1. Automated Image Restoration
Machine learning models can remove scratches, fading, and other forms of degradation from historical photographs, paintings, and manuscripts, preserving their original appearance for future generations.
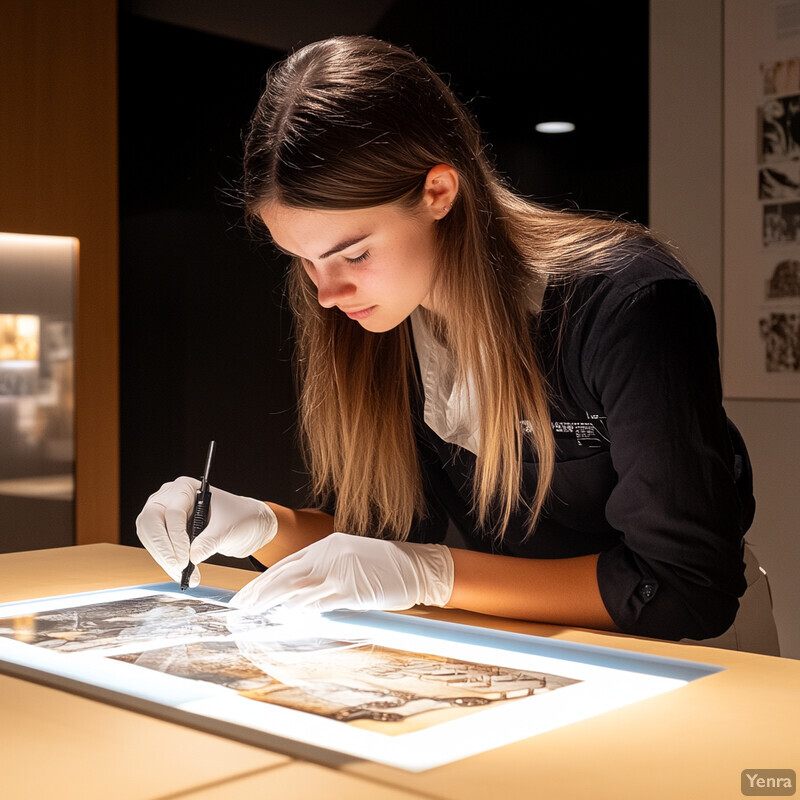
Modern machine learning models employ convolutional neural networks trained on vast datasets of historical and contemporary images to remove damage such as cracks, scratches, stains, and fading from old photographs, manuscripts, and artworks. By learning the patterns of degradation and comparing them to reference images, AI can reconstruct missing details with remarkable precision. This automated process drastically reduces manual labor and subjectivity, allowing conservators to focus on decision-making rather than painstaking retouching. The result is not only a more faithful representation of the original artifact but also a less destructive restoration method that respects the integrity of the cultural heritage object.
2. Colorization of Black-and-White Media
Neural networks trained on extensive image databases can accurately apply realistic colors to old photographs and film footage, providing a more immersive and relatable understanding of the past.
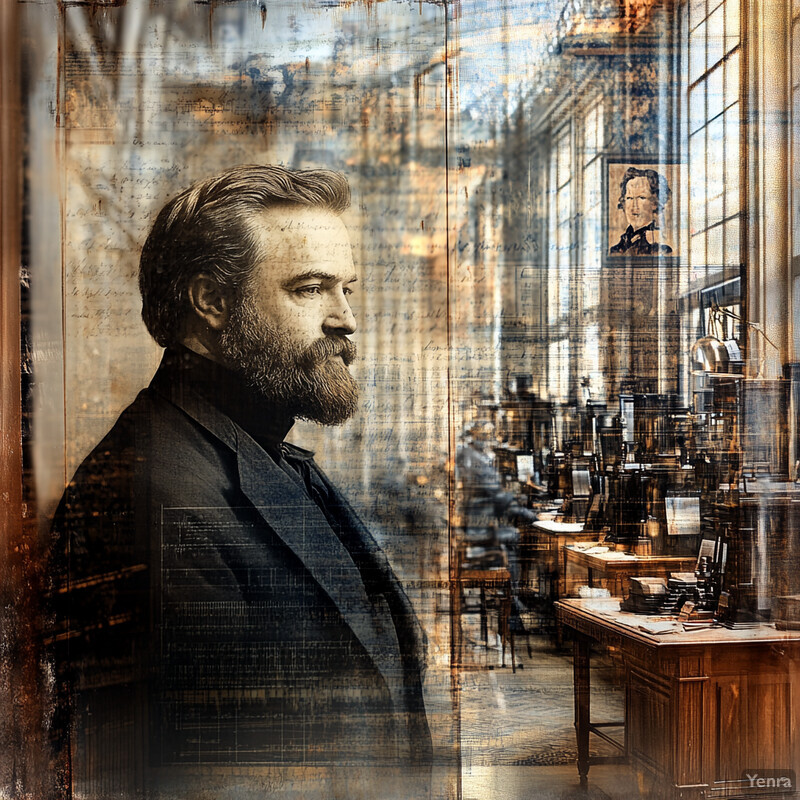
Using deep learning models, AI can convincingly add color to archival black-and-white photographs and film, bridging the gap between the present and the past. Neural networks trained on large color image databases learn to identify objects, materials, and environmental cues that inform realistic color application. These systems produce colorizations that are historically plausible and aesthetically coherent, making old media more accessible and engaging. Historians and curators can use such tools to better understand historical contexts—such as clothing dyes, architectural paints, and natural scenery—while the general public gains a richer and more relatable view of the past.
3. 3D Reconstruction of Ruins and Artifacts
AI-driven photogrammetry and computer vision techniques convert fragmented ruins, incomplete maps, and damaged sculptures into detailed 3D models, helping researchers and restorers visualize structures and artifacts as they once were.
