1. Real-Time Sensor Data Analysis
AI algorithms process large volumes of vibration, acceleration, and pressure data from track and train-mounted sensors in real time, identifying subtle anomalies that may precede a fault.
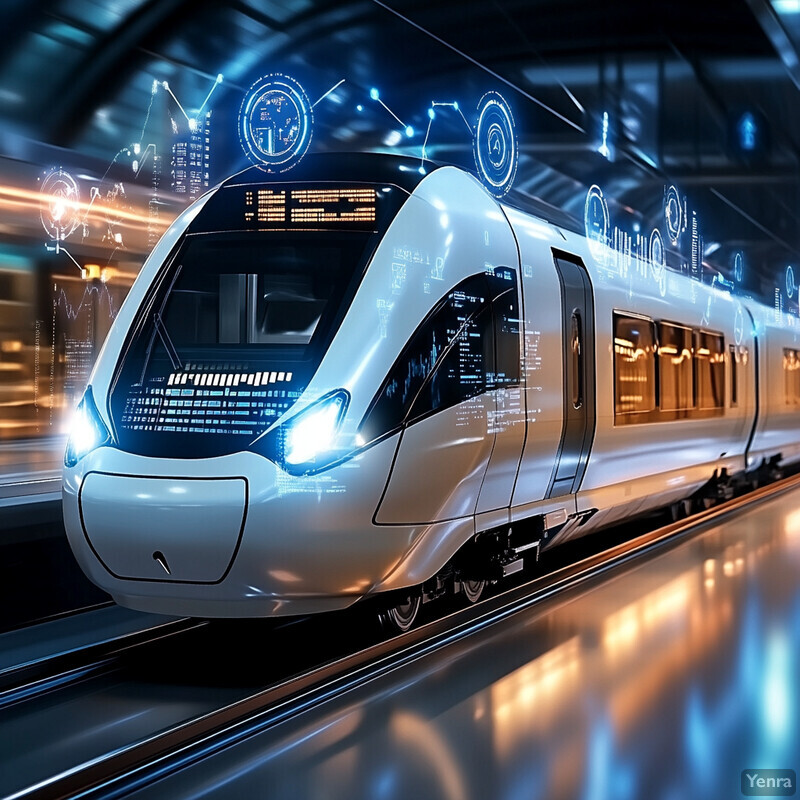
High-speed rail systems generate vast amounts of data from onboard sensors attached to railcars, tracks, and various mechanical components. AI-powered analytical engines continuously process this data stream, looking for patterns that deviate from normal operational baselines. As sensors capture subtle changes in vibration, acceleration, pressure, and structural integrity, AI models can quickly identify early warning signs of component degradation or track irregularities. By alerting maintenance teams as soon as anomalies arise, this real-time analysis shortens response times, reduces downtime, and prevents minor issues from escalating into critical failures that could impact safety and service reliability.
2. Predictive Maintenance Scheduling
Machine learning models forecast when critical components—such as wheels, rails, and overhead cables—are likely to fail, allowing for proactive maintenance rather than reactive repairs.
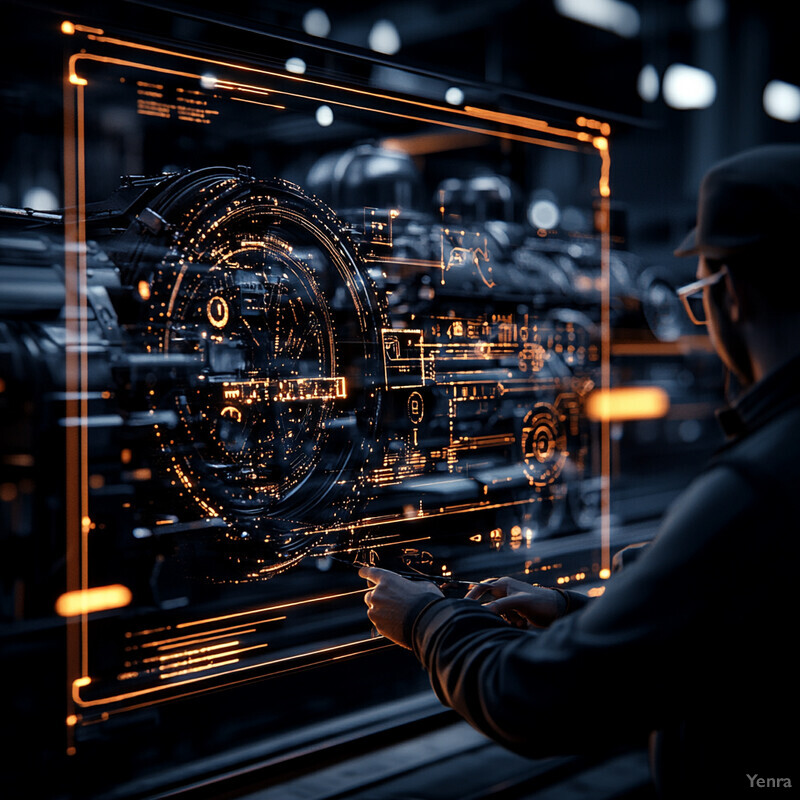
Traditional maintenance in rail systems often relies on fixed schedules or manual assessments, which can be both costly and inefficient. AI-driven predictive maintenance models leverage historical data, environmental factors, and ongoing sensor inputs to forecast when parts are likely to fail. Instead of performing routine checks at fixed intervals, operators can focus on critical components at exactly the right time. This approach optimizes resource allocation, reduces unnecessary inspections, and minimizes the risk of catastrophic breakdowns. By predicting component lifespans accurately, AI helps operators maintain a seamless travel experience, bolster passenger safety, and prolong the life of expensive railway assets.
3. Computer Vision for Track Inspections
Deep learning–based image recognition systems analyze high-resolution video footage of tracks, sleepers, and switches, automatically detecting cracks, misalignments, or foreign objects faster and more accurately than manual inspections.
