1. Enhanced Data Integration
AI can ingest and harmonize vast volumes of heterogeneous data sources—such as historical freight rates, port throughput, vessel schedules, trade statistics, weather data, and geopolitical news—into a single forecasting model. This creates more comprehensive, accurate foundations for predicting future shipping costs.
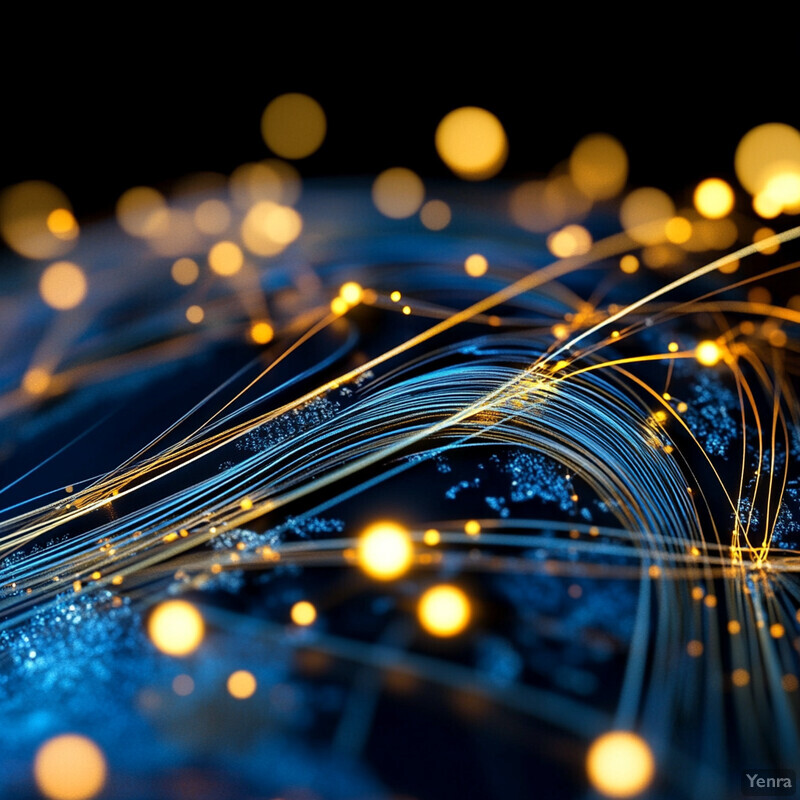
AI-driven freight price forecasting solutions can gather and harmonize massive, disparate sets of data from multiple sources, including historical freight rate databases, port throughput logs, vessel movement records, global trade statistics, and near-real-time satellite imagery of maritime traffic. By using advanced extraction, transformation, and loading (ETL) techniques combined with machine learning methods for data cleaning and feature engineering, AI models can unify these heterogeneous inputs into coherent data structures. This comprehensive data integration reduces gaps, inconsistencies, and noise, ultimately providing analysts and decision-makers with a single source of truth. Such a robust data foundation empowers more reliable forecasts, as the predictive models can consider a wide range of factors that influence pricing—ranging from macroeconomic indicators to granular operational metrics—improving the accuracy and stability of the final predictions.
2. Real-Time Market Monitoring
Advanced machine learning tools can continuously monitor real-time indicators—like current spot rates, commodity price shifts, vessel congestion levels, and container availability—to adjust projections dynamically. This ensures that forecasts remain responsive to rapidly changing market conditions.
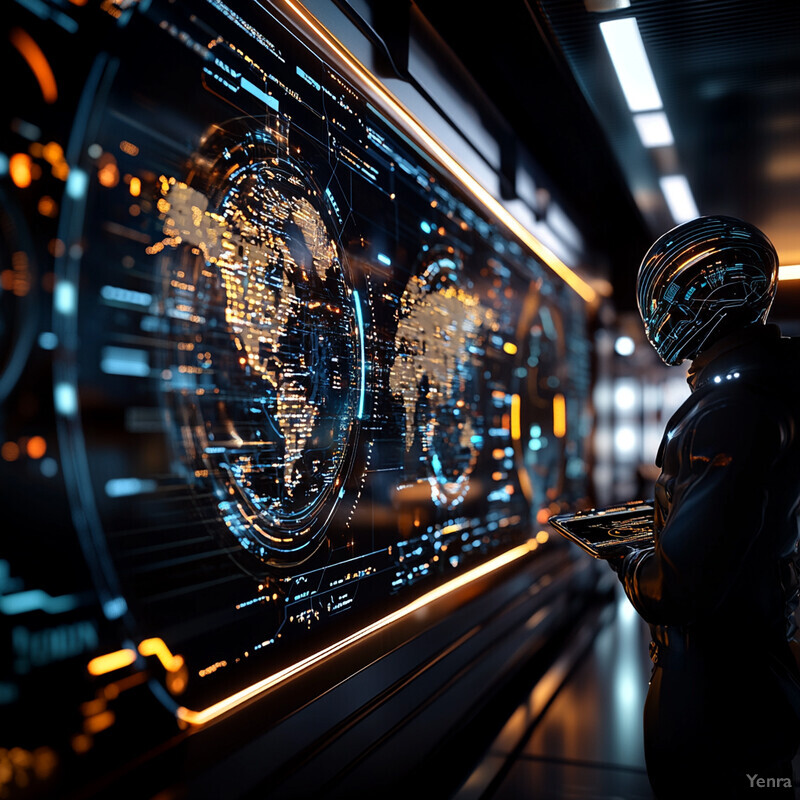
Traditional forecasting approaches often rely on static, historical datasets that struggle to remain relevant in dynamic market environments. AI surmounts this limitation by continuously monitoring real-time indicators that directly affect freight prices. These include spot freight rate indices, global commodity price feeds, vessel congestion statistics, port operational efficiency metrics, and relevant geopolitical events unfolding on news and social media platforms. Machine learning algorithms process and interpret these real-time signals to adjust predictions on-the-fly, making forecasts more agile. In practice, this agility allows supply chain planners to detect emerging trends or disruptions—such as sudden spikes in demand or a port strike—early enough to adapt capacity planning, adjust procurement strategies, and potentially lock in more advantageous freight rates in rapidly changing conditions.
3. Improved Forecasting Accuracy via Deep Learning
Neural networks, especially deep learning architectures like LSTM and Transformer models, can detect intricate, nonlinear patterns in complex freight market data. This leads to more accurate and robust long-term price predictions than traditional statistical models.
