1. Markerless Motion Capture
Artificial intelligence is enabling gait analysis without physical markers or specialized labs. Advanced computer vision algorithms can identify key body points from video, allowing clinicians to measure gait kinematics in everyday settings. Studies show these markerless systems can achieve accuracy comparable to traditional marker-based motion labs. Because no reflective markers or suits are needed, gait assessments become more accessible, faster to set up, and less intrusive for patients. This innovation means therapists can evaluate walking patterns in clinics or at home with just cameras, expanding who can benefit from professional gait analysis. Overall, AI-driven markerless motion capture is making gait analysis more patient-friendly and widely available.
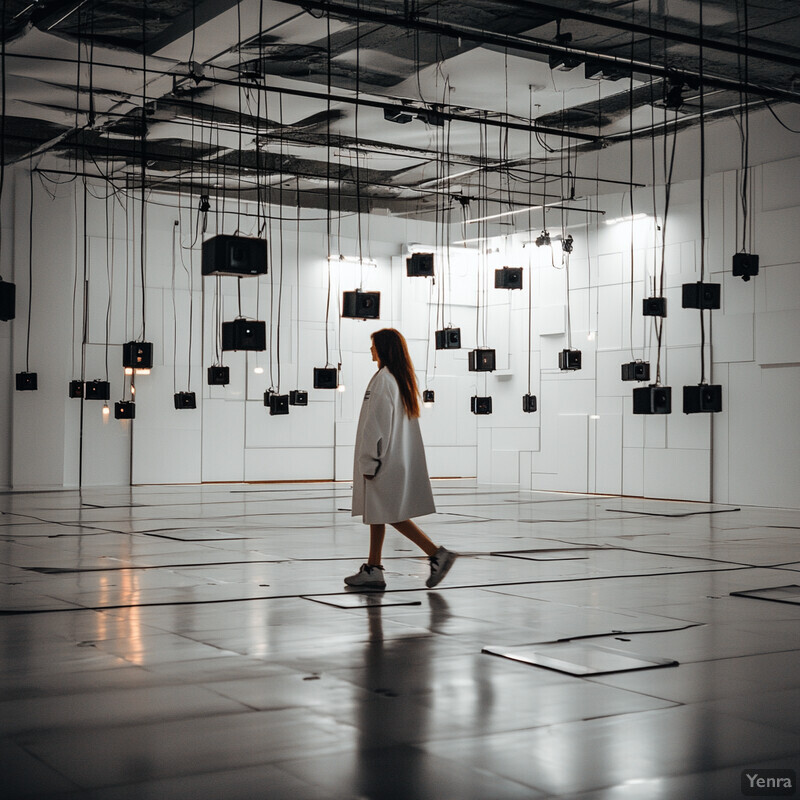
Recent research confirms that AI-powered markerless gait tracking is reliable and precise. For example, a 2025 study validated a markerless system (using deep neural networks for 3D pose estimation) against a gold-standard marker-based system and found high agreement in joint angles and spatiotemporal measures. Open-source platforms like OpenCap use smartphone cameras and AI algorithms to reconstruct 3D gait metrics, achieving low error rates (often under 5-8% difference from lab systems) in stride length and joint motion. Because these systems capture human movement from ordinary video, gait can be analyzed outside the lab – one group demonstrated accurate gait assessments using just two smartphones, with results strongly correlating to traditional motion capture. These advances indicate that markerless AI motion capture can provide clinical-grade gait data in everyday environments, a major step toward routine gait monitoring in rehabilitation.
2. Real-Time Feedback and Biofeedback Integration
Machine learning is enabling real-time gait feedback to patients during therapy. AI algorithms can analyze a person’s walking pattern on the fly and immediately cue them to adjust movements. This means that as a patient walks (for instance, on a treadmill), the system can highlight posture errors or asymmetries in real time and provide corrective prompts – visually, through sound, or via vibrations. Research in stroke and Parkinson’s disease confirms the benefits: real-time auditory or tactile feedback during gait training can significantly improve gait speed, stride length, and symmetry by helping patients correct their form instantly. By closing the loop quickly, these AI-driven feedback systems accelerate motor learning, reinforce proper walking techniques, and keep patients engaged with interactive cues. Overall, integrating instant biofeedback into gait therapy leads to quicker improvements and better outcomes in rehabilitation.
