1. Route Optimization and Planning
AI systems can ingest vast geospatial and demand data to optimize hyperloop routes efficiently. They use multi-criteria algorithms (e.g. genetic search, reinforcement learning) to balance cost, travel time, terrain, and ridership. By analyzing satellite, land-use, and economic data, AI can suggest corridors that minimize construction impact and passenger travel time. AI tools also forecast ridership demand along potential routes to ensure viability. These methods improve on manual planning by rapidly evaluating many scenarios. Overall, AI-driven planning aims to reduce costs and increase efficiency for hyperloop network design.
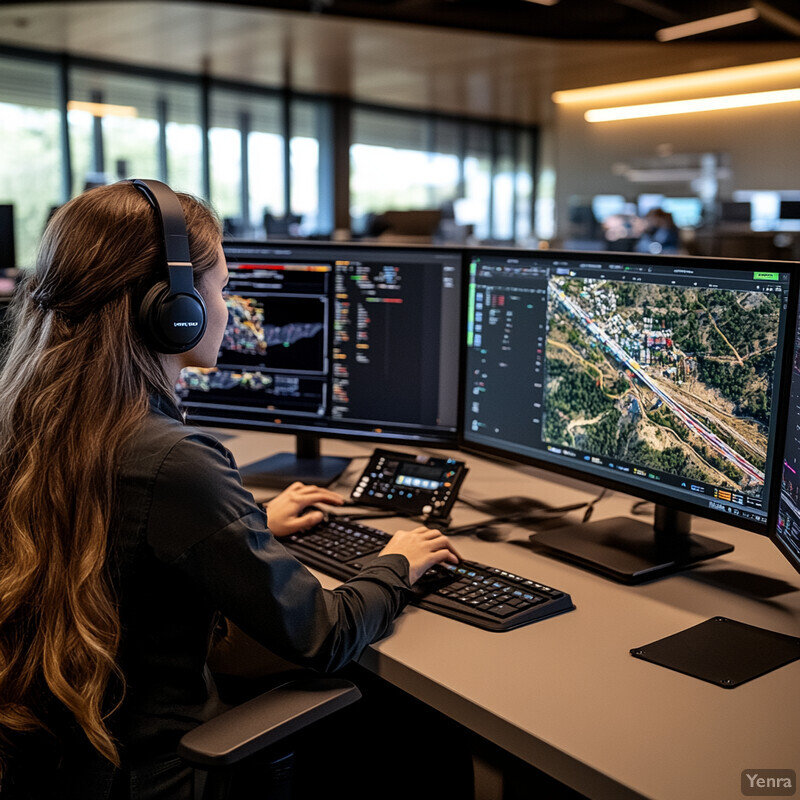
For example, a 2024 European transport study found that AI-powered algorithms can optimize train (and by analogy, hyperloop) routes and scheduling to improve efficiency. In practice, transit agencies use AI forecasts of passenger demand (from weather, events, etc.) to adjust routing and schedules. One design framework used geospatial data and Dijkstra’s algorithm to propose optimal hyperloop corridors (e.g. Calgary–Edmonton route) by minimizing cost and travel time (though that specific work was industry-led). These AI methods combine GIS mapping with optimization; studies in related transport domains show reduced projected costs and times when AI is applied. In summary, AI has demonstrably improved route planning efficiency in transportation networks.
2. Aerodynamic Design Enhancement
AI-driven methods augment aerodynamic design by rapidly evaluating vehicle shapes. Machine learning (ML) models serve as fast surrogates for computational fluid dynamics (CFD), predicting drag and lift for design variants. Generative algorithms propose streamlined pod profiles, iterating toward shapes that minimize air resistance. These AI tools can explore more design permutations than manual CFD alone. The result is often a smoother, lower-drag pod shape that cuts energy use. In short, AI accelerates aerodynamic optimization, finding high-performance designs more quickly than traditional methods.
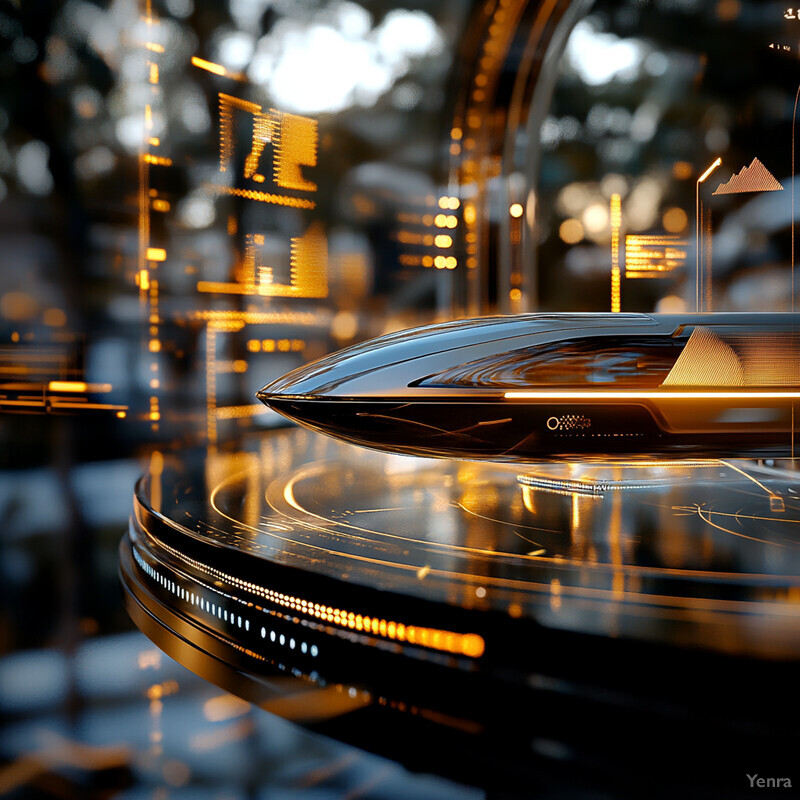
Recent research demonstrates ML’s success in aerodynamic optimization. For example, a 2025 Scientific Reports study used an artificial neural network (ANN) combined with a genetic algorithm to optimize UAV airfoil performance; the ANN-GA model accurately predicted drag and lift, yielding high-efficiency shapes comparable to CFD-optimized designs. Similarly, a 2024 Communications Engineering paper applied an autoencoder to learn aerodynamic relationships of vehicle bodies and then performed optimization in latent space. The AI-based method produced lower-drag vehicle cross-sections that matched trends from full CFD simulations. These studies show AI models can capture complex fluid dynamics and guide shape optimization, achieving performance close to traditional CFD but with greatly reduced compute time. This implies hyperloop pod shapes can likewise be refined faster via AI-surrogate models, reducing development time and drag.