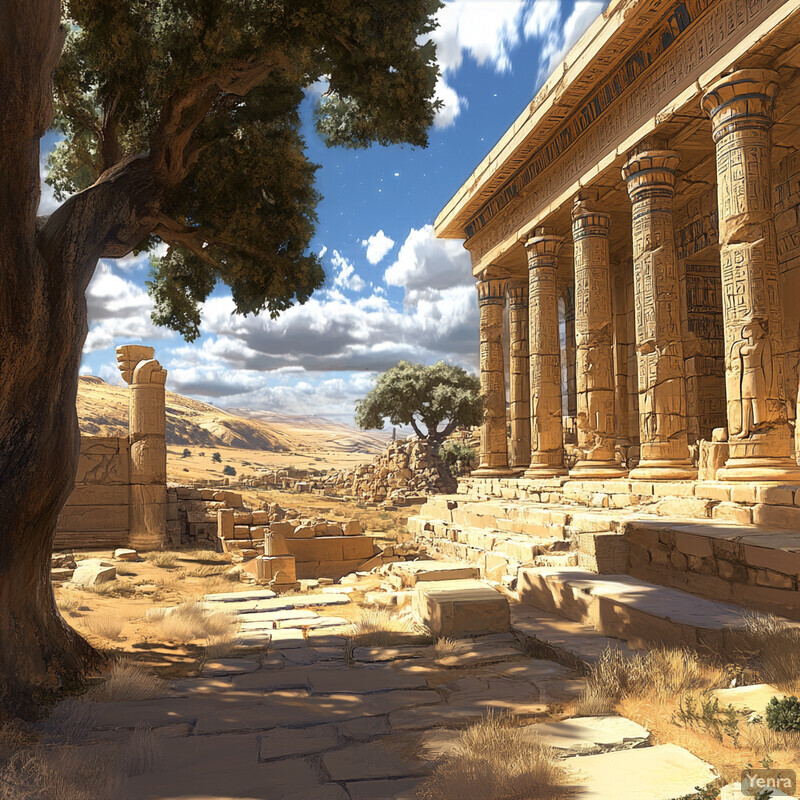
Computer vision and photogrammetry algorithms enable AI to generate detailed three-dimensional models from photographs, scans, and partial datasets, allowing experts to virtually reassemble fragmented ruins, broken statuary, and damaged architectural elements. These reconstructions help historians, archaeologists, and restorers visualize lost structures and compare modern conditions to original states. By adjusting lighting, scaling, and perspective, these models also support immersive museum exhibits, digital archives, and scholarly research, transforming static fragments of the past into dynamic, interactive learning experiences.
4. Damage Prediction and Preservation Modeling
Machine learning algorithms can predict how environmental factors—such as humidity, temperature changes, and pollution—will affect artifacts or structures over time, guiding conservation strategies.
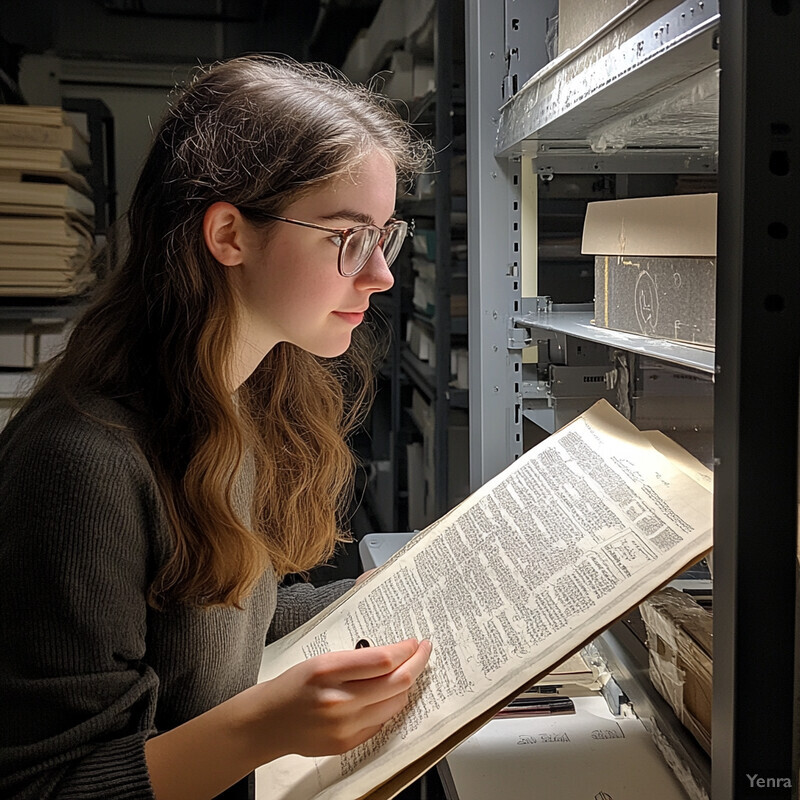
AI can forecast the long-term effects of environmental factors such as temperature fluctuations, humidity levels, airborne pollutants, and light exposure on historical objects and structures. Advanced predictive models identify patterns of wear and tear and simulate future conditions, guiding conservators on preventive measures. For example, museums can use these predictions to select the best materials for protective enclosures, determine optimal storage or display conditions, and schedule maintenance before significant damage occurs. This proactive approach helps extend the lifespan of cultural heritage artifacts, ensuring their survival for future generations.
5. Optical Character Recognition (OCR) on Historical Texts
Deep learning-based OCR can accurately transcribe faded, handwritten, or stylized texts found in old documents, books, and manuscripts, enabling easier indexing and research of historical archives.
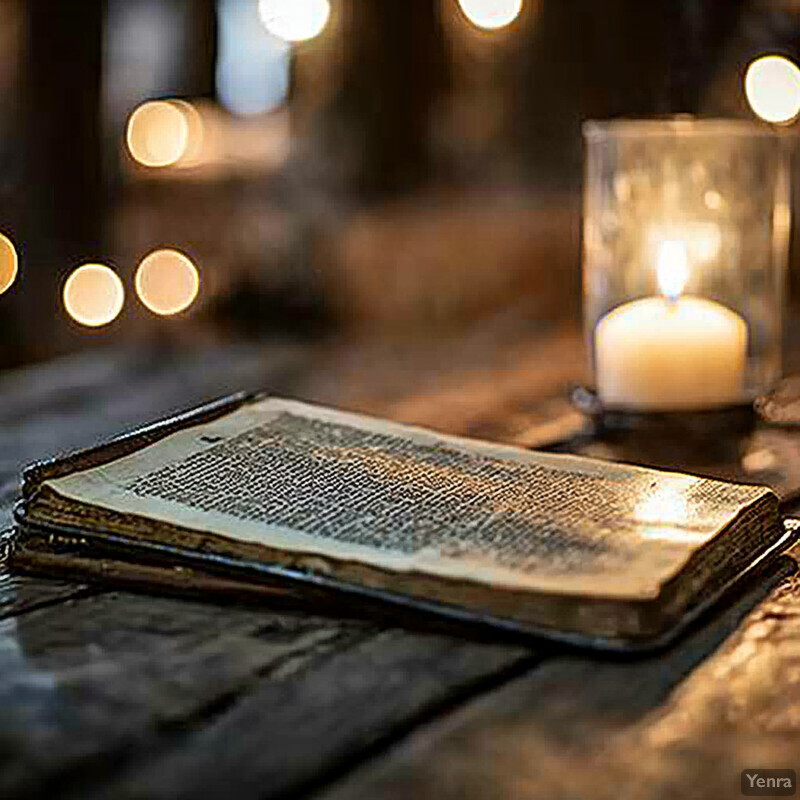
Deep learning-based OCR systems can read and transcribe a wide range of historical texts—many of which are handwritten, faded, or composed in unusual typefaces. By converting scanned pages into machine-readable text, AI removes a significant barrier to accessing and analyzing historical documents. Once digitized, these materials become searchable and indexable, facilitating scholarly work, enhancing metadata creation, and allowing large-scale comparative studies. Researchers can more quickly identify relevant sources, uncover hidden references, and piece together fragmented historical narratives that would be challenging to access through manual reading alone.
