1. Enhanced Data Assimilation
AI-driven algorithms can integrate heterogeneous data sources—such as satellite observations, ground-based measurements, and economic data—into greenhouse gas (GHG) models more seamlessly, improving accuracy and reliability.
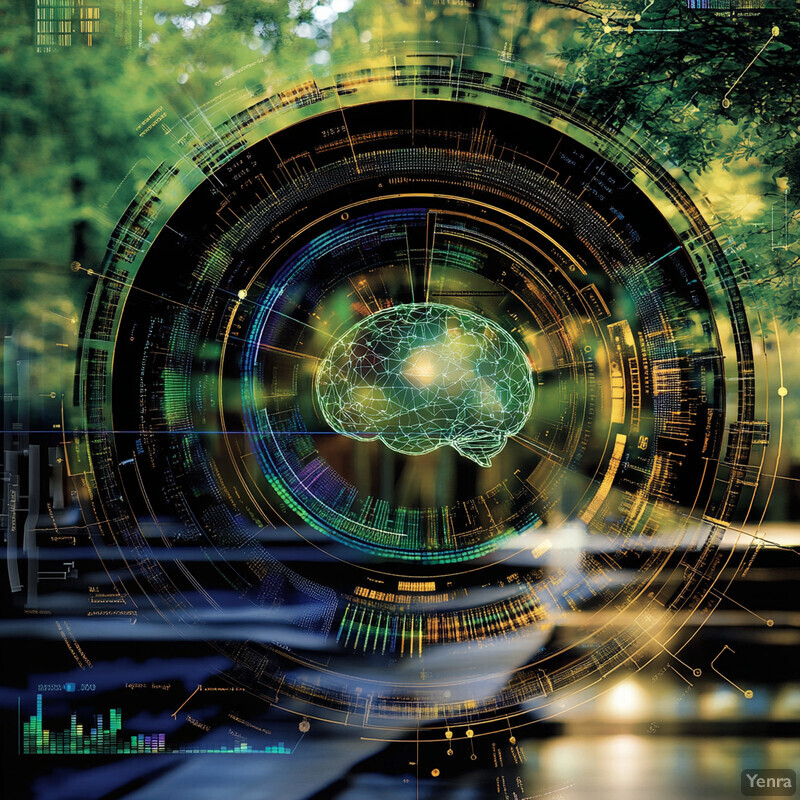
Traditionally, greenhouse gas emission models have struggled with the vast array of inputs that influence atmospheric composition—ranging from industrial output and transportation activity to deforestation and agricultural practices. AI-driven data assimilation methods use machine learning algorithms that can ingest, filter, and harmonize data from disparate sources such as satellite images, ground sensors, historical inventories, and even social and economic indicators. By intelligently combining multiple heterogeneous datasets, these methods reduce noise and data gaps, leading to more accurate and stable results. The machine learning tools can also continuously update and refine models as new data arrives, ensuring that the model output remains current. This approach significantly improves our understanding of spatial and temporal patterns of emissions, guiding more targeted mitigation strategies.
2. High-Resolution Spatial Modeling
Machine learning techniques enable finer spatial resolution in emissions maps, allowing for detailed local- and regional-scale emission assessments.
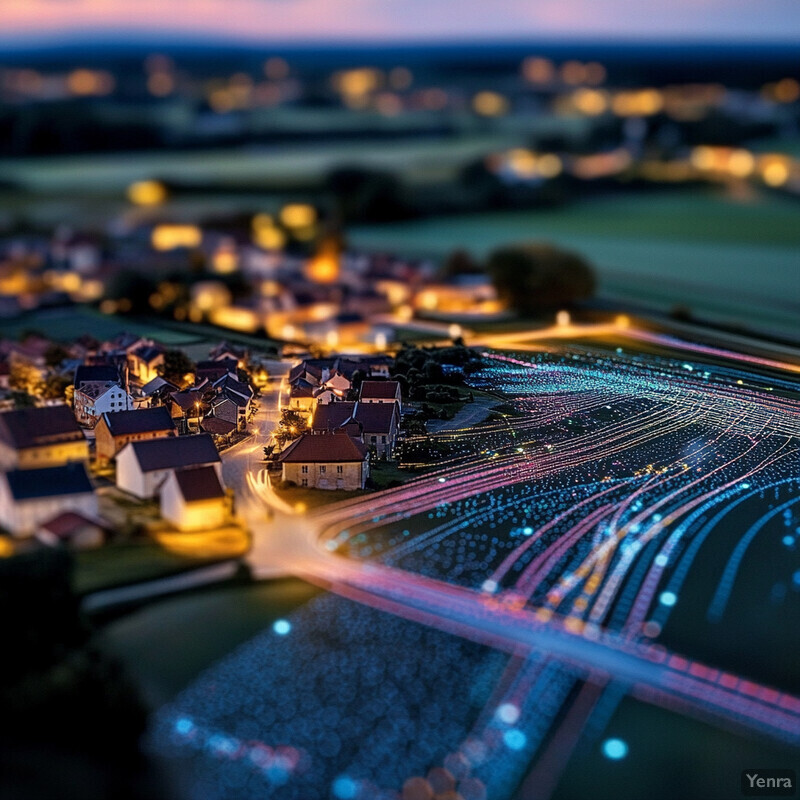
AI techniques, especially convolutional neural networks and other advanced spatial analysis tools, allow for modeling GHG emissions at incredibly fine geographic scales. Instead of coarse national or regional averages, these methods can pinpoint emissions down to the scale of cities, neighborhoods, or even individual facilities. This level of detail helps identify local emission hotspots, enabling policymakers and industry leaders to focus efforts where they are most needed. Higher resolution modeling also supports environmental justice initiatives by revealing disparities in emissions exposure. Over time, improvements in resolution can foster better monitoring of policy impacts and progress toward emission reduction targets at the community level.
3. Temporal Forecasting Accuracy
Recurrent and temporal neural networks can better capture seasonal, annual, and decadal emission trends, improving short-term forecasts and long-term climate scenario planning.