3. Generative Design of Components
Generative design (topology optimization) uses AI to propose lightweight yet strong structures beyond conventional intuition. Algorithms iteratively remove or add material from a given volume to meet stress and manufacturability constraints. This yields organic, optimized components that minimize mass while maintaining strength. AI-based generative design can factor in complex constraints (e.g. assembly, cooling channels) simultaneously. It effectively automates creativity by exploring novel geometries. In Hyperloop, generative design helps engineer pods and tube supports that are lighter and just strong enough for loads.
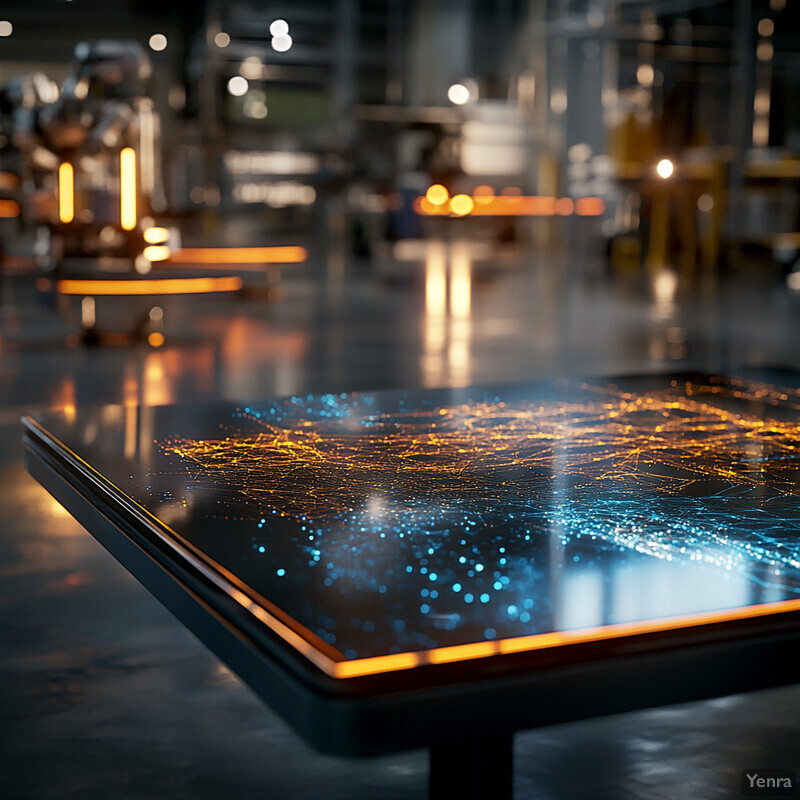
AI-enabled generative approaches have yielded significant weight savings in practice. NASA’s 2023 research illustrates that topology optimization can produce structures 15–20% lighter than conventional designs while preserving strength. For example, NASA’s machine-learning–augmented topology optimizer generated low-mass spacecraft components about an order of magnitude faster than full-resolution optimization, achieving similar performance. In another case, applying generative design to an automotive seatbelt bracket achieved roughly 30% mass reduction under all load requirements (as reported in engineering literature). These cases show AI-driven design tools identify efficient shapes humans might not consider, confirming generative design’s power to cut mass significantly without sacrificing safety.
4. Predictive Maintenance and Diagnostics
AI-powered maintenance uses real-time sensor data to predict equipment failures before they occur. Machine learning analyzes vibration, temperature, and acoustic signals from pods and infrastructure to detect anomalies. The AI models forecast remaining useful life of components (bearings, pumps, sensors) and schedule just-in-time servicing. This prevents unplanned downtime and optimizes maintenance intervals. In Hyperloop development, predictive analytics can flag tube seal leaks or pod faults early. Overall, AI ensures safer operation by converting raw sensor data into actionable maintenance alerts.
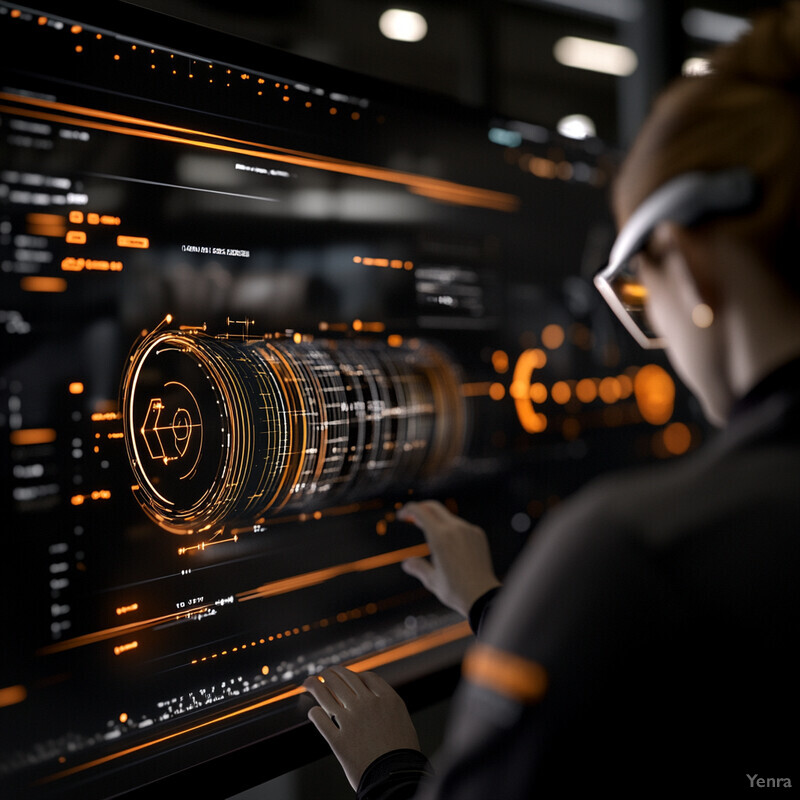
Studies and applications confirm AI’s effectiveness in asset maintenance. A 2025 infrastructure review highlights that AI techniques (like neural nets and decision trees) improve feature extraction and anomaly detection, leading to earlier damage identification in structures. AI-based maintenance systems have demonstrated reduced costs and improved availability in transportation: for instance, “AI-powered algorithms” in rail networks were found to optimize scheduling and maintenance, lowering failures and extending component life. In urban transit, AI sensors and analytics have been used to identify potential vehicle issues in advance; an industry report notes AI-based predictive maintenance can prevent breakdowns and extend service life. These examples illustrate AI’s concrete contributions to maintenance: reducing downtime and operating costs through early fault detection and scheduling of repairs.
