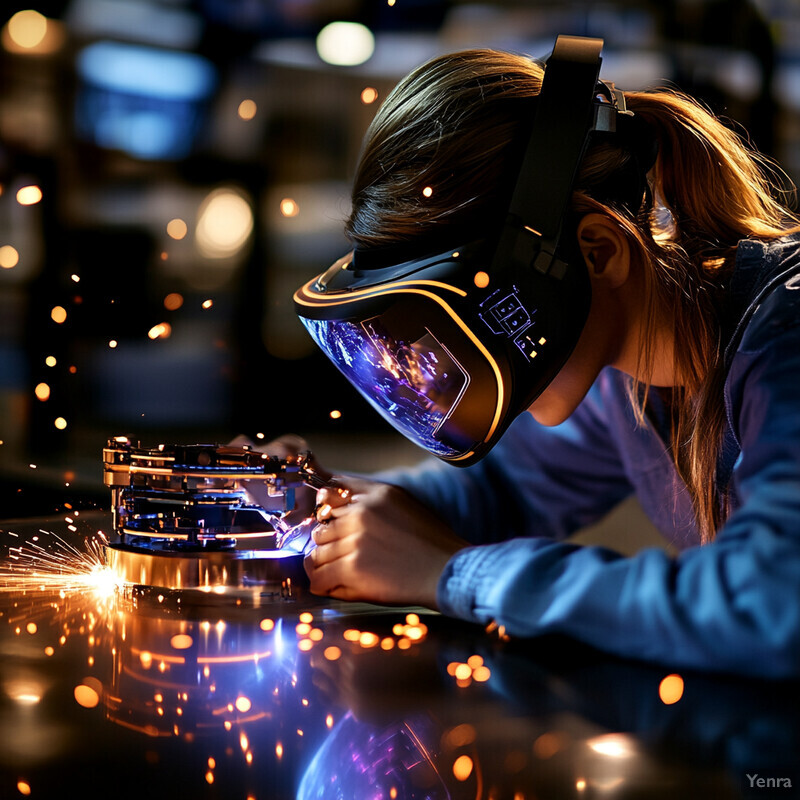
Before any metal is welded, AI-driven simulation tools enable engineers and operators to experiment with different parameters in a virtual environment. By modeling heat distribution, weld pool fluidity, and metallurgical changes, these simulations predict the likely outcome of a given welding strategy. Operators can “test” various approaches without wasting materials or risking costly rework. Once an optimal configuration is identified virtually, it can be directly implemented on the shop floor. This preemptive planning reduces production delays, improves first-pass yield, and leads to higher confidence in the end product’s quality.
16. Enhanced Training Programs
AI can assist in training less-experienced welders by providing immediate feedback on technique, parameter settings, and weld quality indicators.
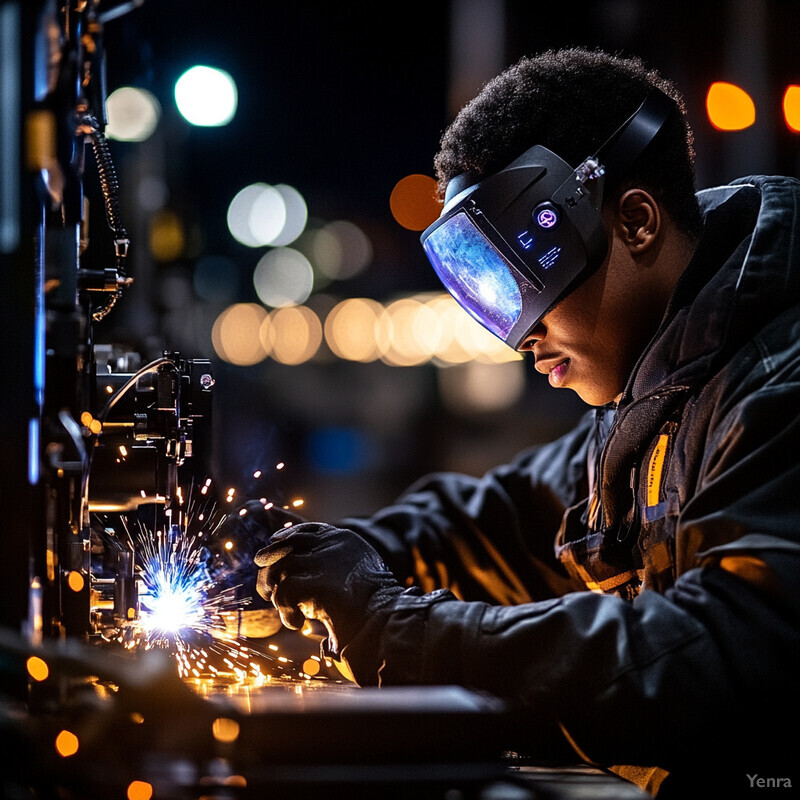
Traditional welding training heavily depends on experienced instructors and involves significant hands-on practice. AI can accelerate and enhance this learning curve by providing immediate feedback and guided instruction. Integrated with welding machines and simulation environments, AI can measure trainee performance—such as hand steadiness, torch angle consistency, and parameter selection—and provide personalized recommendations for improvement. This structured, data-driven coaching helps new welders reach competency faster, improves skill retention, and ensures that the entire welding team is better equipped to produce consistently high-quality work.