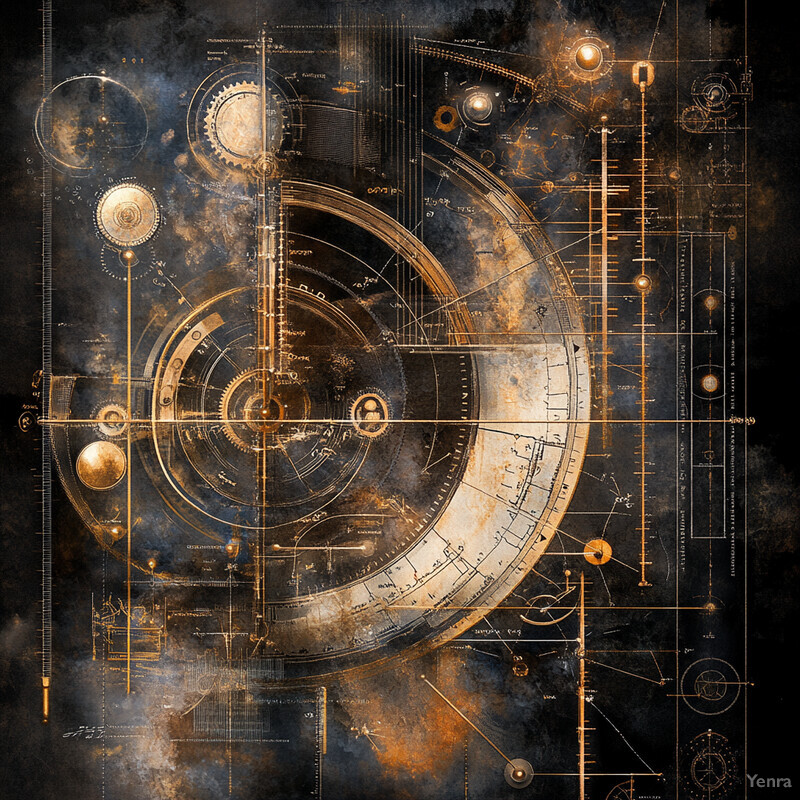
Emission patterns vary over seasons, years, and decades due to economic cycles, policy changes, technological advancements, and consumer behavior. AI models that incorporate time-series analysis, such as recurrent neural networks (RNNs) and long short-term memory (LSTM) networks, can capture these complex temporal dynamics. They learn patterns in historical data to predict future trends, refining forecasts as conditions shift. Improved temporal accuracy means decision-makers can better anticipate near-term changes—such as winter heating demands—and long-term trajectories—such as gradual shifts toward renewable energy. This helps in strategic planning, enabling timely policy interventions and more efficient allocation of resources for climate mitigation.
4. Uncertainty Quantification
Advanced probabilistic modeling techniques, including Bayesian methods and ensemble learning, help quantify uncertainties in emissions estimates, enhancing credibility in decision-making.
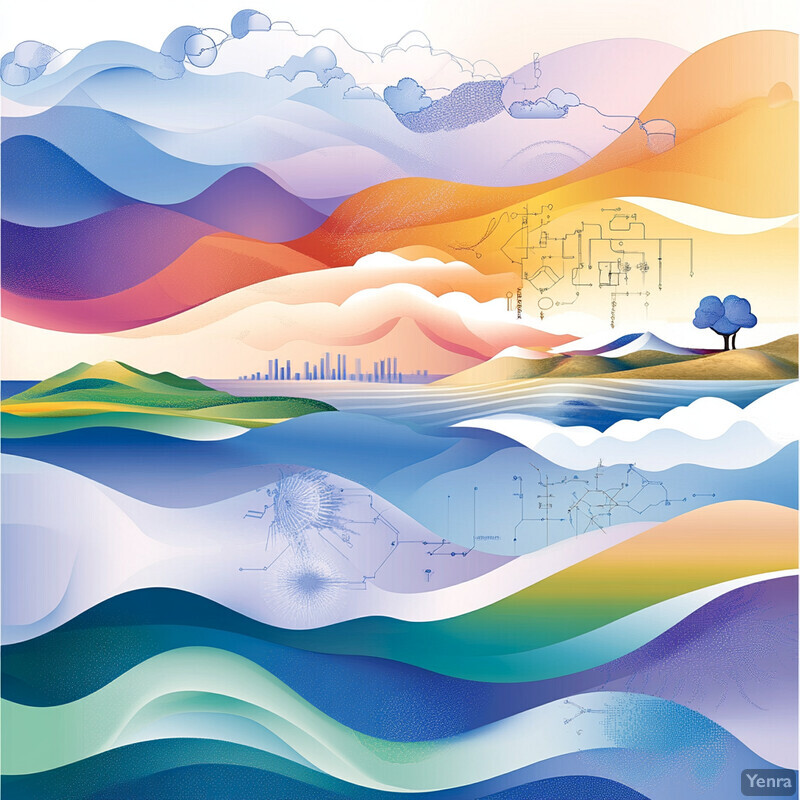
All models contain uncertainties stemming from data variability, incomplete knowledge, and simplifications in representation. AI-driven ensemble methods and Bayesian modeling approaches rigorously quantify the uncertainty in greenhouse gas predictions. By generating probability distributions rather than single-point estimates, these techniques highlight the range of possible outcomes. Policymakers can then assess risks and make decisions with a clear understanding of confidence levels. Improved uncertainty quantification also aids in model validation and intercomparison, fostering collaboration and consensus in the scientific community about the reliability of emissions models.
5. Incorporation of Nonlinear Interactions
Deep learning models can represent complex, nonlinear relationships between emissions drivers—like changes in land use, energy consumption patterns, and economic growth—uncovering underlying dynamics missed by linear models.
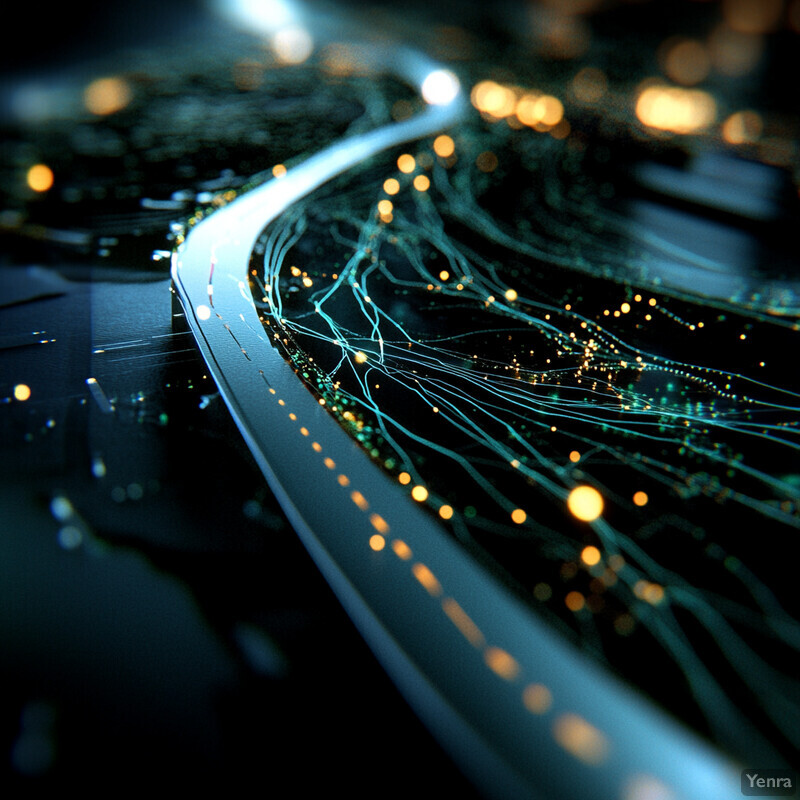
The relationships driving GHG emissions—from energy consumption and land-use change to technological innovation—are rarely linear. Deep learning models excel at uncovering hidden, nonlinear patterns within complex, high-dimensional datasets. By modeling complex feedback loops, interactions, and threshold effects, AI can reveal insights that traditional linear regression techniques might miss. This includes understanding how small policy shifts might lead to disproportionately large emission reductions or identifying non-intuitive linkages between sectors. Ultimately, capturing these nonlinearities improves scenario modeling, allowing for more nuanced strategies to achieve ambitious climate targets.