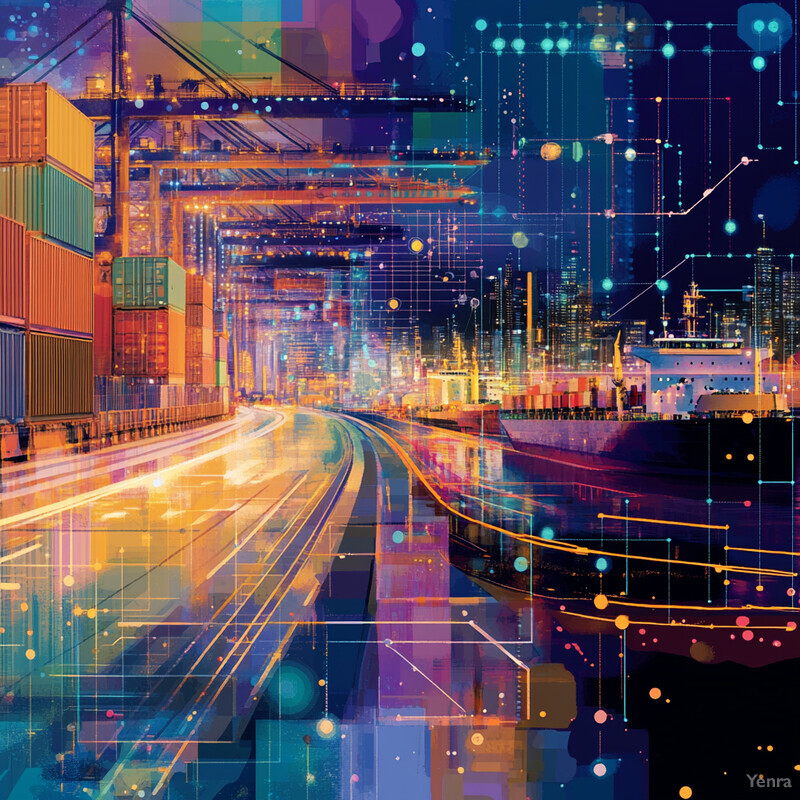
Deep learning architectures, particularly those designed for time series analysis—like LSTM (Long Short-Term Memory) networks and Transformers—excel at modeling complex, non-linear relationships present in freight markets. Unlike traditional econometric models that may falter when patterns shift or when data relationships become multifaceted, deep learning approaches can uncover hidden correlations and subtle seasonalities. By learning from extensive historical data, these models understand intricate market dynamics and can interpolate across missing data points, handle a broad range of inputs, and fine-tune their parameters as more data becomes available. This results in substantially improved forecasting accuracy, enabling stakeholders to predict future freight rates with higher confidence and plan more strategically for upcoming shipping cycles.
4. Scenario Analysis with Simulation Models
AI-driven simulation models can run millions of "what-if" scenarios—such as sudden port closures, trade policy shifts, or supply chain shocks—to predict how freight rates might evolve. This gives shippers and forwarders greater confidence in contingency planning.
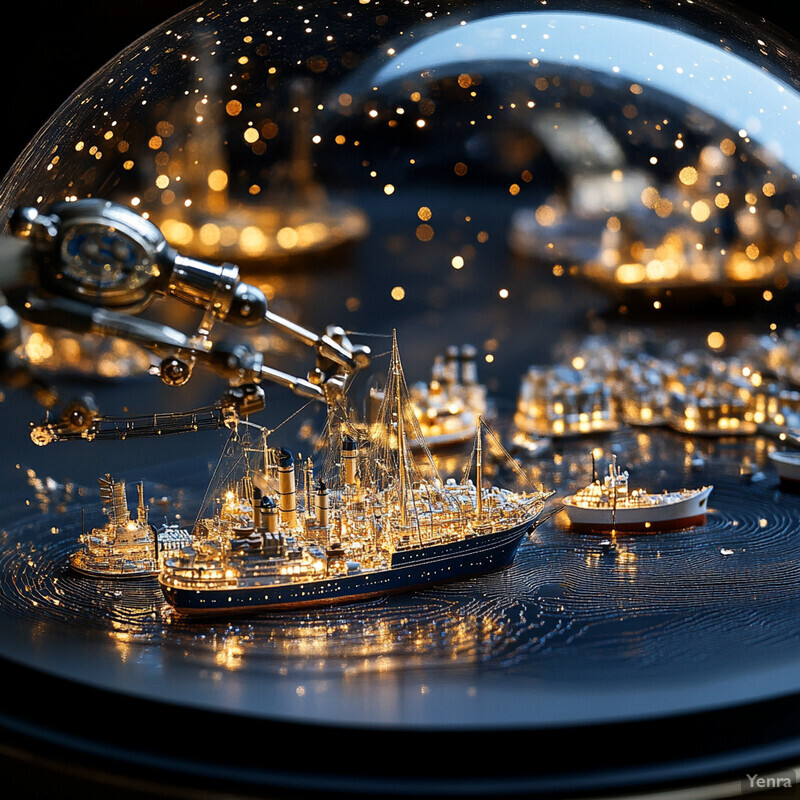
Global freight markets are influenced by a dizzying array of variables, many of which can change unexpectedly—extreme weather events, policy changes, logistical bottlenecks, or sudden economic downturns. AI simulation models can run thousands or even millions of “what-if” scenarios to forecast how freight prices might respond to these hypothetical conditions. By systematically altering input parameters—like fuel costs, trade route restrictions, or container availability—simulation models can provide probability distributions of potential outcomes. Businesses benefit from such scenario analyses by identifying robust strategies that perform well across a range of plausible futures. This foresight is crucial for risk management, contingency planning, and strategic decision-making in volatile shipping environments.
5. Advanced Anomaly Detection
Machine learning algorithms can spot unusual patterns or anomalies in freight pricing—like sudden spikes or drops due to unexpected events—and alert stakeholders. Early detection of anomalies helps companies respond faster and potentially secure more favorable freight contracts.
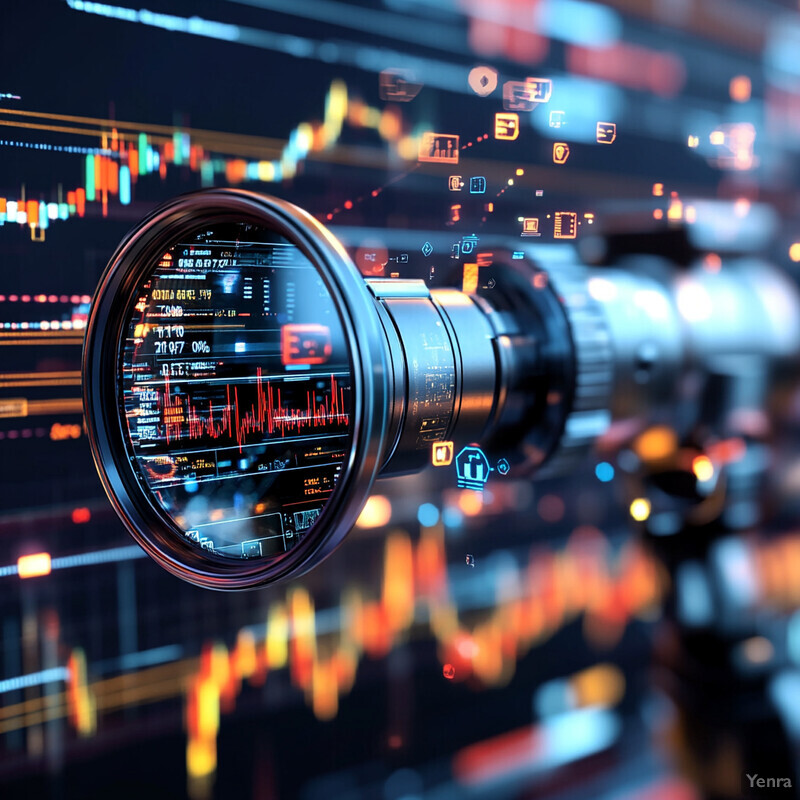
Freight markets sometimes experience price shifts that are out of line with historical patterns. Such anomalies may occur due to sudden disruptions—port closures, labor strikes, commodity shortages, or political unrest. AI algorithms trained in anomaly detection can flag these unusual price movements early, alerting stakeholders to conditions that demand immediate attention. These methods leverage statistical thresholds, clustering techniques, and machine learning-driven pattern recognition to differentiate between normal market fluctuations and legitimate deviations. Early detection empowers logistic managers to investigate underlying causes, deploy corrective actions, adjust booking strategies, and potentially secure capacity before prices move unfavorably. Over time, building a robust anomaly alerting system reduces surprise costs and helps maintain more stable and predictable shipping budgets.