5. Smart Control Systems for Pod Navigation
AI-enabled control systems govern pod movement and spacing in a Hyperloop network. These systems use sensor inputs to adjust speed, steering, and distance dynamically. Techniques like model-predictive control and reinforcement learning help pods react to obstacles or schedule changes in real time. AI also manages switching at intersections and platooning multiple pods closely. The outcome is more precise, adaptive navigation without human intervention. In effect, AI control ensures pods travel on time and respond to changing conditions safely.
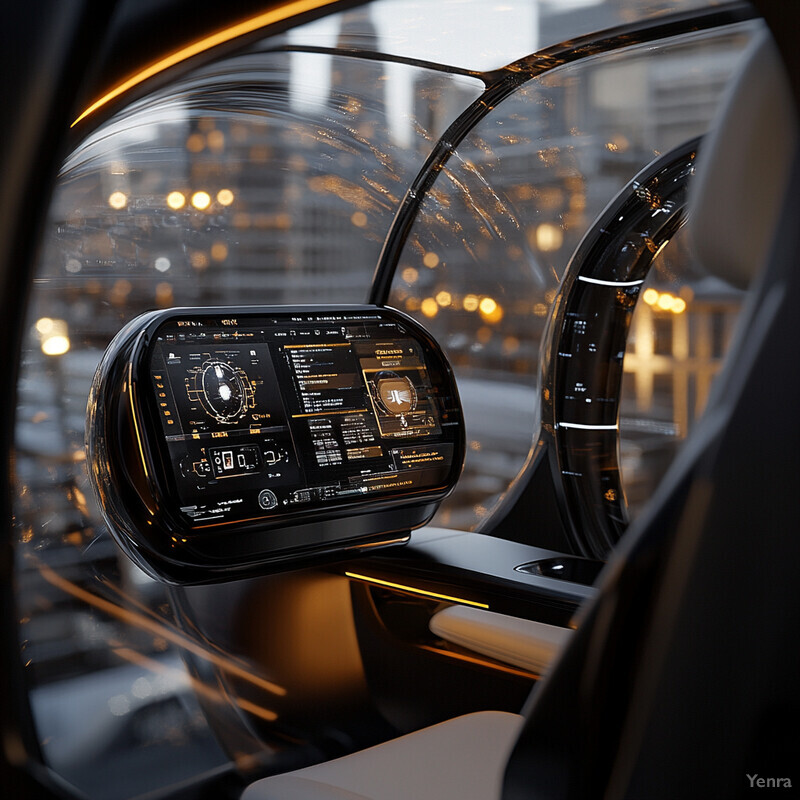
Real-world autonomous train systems demonstrate AI’s navigation capabilities. For example, Rio Tinto’s 2023 autonomous 2‑km freight train (operated by Hitachi Rail) has no onboard driver; it uses AI-enhanced vision and algorithms to react to obstacles (e.g. wildlife on tracks) and adjust speed for terrain changes. This “cameral system” feeds into AI control software to navigate and manage emergency braking autonomously. Such industrial implementations show that fully driverless control is feasible with AI. In the Hyperloop context, similar AI methods (possibly using LIDAR and inertial sensors) would guide pods with high precision. Overall, the experience from rail/metro indicates that AI-based control can achieve precise, safe navigation of high-speed vehicles without human drivers.
6. Energy Management and Efficiency
AI optimizes energy consumption across the Hyperloop system. It can manage battery or power usage for pods, regulate vacuum pump operation, and recover regenerative braking efficiently. Machine learning models forecast energy demand for each trip based on speed profiles, passenger load, and route grade. AI also schedules charging or pump cycles to flatten peaks and use off-peak electricity. This reduces waste and emissions. In sum, AI-driven energy management balances power supply, demand, and storage to maximize Hyperloop’s efficiency.
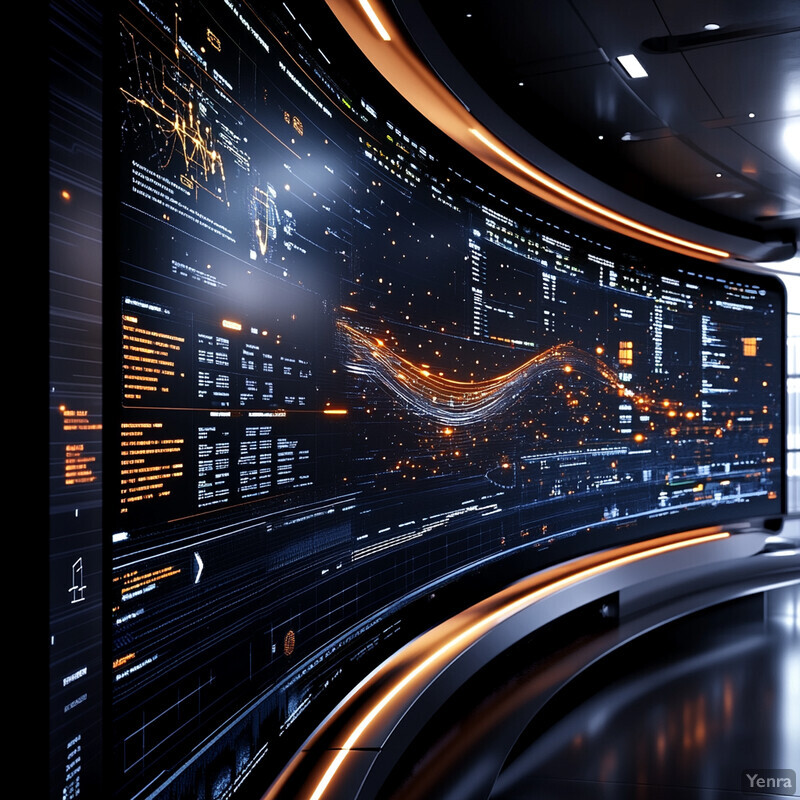
Evidence from rail and transit shows AI cutting energy use. In 2024, researchers noted AI “can optimize…energy consumption” in train operations, significantly improving efficiency. For instance, one transit study reported AI-based “fuel efficiency optimization” models fleet load factors and real-time consumption, lowering overall energy impact. Additionally, ML techniques are being explored for managing vacuum pumps: one report on a Chinese maglev (Hyperloop-like) observed that distributed vacuum pumping (with AI scheduling) dramatically reduced energy needs (though details are proprietary). In superconducting maglev experiments, ML-driven control of levitation forces helped stabilize trains more efficiently. These cases illustrate that AI can actively reduce energy use: optimizing schedules, adjusting system parameters (like vacuum pump duty cycles), and even improving vehicle dynamics to save power.