6. Inverse Modeling of Emissions
AI-based inverse modeling techniques use atmospheric concentration data to infer underlying source strengths and emissions distributions, helping pinpoint emission hotspots and validate national inventories.
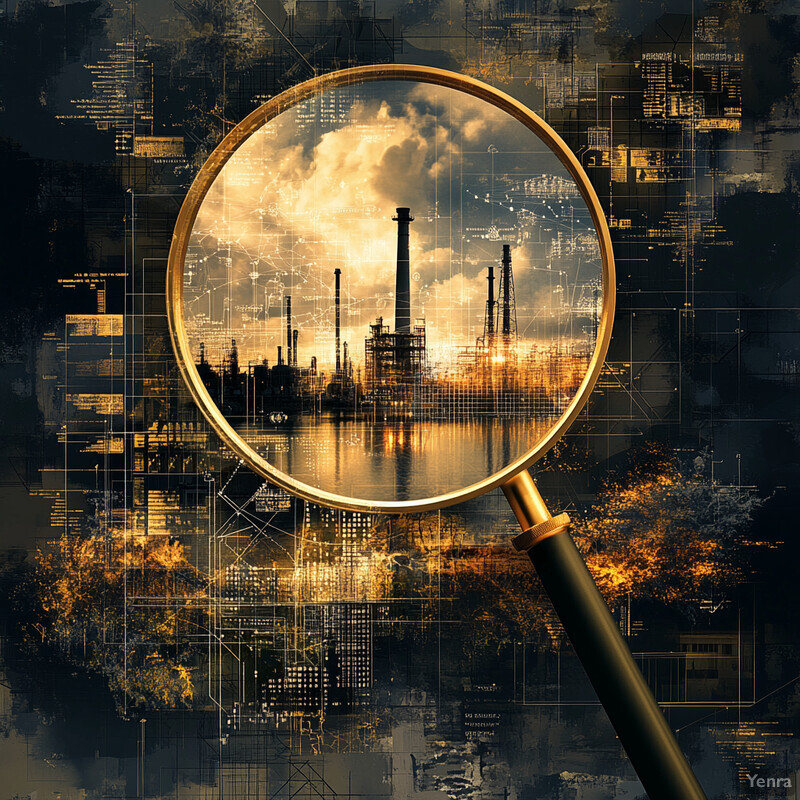
While emission inventories often start with known sources and estimate their contributions, inverse modeling takes an atmospheric perspective. AI-driven inverse modeling uses observed concentrations of greenhouse gases in the atmosphere and works backward to infer the location and magnitude of their sources. This is computationally challenging, but machine learning optimization methods have made it more feasible. Such techniques are invaluable for identifying unreported or underestimated sources, verifying compliance with emission targets, and improving the accuracy of national and regional emission inventories. In turn, this supports international climate agreements and enhances transparency in global climate governance.
7. Automated Feature Selection
Machine learning frameworks can automatically identify relevant input variables, simplifying model development and focusing attention on the most influential factors affecting emissions.
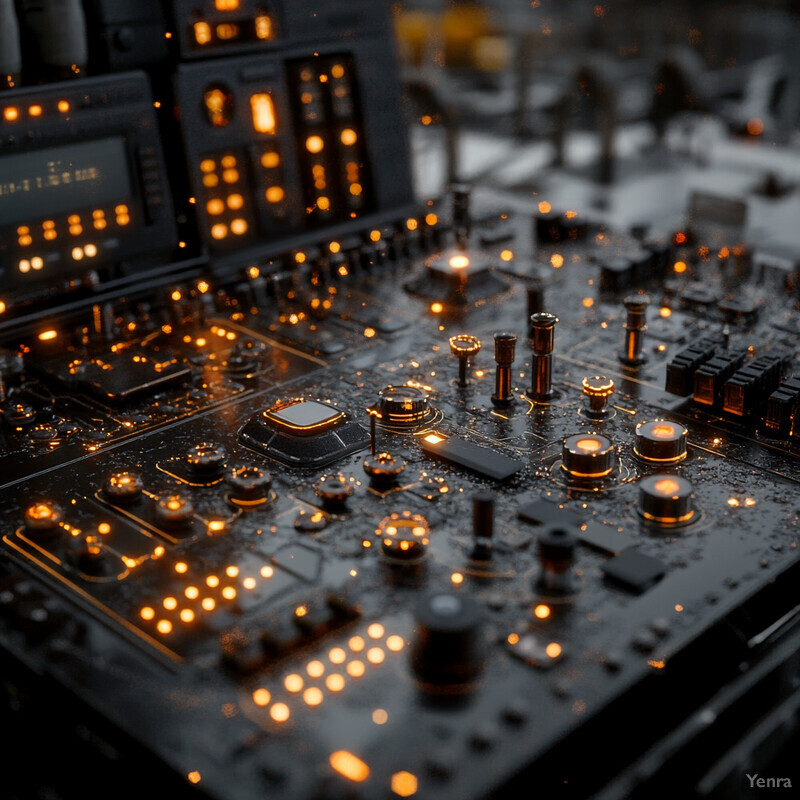
Determining which variables drive emissions is a critical but often time-consuming task. AI models can automatically rank and select features—be they temperature records, industrial output metrics, energy consumption patterns, or land cover changes—that most significantly influence emissions. This streamlines model development by reducing the dimensionality of input datasets and focuses analytical effort on the most impactful variables. The resulting models are both simpler and more robust, enabling researchers and policymakers to direct resources toward the key drivers of greenhouse gas changes and design more effective mitigation measures.
8. Integration with Remote Sensing Data
AI methods can rapidly process large volumes of satellite imagery and LiDAR data, detecting subtle changes in vegetation, land cover, and industrial activity related to emissions.