17. Closed-Loop Control Systems
AI can integrate sensor feedback into a closed-loop system that instantly corrects deviations, maintaining stringent quality tolerances without human intervention.
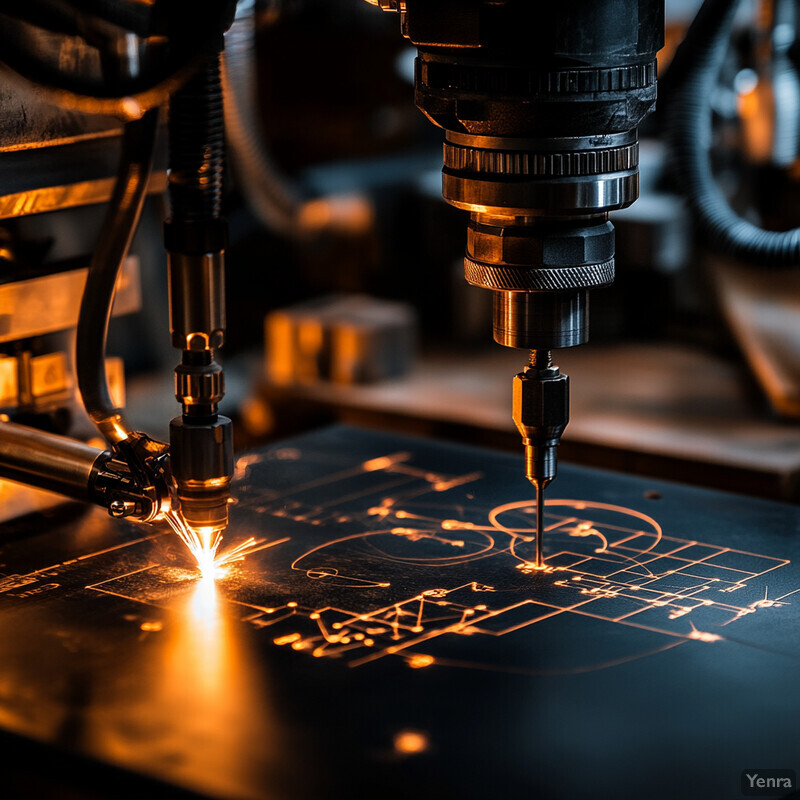
Most conventional welding setups rely on open-loop control, where machines run predefined parameters without adjusting to real-time conditions. AI enables closed-loop control by integrating sensor feedback and computational intelligence directly into the welding cycle. If heat input dips or rises unexpectedly, or if the weld seam shifts slightly, the system can automatically compensate by changing current, adjusting torch speed, or altering filler feed. This ongoing feedback loop maintains stable and optimal welding conditions, resulting in fewer defects, improved process reliability, and a more robust manufacturing operation.
18. Intelligent Scheduling and Workflows
By analyzing production data, AI can optimize the welding sequence and timing, balancing workloads to maintain quality across the entire production line.