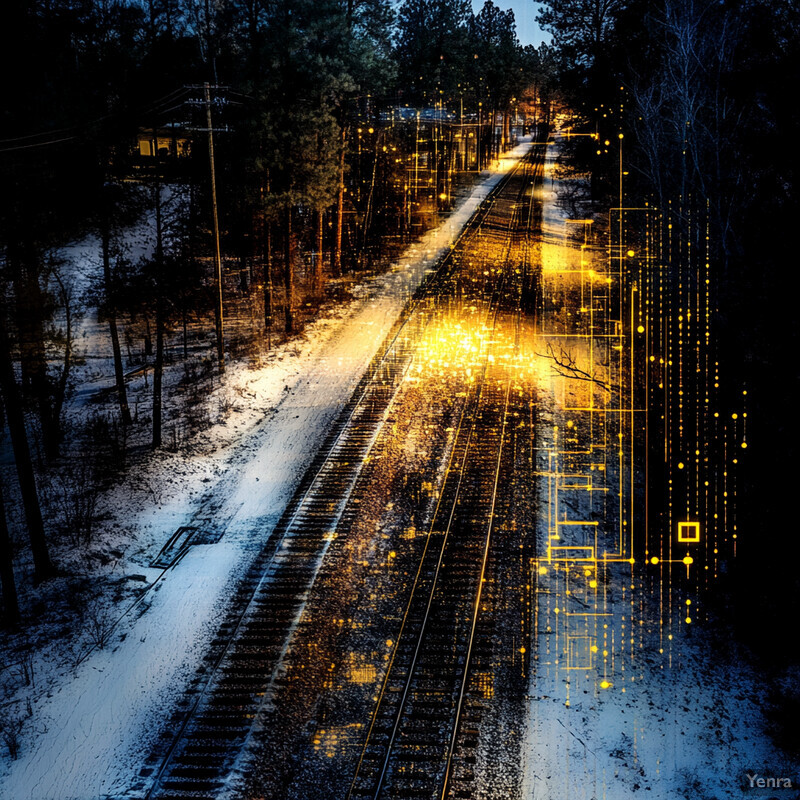
Traditional track inspections involve human inspectors traveling along lines, checking for defects by eye. AI-enhanced computer vision automates this labor-intensive process. High-resolution cameras mounted on rail vehicles or drones capture continuous video streams of rails, sleepers, and other track elements. Deep learning models are then trained to detect cracks, loose fasteners, track misalignments, and foreign objects. By analyzing thousands of images per second, these systems identify potential issues far more quickly and precisely than manual inspections, enabling timely repairs. As a result, computer vision reduces labor costs, shortens inspection times, and enhances the overall safety and quality of the infrastructure.
4. Thermal Imaging Fault Detection
Infrared cameras integrated with AI frameworks detect abnormal temperature variations in components like bearings, brakes, and electrical systems, identifying early signs of wear or potential fire hazards.
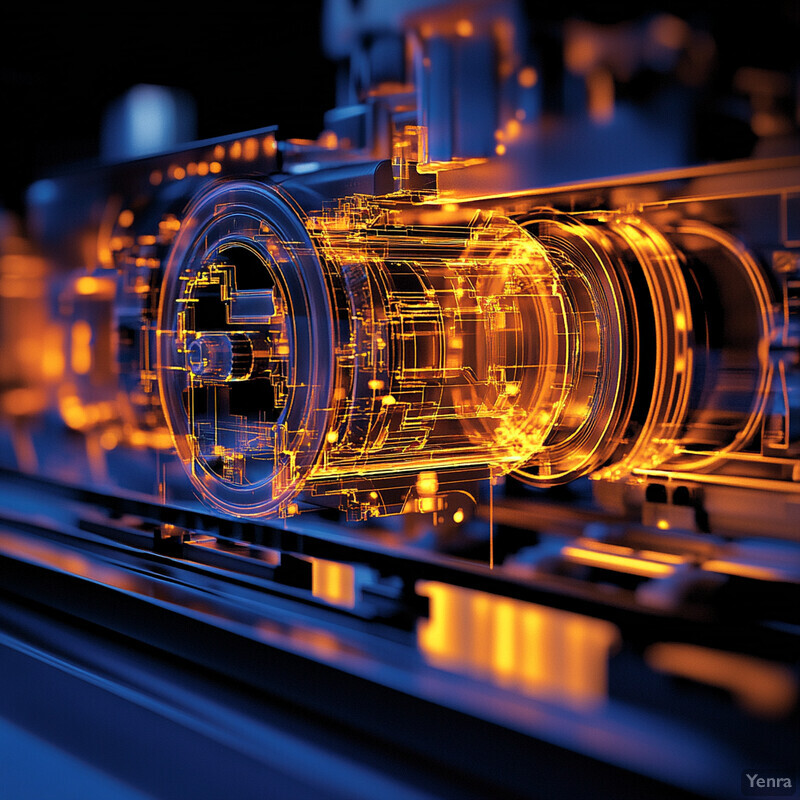
Heat signatures can reveal early signs of mechanical stress or electrical faults, often before visible damage occurs. By integrating infrared (IR) cameras with AI, high-speed rail operators can continuously monitor temperature patterns in bearings, brakes, overhead cables, and other components. When the AI detects abnormal heat levels—indicating friction, insulation failures, or metal fatigue—it alerts maintenance teams to investigate. This proactive approach mitigates the risk of severe malfunctions and catastrophic fires. Ultimately, the fusion of thermal imaging and AI not only enhances fault detection accuracy but also helps preserve critical infrastructure and maintain smooth, safe train operations.
5. Acoustic and Vibration Signal Analysis
AI-driven signal processing identifies changes in the acoustic signatures of rails, wheels, and mechanical parts, enabling early detection of material fatigue, loosened fasteners, or wheel flats.
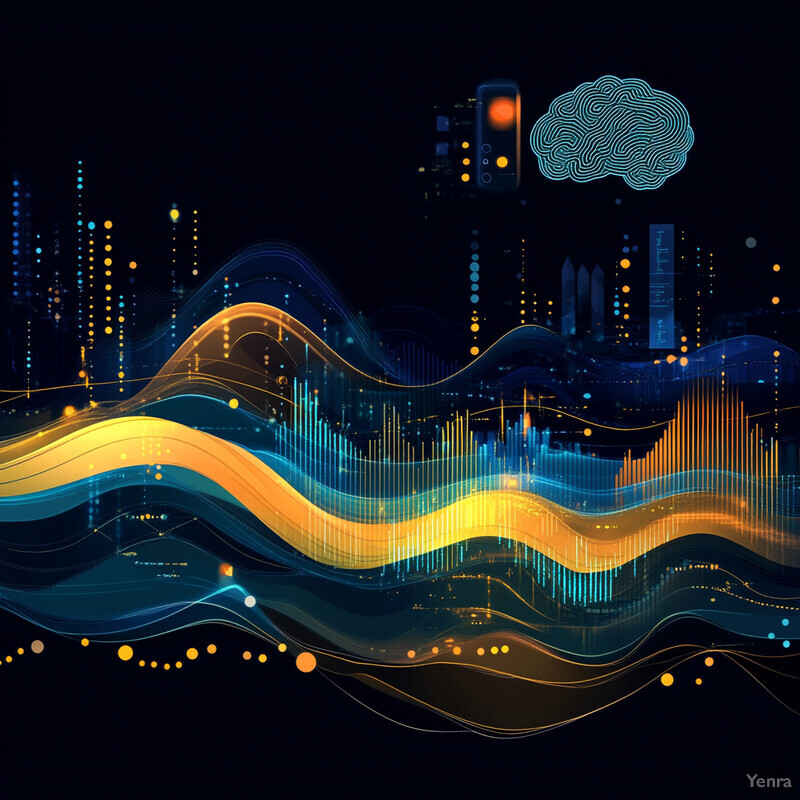
Each rotating axle, wheel assembly, and track segment produces unique acoustic and vibration signatures. AI algorithms trained on these baseline signatures can discern minute deviations that hint at hidden defects. Using advanced signal processing techniques, the AI pinpoints issues like wheel flats, loosened fasteners, or microscopic cracks. Unlike human technicians who might overlook subtle variations, AI’s data-driven methods consistently detect problems at their nascent stage. By identifying early changes in vibration or sound patterns, rail operators can schedule maintenance before serious damage occurs, ensuring that trains run smoothly, quietly, and efficiently across the network.