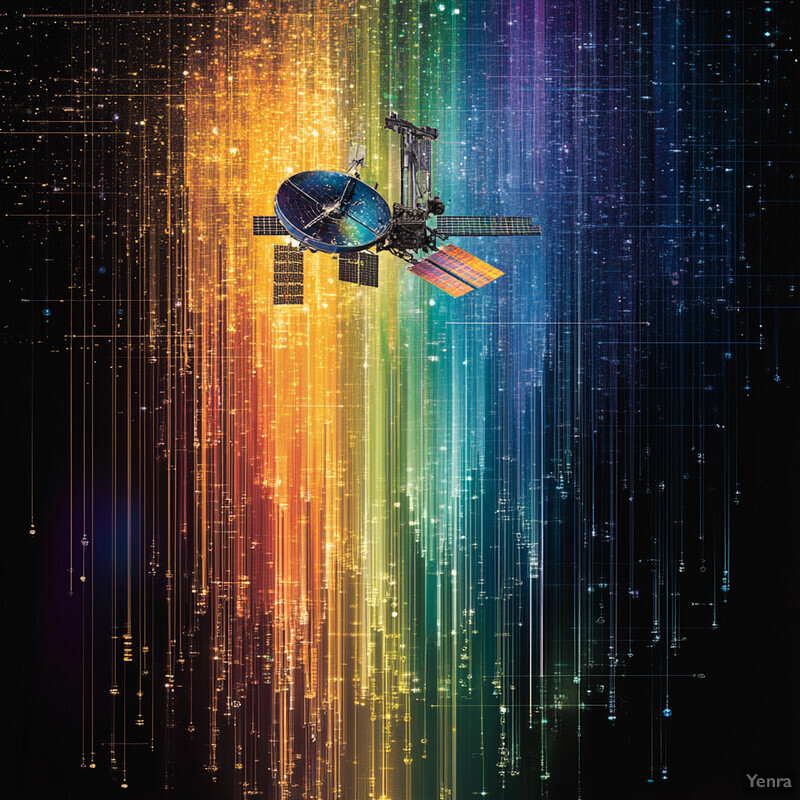
Massive volumes of satellite imagery and other remote sensing outputs—thermal readings, hyperspectral data, radar measurements—hold a wealth of information on emissions. However, extracting meaningful signals from these noisy, large-scale datasets is a challenge. AI-powered image recognition, object detection, and signal processing methods can quickly identify signs of industrial activity, changes in vegetation cover that influence carbon sinks, and alterations in land use patterns. By integrating remote sensing with ground-based and economic data, AI helps produce consistent, spatially explicit emission estimates. This integration also accelerates the detection of trends, enabling proactive environmental management on a global scale.
9. Real-Time Monitoring and Alert Systems
Streaming analytics powered by AI can flag anomalies in emissions data in near-real time, enabling quicker interventions and targeted policy responses.
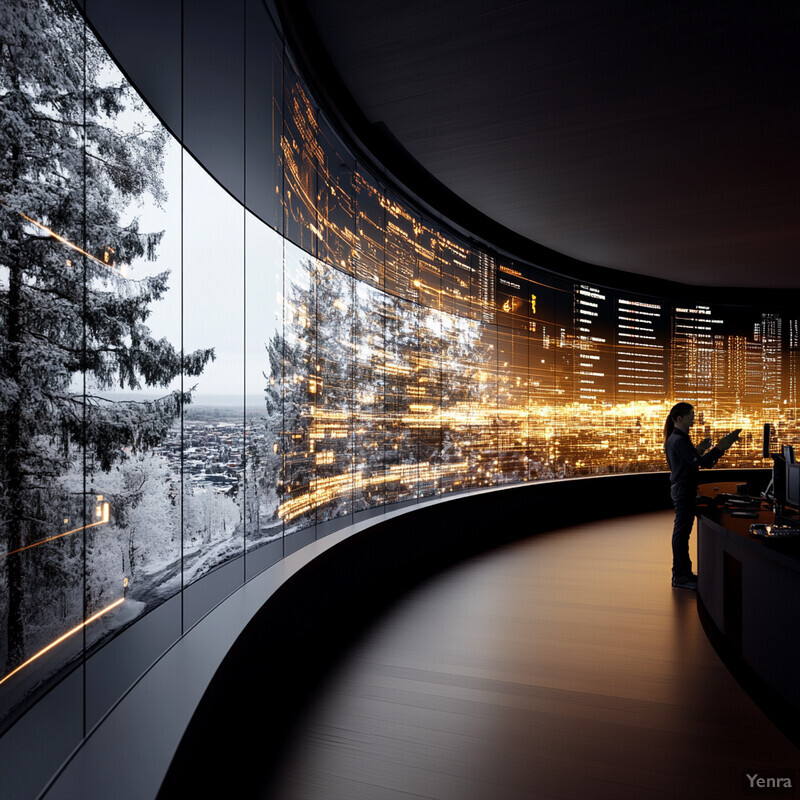
AI models can process streaming emissions data—from continuous sensor networks or frequent satellite overpasses—in real time. These systems detect anomalies, such as sudden surges in methane from a leaking pipeline or unexpected CO₂ spikes near heavy industrial zones. Alerting stakeholders promptly allows for quick interventions, potentially preventing minor issues from becoming major environmental hazards. Over time, continuous monitoring also refines emission inventories and supports adaptive policies that respond dynamically to on-the-ground realities. This shift from static reporting to dynamic, proactive management is a key advancement in emissions control.
10. Predictive Maintenance in Emission-Intensive Sectors
Reinforcement learning and predictive analytics can anticipate equipment failures or inefficiencies in factories, refineries, or power plants, reducing unnecessary emissions through timely maintenance.
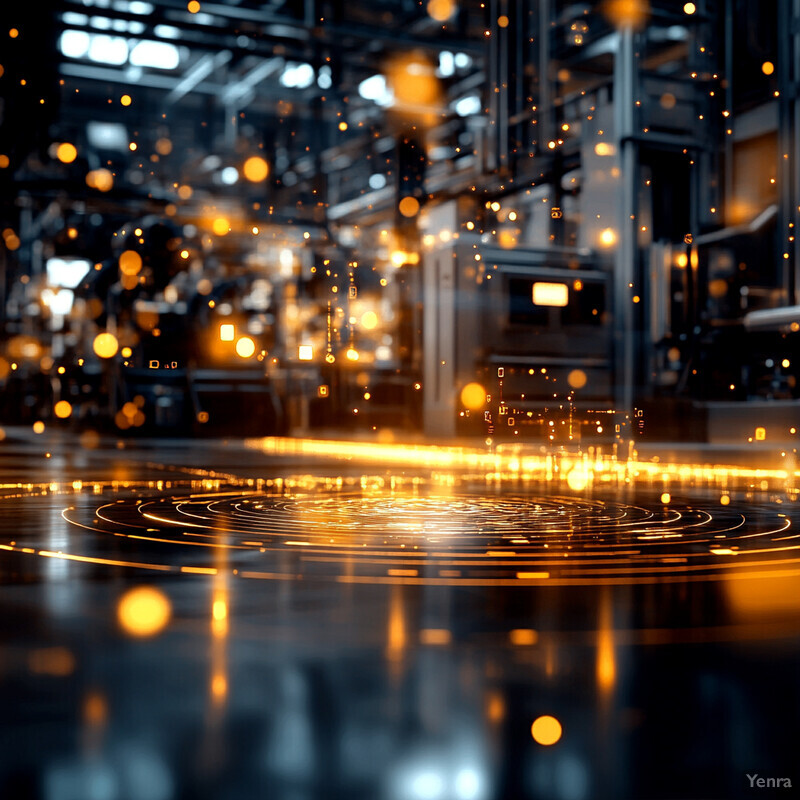
Many industrial processes and energy systems produce emissions, but not always efficiently. AI-driven predictive maintenance algorithms analyze machinery sensor data—temperature, pressure, vibration—to detect signs of wear or impending failure. By anticipating these issues, operators can schedule maintenance or upgrades before inefficiencies escalate emissions unnecessarily. Such optimizations can significantly reduce the carbon footprint of factories, refineries, and power plants. Beyond lowering emissions, predictive maintenance also cuts costs and enhances productivity, aligning economic and environmental goals.