6. Natural Language Processing for Historical Context Analysis
NLP models can understand archaic or extinct languages, old dialects, and stylistic conventions, thereby uncovering nuances in historical documents that might otherwise remain misunderstood or overlooked.
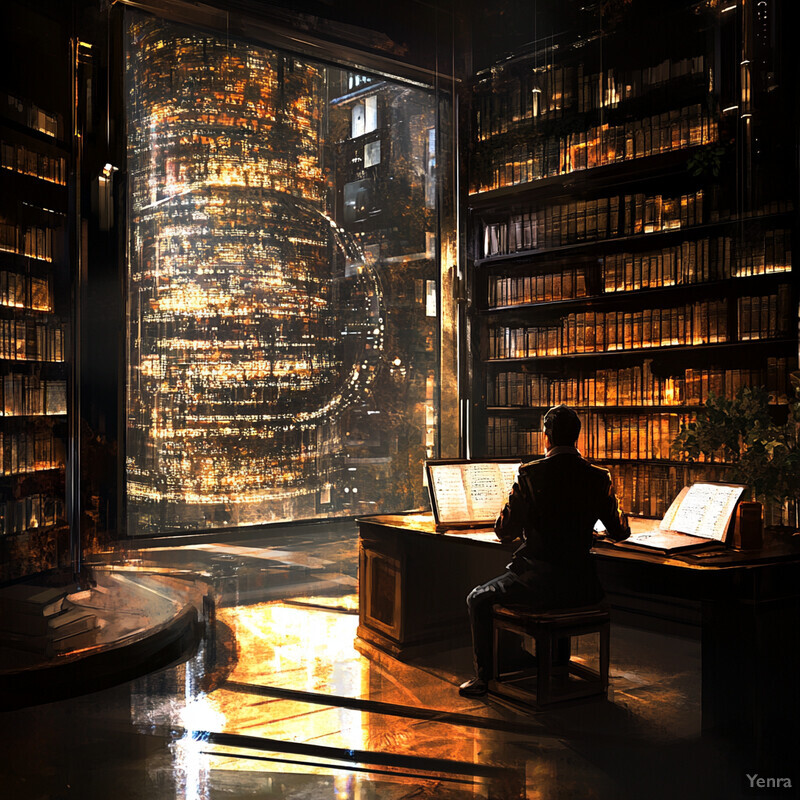
NLP tools are increasingly adept at handling archaic languages, obsolete spellings, and old idiomatic expressions. By training models on historical corpora, AI can interpret documents within their original cultural and linguistic contexts. This includes understanding shifts in meaning over time, recognizing lost vernaculars, or even detecting subtle political, religious, or social nuances in texts. Historians benefit by gaining deeper insights into primary sources, as the enhanced textual understanding supports more accurate interpretations of events, motivations, and social dynamics that shaped the past.
7. Automated Metadata Generation and Archiving
AI tools can scan large collections of documents, images, and artifacts to generate rich metadata—dates, locations, authors, and topics—improving archival organization and searchability.
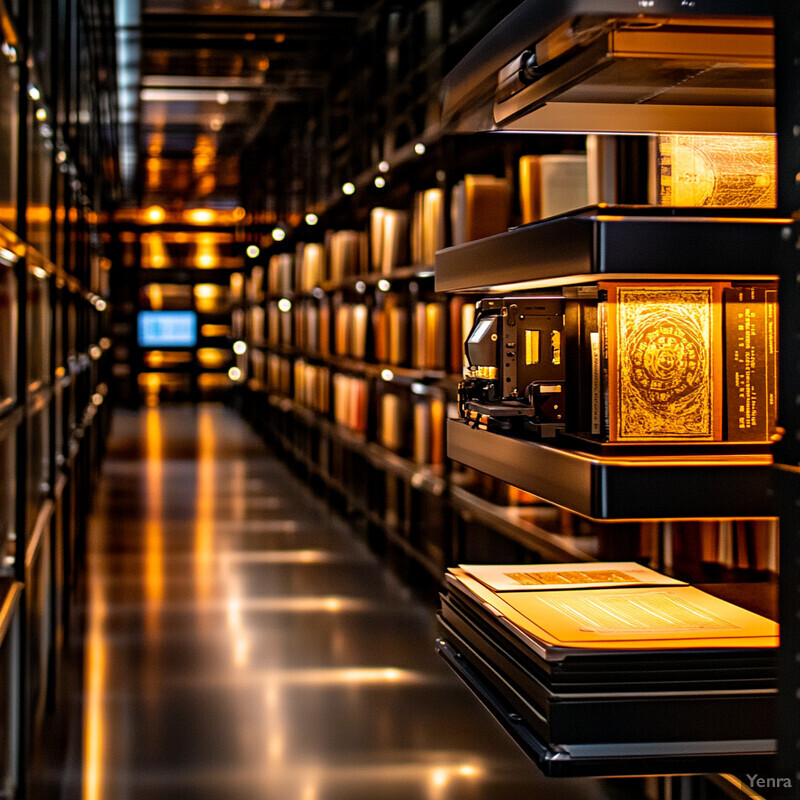
AI-driven classifiers and clustering algorithms can sift through massive collections of historical documents, photographs, and videos to generate rich, standardized metadata. By tagging items with meaningful attributes—such as date, location, author, subject matter, and related themes—AI streamlines the archiving process. This automated categorization improves the organization, discoverability, and accessibility of archival materials. In turn, researchers, curators, and educators can efficiently navigate vast repositories, quickly finding the exact documents or objects needed for their work.
8. Pattern Detection in Historical Data
Advanced algorithms can find correlations and patterns in vast historical records, such as trade data, census reports, or migration patterns, offering novel insights into social, economic, and cultural transformations over time.