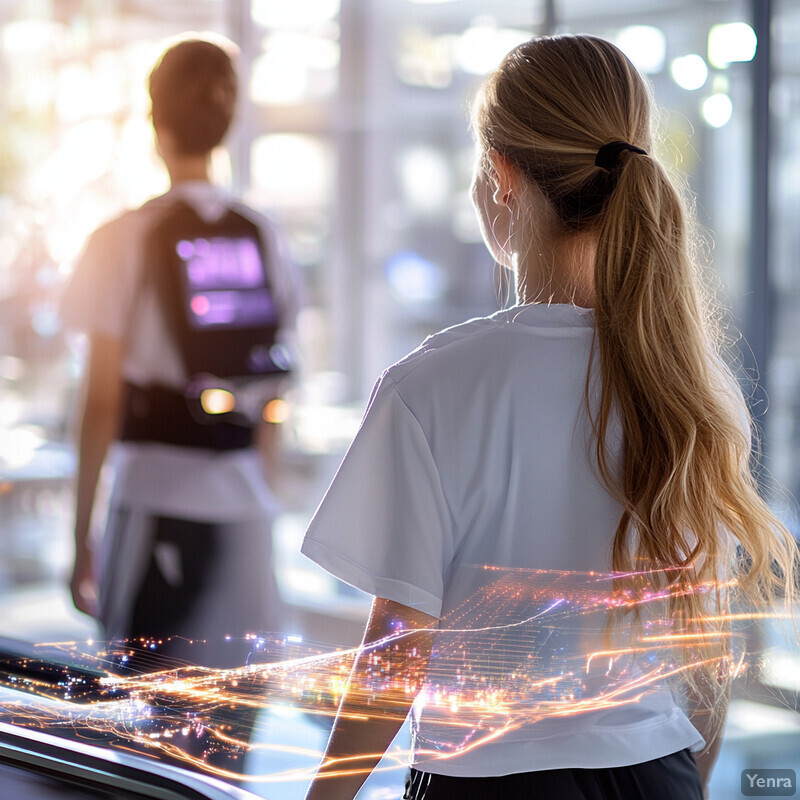
Recent clinical studies demonstrate how AI-enhanced biofeedback improves gait rehabilitation. In Parkinson’s disease, a 2024 case series used a wearable AI system that delivered customized vibratory feedback at specific gait phases; patients showed notable gains in walking speed (from ~0.72 to 0.95 m/s) and step length after just a few training sessions. Similarly, in stroke patients, researchers implemented real-time auditory feedback targeting ankle push-off and knee extension – this led to significant increases in gait speed and stride length compared to no-feedback walkingnature.com . These systems leverage sensors (like foot pressure insoles or motion trackers) and machine learning models to detect gait deviations in milliseconds. They then trigger feedback: for example, a vibration on the weaker leg when stance time is too short, or a tone when posture deviates. The immediate correction helps patients adjust each step, resulting in measurably better balance and symmetry over time. As evidence grows, it’s clear that AI-driven, real-time feedback is a powerful tool to enhance gait training efficacy.
3. High-Fidelity Joint Angle Estimation
AI techniques now extract detailed joint angles and movement trajectories from gait data with very high accuracy. By training deep learning models on large motion datasets, researchers have achieved more precise tracking of hip, knee, and ankle angles throughout the walking cycle than previously possible with manual methods. For example, modern neural networks can estimate sagittal plane joint angles within about 5 degrees of error compared to gold-standard motion capture. These models combine inputs like wearable sensor signals (from inertial measurement units attached to the legs) or video frames and output continuous angle measurements for each joint in real time. The result is a high-fidelity picture of how a patient’s joints are moving – every bend of the knee or flex of the ankle – even outside of a lab. This level of detail gives therapists clearer insight into gait abnormalities and compensations. In short, AI-driven gait analysis provides clinicians with highly accurate joint kinematics, enabling more precise diagnoses and the tailoring of interventions to subtle movement deficits.