11. Optimization of Mitigation Strategies
AI-driven optimization can compare various greenhouse gas reduction strategies—such as renewable energy deployment or improved forestry management—to determine the most cost-effective paths.
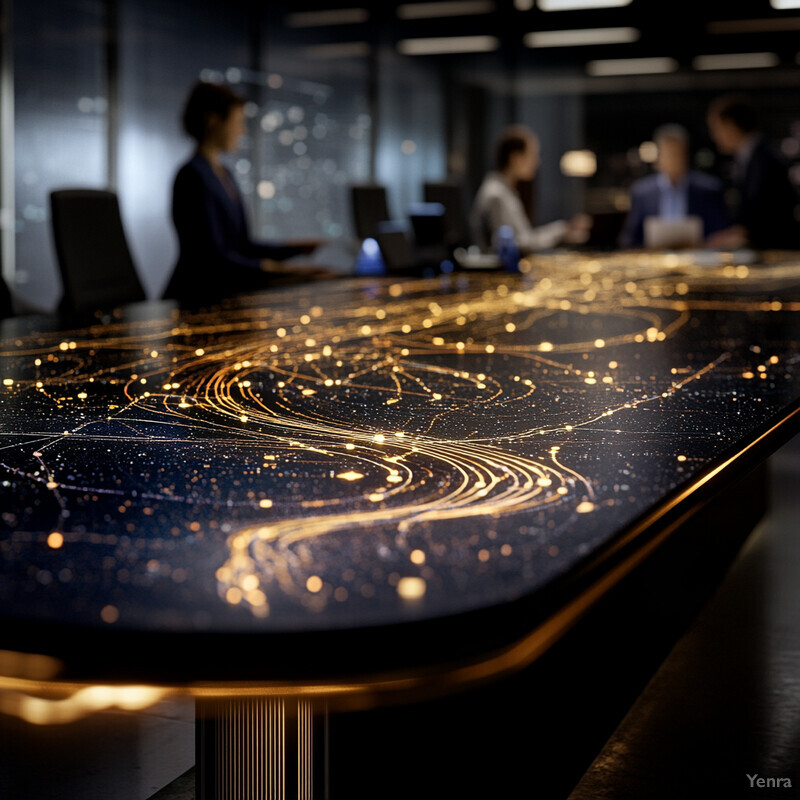
Crafting effective greenhouse gas reduction plans involves balancing costs, technological feasibility, and policy constraints. AI optimization algorithms evaluate thousands or millions of scenarios to identify the most cost-effective and socially acceptable approaches. They can consider a mix of renewable energy deployment, improvements in public transportation, sustainable agriculture practices, or carbon capture technologies. By rapidly exploring these complex solution spaces, AI tools guide policymakers toward interventions that deliver the highest emission reductions for each dollar spent. This leads to more targeted investments and a faster transition to low-carbon economies.
12. Improved Scenario Analysis
Generative AI models can produce multiple plausible future scenarios for greenhouse gas trajectories under different policy and technological assumptions, aiding in robust strategic planning.
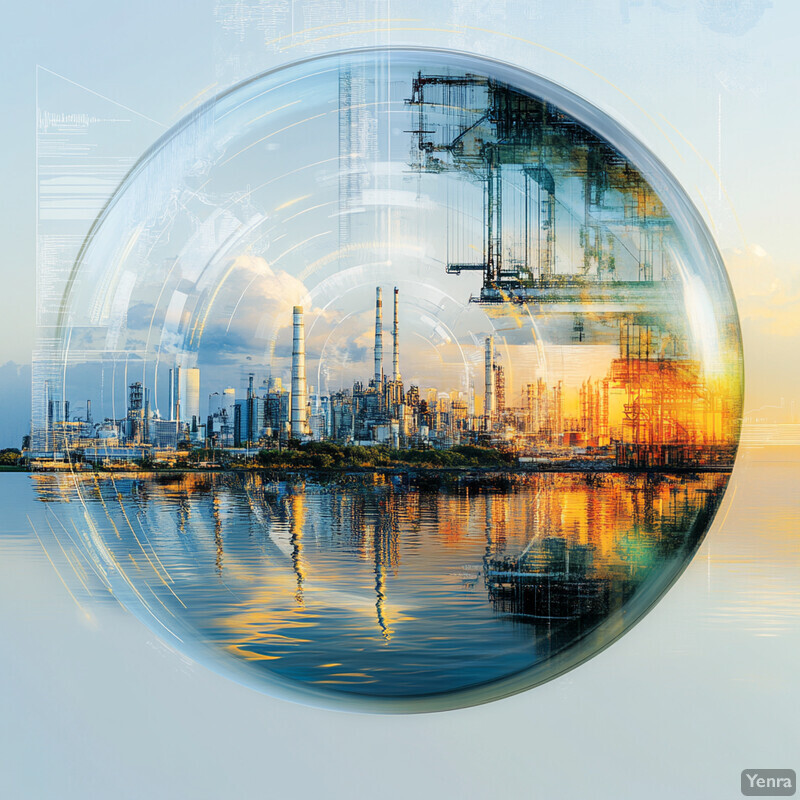
Scenario analysis is central to climate planning, helping us envision what the future might look like under different assumptions. AI-enhanced scenario modeling uses generative models to produce a wide range of plausible emission trajectories. These scenarios incorporate varying degrees of economic growth, policy stringency, technological breakthroughs, and behavioral changes. Policymakers can then stress-test their plans against these scenarios, ensuring policies are robust and flexible. This comprehensive approach to scenario analysis improves resilience, allowing decision-makers to prepare for a range of possible futures.
13. Land Use and Agricultural Emissions Modeling
Deep learning can better estimate GHG emissions from agriculture, deforestation, and land-use changes by interpreting complex environmental indicators from diverse datasets.