6. Sentiment and News Analysis
Natural Language Processing (NLP) allows AI systems to analyze news reports, social media, and industry publications for market sentiment, policy changes, or disruptions. This sentiment data is then integrated into pricing forecasts, providing a more holistic view of future trends.
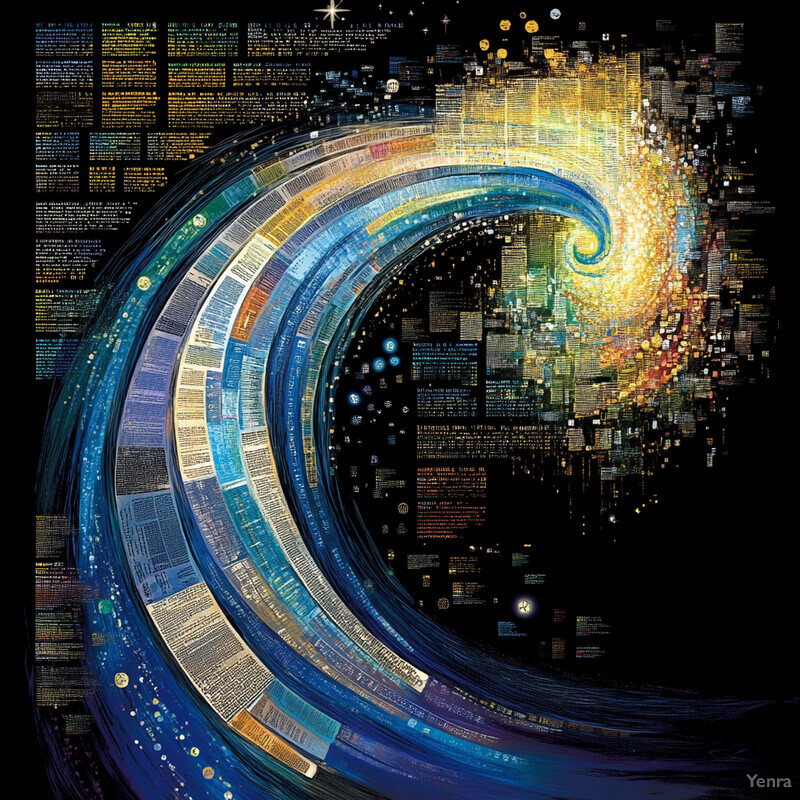
Freight prices are partially driven by expectations—market sentiment often shifts based on global political climates, trade policies, and industry buzz. AI systems equipped with Natural Language Processing (NLP) capabilities can continuously scan news outlets, social media feeds, trade journals, and analyst reports to gauge market mood. By extracting entities, topics, and sentiment scores from unstructured text, these models infer how emerging narratives might influence freight supply and demand. For instance, reports of factory shutdowns in a major manufacturing hub or speculation about environmental regulations affecting shipping lanes can be integrated into pricing forecasts. This enriched intelligence allows forecasters to anticipate market shifts before they fully materialize in numerical indices, resulting in forecasts that better mirror the real state of global trade psychology.
7. Predictive Maintenance Insights
By analyzing vessel and infrastructure data, AI can forecast maintenance-related delays or operational issues at ports and terminals. Knowing when such disruptions may occur allows forecasters to adjust expected freight availability and prices accordingly.
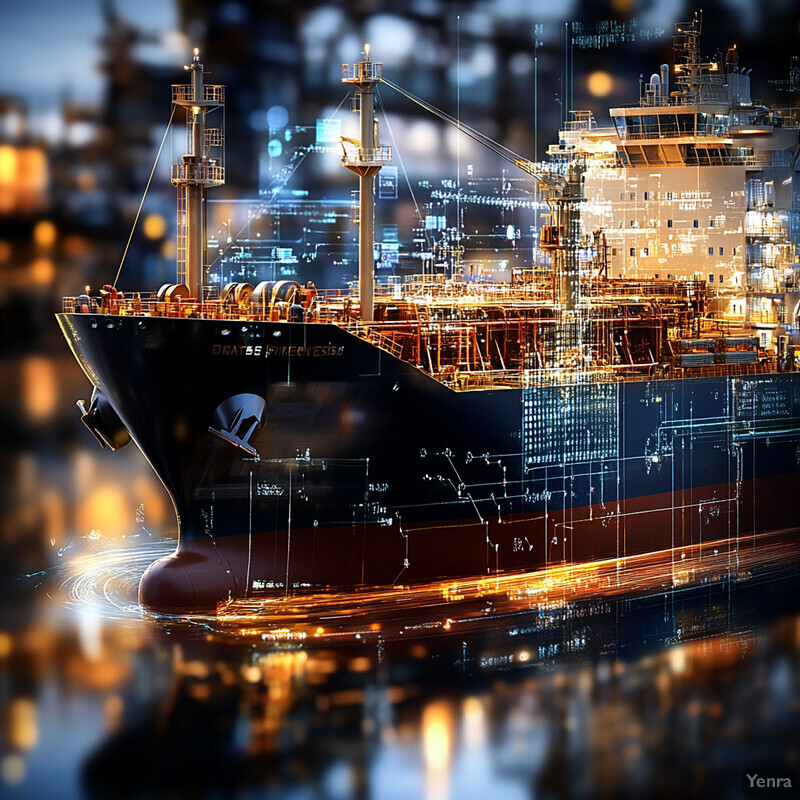
Freight capacity and operational efficiency are directly linked to the condition and availability of vessels, cranes, trucks, and port infrastructure. AI models that incorporate predictive maintenance insights can forecast when critical equipment is likely to fail or require downtime. Such predictions rely on analyzing machine sensor data, maintenance logs, and performance metrics to estimate the health of key assets. By understanding when maintenance may disrupt terminal throughput, slow vessel turnaround times, or reduce the number of ships in service, forecasters can anticipate shortages or surpluses of freight capacity and adjust future rate predictions accordingly. This proactive approach avoids unforeseen rate volatility by ensuring that potential operational constraints are factored into pricing forecasts well in advance.
8. Automated Risk Assessment
AI models can incorporate political and economic risk metrics, factoring in sanctions, tariffs, and other trade barriers. By quantifying these risks, freight price forecasting becomes more resilient to external shocks and better aligned with global risk landscapes.