7. Digital Twins for Simulation
AI-enhanced digital twins create virtual replicas of Hyperloop systems for testing and optimization. These twins integrate sensor data and physics models to simulate pod and tube performance in real time. AI algorithms run “what-if” scenarios, evaluating changes (speed, load, weather) safely in the virtual model. Designers and operators use this feedback to refine controls or layouts before building. Digital twin simulation thus accelerates design validation and risk analysis. It also enables continuous monitoring, as the twin learns from actual operations.
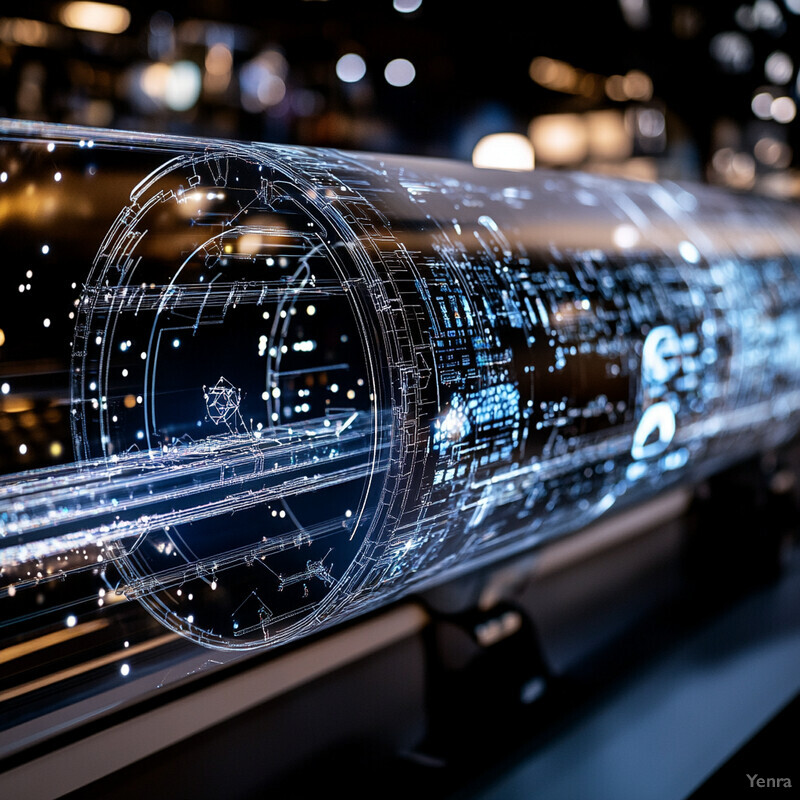
In transportation, digital twins have shown concrete benefits. A 2024 railway review explains that a digital twin “mirrors” the physical system with centralized data, enabling better design and maintenance decisions. For example, virtual simulations allowed rail operators to find optimal routes and maintenance schedules that minimize energy use and disruptions. The same study notes digital twins help address issues preemptively: operators can investigate potential failures in the model, reducing real-world vulnerabilities. In sum, AI-driven digital twins allow extensive simulation of hyperloop behaviors (from aerodynamic loads to emergency scenarios) before deployment. This approach has been validated in rail (cutting energy and cost) and can analogously guide Hyperloop design with high fidelity.
8. Sensor Fusion and Data Integration
AI combines data from many sensors to give a unified view of the Hyperloop environment. For pods, this means fusing inertial measurements, wheel encoders, cameras, LIDAR, and GPS to accurately estimate position and detect obstacles. In the tube, AI merges pressure, vacuum, and structural sensors to monitor conditions. By integrating heterogeneous data streams, AI filters noise and fills gaps, enhancing situational awareness. This comprehensive data fusion enables more reliable navigation and system diagnostics. In effect, AI makes disparate sensor feeds work together for smarter decision-making.