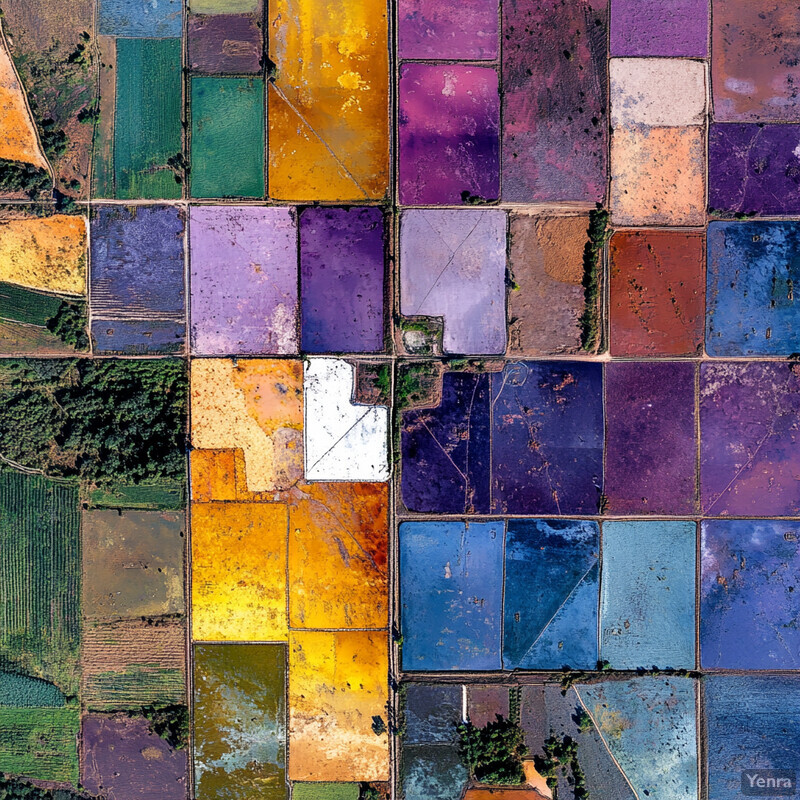
Land use changes—like deforestation, urban sprawl, or peatland draining—have major impacts on greenhouse gas fluxes, as do agricultural practices such as fertilizer use and livestock management. AI models can integrate satellite imagery, soil data, crop yield records, and climate variables to better estimate GHG fluxes from these sectors. They capture subtle trends, like how shifts in crop varieties or irrigation methods affect methane and nitrous oxide emissions. By clarifying these relationships, AI helps guide policies and practices in agriculture and land management, reducing emissions while maintaining food security and ecosystem health.
14. Integration into Climate-Economic Models
Incorporating AI into Integrated Assessment Models (IAMs) can link economic activities and policies directly with climate feedback loops, refining projections of emissions under various socioeconomic conditions.
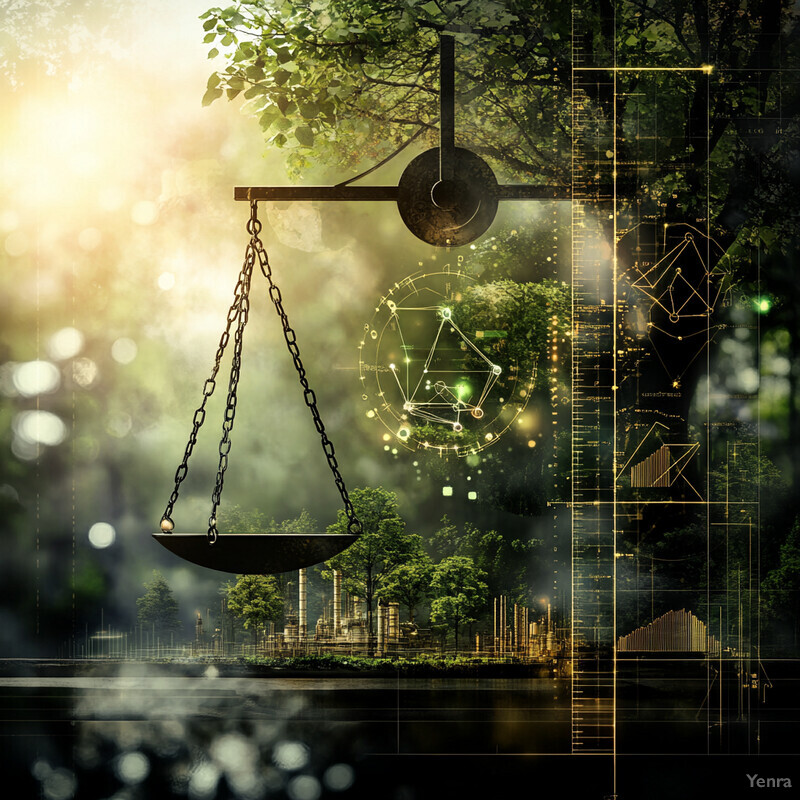
Integrated Assessment Models (IAMs) combine knowledge from economics, energy systems, and climate science to inform policy decisions. AI can enhance these models by linking socio-economic data with complex climate feedbacks more dynamically. Machine learning methods can handle the uncertainty and complexity inherent in these linkages, providing more realistic simulations of how policy actions influence both economic outputs and emissions. In turn, this leads to more informed decisions that balance economic growth, fairness, and emission reduction goals. Over time, AI can continuously refine these IAMs as new data and policies emerge.
15. Better Handling of Big Data
Advanced AI pipelines streamline the ingestion, cleaning, and processing of massive climate and emissions datasets, ensuring that the latest measurements are always informing the models.
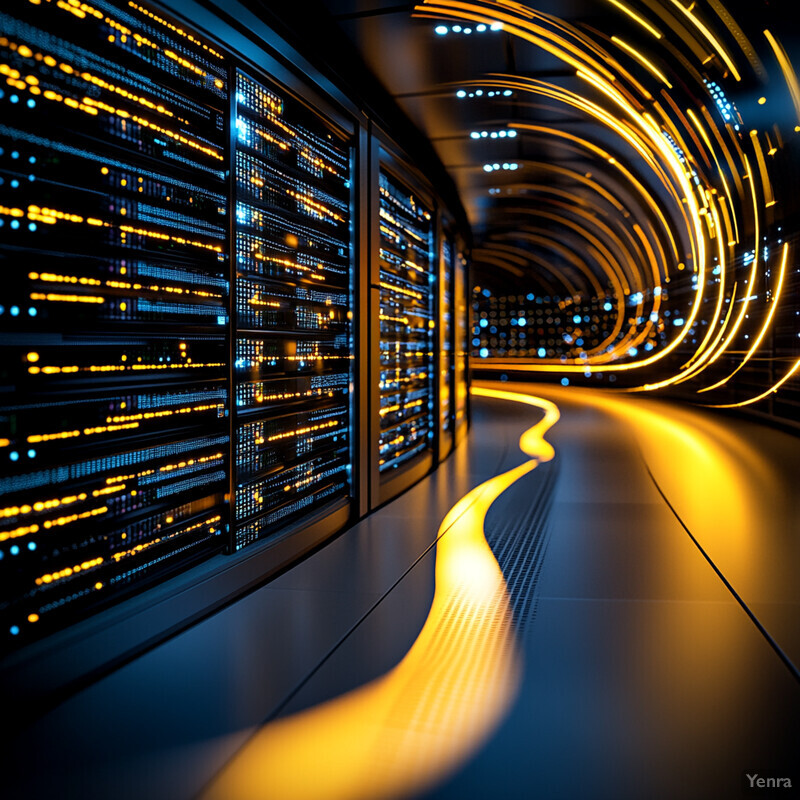
Climate science now operates in an era of big data, with terabytes of information flowing from sensors, research institutions, and international databases daily. AI-driven data pipelines automate the collection, cleansing, and preprocessing of these massive datasets. This ensures that models always use the most up-to-date, high-quality information. Better data handling streamlines research, frees up human experts for deeper analytical work, and enables faster iteration cycles. Ultimately, it results in more accurate and timely emission estimates that support informed policymaking.