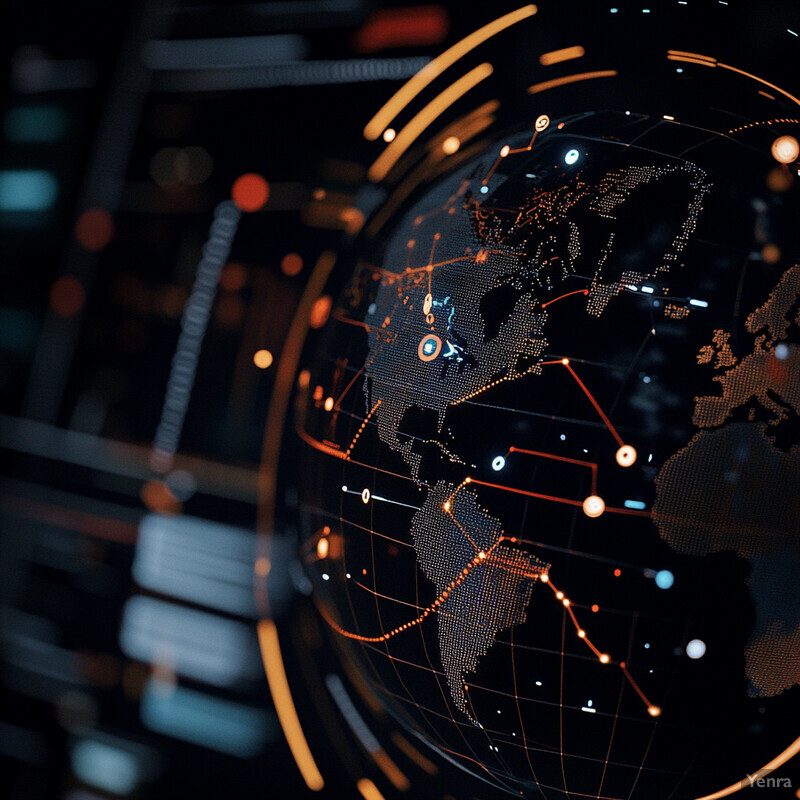
Global freight markets are subject to a wide range of risks—geopolitical instability, currency fluctuations, trade wars, and sanctions. AI-based risk assessment tools integrate macroeconomic indicators, political risk indexes, and market volatility metrics into forecasting models. They use sophisticated algorithms to estimate the probability and potential impact of disruptive events on future freight prices. By incorporating these risk factors, pricing forecasts become more nuanced and robust, reflecting not only baseline market fundamentals but also the sensitivity of those prices to broader uncertainties. Stakeholders can use these insights to develop hedging strategies, diversify route options, or secure longer-term contracts to mitigate identified risks.
9. Granular Route-Level Forecasting
Instead of general market averages, AI enables highly specific forecasting at the route, port pair, or even vessel level. This granular view helps logistics planners identify more precise opportunities for cost savings and reduce uncertainty in complex supply chains.
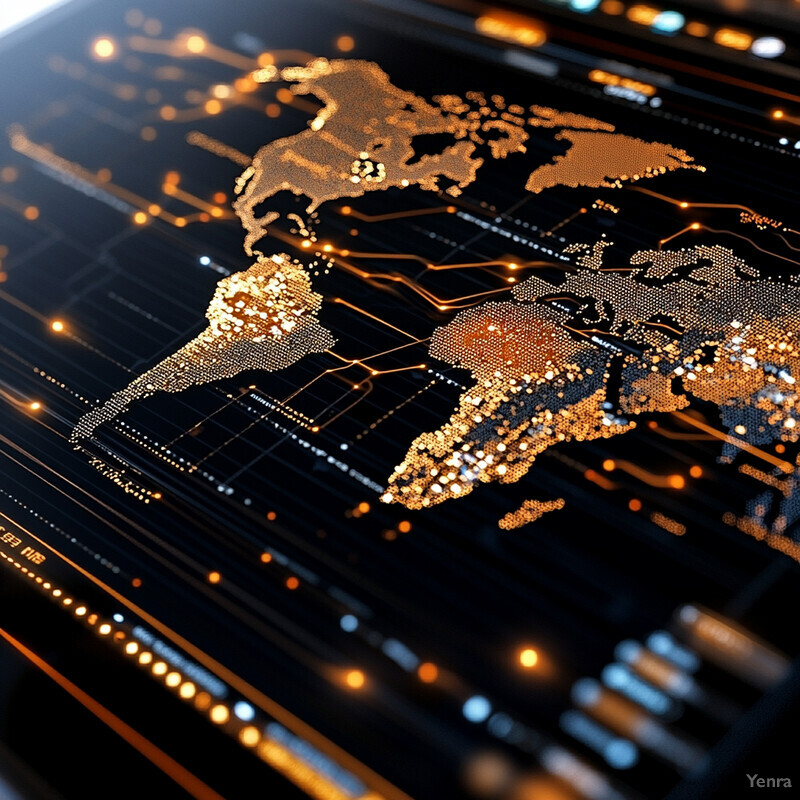
Traditional freight forecasting often provides aggregate or broad market-level insights that may not suffice for finely tuned logistics planning. AI models enable forecasts at a highly granular level, including specific trade lanes, port-pairs, or even individual vessel routes. Such fine-grained predictions capture local congestion patterns, region-specific regulations, and port operational differences. These disaggregated forecasts inform more nuanced decisions—like when to reroute cargo, which carriers to engage, or which ports offer the best balance of cost and reliability. Over time, access to detailed route-level forecasts can significantly improve operational efficiency, reduce demurrage and detention fees, and enhance overall supply chain resilience.
10. Dynamic Demand Forecasting
Integrating AI demand forecasting with freight price predictions helps capture the interplay between shifting consumer demand patterns, inventory cycles, and shipping capacity. This synergy leads to more accurate rate projections tied directly to market fundamentals.
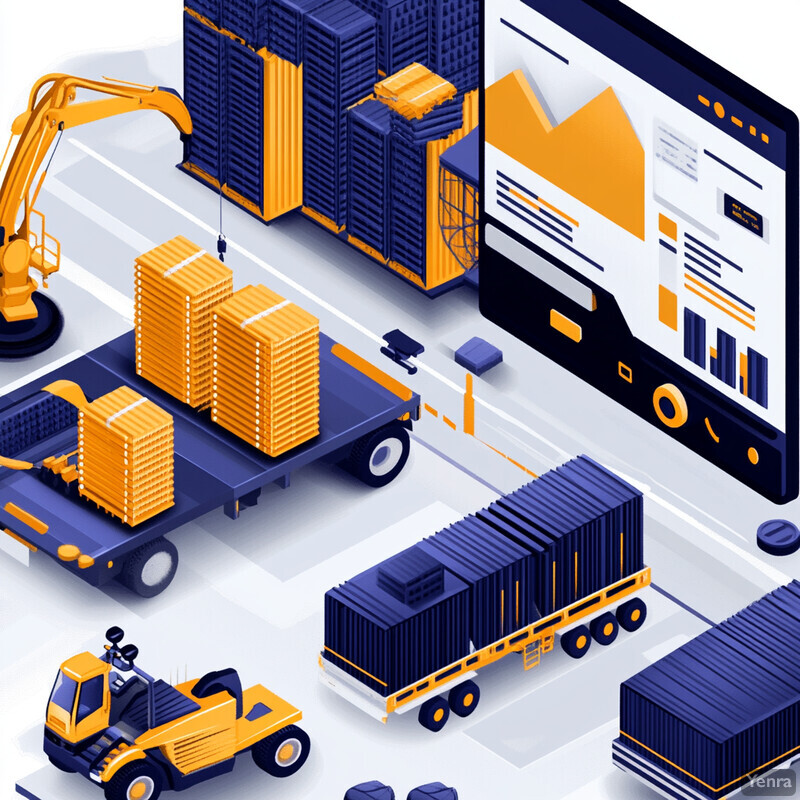
Understanding freight prices requires understanding not just supply constraints but also shifting patterns of demand. AI can integrate advanced demand forecasting—leveraging historical sales, consumer trends, inventory cycles, and even climate patterns that influence agricultural output—into freight price predictions. By linking anticipated demand fluctuations to freight market capacity and route availability, these integrated models produce more accurate rate forecasts that align with real market conditions. The synergy between demand and pricing forecasts helps operators optimize booking strategies, plan inventory replenishment more strategically, and align transportation choices with expected future costs, improving profitability and minimizing stockouts or overstock situations.