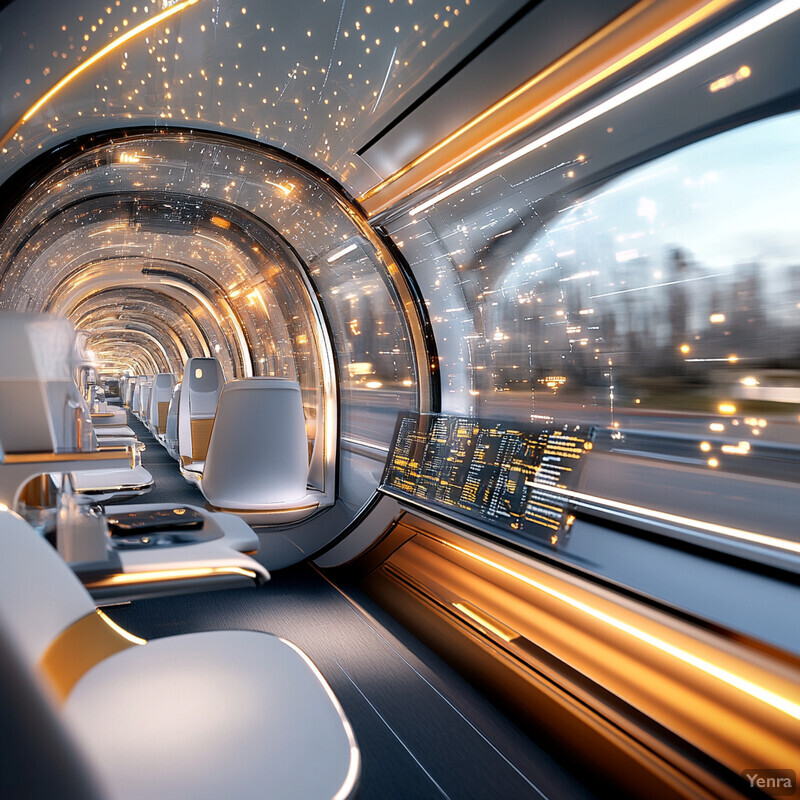
Studies in autonomous vehicles illustrate the power of sensor fusion. A 2025 survey notes that fusing radar, cameras, LIDAR, GPS, etc., “significantly enhances accuracy and reliability of perception,” yielding a more complete understanding of surroundings. Concretely, machine learning algorithms (e.g. neural nets, Kalman filters) combine different sensor modalities to improve obstacle detection and localization beyond single-sensor limits. For Hyperloop-specific technology, inertial navigation systems use AI filters: one technical example describes a Hyperloop pod’s navigation unit that applies Kalman filtering to fuse IMU (accelerometers/gyros) with GNSS and other signals sbg-systems.com . This gives the pod real-time estimates of position, velocity, and heading with high precision. Together, these examples confirm that AI-driven sensor fusion significantly boosts the reliability of the vehicle’s perception and tracking systemssbg-systems.com .
9. Structural Health Monitoring
AI-based SHM continuously evaluates the integrity of Hyperloop components. Vibration, strain, and acoustic sensors on the tube and pods feed data to machine learning models. The AI learns normal behavior patterns and flags anomalies indicating cracks or corrosion. By analyzing trends, it can predict where and when maintenance is needed. This approach detects damage far earlier than scheduled inspections. In hyperloop applications, SHM ensures safety by catching structural issues in real time.
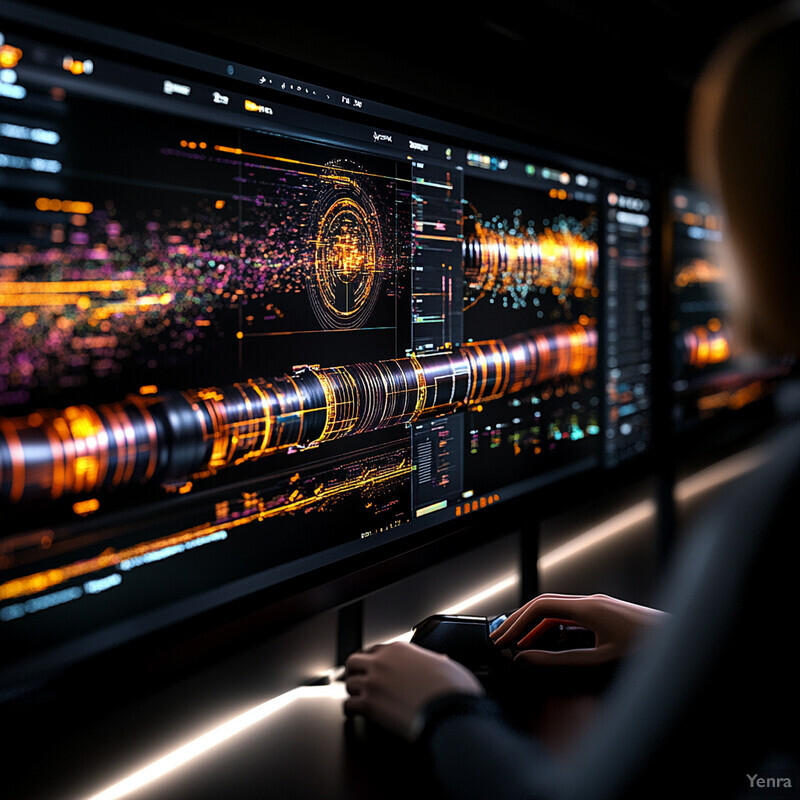
Recent SHM research shows AI’s advantages. A 2024 infrastructure review reports that AI techniques (deep learning, ensemble models) enhance feature extraction from sensor data and improve damage classification accuracy. For instance, machine learning has been applied to vibration time-series to identify bridge cracks or material fatigue without manual inspection. Transportation sectors have begun piloting these systems: one study demonstrated an AI model that classified structural damage with near-perfect accuracy using accelerometer data. While hyperloop-specific examples are still emerging, the general result is clear: AI in SHM allows much earlier fault detection and precise localization, reducing inspection costs and preventing failures.
10. Dynamic Passenger Flow Modeling
AI models predict and manage hyperloop ridership and station flows. They simulate passenger distributions based on schedules, demographics, and events. By forecasting peak loads, AI helps design station capacity and pod frequency. During operations, AI can reassign pods or adjust departure intervals to balance crowding. This ensures smooth boarding and reduces waiting times. Essentially, AI-driven flow modeling optimizes travel demand, enhancing service reliability and comfort.