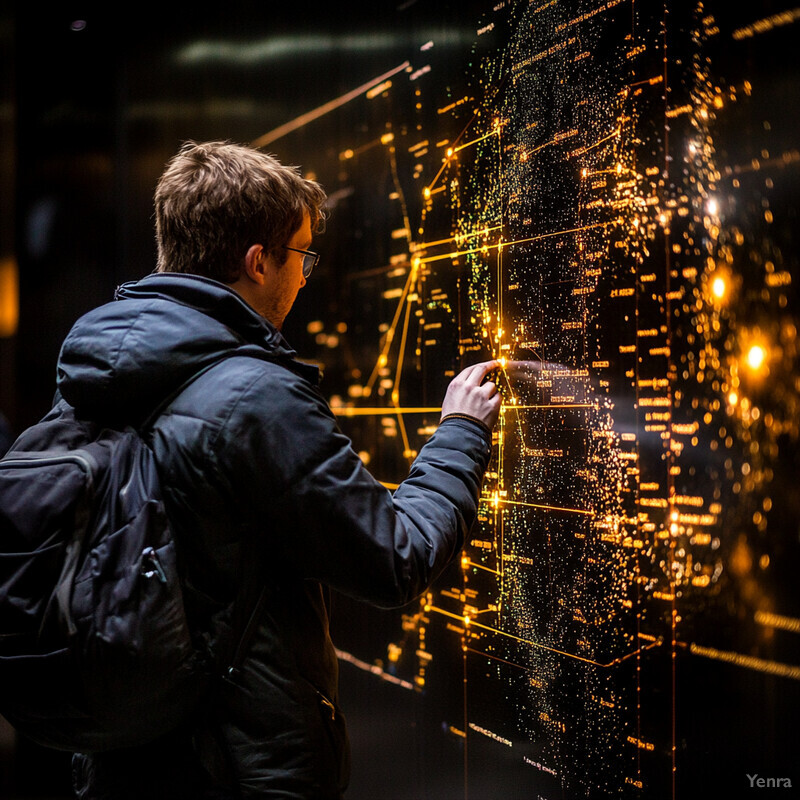
Machine learning models can process and analyze massive sets of historical records—ranging from census data to trade logs and political events—to identify previously undetected correlations and trends. For instance, AI might reveal how climate shifts influenced migration patterns, or show the relationship between economic policies and changes in living standards across centuries. These patterns, often invisible to the naked eye, allow historians and social scientists to generate new hypotheses, refine historical narratives, and enrich our understanding of complex societal transformations.
9. 3D Printing Integration with AI Models
AI-assisted modeling can lead directly to accurate 3D-printed replicas of historical objects or structural elements, helping museums and educational institutions present tactile learning experiences.
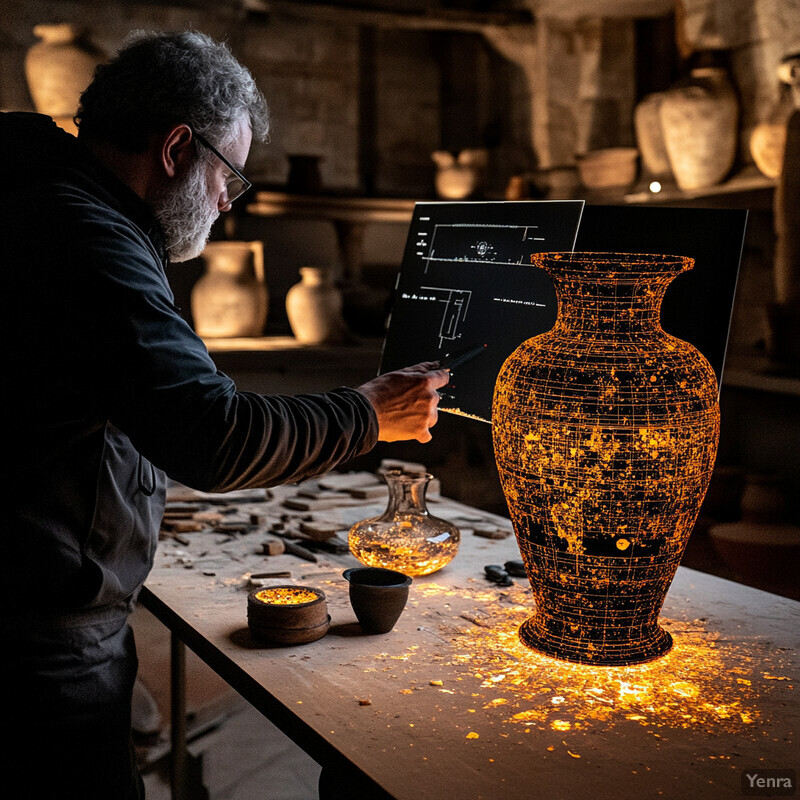
When AI-generated 3D reconstructions are paired with advanced additive manufacturing technologies, museums and research institutions can produce highly accurate replicas of artifacts and structures. These copies serve various purposes: they enable handling by students and visitors, reducing wear on the original piece; they can fill gaps in exhibitions where genuine artifacts are too fragile or rare; and they support hands-on educational activities that bring historical study to life. By leveraging AI’s reconstruction capabilities and modern manufacturing, cultural heritage becomes more accessible and experiential.
10. Style Transfer for Artistic Reconstruction
AI style transfer models can infer missing sections of art or architecture, guiding restorers in selecting materials, patterns, and color schemes that align with the original creator’s style.
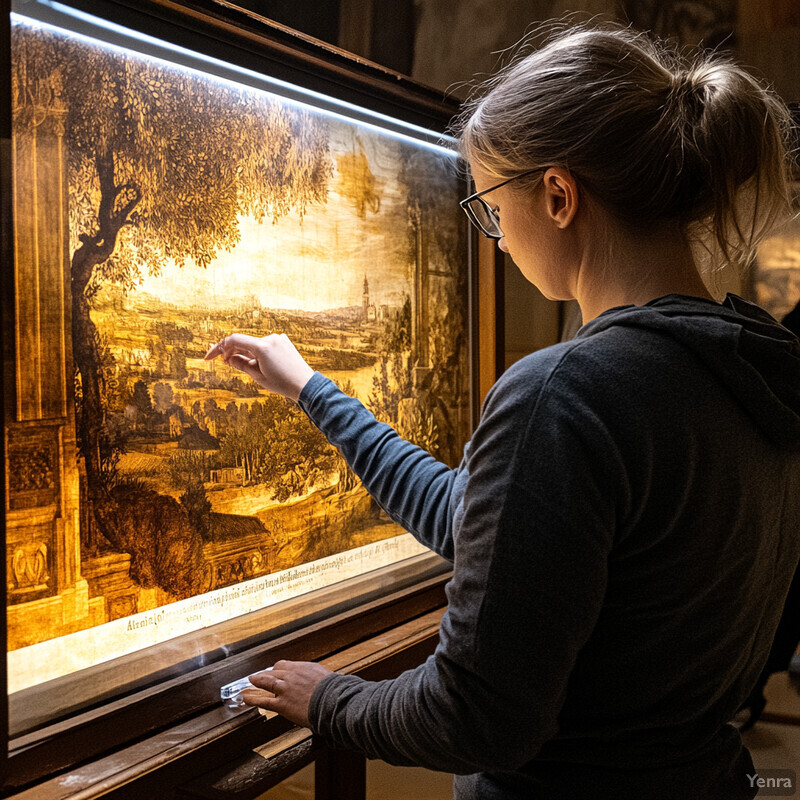
Style transfer algorithms trained on historical artworks can help restorers fill in missing details or reconstruct lost sections of a painting, tapestry, or architectural decoration. By understanding the stylistic rules—brushstrokes, patterns, color palettes—that governed the original creator’s work, AI suggests visually harmonious restorations. This approach aids human conservators by providing references that blend naturally with the authentic portions of the piece, ensuring that restorations are faithful to the historical context and aesthetic principles rather than introducing anachronistic styles or guesswork.