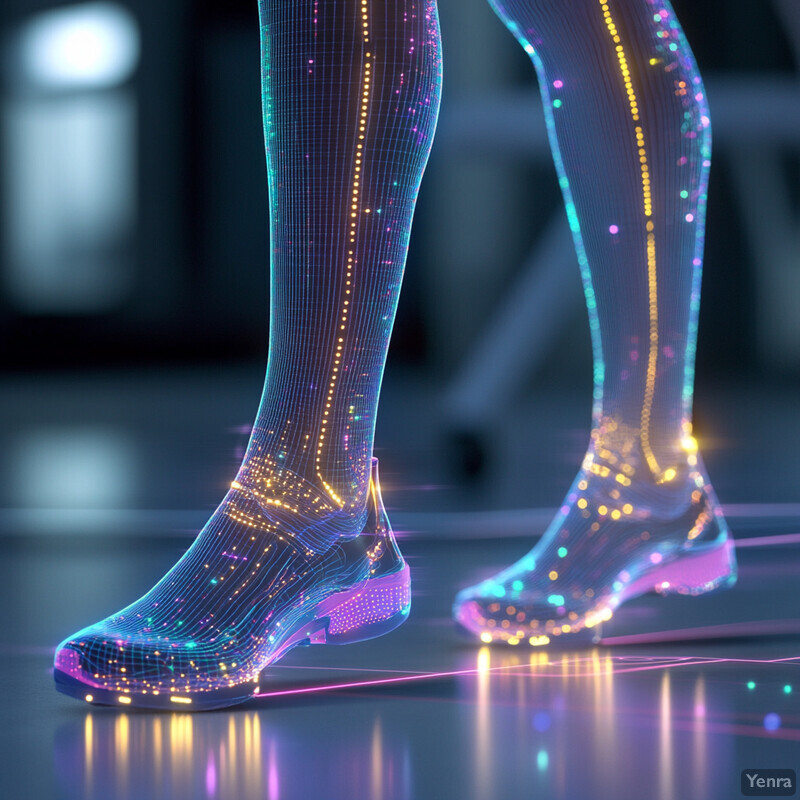
Technical studies confirm that machine learning models can achieve near lab-grade joint angle measurements from simple sensors. One 2024 study used deep learning on IMU (inertial sensor) data to predict lower limb angles during walking and running; the model’s angle estimates were within ~5–6° of error for hip, knee, and ankle angles compared to a camera-based motion lab. Another experiment employed an AI pose-estimation algorithm (OpenPose) on standard video to measure bilateral leg kinematics. Researchers found the AI’s angle measurements were statistically on par with a traditional 3D motion capture system for most gait phases. Additionally, machine learning methods have been used to fuse multiple sensor inputs (e.g. combining foot-mounted IMUs with pressure insoles) to further enhance joint angle accuracy and reduce noise. The consensus from these studies is that AI methods reliably capture fine-grained joint motion. These high-fidelity angle outputs allow clinicians to spot subtle gait issues – like a slight reduction in knee flexion or delayed ankle push-off – that might be missed without advanced technology. With AI’s precision, gait assessments become both quantitative and comprehensive, improving clinical decision-making.
4. Enhanced Gait Parameter Extraction
Artificial intelligence automates the calculation of key gait parameters, making analysis faster and more consistent. AI systems can instantly compute metrics like stride length, walking speed, cadence (steps per minute), and stance/swing time for each leg by processing sensor or video data. This replaces manual timing with stopwatches or laborious step counting. Importantly, AI does this with high reliability – studies report excellent repeatability (intraclass correlations often greater than 0.90) for AI-derived spatiotemporal gait measures across different sessions. By quickly crunching large datasets, machine learning algorithms can also derive more complex indicators (e.g., variability of stride time or symmetry indices) that help characterize a patient’s gait quality. In practice, this means therapists get a comprehensive set of objective gait metrics at the push of a button. The consistency of AI-extracted parameters removes observer bias and helps track patient progress accurately. Overall, AI greatly streamlines gait parameter extraction, allowing clinicians to focus on interpretation and treatment rather than data gathering.