16. Improved Calibration and Validation
Machine learning helps calibrate complex simulation models against historical observations more efficiently, adjusting model parameters to produce more accurate future projections.
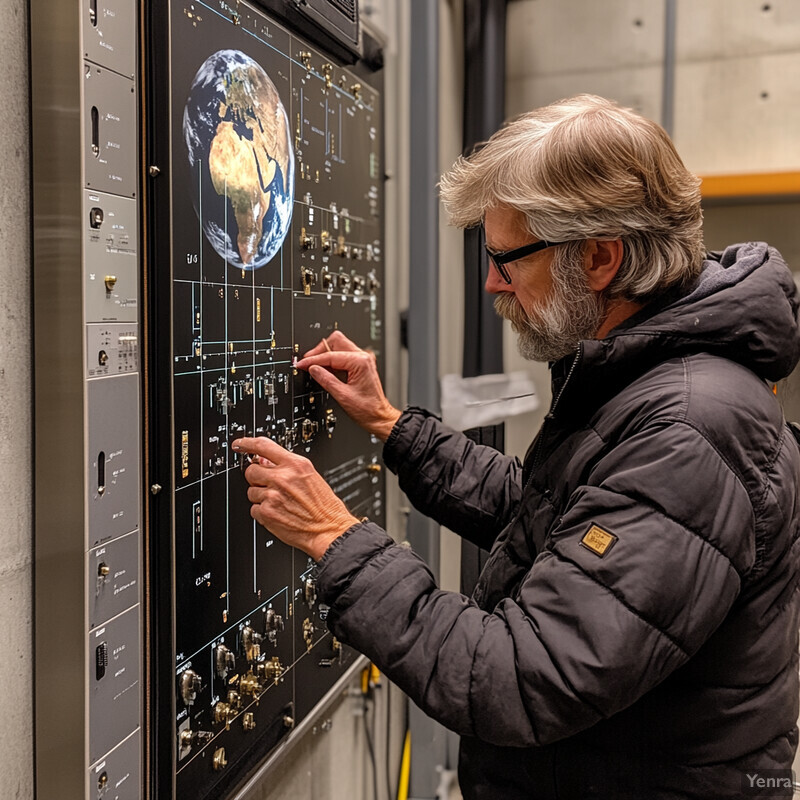
Calibration aligns model outputs with observed data, while validation checks how well a model predicts independent datasets. AI helps by quickly exploring parameter spaces and identifying the best-fit parameters that minimize errors between modeled and measured emissions. Advanced techniques like genetic algorithms or Bayesian optimization guide this calibration more efficiently than traditional trial-and-error methods. Once calibrated, models can be tested against diverse datasets, improving their overall reliability. Rigorous calibration and validation increase stakeholders’ confidence in model outputs, encouraging the use of these tools in high-stakes policy decisions.
17. Sensitivity Analysis and Model Transparency
Interpretability tools can help identify which features most strongly influence emissions estimates, enhancing model transparency and trust among policymakers and stakeholders.
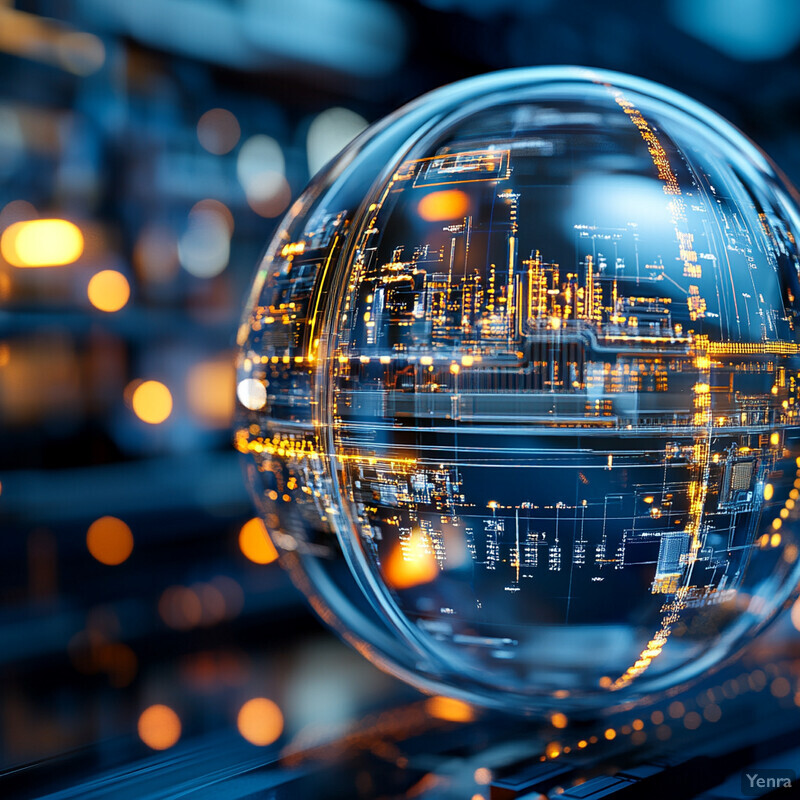
Understanding how sensitive emissions predictions are to changes in inputs is crucial for trust and effective policymaking. AI interpretability methods—such as feature importance rankings and partial dependence plots—reveal which factors most strongly drive the model’s results. This transparency fosters stakeholder confidence by showing that predictions are not “black box” outputs but follow from understandable relationships. Policymakers can then identify levers that offer the greatest impact on emissions and adjust policies accordingly. Enhanced transparency also supports scientific rigor and cross-disciplinary collaboration, improving the credibility of emissions modeling.
18. Early Detection of Policy Impacts
AI-based analysis of policy documents, environmental reports, and socioeconomic indicators can quickly reveal whether certain policies are leading to reductions or increases in emissions.