6. Condition Monitoring of Overhead Lines
AI algorithms analyze drone or camera footage of overhead contact lines, pantographs, and insulators, ensuring minor defects are caught before they cause major service disruptions.
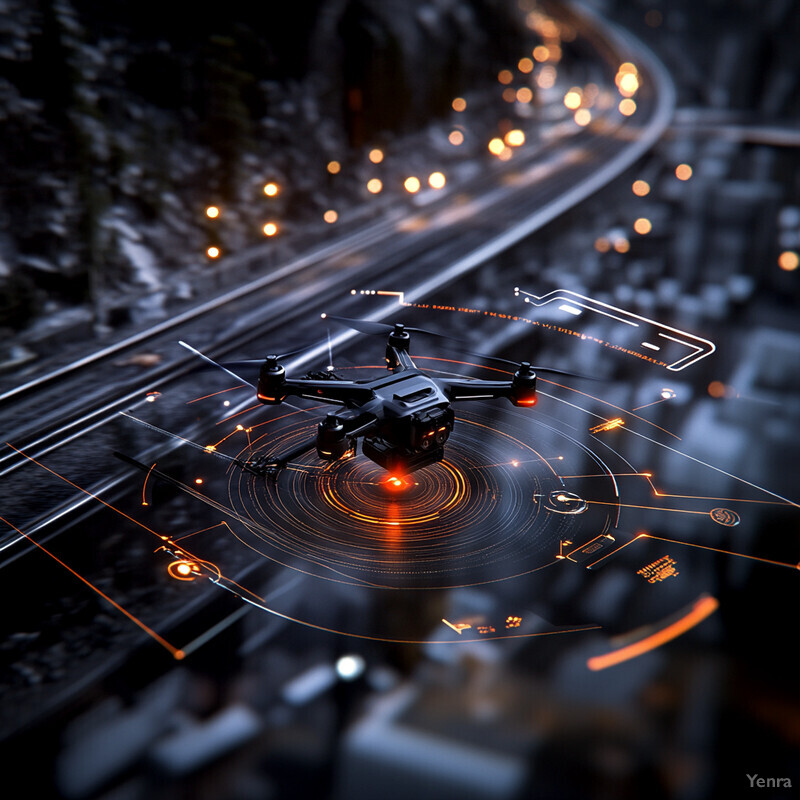
Overhead contact lines (catenaries), pantographs, and insulators are critical to delivering stable electrical power to high-speed trains. Inspecting these components traditionally requires manual checks and track closures. AI-powered condition monitoring uses high-resolution imagery captured by drones, trackside cameras, or specialized rail vehicles to detect worn components, slack wires, or debris. Machine learning models analyze patterns and compare them to known standards, flagging any anomalies. By enabling regular and automated checks, operators can prevent sudden power losses, reduce downtime, and optimize maintenance intervals—all while minimizing human exposure to high-risk environments.
7. On-Board Edge Computing for Fault Diagnosis
Integrating AI on trains themselves enables on-the-spot fault detection without needing to send raw data to central servers, reducing latency and improving the speed of safety responses.
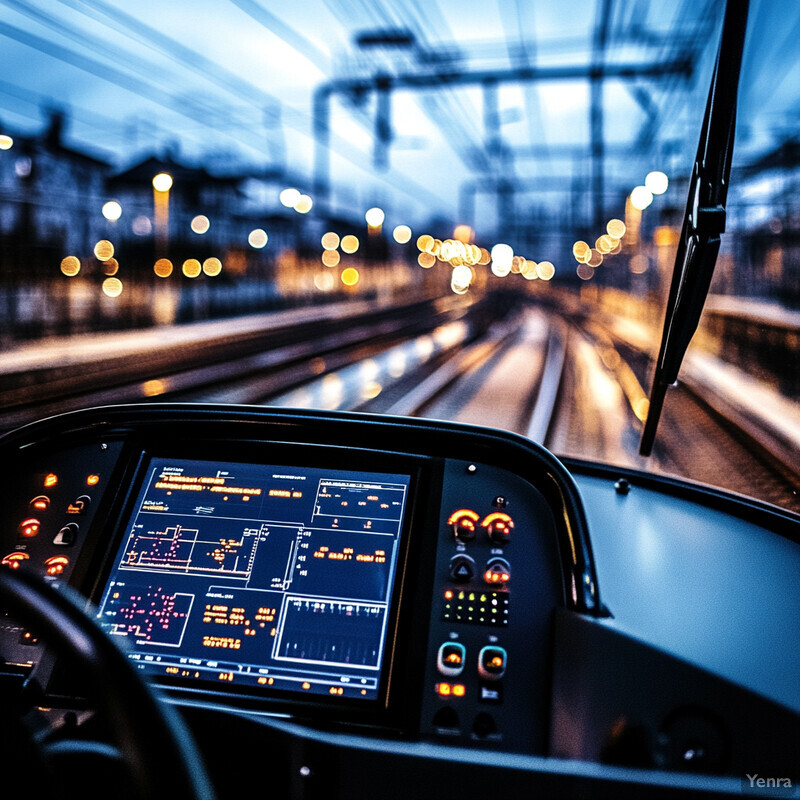
Relying solely on central servers to process sensor data can introduce delays, especially with increasingly large datasets. On-board edge computing places AI processing units directly on the train, allowing real-time analysis of data without needing continuous high-bandwidth connectivity. When a potential fault occurs, the AI on board can immediately evaluate sensor inputs and classify the issue. This rapid, decentralized approach allows operators to take corrective actions—like slowing the train or dispatching maintenance teams—much faster. Edge computing ensures a responsive, adaptable maintenance regime that enhances safety and reduces the likelihood of severe failures.
8. Sensor Fusion Techniques
By combining data from multiple sensing modalities—such as LIDAR, accelerometers, and ultrasonic sensors—AI improves detection accuracy, reduces false alarms, and provides a clearer view of asset health.