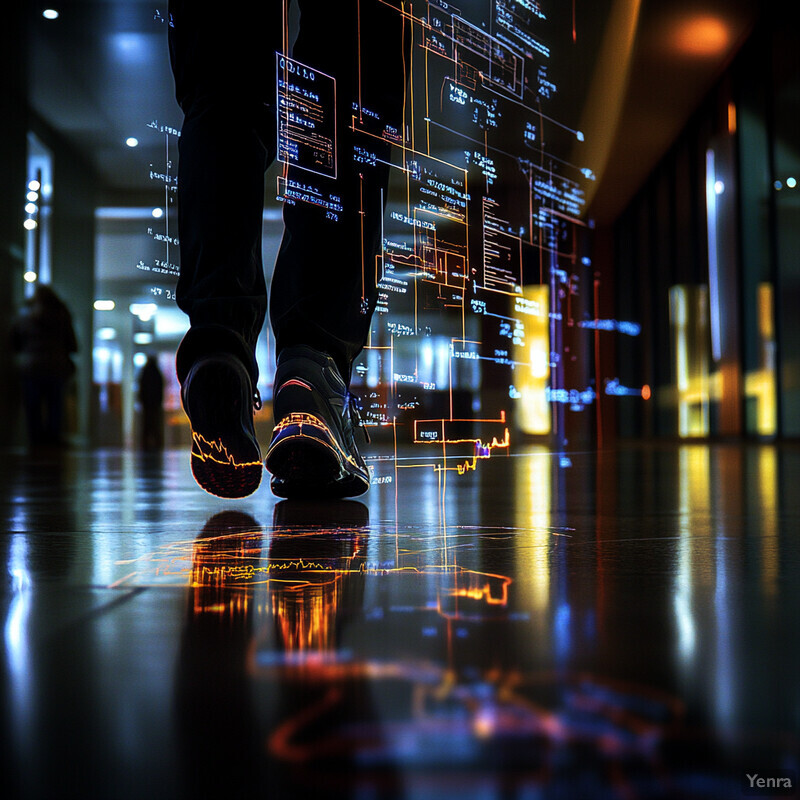
Automated gait parameters from AI systems have been shown to match or exceed human measurement accuracy. For instance, a 2025 study using wearable sensors and AI reported excellent agreement in all standard gait parameters (stride length, step time, cadence, etc.) when compared to reference measurements – most parameters had reliability coefficients above 0.90, indicating very stable results across tests. Another project (the Mobilise-D consortium) validated that an AI algorithm measuring gait speed from a wearable device produced results virtually identical to in-lab assessments across thousands of steps. These systems not only measure basic metrics but can also output comprehensive gait profiles. For example, AI can generate temporal plots of each joint’s motion and detect subtle timing differences between left and right leg steps. In a clinical trial, an AI platform automatically calculated 13 spatiotemporal gait parameters for each patient in seconds, with les than 5% error, significantly reducing the workload for gait labs. Such robust data extraction means that every stride a patient takes can be quantified, helping therapists identify specific gait deficits (like a shorter step length on the affected side) and monitor improvements over time with objective numbers.
5. Predictive Analytics for Fall Prevention
AI is being used to predict fall risk by detecting subtle gait abnormalities that precede falls. By training on large datasets of seniors or patients (with known fall histories), machine learning models have learned to recognize patterns – for example, irregular stride timing, reduced foot lift, or gait variability – that statistically increase risk of falling. These algorithms can then flag individuals who exhibit similar gait traits, even before they’ve fallen. In practical terms, this allows early intervention: a therapist might see an AI-generated fall risk score and introduce balance training or assistive devices proactively. Recent studies show promising accuracy for these predictions. For instance, AI models have distinguished “fallers” from “non-fallers” among older adults with up to ~90–96% accuracy under certain conditions. In Parkinson’s disease, where falls are common, an AI tool integrating gait and clinical data was able to estimate patients’ 6-month fall risk across multiple clinics, enabling tailored fall-prevention strategies. Overall, predictive analytics transforms gait data into actionable fall risk assessments, moving care from reactive to preventive.