11. Enhancement of Audio Recordings and Film
Machine learning can clean up historical audio recordings—removing noise, clarifying speech—and restore old film reels by stabilizing frames and improving image quality, preserving important cultural moments.
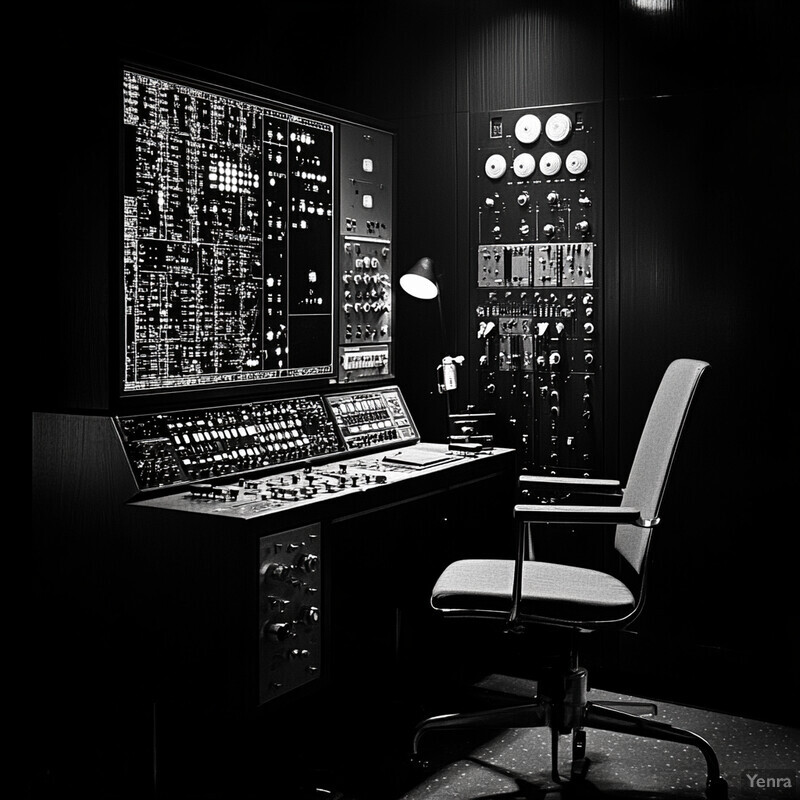
Old audio and film materials often suffer from degradation—crackling audio, muffled voices, grainy images, and unstable frames. AI-driven restoration techniques can remove background noise, enhance speech intelligibility, stabilize shaky footage, sharpen blurry frames, and even upscale resolutions. By improving the quality of these historical media formats, AI brings voices, stories, and events from the past back to life. This quality enhancement not only benefits researchers studying cultural or political events but also enriches public exhibitions, documentaries, and educational content.
12. Virtual Reality (VR) and Augmented Reality (AR) Experiences
AI-powered VR/AR reconstructions can bring ancient sites and historical events to life, allowing researchers and the public to “step into” lost worlds and interact with history in an immersive manner.
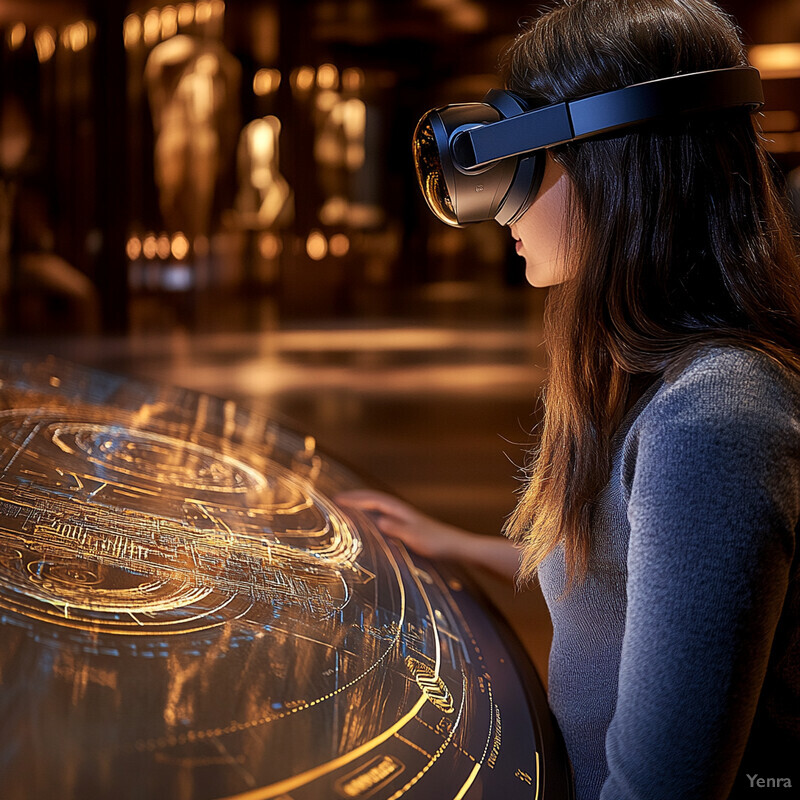
AI enables the creation of highly realistic and contextually accurate VR and AR environments by combining digital reconstructions, historical data, and machine learning insights. Users can virtually “enter” ancient buildings, witness historical events, or navigate reconstructed streetscapes as they would have appeared centuries ago. Historians, educators, and museum-goers gain immersive and interactive tools for understanding history in a visceral way. Such experiences break down barriers of time and geography, making history more engaging and allowing learners to grasp complex concepts through experiential learning.
13. Machine Translation of Ancient Texts
AI models trained on multilingual corpora can propose translations for texts written in rare or ancient languages, breaking down language barriers and expanding global access to historical knowledge.