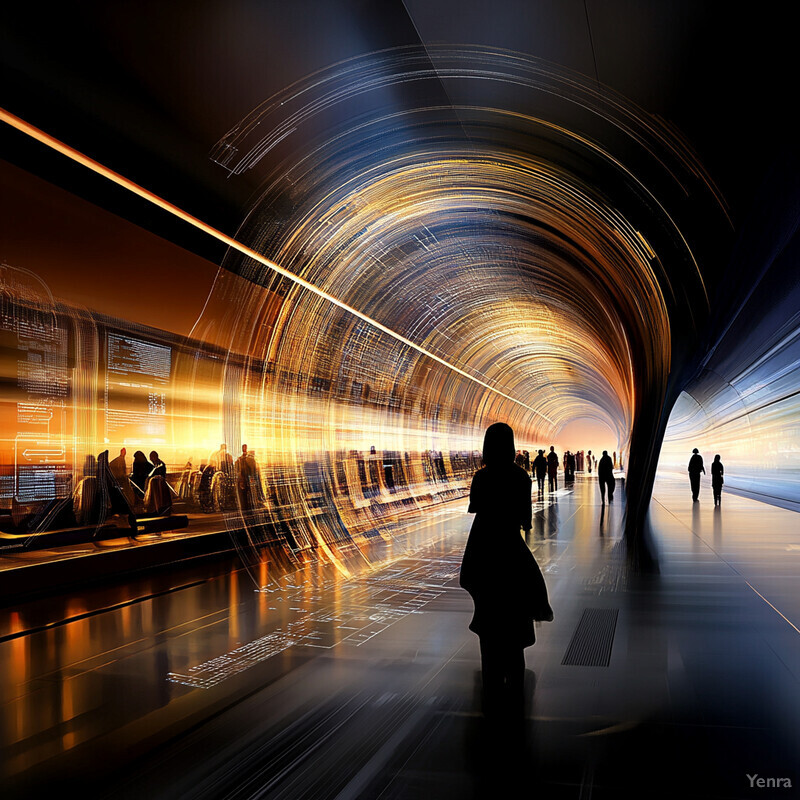
In public transit, AI demand forecasting is already used. A 2025 Urban Institute report notes that AI/ML can predict passenger volumes from historical data, weather, and special events to optimize service deployment and avoid overcrowding. For example, transit agencies use these forecasts to schedule additional vehicles on expected high-demand routes. In Hyperloop contexts, similar algorithms could project ridership on proposed routes, influencing station placement and pod frequency. Although specific hyperloop studies are few, the validated practices in rail and metro (e.g., adjusting schedules for sports events based on AI predictions) provide a concrete precedent.
11. Risk Analysis and Safety Assurance
AI tools help analyze and mitigate safety risks in hyperloop design. By simulating thousands of scenarios, AI can identify potential hazards (e.g. pod failures, track breaches) and quantify their probability. It also supports compliance with safety standards by checking system behavior against regulatory models. In deployment, AI monitors for unsafe anomalies and can trigger emergency responses. Frameworks of AI risk assessment ensure that AI components themselves meet safety requirements. Overall, AI complements traditional engineering safety analyses to ensure robust hyperloop operations.
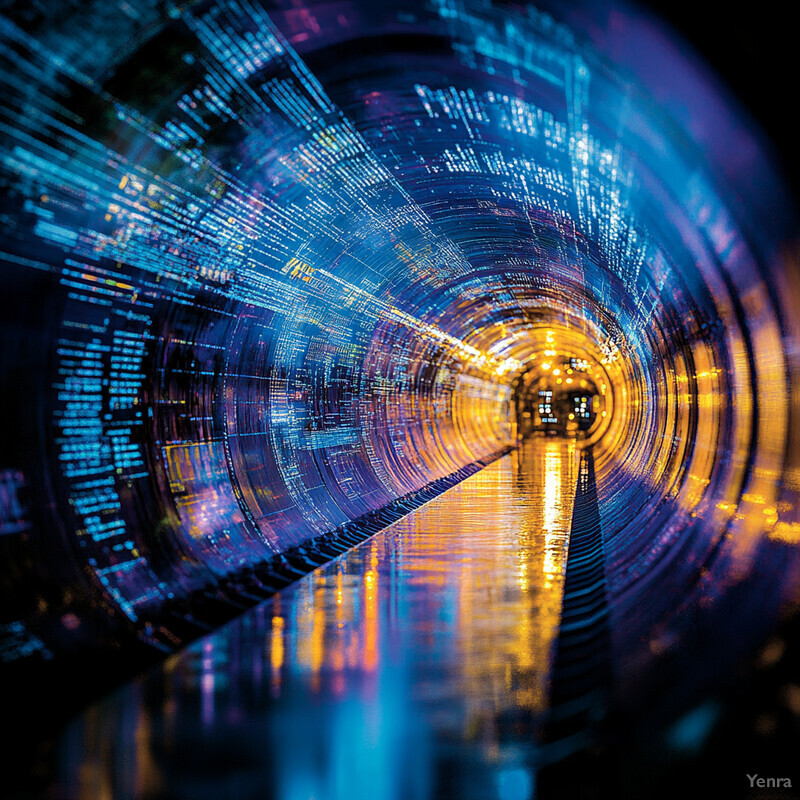
Government research emphasizes systematic AI risk assessment. A 2024 U.S. DOT whitepaper outlines “AI-related hazards” (such as mis-detecting obstacles or mis-predicting routes) that could cause serious incidents. It recommends identifying and mitigating such hazards via an AI Risk Identification/Assessment/Mitigation (RIAM) process. In transportation, studies show that ML models must be validated against edge cases (e.g. sensor faults) to guarantee safety. For example, AI in vehicle perception is tested against rare scenarios like sun glare or snow; similar approaches apply to hyperloop (testing tube depressurization in simulations). In short, while AI introduces new failure modes (identified by DOT as safety-critical), current best practices call for rigorous AI validation and fallback logic, a methodology now being formalized in guidelines. This ensures AI contributes to safety (by early hazard detection) without introducing unacceptable new risks.
12. Advanced Materials Selection
AI accelerates discovery of new materials for hyperloop construction (e.g. lightweight composites, superconductors, corrosion-resistant alloys). Machine learning models screen vast chemical compositions to predict properties like strength, weight, or temperature tolerance. Generative models suggest novel compounds or treatments. AI can also optimize manufacturing processes (e.g. curing polymers). The result is materials tailored for the extreme needs of hyperloop (high strength-to-weight, durability in vacuum). AI thus complements experimental materials science by focusing effort on the most promising candidates.