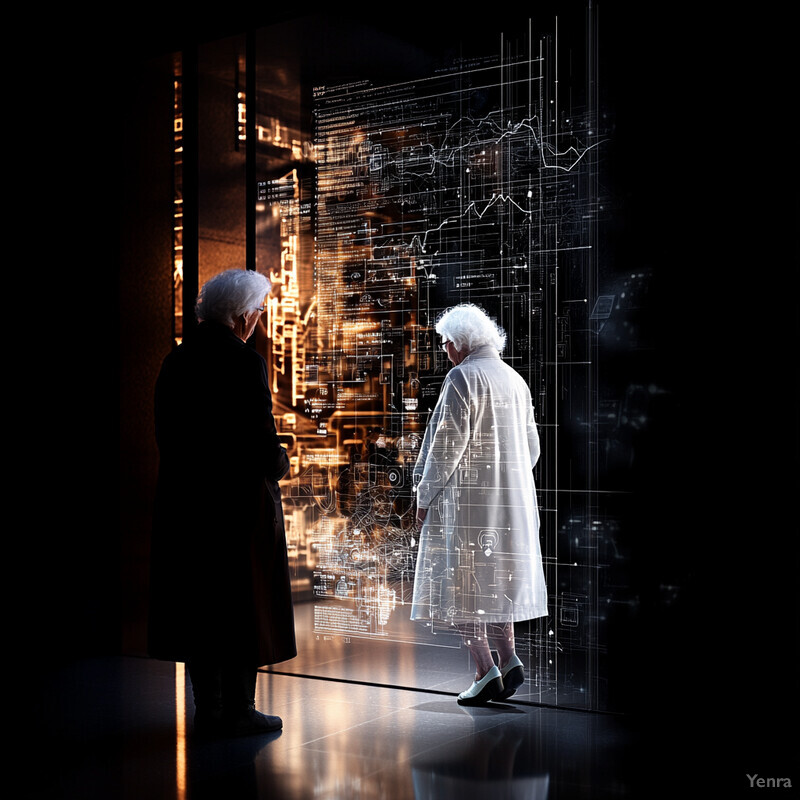
Specific machine learning implementations have demonstrated how gait analysis can forecast falls. A 2024 study used a range of gait features (stride time, double-support time, etc.) from older adults performing a Timed Up-and-Go test and achieved 96% accuracy in identifying those with a history of falls using a LightGBM classifier. The model highlighted that variability in step timing and slower walking speed were strong predictors of fallers. In another example, a multi-center 2025 study of Parkinson’s patients employed an AI model (Random Forest) that combined gait characteristics with clinical data; it successfully predicted fall occurrences over the following months, allowing clinicians to pinpoint high-risk patients. Notably, these AI systems can process complex combinations of factors – something traditional fall risk assessments struggle with. They might note, for instance, that a slight increase in gait asymmetry coupled with cognitive dual-tasking difficulty sharply raises risk in a given individual. By crunching thousands of data points, AI-based predictors can outperform conventional assessments (like questionnaires or single metrics) in sensitivity and specificity. As a result, therapists get a powerful decision aid to identify who needs fall prevention measures most urgently, potentially reducing fall-related injuries through early action.
6. Personalized Rehabilitation Plans
AI is enabling more personalized physical therapy by tailoring rehab exercises to each patient’s needs. Instead of a one-size-fits-all approach, machine learning can analyze an individual’s gait deficits, strength, and progress data, then suggest a customized therapy regimen. This might mean selecting specific balance exercises for a patient with instability or adjusting the difficulty and intensity of gait drills in real-time based on performance. Early implementations show that AI-driven systems can boost patient outcomes by optimizing the therapy to the person. For example, an AI might recommend longer stride practice for someone with short step length, or more ankle dorsiflexion training if it detects foot drop in gait. A 2025 review highlighted that AI systems in rehab can adapt treatments to individual needs, which increases patient engagement and satisfaction. Importantly, therapists remain in control – the AI provides data-driven insights (like “patient X responds better to task-oriented gait exercises”), helping clinicians refine and personalize the rehab plan. In summary, artificial intelligence adds a layer of precision to rehabilitation planning, making therapy more patient-specific and potentially more effective.