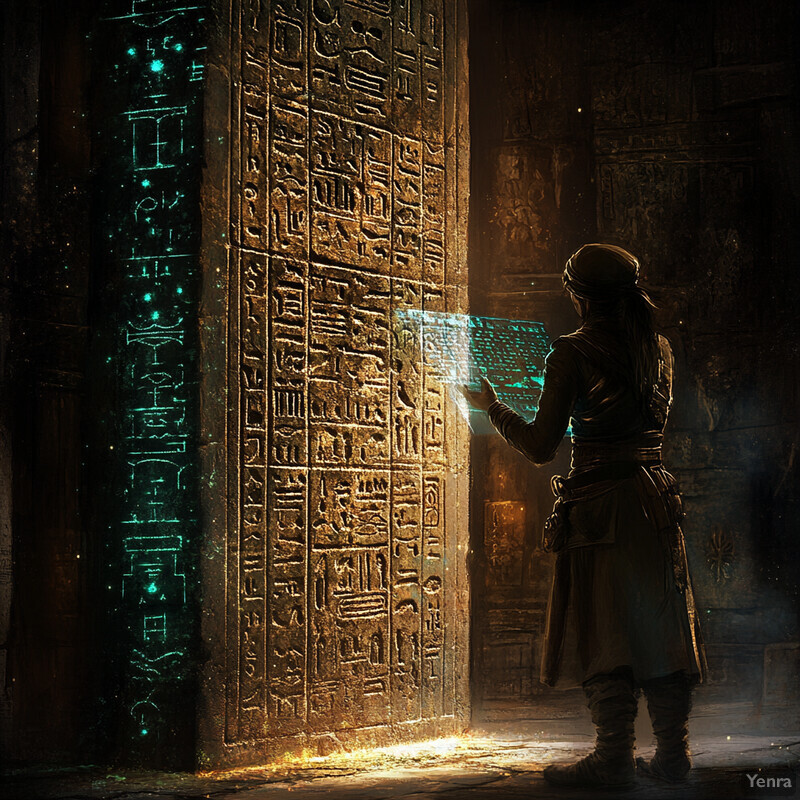
By using neural machine translation systems trained on parallel texts and linguistic datasets, AI can offer preliminary translations of documents in ancient or obscure languages. This opens up opportunities to explore primary sources previously locked behind linguistic barriers. Although human experts still refine and validate these translations, AI-driven translation significantly accelerates the initial stage of understanding. It broadens global access to rare manuscripts, inscriptions, and historical works that would otherwise remain restricted to a handful of specialized scholars.
14. Facial Recognition for Historical Figures
By comparing portraits, photographs, and descriptions, AI can help identify historical figures in unlabeled images or confirm their presence at specific events, enhancing biographical research.
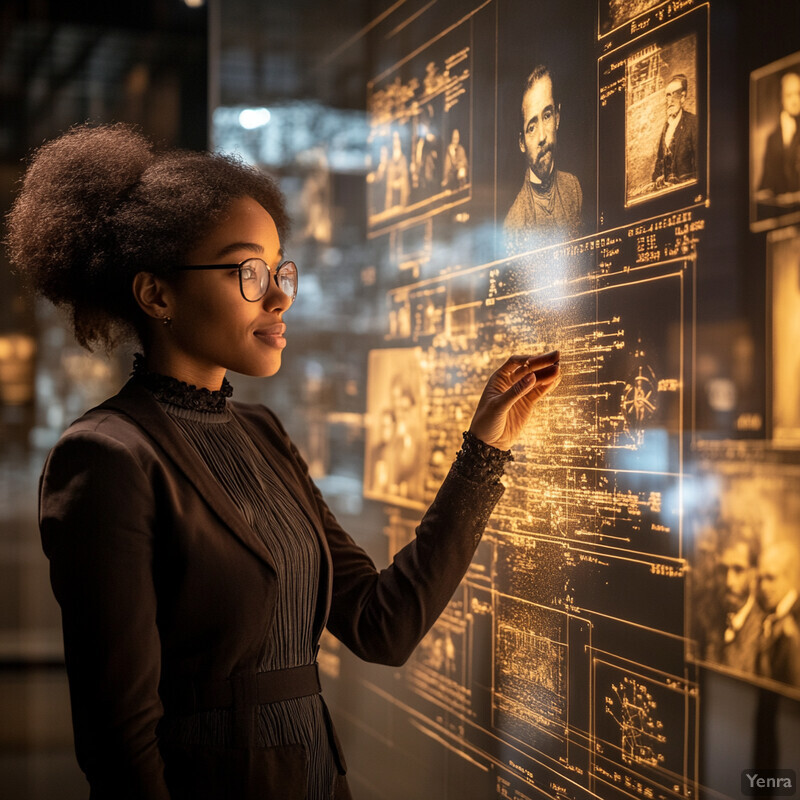
AI-based facial recognition software can match faces across portraits, photographs, sculptures, and coinage, linking depictions of historical figures to their known identities. This helps confirm or refute the presence of specific individuals in archival images, determine the chronology of their portraits, or reveal their appearances in newly discovered media. By providing visual anchors to historical narratives, researchers can strengthen biographical details, contextualize important meetings or gatherings, and contribute to a richer understanding of social networks in the past.
15. Provenance Tracing of Artifacts
ML algorithms can detect stylistic elements, materials, and techniques to help confirm the provenance of historical objects, reducing forgery and helping establish authenticity.
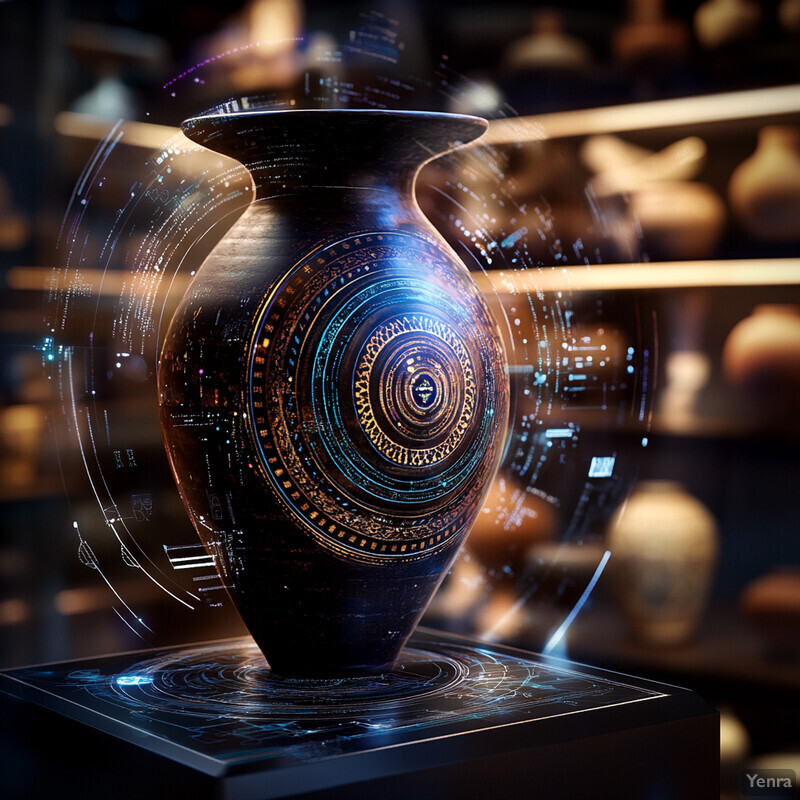
AI algorithms can analyze stylistic patterns, materials, and manufacturing techniques to help authenticate artifacts and trace their origins. By comparing an object’s characteristics with large databases of known works, these tools support curators and art historians in confirming authenticity, identifying workshops or schools of craftsmanship, and detecting forgeries. Strengthening the chain of provenance builds trust in museum collections and the art market, ensuring that cultural heritage is preserved accurately and ethically.