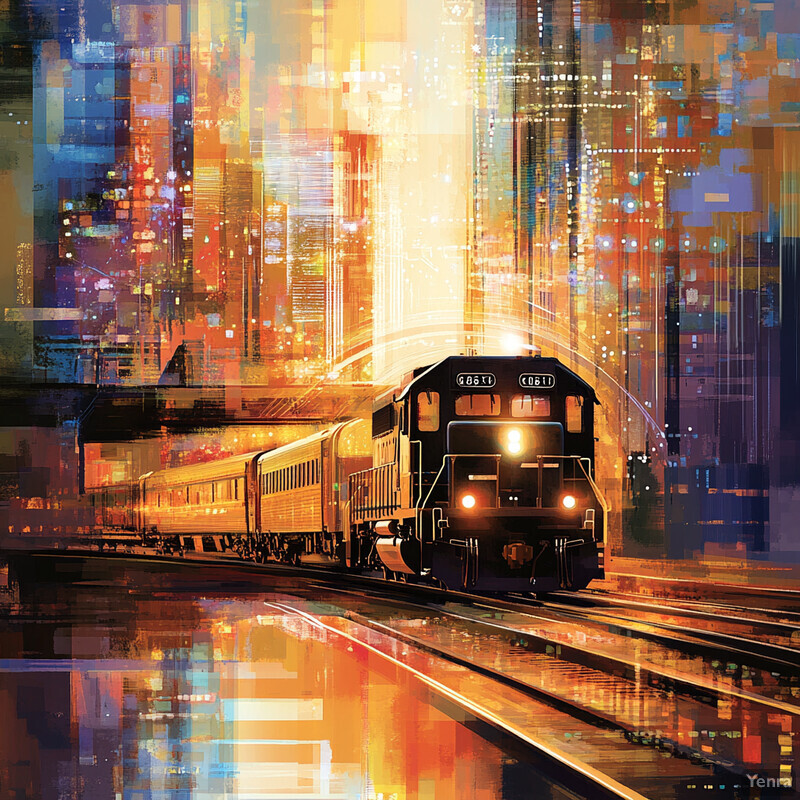
No single sensor type can provide a full picture of asset health. Sensor fusion combines inputs from multiple devices—such as cameras, LIDAR, ultrasonic detectors, accelerometers, and temperature gauges—into a unified data model. AI algorithms merge and interpret these diverse data streams to detect faults with unprecedented accuracy. By integrating complementary views of infrastructure conditions, sensor fusion reduces false alarms, improves detection rates, and highlights issues that might remain hidden if analyzed in isolation. Ultimately, this holistic perspective on rail health leads to more reliable predictions, faster interventions, and improved overall network performance.
9. Deep Learning-Based Pattern Recognition
Neural networks learn to recognize complex fault patterns over time, making them adept at catching subtle emerging issues that traditional rule-based systems may overlook.
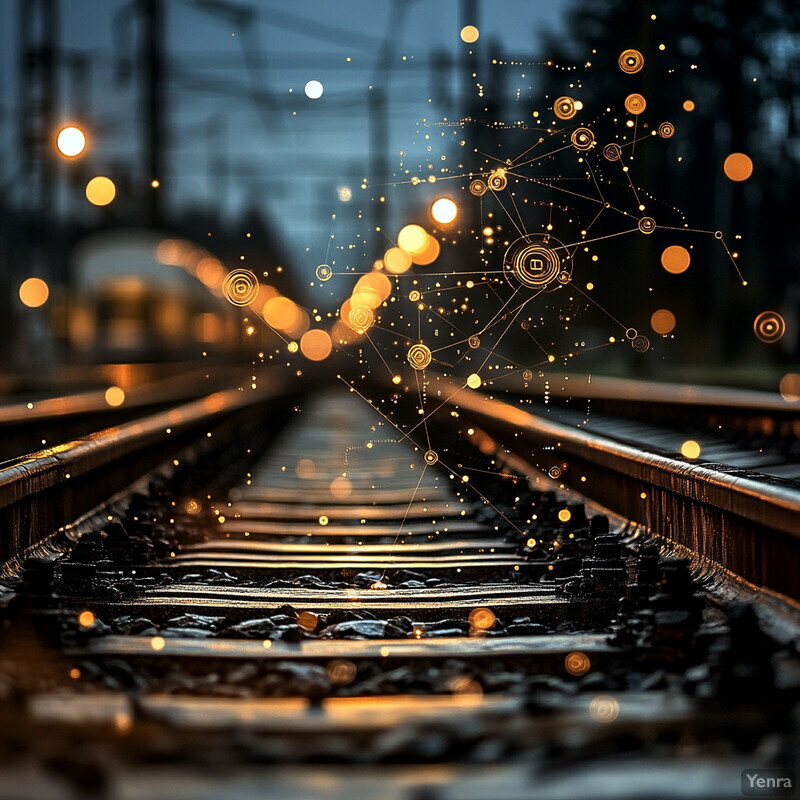
Many potential rail defects follow complex patterns that are not easily codified into simple rules. Deep learning methods excel at extracting intricate patterns from large, unstructured datasets. Over time, these models learn what “normal” operation looks like, and can spot deviations that signal emerging faults. This capability grows stronger with every new data point, making the AI system more accurate and adaptive. As a result, deep learning approaches transform raw sensor data into actionable intelligence, flagging subtle anomalies before human inspectors would ever suspect an issue, thereby enhancing preventative maintenance strategies.
10. AI-Driven Data Cleaning and Integration
With multiple data sources often producing noisy, incomplete information, AI can intelligently filter, standardize, and integrate various datasets, ensuring that analysts work with the highest quality inputs.
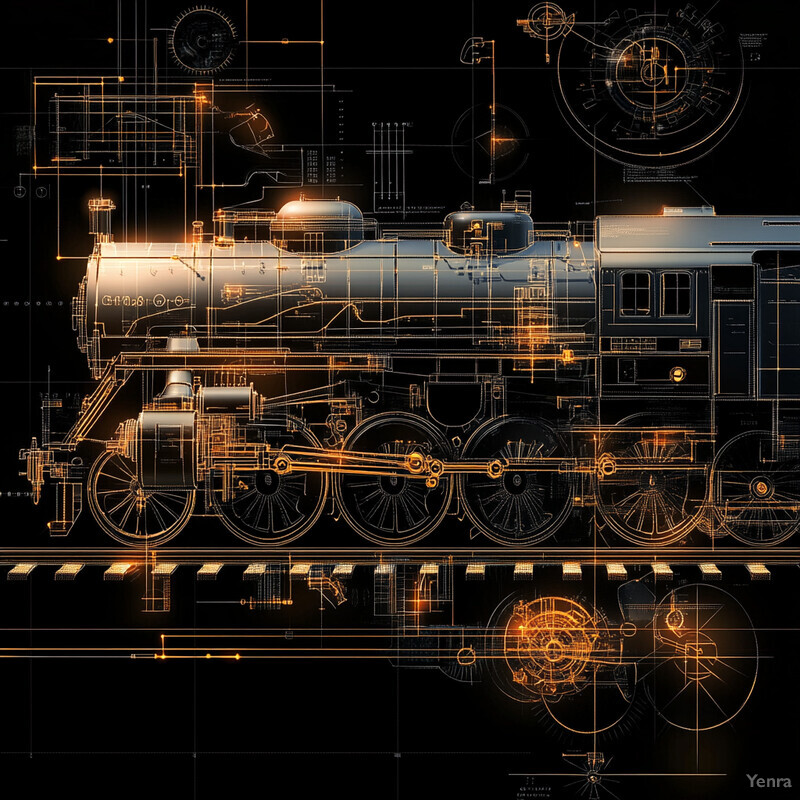
High-speed rail networks generate heterogeneous data: sensor signals, visual inspections, maintenance logs, weather records, and more. Each source may come in different formats and contain noise, errors, or gaps. AI tools for data cleaning and integration ensure that the information fed into fault detection models is accurate, consistent, and trustworthy. By intelligently filtering out noise, reconciling discrepancies, and merging various data streams, AI provides a solid foundation for subsequent analysis. In turn, this high-quality, consolidated dataset empowers more effective anomaly detection, predictive maintenance, and strategic decision-making across the entire rail operation.