11. Automated Price Benchmarking
AI can continuously benchmark forecasted prices against industry standards, competitor rates, and historical averages. This automated benchmarking ensures that stakeholders maintain visibility into whether projected prices are competitive or out of line with market norms.
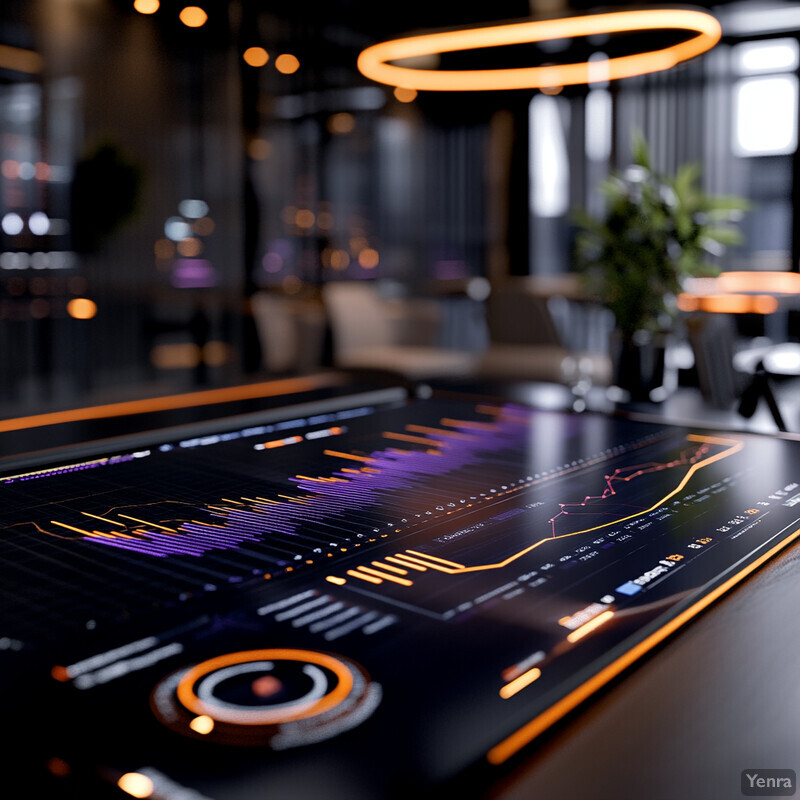
In a global market where freight rates vary by carrier, region, season, and contract terms, maintaining a clear sense of whether offered rates are fair or competitive can be challenging. AI-powered solutions automatically compare forecasted prices against historical averages, current spot rates, peer benchmarks, and relevant indices. They identify deviations, highlight how evolving conditions compare to past trends, and pinpoint opportunities for more cost-effective shipping solutions. This benchmarking process ensures that shippers and forwarders can confidently evaluate pricing proposals, negotiate contract terms, and select carriers based on objective, data-driven insights rather than guesswork or outdated reference points.
12. Adaptive Models with Feedback Loops
AI freight forecasting models often incorporate feedback mechanisms. As they make predictions and receive updated actual rate data, they learn from their forecasting errors, gradually improving accuracy and reliability over time.
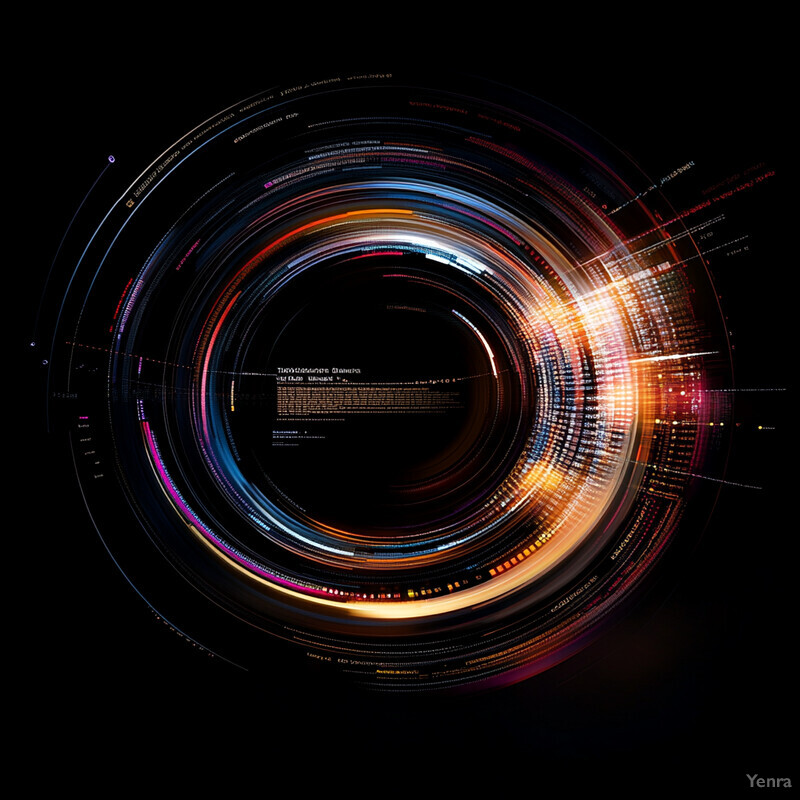
One of AI’s greatest strengths is its ability to learn and improve over time. Freight forecasting models with feedback loops ingest recent market outcomes—actual prices paid, realized transit times, newly observed congestion patterns—and compare them to predicted values. This feedback allows the models to adjust their parameters, refine their features, and correct errors. Over iterative cycles, the predictions grow more precise, fostering a self-improving ecosystem of analytics. Ultimately, this virtuous feedback cycle ensures that models stay current, adapt to structural changes in trade routes, account for evolving fleet compositions, and remain resilient against shifts in demand or geopolitical climates.
13. Integration with Supply Chain Control Towers
AI-driven freight forecasts can feed into integrated supply chain control towers, enabling end-to-end visibility of costs. This creates a strategic advantage by aligning procurement, warehousing, and logistics decisions with expected freight fluctuations.