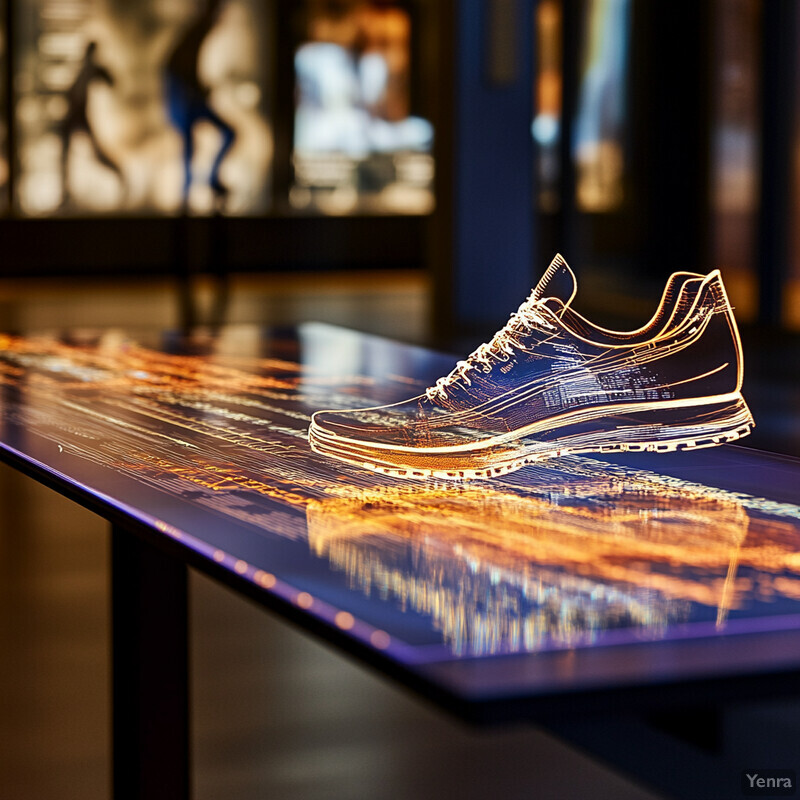
Concrete examples of AI-assisted personalized rehab are emerging. In one study, researchers developed an AI system that monitored stroke patients’ gait symmetry and dynamically adjusted the difficulty of stepping exercises: if a patient improved, the system introduced slightly more challenging tasks; if they struggled, it eased up. This led to faster improvements compared to a fixed exercise program, as each patient received a continually optimized challenge level. Another project (detailed in a 2025 narrative review) described how AI can predict a patient’s rehab progress trajectory from initial assessments and thus recommend a tailored treatment path. For instance, if AI analysis of a patient’s baseline gait indicates high fall risk and slow gait speed, it might prioritize balance and speed training modules in their plan. Clinical adoption is underway: some smart rehab platforms use algorithmic recommendations to assist therapists in choosing exercises – one such system was shown to improve functional outcomes in knee replacement patients by personalizing their post-surgery walking and balance drills. While still developing, these AI-driven plan optimizations consistently point toward the same result: more individualized therapy, where the type, intensity, and progression of exercises are finely tuned to what each patient needs for optimal recovery.
7. Automated Gait Classification
AI can automatically classify gait patterns, helping in diagnosis and tracking of conditions. By learning from examples of different gait types, machine learning models can recognize whether someone’s walking pattern is normal or exhibits characteristics of specific disorders. For instance, AI has been used to distinguish Parkinsonian gait (marked by shuffling steps and reduced arm swing) from healthy gait, or to identify gait signatures of conditions like cerebral palsy, stroke hemiparesis, or neuropathic gait. These classifications can aid clinicians: an algorithm might flag that a patient’s gait is similar to a fall-prone pattern or that it matches the profile seen in early multiple sclerosis. Studies report high accuracy for such classification tasks. In one case, a deep learning model separated pathological gait from normal with about 92% accuracy using wearable sensor data. Another system categorized gait into profiles – like “arthritic gait” vs “Parkinson’s gait” – to assist physicians in diagnosis. Overall, AI-based gait classification offers a consistent, objective way to categorize walking patterns, which can be especially useful for early detection of diseases and for monitoring changes over time in known patients.