11. Digital Twin Simulations
AI-powered digital twins replicate entire rail systems, allowing engineers to simulate faults, stress tests, and maintenance actions, thus improving real-world detection and mitigation strategies.
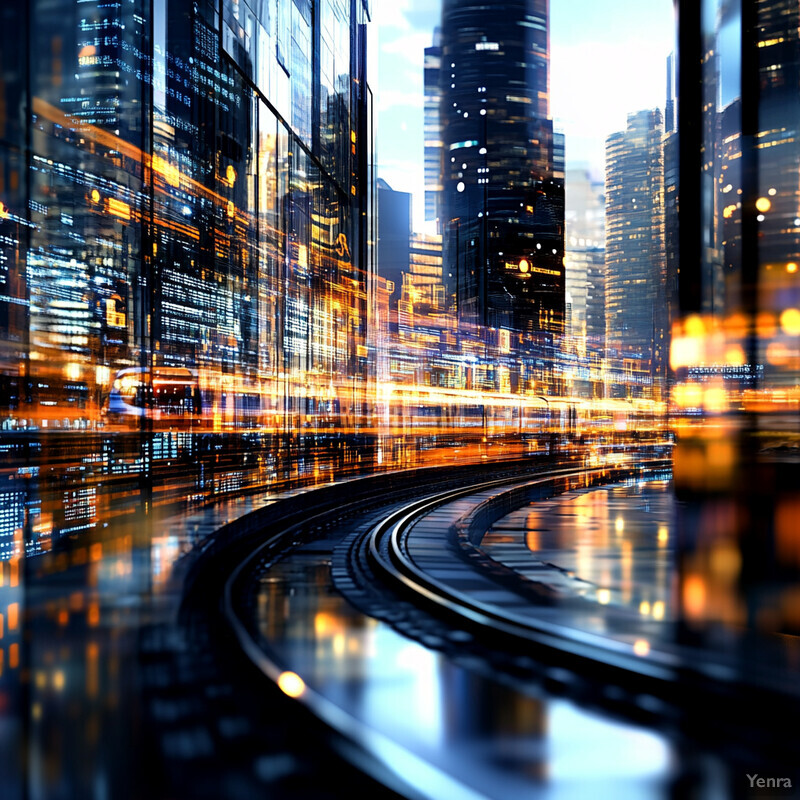
A digital twin is a virtual replica of the entire rail system, from trains and tracks to power supplies and signaling infrastructure. AI models run simulations in this virtual environment to test how components behave under stress, extreme weather, or heavy usage. By experimenting with various fault conditions, engineers can predict failure points, refine maintenance schedules, and improve design strategies. As real-world data flows in, the digital twin updates and evolves, providing ongoing insights into the system’s health. This synergy between physical and virtual realms leads to more proactive fault detection and informed decision-making.
12. Anomaly Detection in High-Frequency Data
Advanced AI models can continuously monitor and analyze the extremely high-frequency data streams generated by modern HSR systems, catching anomalies that human inspectors would miss.
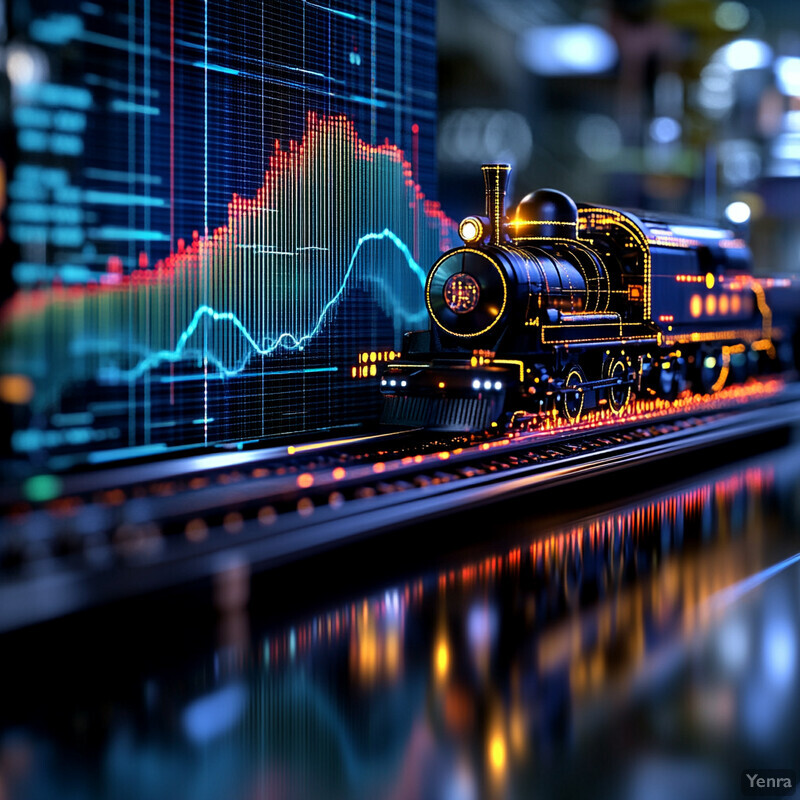
High-speed trains and modern track infrastructures generate massive data volumes at extremely high frequencies. Identifying meaningful patterns within such torrents of information can overwhelm human analysts. AI excels at sifting through high-frequency datasets, spotting subtle shifts that might indicate a crack forming or a component loosening. Advanced models can filter out normal fluctuations—like noise from changing loads or small temperature shifts—and isolate significant anomalies. By giving operators timely warnings, these systems provide the opportunity to intervene early, minimizing the risk of sudden breakdowns or safety incidents.
13. Predicting Environmental Impact on Rails
By factoring in weather conditions, temperature fluctuations, and seasonal effects, AI can predict how environmental variables might contribute to cracks, corrosion, or other rail defects.