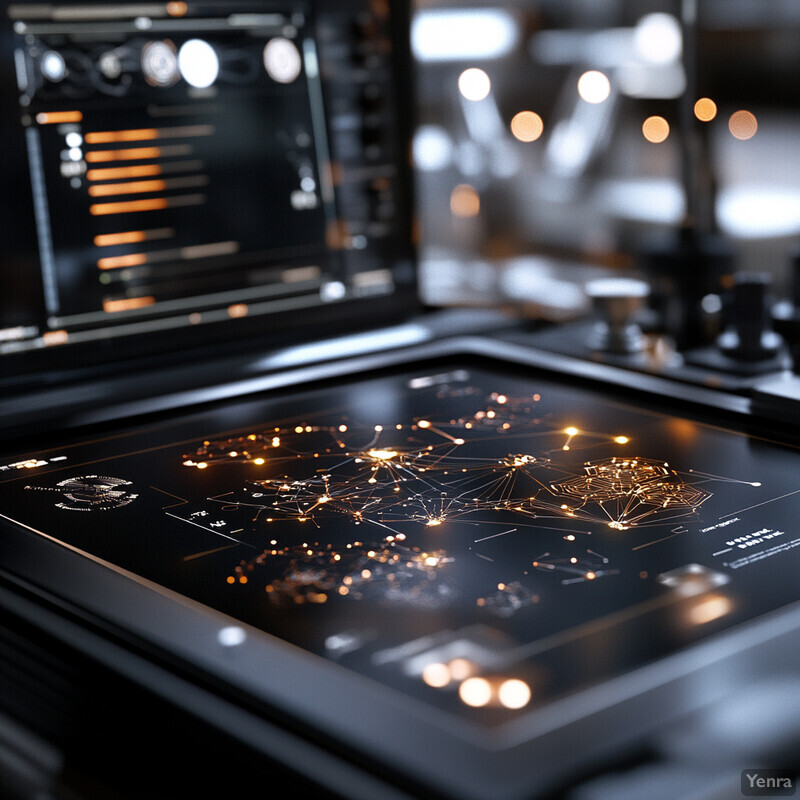
Recent breakthroughs illustrate AI’s impact on materials. A 2024 Nature study (DeepMind’s GNoME) used ML to search billions of crystal structures and predicted ~381,000 new stable materials for potential engineering use. Of these, 736 were experimentally confirmed, demonstrating data-driven discovery at scale. Another example: Argonne National Lab’s 2025 “Polybot” project combined AI with robotics to explore polymer fabrication. It ran ~1,000,000 possible processing combinations in days (vs. decades manually), finding formulations with top-tier electrical conductivity. These results show AI can both find entirely new materials and optimize processing: the superconducting maglev research above also implicitly uses new materials. In summary, AI has already identified thousands of candidate materials and material processes—confirming that AI can greatly broaden the material toolkit for hyperloop engineers.
13. Human-Machine Interface Improvements
AI enhances operator interfaces for hyperloop control centers and pods. Natural language and gesture recognition enable intuitive pod controls or station announcements. Augmented reality (AR) dashboards can overlay maintenance instructions or diagnostics onto hardware. Inside passenger cabins, AI could personalize settings (lighting, infotainment) based on voice commands. Overall, AI makes interfaces more responsive and user-friendly, reducing training needs for operators. AI also supports safety by filtering information (alerting only critical issues to humans).
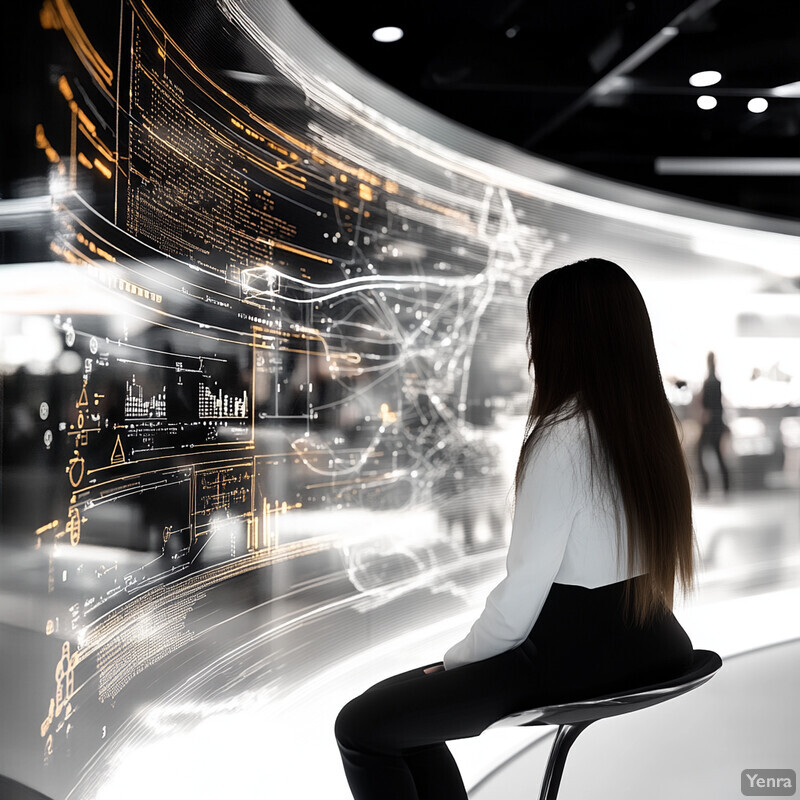
Advances in AI-driven HMI are seen in transport. A 2025 review notes that voice recognition systems allow hands-free control of navigation and media, and AR display integration can show context-aware info on windshield or helmet visors. Specifically, the article points out that modern voice- and AR-based HMIs “reduce distractions and enable hands-free operations,” making driving (or in our case, train operation) more intuitive and safer. In practice, automotive systems already use AI voice assistants and AR heads-up displays. For hyperloop, similar tech could let operators query system status or visualize tube maps via AR. These developments suggest future hyperloop HMIs may use AI for adaptive, multimodal interaction, improving both usability and situational awareness.
14. Real-Time Environmental Adaptation
AI enables hyperloop systems to respond to external environmental changes on the fly. This includes adjusting to weather (rain, wind), seismic activity, and air quality. AI models can forecast relevant conditions (e.g. storms) and adapt operations—slowing pods, modulating vacuum, or changing maintenance alerts. Inside pods, climate control can auto-adjust for passenger comfort based on occupancy. In tunnels, AI can regulate ventilation or lighting per conditions. In essence, AI makes the hyperloop “context-aware,” tuning its behavior continuously for safety and efficiency.