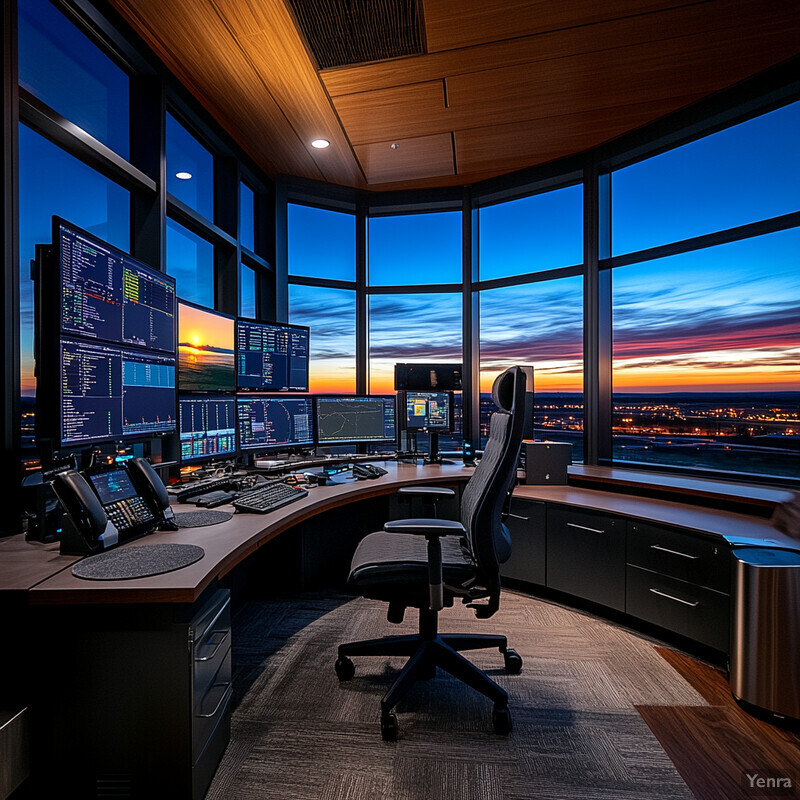
Modern supply chains often operate through digital “control towers” that provide a centralized view of inventory, procurement, transportation, and distribution. By feeding AI-driven freight forecasts directly into these platforms, companies achieve synchronized visibility and decision support across their entire operation. Freight price forecasts can influence purchasing schedules, storage strategies, and distribution center allocations. When aligned with other supply chain signals—like inventory management systems or demand forecasts—companies can optimize their logistics holistically. Such integration promotes cost savings, increases agility, reduces waste, and ensures that strategic decisions reflect both current and projected logistics market conditions.
14. Commodity Price Correlation Analysis
AI can identify subtle correlations between freight rates and commodity price movements. These insights help market participants anticipate how changing raw material prices or energy costs might drive up or pull down shipping costs.
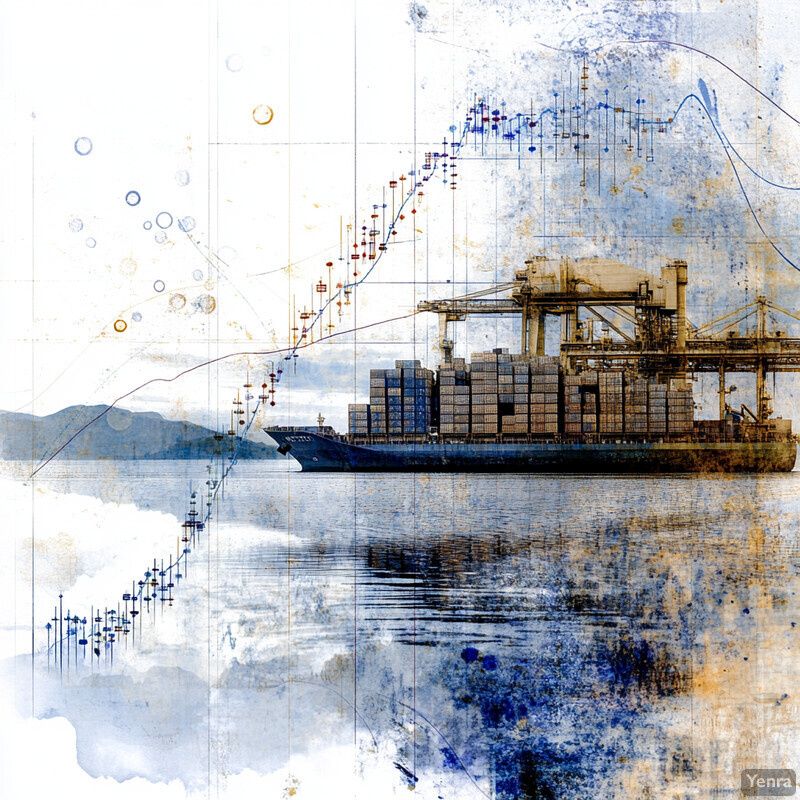
Freight costs are often correlated with commodity prices, energy costs, and raw material availability. AI can analyze massive datasets to detect subtle relationships between freight rates and these underlying economic drivers. By understanding how changes in commodity prices ripple through the transportation network—raising or lowering shipping demand—forecasts become more accurate and timely. For instance, a spike in the price of an essential manufacturing input may soon translate to increased shipments or route changes. Uncovering these correlations empowers decision-makers to anticipate freight rate adjustments and to develop proactive strategies—like securing capacity or renegotiating contracts—before shifts materialize in the wider market.
15. Predicting Seasonal and Cyclical Patterns
Many freight markets exhibit seasonal trends or cyclical behavior. Machine learning models, trained on multi-year historical data, can capture these complex cycles, providing more dependable seasonal forecasts and capacity planning recommendations.
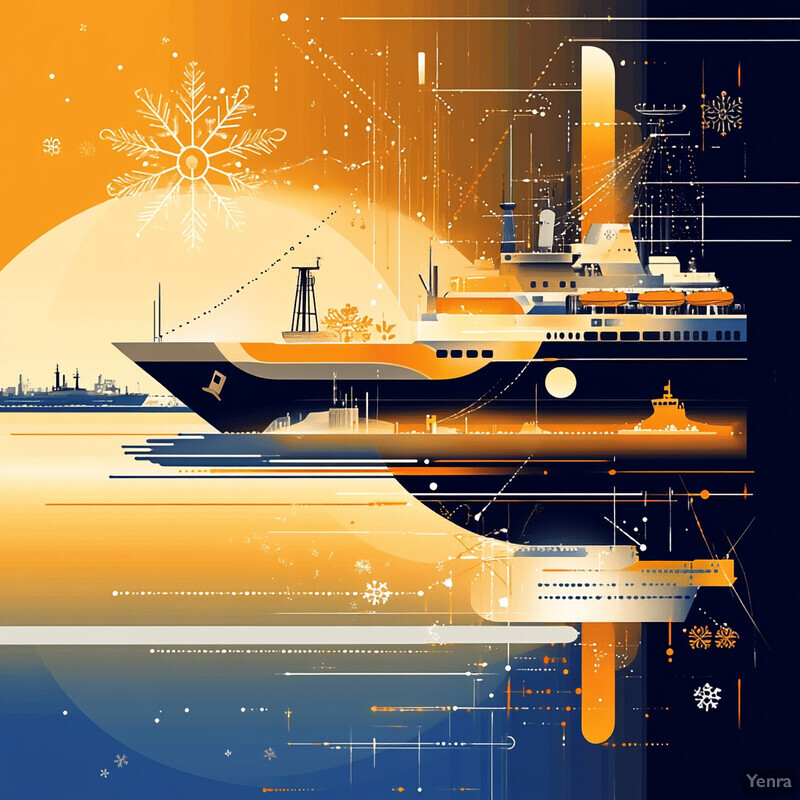
Freight markets often exhibit seasonal peaks and troughs, influenced by harvest cycles, holiday shopping periods, and global production schedules. AI models, trained on multi-year historical data, can learn these seasonal rhythms and anticipate cyclical upswings or downswings in freight prices. Moreover, they can differentiate between normal seasonality and unusual patterns caused by external shocks. This predictive capacity enables firms to plan ahead, adjusting inventory levels, reevaluating carrier contracts, and timing shipments to capitalize on favorable rates. By preempting seasonal fluctuations, companies reduce costs, enhance customer satisfaction, and maintain more stable supply chain operations throughout the year.