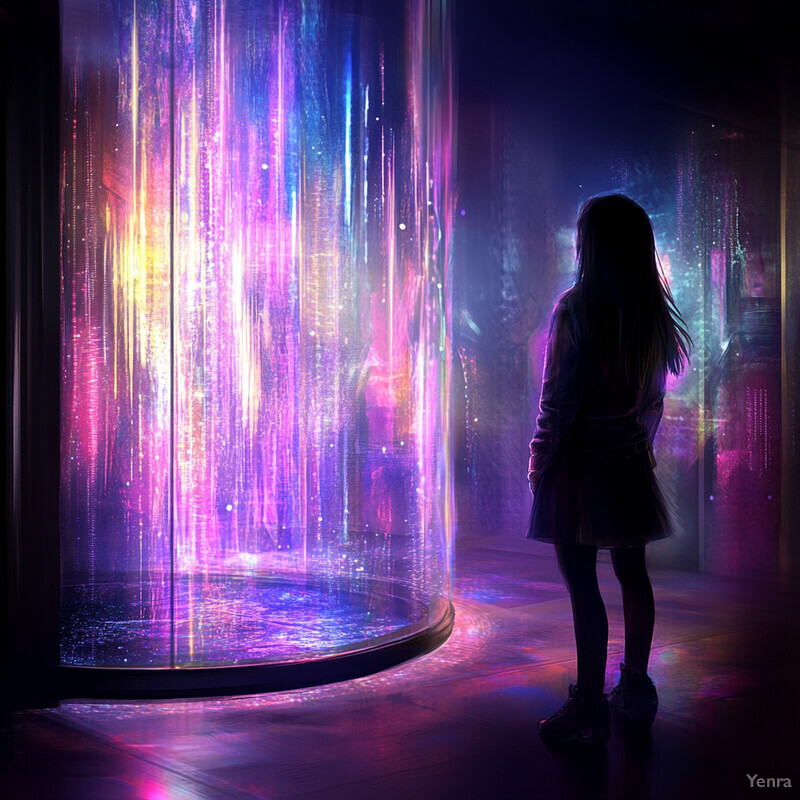
Policies to mitigate GHG emissions—such as carbon pricing or fuel efficiency standards—take time to show measurable effects. AI can track and analyze early indicators from a broad range of data sources—news articles, regulatory filings, economic metrics, and preliminary emissions reports—to detect subtle changes before they show up in official statistics. Early detection helps policymakers refine or correct course rapidly if initial policies are underperforming. It also encourages iterative policy design, where feedback loops ensure continuous improvement in achieving emission reduction goals.
19. Leveraging IoT Sensor Networks
AI can interpret continuous data streams from IoT sensors placed in factories, transportation networks, and agricultural fields, feeding real-time insights into emissions models.
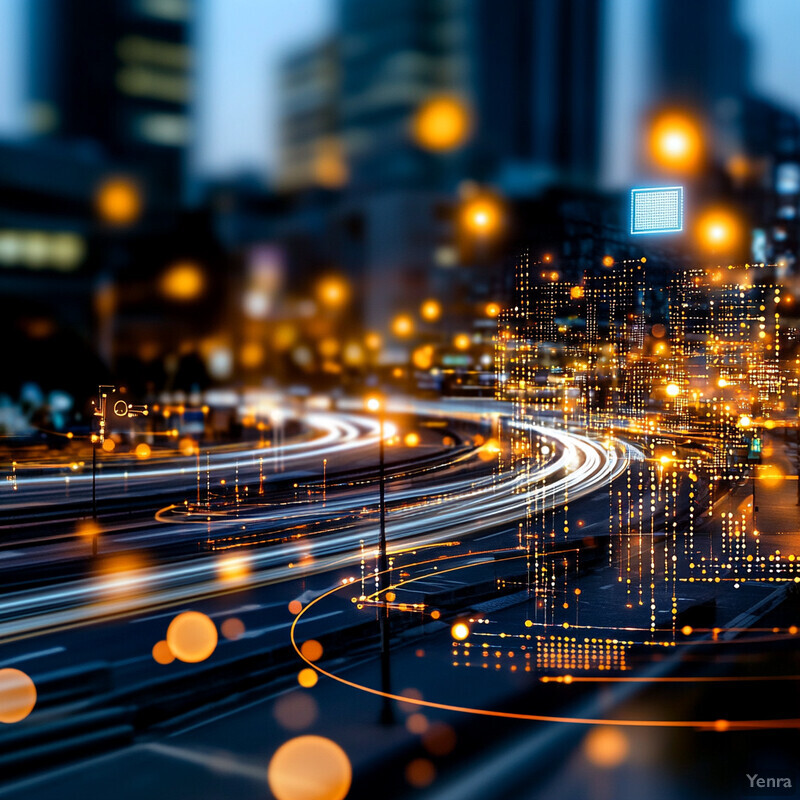
As the Internet of Things (IoT) expands, more sensors measuring CO₂, methane, and other emissions will be deployed in factories, farms, transportation corridors, and even households. AI models can integrate these real-time streams, handling intermittent data and noise gracefully. By providing a holistic, continuous view of emissions, AI-driven IoT platforms enable fine-grained monitoring, pinpointing inefficiencies at their source. This empowers local decision-makers—plant managers, city planners, farm operators—to make immediate improvements. Over time, such bottom-up data feeds into broader models, increasing their accuracy and relevance.
20. Adaptive Models for Rapid Changes
Machine learning approaches can adapt more quickly to unexpected shifts in emission drivers, such as sudden economic downturns or swift policy changes, ensuring models remain accurate and actionable.
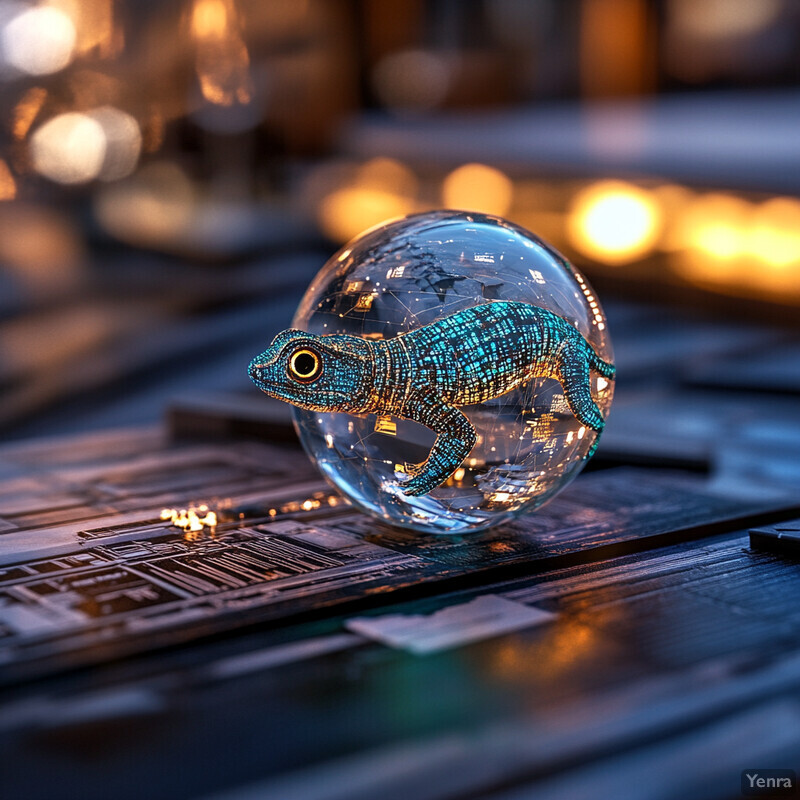
Climate and economic systems are not static, and neither are emission patterns. Sudden events like economic shocks, geopolitical shifts, or extreme weather can dramatically alter emissions trajectories. AI models, particularly those with online learning capabilities, can adapt to these changes quickly. They can update parameters and reevaluate relationships as new data enters the system, maintaining model relevance and reliability. Adaptive modeling ensures that predictions and policy guidance remain up-to-date in a rapidly evolving world, supporting more responsive and effective emissions management over the long term.