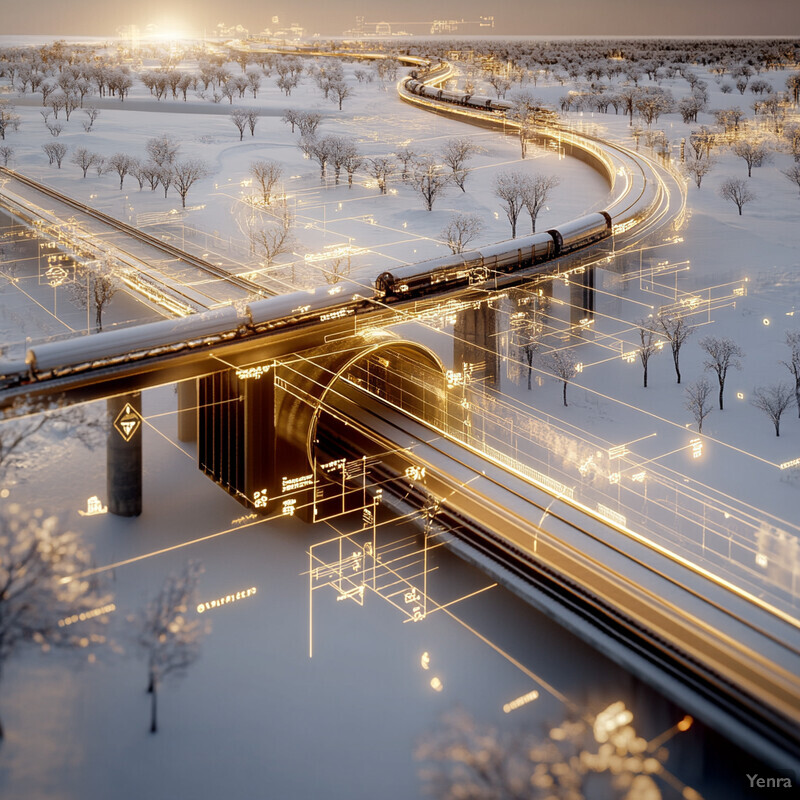
Rails and their supporting structures must withstand harsh environmental conditions, from intense heat and cold to seasonal rains and freeze-thaw cycles. AI systems integrate weather forecasts, historical climate data, and knowledge of material properties to predict how environmental factors will affect rails over time. For example, it can foresee where thermal stresses might cause cracks or where water infiltration could lead to corrosion. Armed with these insights, maintenance teams can preemptively treat vulnerable sections, choose more durable materials, or adjust inspection intervals, thereby reducing environmentally induced faults and extending infrastructure life.
14. Adaptive Algorithms for Continual Learning
As new fault data are collected, AI systems update their models, becoming progressively better at distinguishing normal wear from critical failures, and adapting to evolving rail technology and materials.
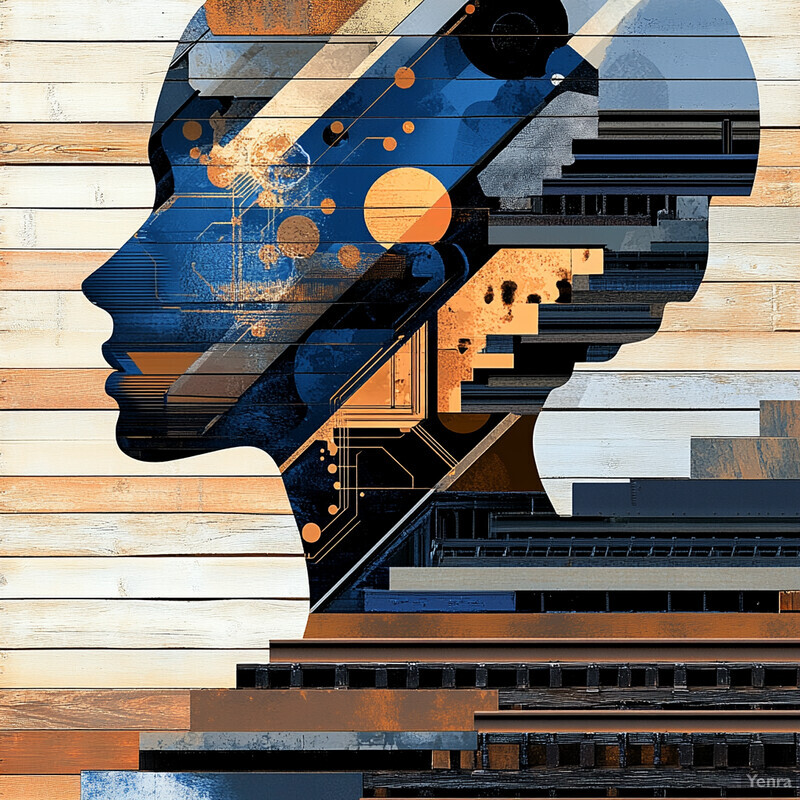
Railway systems evolve as new technologies emerge, track materials improve, and maintenance techniques change. AI’s continual learning capabilities ensure that detection models stay relevant over time. As more data is gathered—including feedback from past maintenance actions—the algorithms refine their understanding of what constitutes a fault and how best to address it. They adapt to new track configurations, rolling stock designs, or environmental shifts, maintaining high detection accuracy. In effect, the AI grows smarter as it accumulates experience, guaranteeing that detection capabilities remain cutting-edge, no matter how the railway landscape changes.
15. Automated Root Cause Analysis
When faults are detected, AI can assist in determining their underlying causes—whether it’s a manufacturing defect, improper maintenance, or environmental stressors—enhancing problem-solving efficiency.
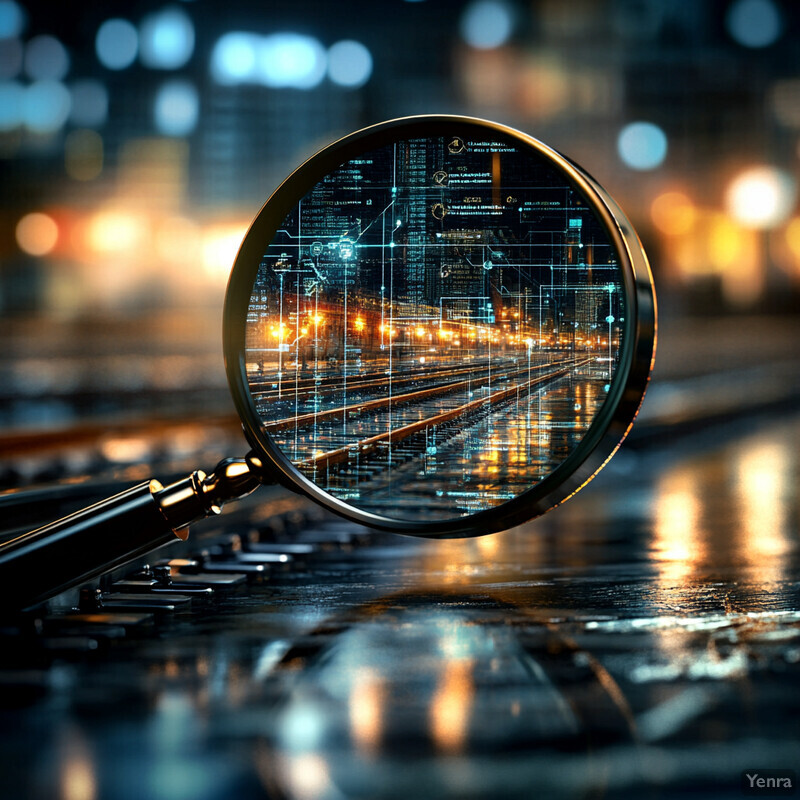
Detecting a fault is only the first step—understanding why it occurred is equally important. AI automates this root cause analysis by examining patterns in sensor data, maintenance logs, operational decisions, and environmental factors. By correlating anomalies with historical incidents, component quality records, or installation practices, AI isolates the underlying causes of failures. This knowledge enables operators to implement targeted interventions, from improved maintenance techniques to supplier changes, and prevents recurrence of similar defects. The result is a more resilient infrastructure that continually improves as lessons from past incidents are incorporated into future decisions.