16. Optimized Contract Negotiations
Armed with high-quality AI-driven forecasts, shippers and forwarders can enter contract negotiations with carriers on firmer ground. Predictive insights help them lock in more favorable long-term freight rates and reduce exposure to volatile spot markets.
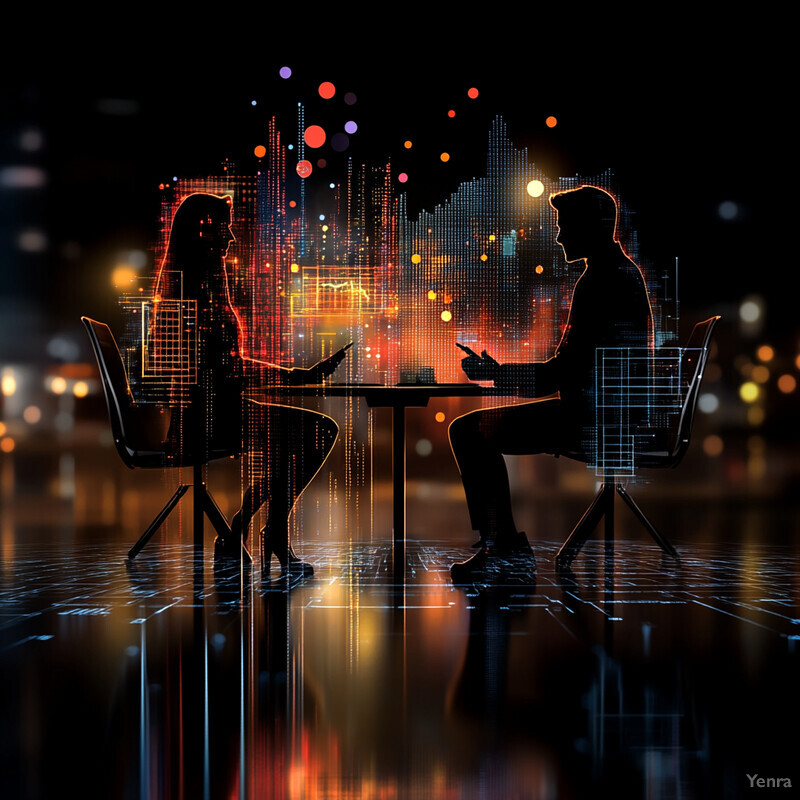
Armed with reliable AI-driven forecasts, shippers and freight forwarders can approach contract negotiations with more confidence and sophistication. Instead of relying on outdated or generic industry benchmarks, they can present carriers with data-backed projections of future market conditions. Such insights help secure terms that anticipate future rate environments, lock in capacity at competitive rates, and structure longer-term agreements that hedge against expected volatility. Over time, these optimized negotiations can yield better overall pricing, smoother supplier relationships, and a reduced risk of last-minute spot market surges that erode profit margins.
17. Network Effect Insights
AI can assess how changes in one part of the transportation network (e.g., a port strike in Asia) cascade through global shipping lanes, eventually affecting freight rates in Europe or the Americas. Modeling these network effects leads to more globally aware pricing forecasts.
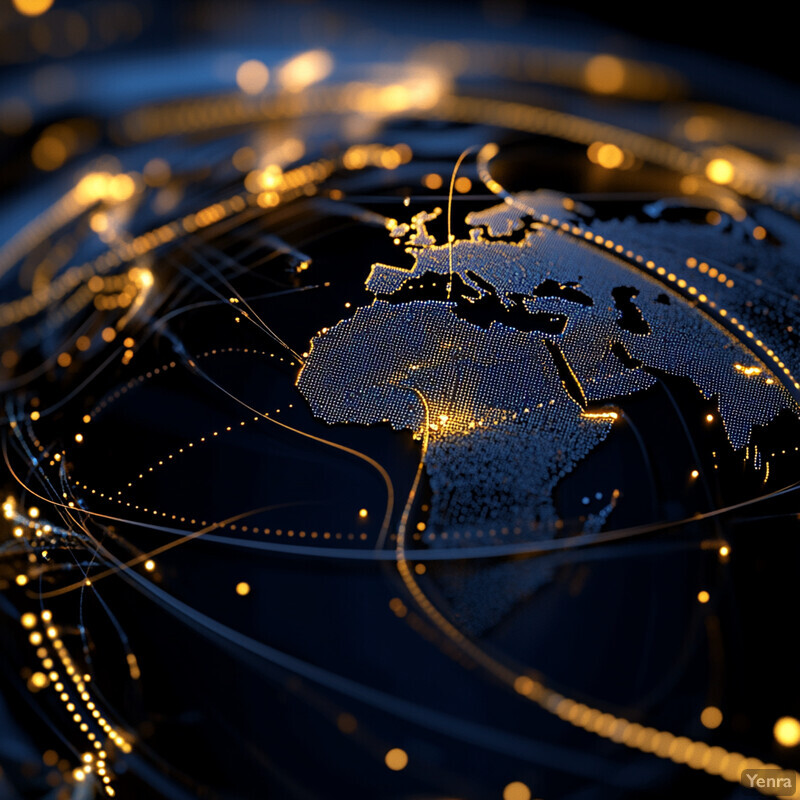
Global freight isn’t simply a collection of independent routes; it’s an interconnected network where changes in one region reverberate throughout the world. AI can model these network effects, showing how a port closure in Asia might cascade into higher rates in Europe or disrupt transatlantic supply chains. By simulating network-level dynamics and quantifying the interplay among different nodes and edges, these advanced models help shippers identify bottlenecks, alternative routing options, and strategic fallback plans. Understanding these complex ripple effects empowers decision-makers to build more resilient and agile supply networks, avoiding potential capacity crunches and minimizing the impact of localized disruptions.
18. Sustainability and Emissions Forecasting Integration
As regulators introduce emissions standards and environmental policies, AI models that factor in sustainability constraints can predict how greener shipping requirements might influence freight capacity, operational costs, and ultimately shipping rates.