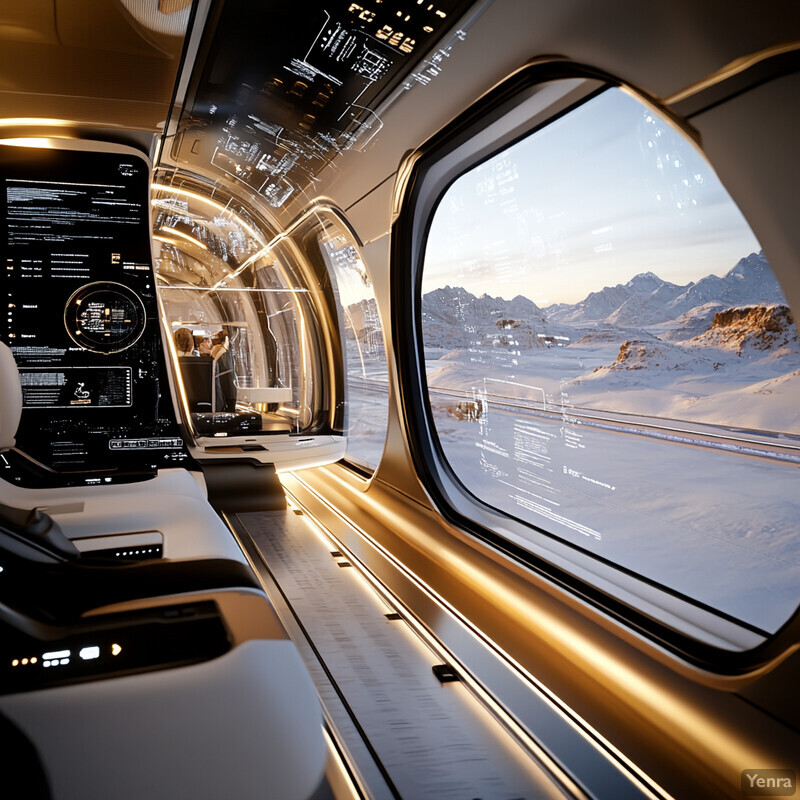
Cutting-edge AI-driven forecasting illustrates this capability. For example, NOAA has developed an AI weather model that predicts storms 10× faster than traditional algorithms, allowing rapid response (e.g. cancel or reroute trips before a storm). Google’s “Aurora” AI model (2025) outperformed conventional forecasts in 92% of high-resolution weather tests and predicted cyclones perfectly. Such advances mean hyperloop operators could use AI forecasts to adjust schedules in real time. Moreover, AI models are being used for real-time air-quality and seismology data analysis in transit systems. While specific hyperloop trials are not yet public, these examples show AI can accurately model environmental dynamics. Thus, the technology exists to allow hyperloop control systems to adapt in real time to changing environment for optimal operation.
15. Data-Driven Design Validation
AI uses operational and test data to validate and refine Hyperloop designs. Sensor measurements from prototypes and simulations feed back into the design loop. AI algorithms detect discrepancies between predicted and real-world performance. They can also recommend design tweaks based on data trends (e.g. stiffen a pod chassis if strain data is high). This continuous feedback ensures designs meet safety margins under actual conditions. In short, AI leverages data (sim, flight tests, sensor logs) to confirm and improve design assumptions.
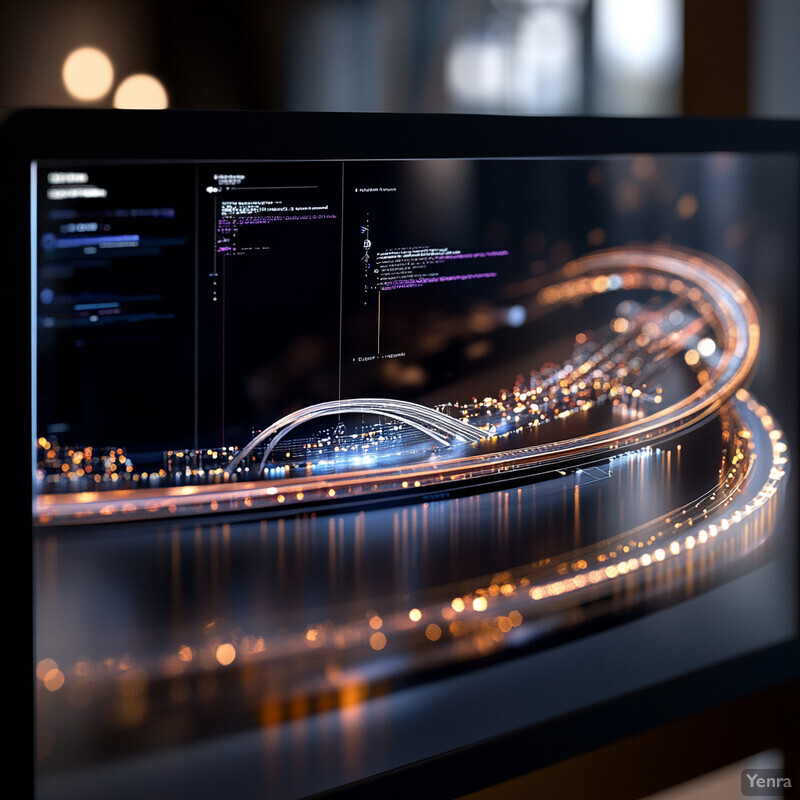
Data-driven validation is exemplified in recent research. In the deep materials study, 736 of the AI-predicted new materials were confirmed by experiment, showing the predictions held up in practice. Similarly, AI models in CFD are often validated against wind-tunnel data; e.g., an ANN surrogate’s predicted drag closely matched validated CFD results in the UAV airfoil study. For hyperloop, one would expect iterative cycles: simulation with AI models followed by physical tests. Although specific hyperloop design validations using AI are not yet published, these analogous cases (airfoil CFD validation, materials discovery) demonstrate that AI-generated designs can and must be checked against real data, with AI tools smoothing the iteration by quickly identifying model errors. This evidence supports that a data-driven approach (incorporating AI) can improve design accuracy over time.