16. Remote Monitoring for Inaccessible Infrastructure
In tunnels, bridges, or remote track sections, AI analyzes data from autonomous drones or robots that inspect infrastructure where human access is limited, ensuring that faults are caught early.
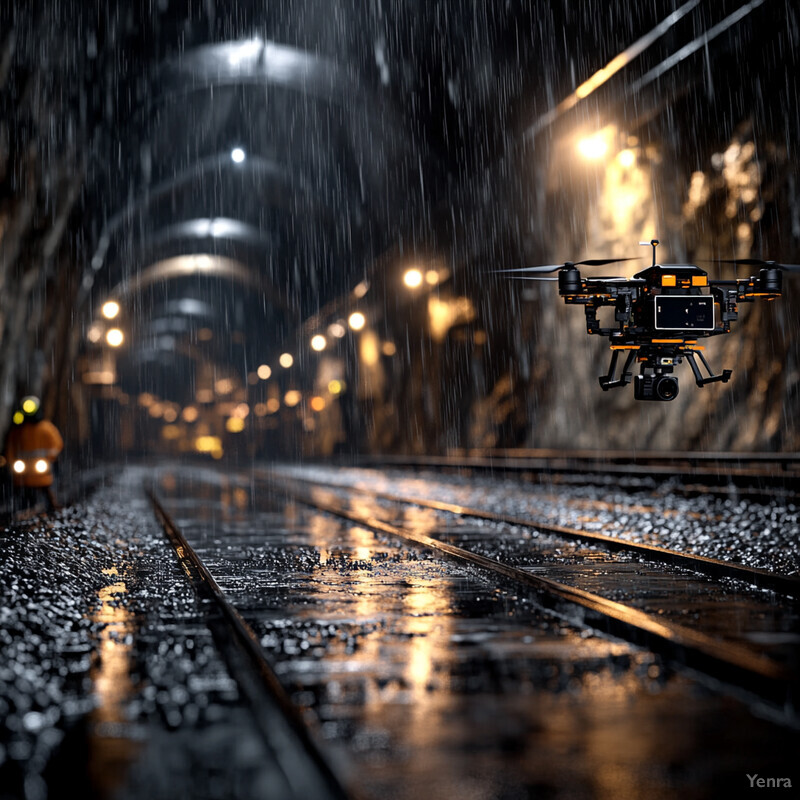
Some railway segments—tunnels, bridges, or high-grade viaducts—are challenging and dangerous for humans to inspect regularly. AI-powered drones and robots equipped with cameras, sensors, and data-processing capabilities can reach these areas safely. By capturing detailed environmental data and imagery, the AI detects structural issues, water leaks, or rail misalignments in places that would otherwise be seldom checked. This approach reduces maintenance costs, mitigates safety risks for workers, and ensures that potential faults in hard-to-access areas do not go unnoticed, thus maintaining the integrity and reliability of the entire rail network.
17. Intelligent Alarm Management
By filtering out false positives and prioritizing critical alerts, AI reduces 'alarm fatigue' for operators, ensuring that legitimate faults are addressed promptly and effectively.
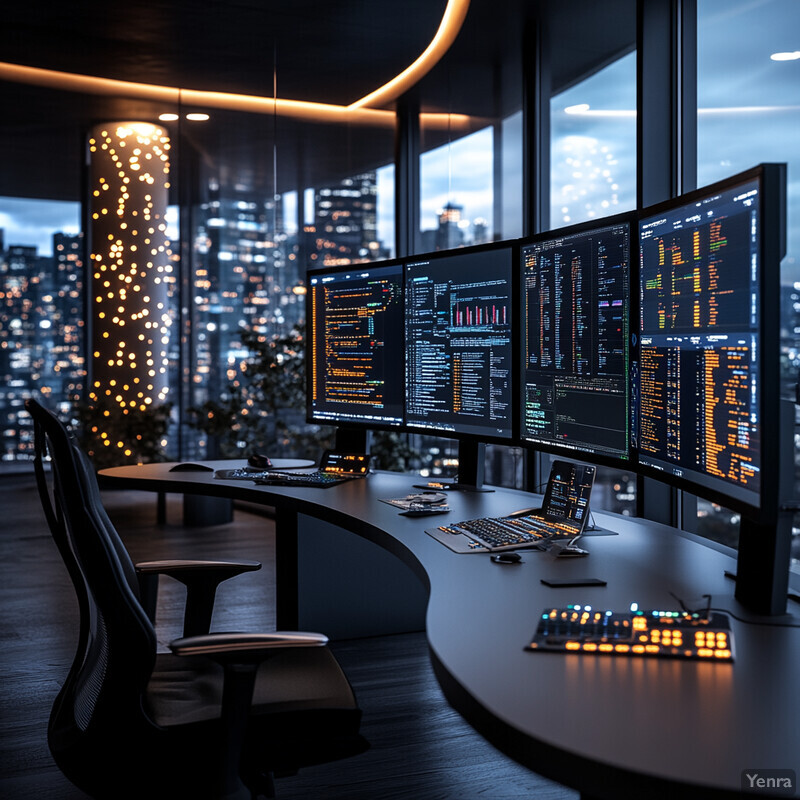
Without proper filtering, advanced detection systems can generate numerous alarms, many of which might be false positives or low-priority alerts. AI-driven intelligent alarm management discriminates between critical issues and minor deviations. It weighs multiple indicators—severity, frequency, location, and historical data—before escalating the alert. By reducing “alarm fatigue,” operators can focus on genuine threats without being distracted by inconsequential warnings. This improves operational efficiency, ensures that maintenance teams address the most pressing issues first, and generally enhances the overall responsiveness of rail operations to fault conditions.
18. Optimized Inspection Intervals
With data-driven insights, AI can dynamically adjust inspection intervals, ensuring that maintenance teams focus on areas of highest risk, rather than following a rigid schedule.