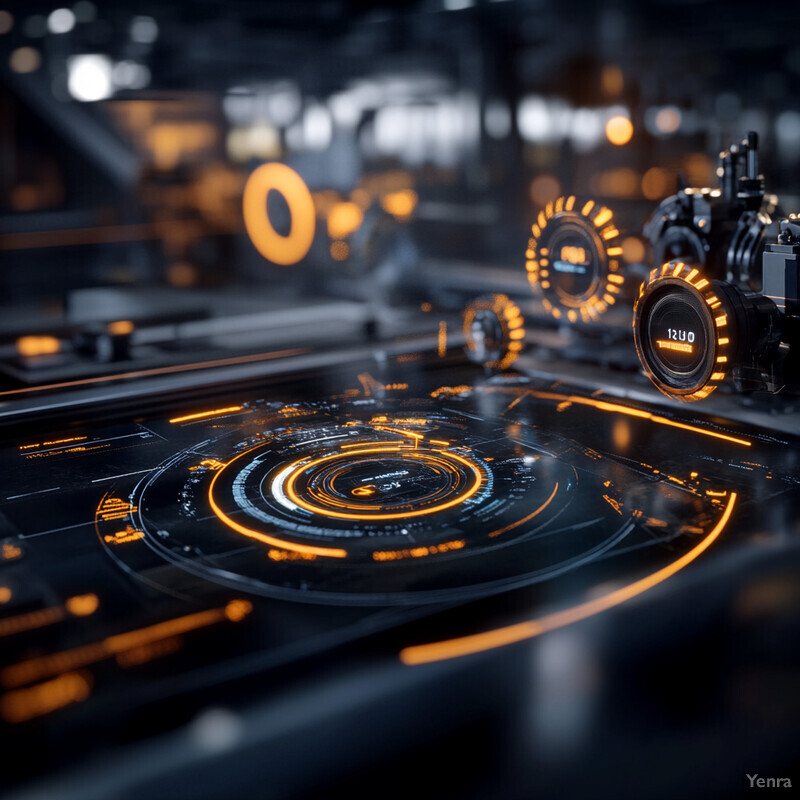
In a busy production environment, keeping track of welding tasks, machine availability, and material constraints can be complex. AI-driven scheduling systems analyze operational data, backlog, equipment capabilities, and historical performance to optimize workflow planning. By aligning welding tasks with machine readiness and material availability, these systems ensure that weld quality does not suffer from rushed jobs, improper sequencing, or overburdened operators. This results in a more balanced workload, higher efficiency, and consistent adherence to quality standards throughout the production line.
19. Multi-Modal Inspection
AI can merge data from visual, infrared, ultrasonic, and acoustic sensors to get a holistic view of weld quality, increasing the accuracy of defect detection.
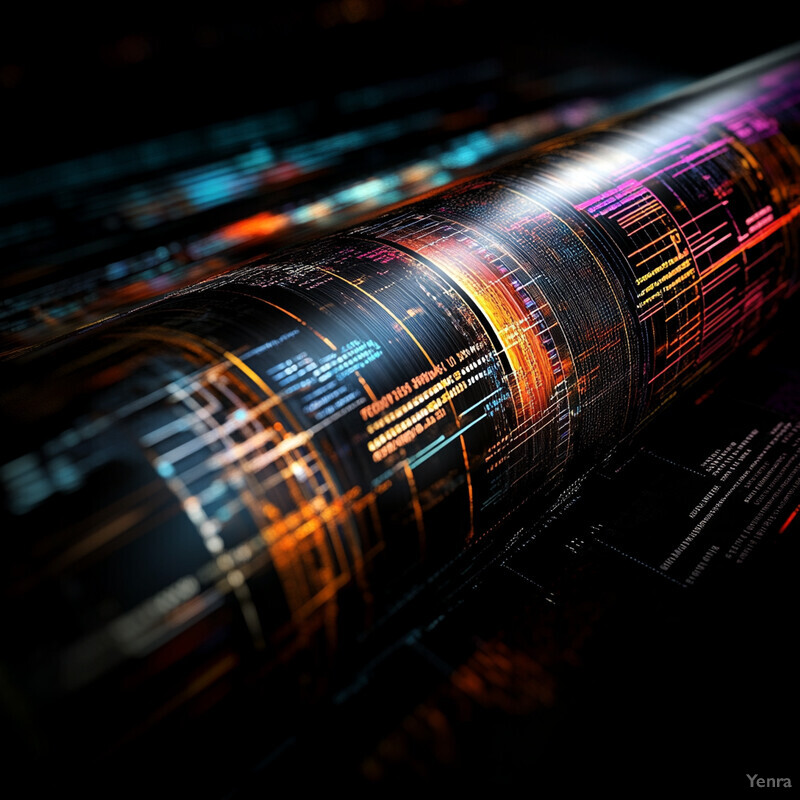
Welding defects are not always visible to the naked eye; some might be internal or too subtle for standard vision inspection. AI can integrate data from multiple inspection modalities—such as ultrasonic testing, infrared thermography, acoustic emission, and high-resolution imaging—to build a comprehensive understanding of weld integrity. By correlating data streams and recognizing