16. Predictive Modeling for Archaeological Prospecting
AI can analyze topographical and geological data to predict where undiscovered archaeological sites might be located, guiding more efficient and targeted excavation efforts.
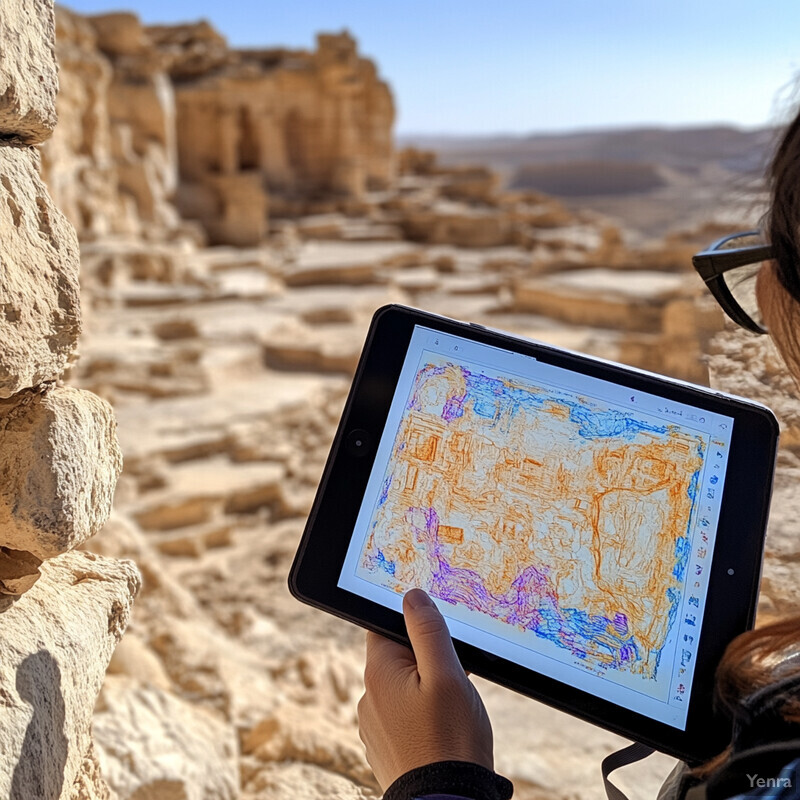
Archaeologists can use AI to process geological, environmental, and historical data—such as satellite imagery, terrain maps, and climate records—to predict where undiscovered archaeological sites might lie. By identifying promising areas, machine learning reduces time-consuming manual surveys and costly excavations that yield no finds. This approach helps target excavations more precisely, preserving delicate landscapes and focusing resources on locations with a higher probability of uncovering valuable historical information.
17. Temporal Data Analysis in Chronological Studies
Deep learning models can help pinpoint the sequence and timelines of events in complex historical narratives, making it easier to understand causes and effects across eras.
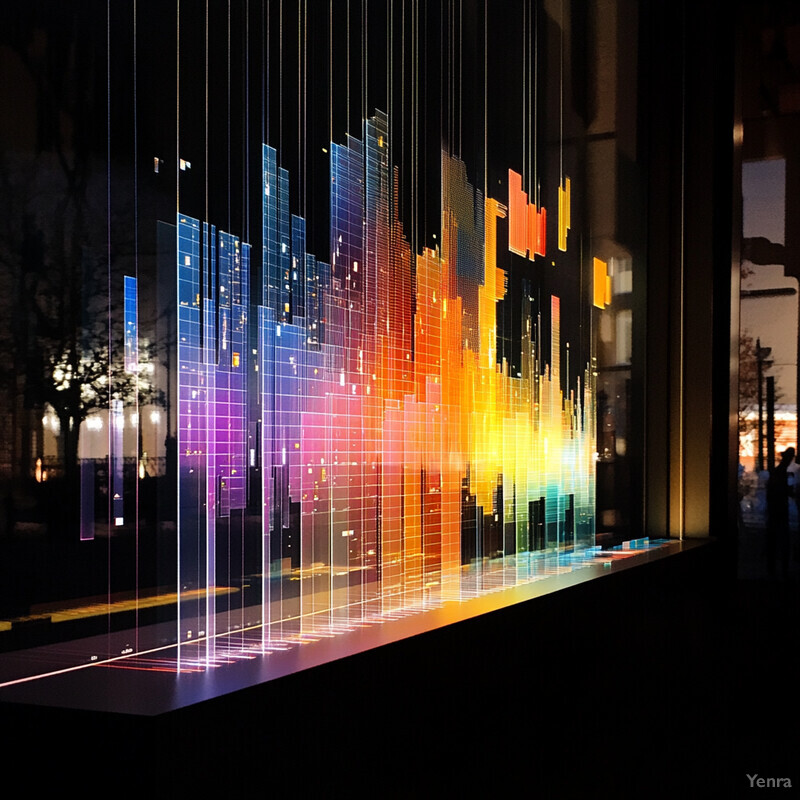
AI tools can assist historians in establishing more accurate and detailed timelines of events, cultural shifts, and technological progressions. By analyzing textual data, inscriptions, carbon dating results, and even styles of material culture, AI models can infer chronological sequences and detect anomalies. These insights help scholars align events across different regions, correlate historical records from multiple sources, and resolve debates around the timing or order of significant occurrences, ultimately refining our understanding of historical cause and effect.
18. Climate and Environmental Context Reconstruction
By modeling climate patterns and ecological data, AI can help historians and archaeologists understand how environmental conditions influenced historical migrations, trade routes, or collapses of civilizations.