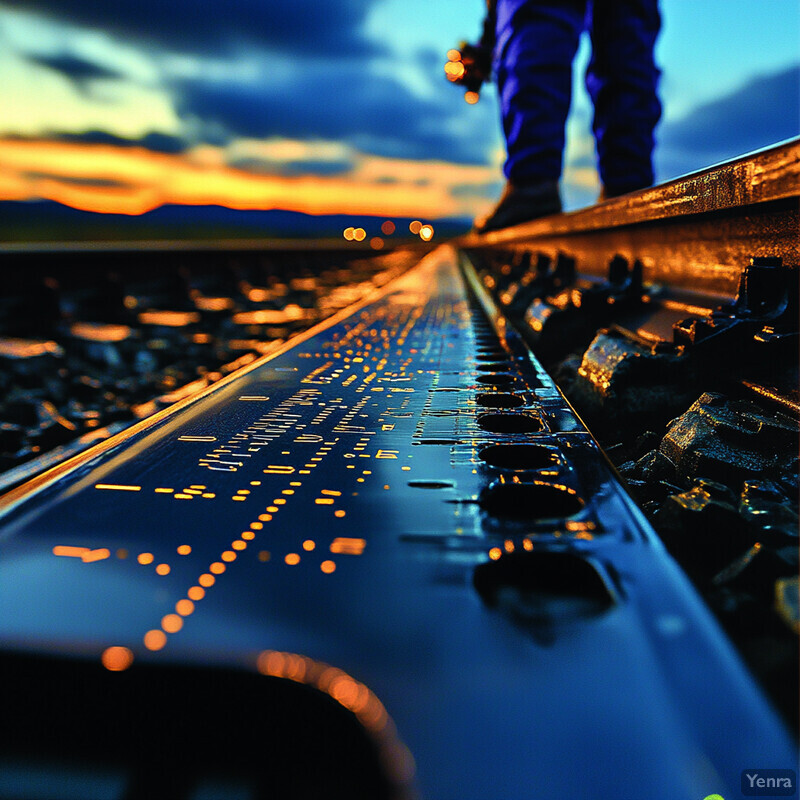
Routine inspections can be expensive and time-consuming. By leveraging AI insights, operators can move from fixed schedules to condition-based inspections. When the AI’s predictive models indicate that certain track segments or components are more likely to develop faults soon, teams can inspect those areas sooner. Conversely, if a component appears stable, inspections can be safely postponed. This dynamic, data-driven approach minimizes unnecessary downtime, optimizes the use of human and material resources, and ensures that the most critical maintenance tasks receive prompt attention.
19. Natural Language Processing (NLP) on Maintenance Logs
AI reads and interprets historical maintenance logs, technician notes, and inspection reports, extracting patterns or recurring issues that might not be evident from sensor data alone.
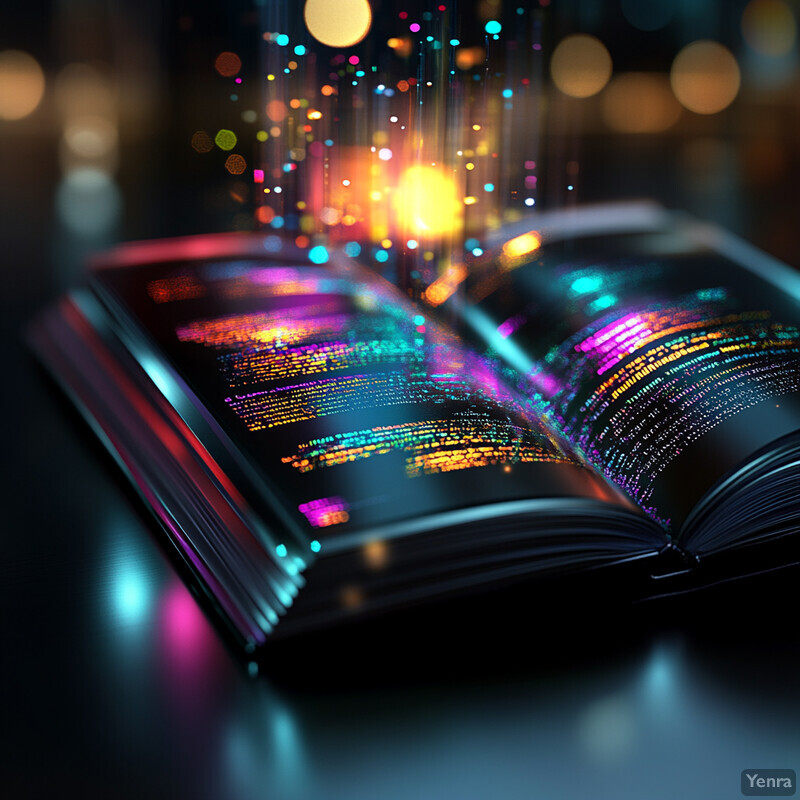
Vast amounts of institutional knowledge are locked in unstructured text data—maintenance reports, technician notes, and historical logs. NLP algorithms can read and interpret this text, identifying patterns that quantitative sensor data might miss. For example, repeated mentions of a specific failure type in a particular region might highlight a systemic issue or vendor-related problem. By extracting actionable insights from written records, AI enriches the overall understanding of rail system health and guides operators toward better fault prevention strategies, ultimately enhancing the reliability of high-speed rail services.
20. Enhanced Safety with Real-Time Decision Support
AI systems provide guidance and recommendations to control center staff, enabling them to swiftly implement contingency plans, slow train speeds, or reroute services to prevent accidents stemming from undetected faults.
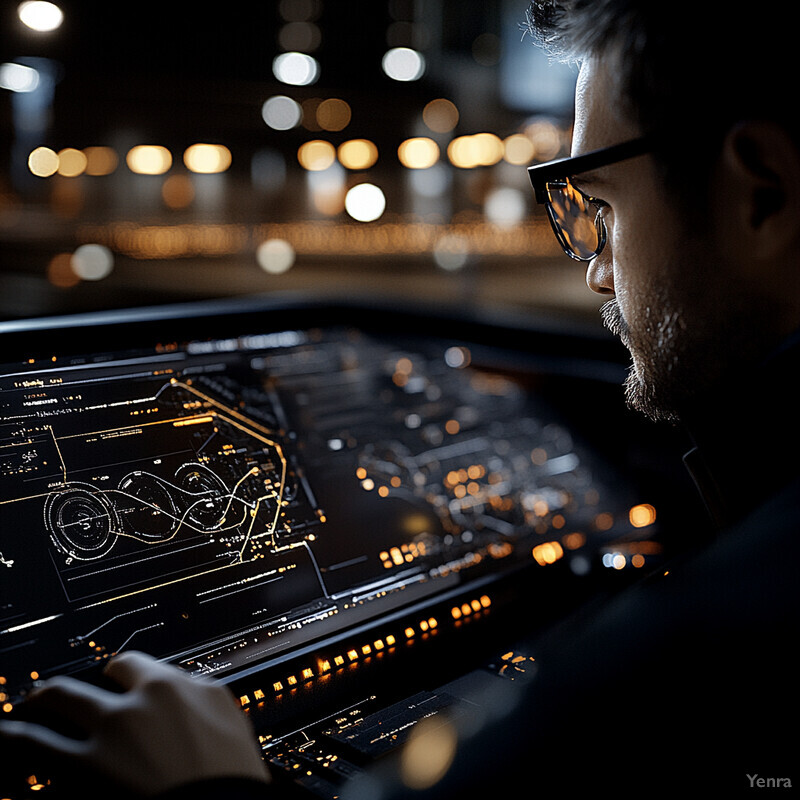
When potential faults are detected, timely action is crucial. AI-powered decision support tools provide controllers and operators with data-driven recommendations in real time. For example, they might advise reducing train speed, rerouting traffic, or scheduling an immediate inspection. By evaluating possible outcomes and assessing risks quickly, the AI ensures that stakeholders can react promptly and effectively, minimizing the likelihood of accidents or prolonged service disruptions. This continuous guidance not only enhances safety but also builds confidence in the reliability and resilience of modern high-speed rail networks.