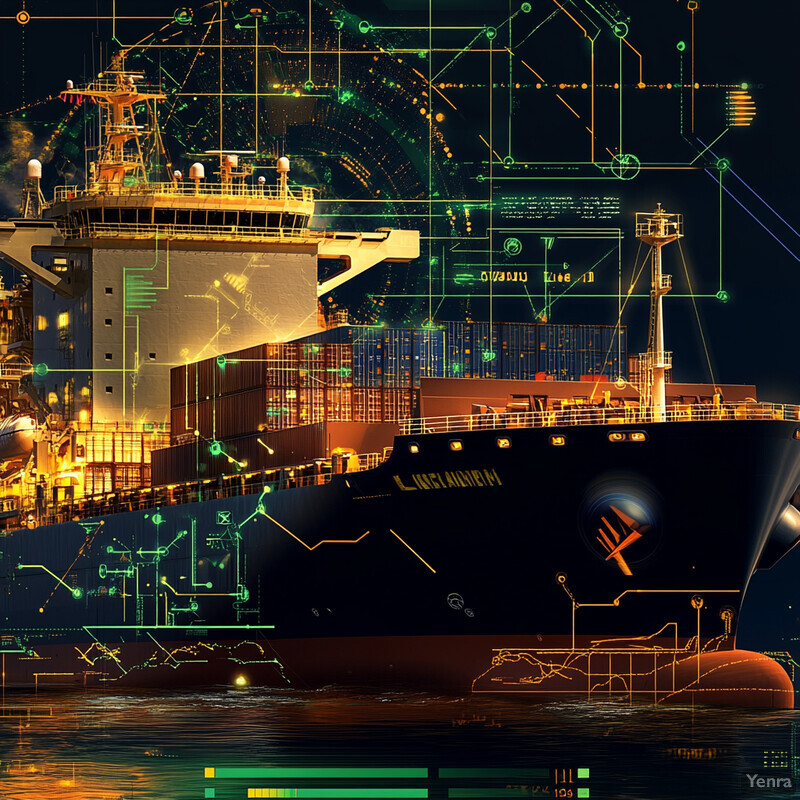
As environmental regulations tighten and sustainability becomes a strategic imperative, freight forecasting can no longer ignore the cost implications of greener shipping methods. AI-powered models can integrate emissions data, fuel consumption patterns, low-sulfur fuel mandates, and carbon pricing mechanisms into their predictions. By anticipating how cleaner propulsion technologies, route optimization for fuel efficiency, and evolving environmental policies will affect freight capacity and operational costs, these models deliver forecasts that reflect the future landscape of sustainable logistics. Companies benefit by aligning their supply chain strategies with regulatory trends, preparing for shifting market expectations, and potentially leveraging their green initiatives to secure favorable shipping terms.
19. Algorithmic Trading and Freight Rate Derivatives
In emerging freight derivatives markets, AI models help traders anticipate price moves for forward freight agreements (FFAs). This advanced forecasting informs hedging strategies and stabilizes budgeting for shipping costs over time.
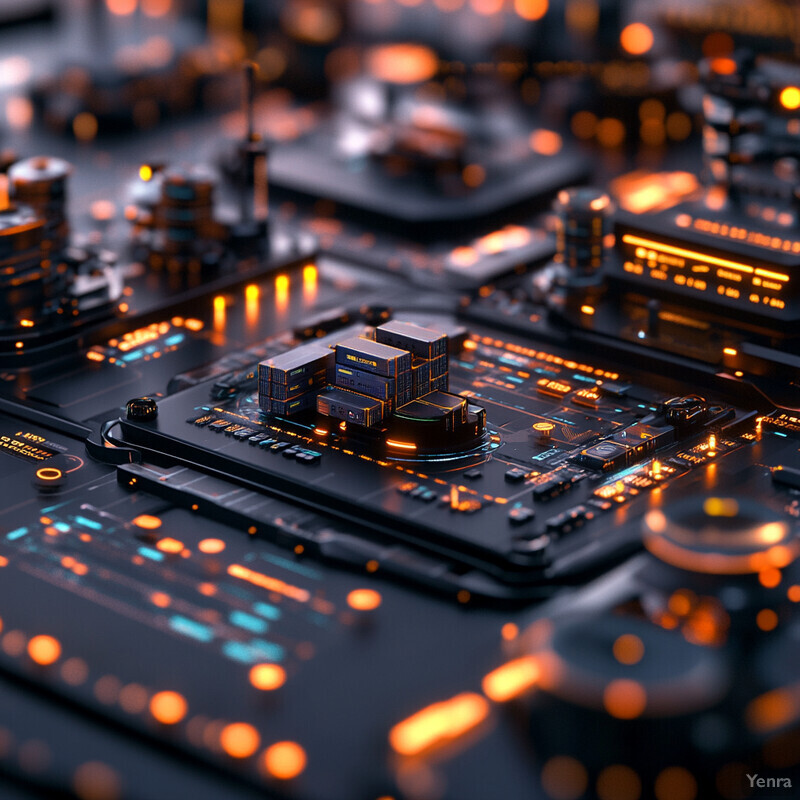
The freight market is increasingly financialized, with the emergence of forward freight agreements (FFAs) and other derivatives products designed to hedge shipping cost risks. AI models that excel at predicting price movements can inform algorithmic trading strategies and risk management decisions. By analyzing historical price movements, contract settlement patterns, and correlating macroeconomic indicators, these algorithms can identify profitable entry and exit points or suggest dynamic hedging positions. Shippers, carriers, and investors benefit from more stable budgeting processes, reduced uncertainty, and smoother financial planning. Over time, improved forecasting accuracy can help create more liquid and stable freight derivatives markets, ultimately enhancing the entire industry’s ability to manage volatility.
20. Continuous Model Refinement with Reinforcement Learning
Some state-of-the-art AI approaches leverage reinforcement learning to continuously refine pricing strategies. By simulating different actions—like when to secure capacity or how to distribute cargo—and receiving performance feedback, these systems improve their freight price forecasting acumen iteratively.
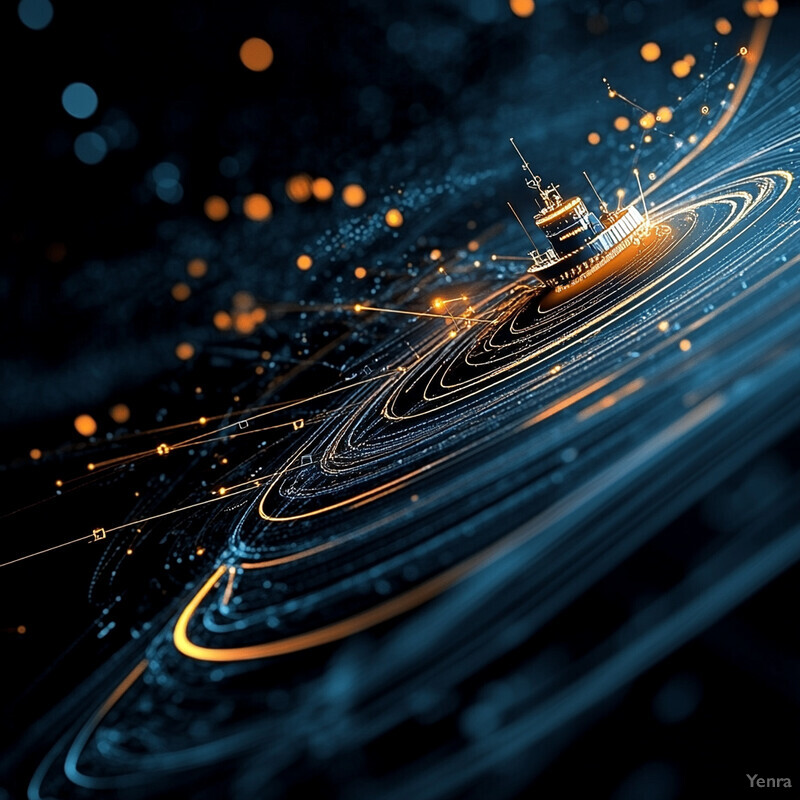
Beyond traditional supervised learning methods, some cutting-edge AI approaches use reinforcement learning (RL) to refine freight pricing strategies. RL models treat the forecasting and decision-making process like a game, experimenting with different actions (such as securing early capacity or altering routing patterns) and receiving feedback in the form of cost savings or on-time delivery improvements. Through iterative trial-and-error, the models learn strategies that yield better outcomes over time. This continuous refinement ensures that the system not only improves the accuracy of freight price predictions but also learns to propose actionable steps that help organizations capitalize on their forecasts, making freight pricing and logistics operations more efficient, adaptive, and intelligent.