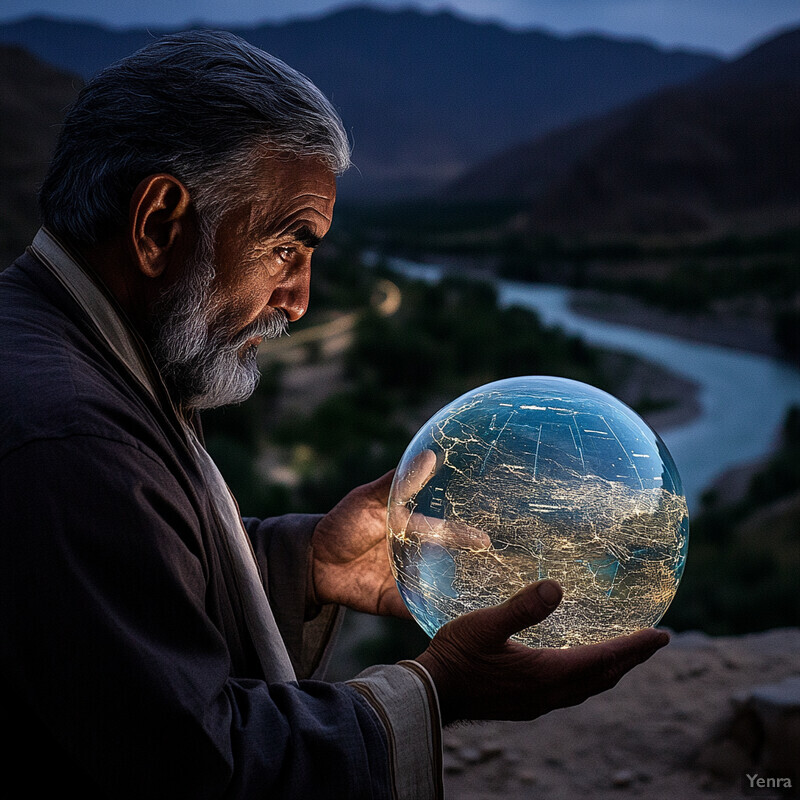
Through environmental modeling and analysis of historical data, AI can reconstruct past climate conditions and correlate them with historical developments. For example, it can show how droughts influenced migration routes, how volcanic eruptions impacted crop yields and trade, or how periods of warming or cooling shaped settlement patterns. By integrating paleoenvironmental data, archeological findings, and historical texts, AI helps researchers appreciate the intimate relationship between human societies and their natural surroundings, revealing how changes in climate and environment have shaped the course of human history.
19. Pattern Recognition in Iconography and Symbolism
Computer vision can analyze recurring symbols, motifs, and patterns in ancient art, inscriptions, and artifacts to reveal the spread of cultural influences and religious beliefs over time.
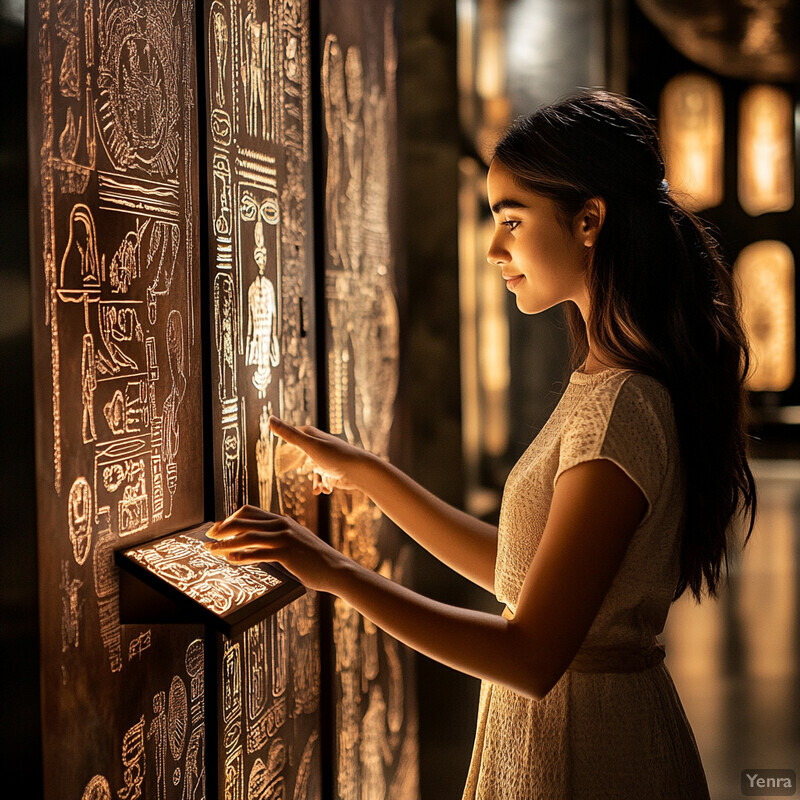
Computer vision can analyze patterns in ancient iconography, inscriptions, motifs, and religious symbols, identifying stylistic influences and tracing the diffusion of ideas across regions and time. This automated pattern recognition helps historians classify cultural artifacts, understand the significance of recurring symbols, and discover connections among distant civilizations. It can also highlight how religious or political iconography evolved over centuries, offering a nuanced interpretation of cultural identity, belief systems, and socio-political messages conveyed through art.
20. Digital Reassembly of Fragmented Texts and Objects
When documents or artifacts are fragmented into pieces, AI can intelligently match and piece them together digitally, reducing guesswork and accelerating the reassembly process for historians and curators.
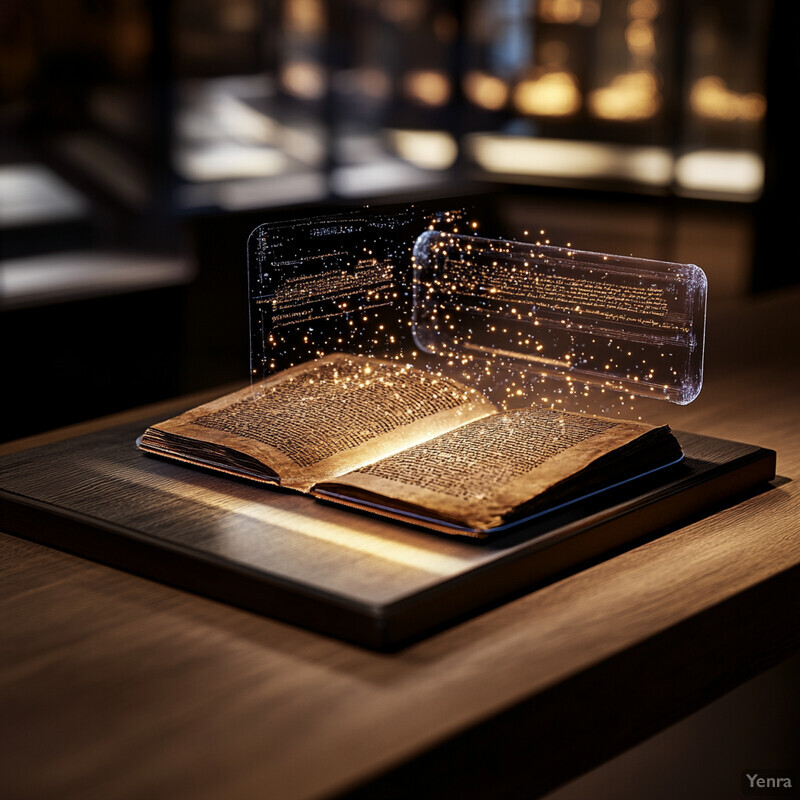
AI algorithms can match and align scattered fragments of manuscripts, pottery, murals, and other artifacts that have been lost or broken over time. By comparing the shapes of fragment edges, the texture of materials, and the continuity of patterns or texts, machine learning can suggest how pieces fit together. This digital puzzle-solving spares scholars guesswork and manual trial-and-error. Once reassembled virtually, the full artifact or document often reveals previously hidden information, clarifying historical narratives and providing more comprehensive insights into the past.