complex patterns, the AI can detect hidden flaws, voids, or inclusions that might be missed by single-method approaches. This holistic inspection capability leads to more accurate assessments, improves trust in final product quality, and reduces the risk of structural failures downstream.
20. Integration with Industry 4.0 Ecosystems
By connecting to smart factory networks, AI-driven welding quality assurance can interact with other manufacturing processes, ensuring seamless quality control across the entire supply chain.
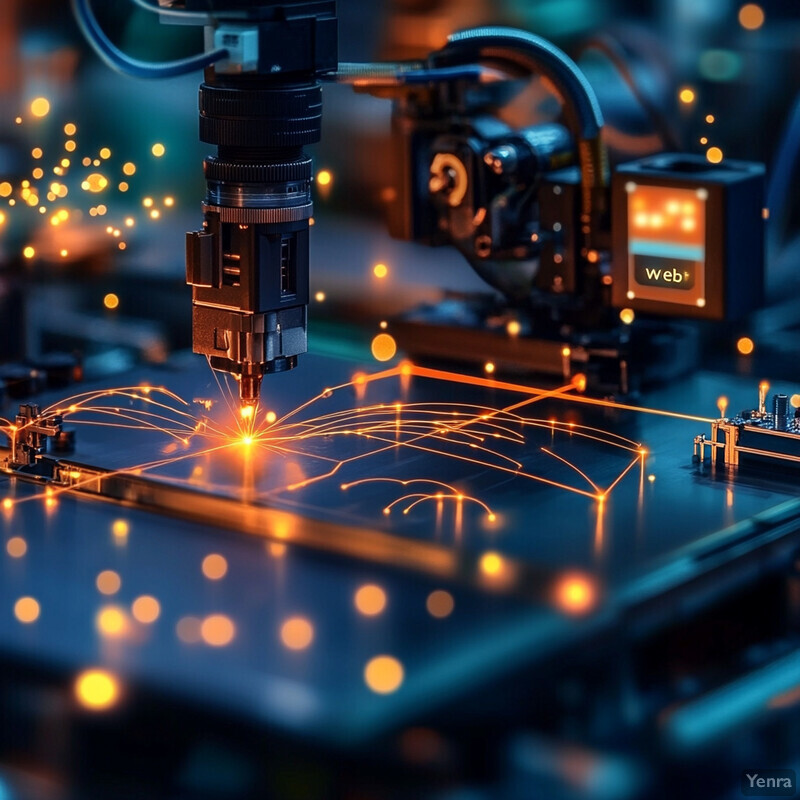
As smart factories become the norm, welding no longer stands alone. AI-driven quality assurance integrates seamlessly with other production systems, enabling real-time data sharing with design, machining, assembly, and finishing processes. By feeding back quality-related insights, the welding station can help upstream and downstream operations adjust their methods. This connectivity supports traceability, ensuring that when a defect is discovered, the cause can be traced back through the digital thread. Ultimately, Industry 4.0 integration fosters continuous improvement, streamlined communication, and a cohesive approach to manufacturing excellence across the entire supply chain.