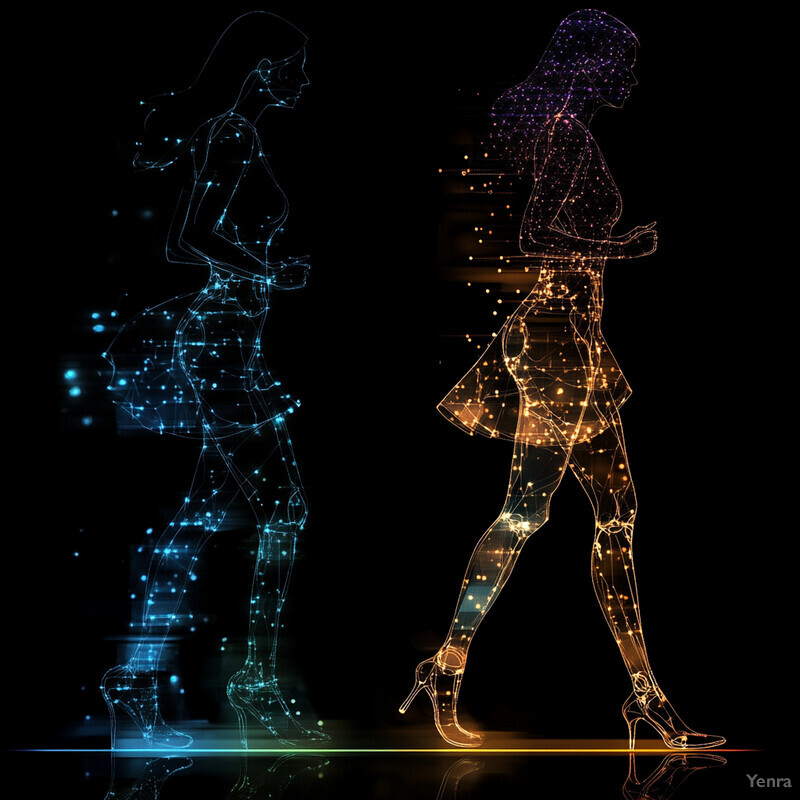
Technical validation of automated gait classification is compelling. In a 2024 study, researchers developed a machine learning algorithm that used signals from inertial sensors on the legs to classify three types of gait: normal gait, gait with knee impairment, and gait with ankle impairment (simulating orthopedic conditions). The AI achieved over 91% classification accuracy distinguishing these categories, outperforming human experts and even a traditional gait analysis mat (which was only ~76% accurate). Similarly, multiple groups have shown that neural networks can identify Parkinson’s disease from gait. One such model analyzed subtle features like stride length variability and asymmetry; it correctly identified early-stage Parkinson’s gait versus healthy gait about 97% of the time in a controlled study. These systems typically undergo training on large datasets – for example, hundreds of gait recordings labeled by diagnosis – so they learn the distinguishing features of each condition’s gait. Once trained, the AI can take a new patient’s gait data and output a classification (e.g., “gait suggests possible Parkinson’s” or “gait indicates left-sided hemiparesis”). Clinicians can use this as a decision support tool, combining it with clinical exams. As classification models continue to improve, they may enable screening programs (flagging people whose gait looks pathological) and provide quantitative endpoints (like “gait now classifies as normal” after rehab).
8. Wearable Sensor Data Integration
AI enables the fusion of data from multiple wearable sensors to paint a complete picture of gait dynamics. In modern gait analysis, it’s common for patients to wear several sensors – for example, inertial measurement units (IMUs) on the legs, pressure-sensing insoles in the shoes, and perhaps EMG electrodes on muscles. Machine learning algorithms can combine all these data streams in real time, correlating foot pressure patterns with leg kinematics and muscle activation. By integrating signals, AI can derive gait metrics that single sensors alone might miss, such as ground reaction forces estimated from IMU data or joint moments inferred from the synergy of motion and force information. This sensor fusion provides a more robust and noise-resistant analysis. It also allows comprehensive gait assessment outside labs – for instance, a patient at home might have a smart insole and a thigh-worn IMU; an AI model running on a smartphone can merge their data to calculate stride length, foot impact force, and joint angles on each step. Ultimately, integrating wearable sensor data through AI gives clinicians a rich, clinically meaningful set of gait measures in any environment, facilitating remote monitoring and detailed analysis without full motion laboratories.
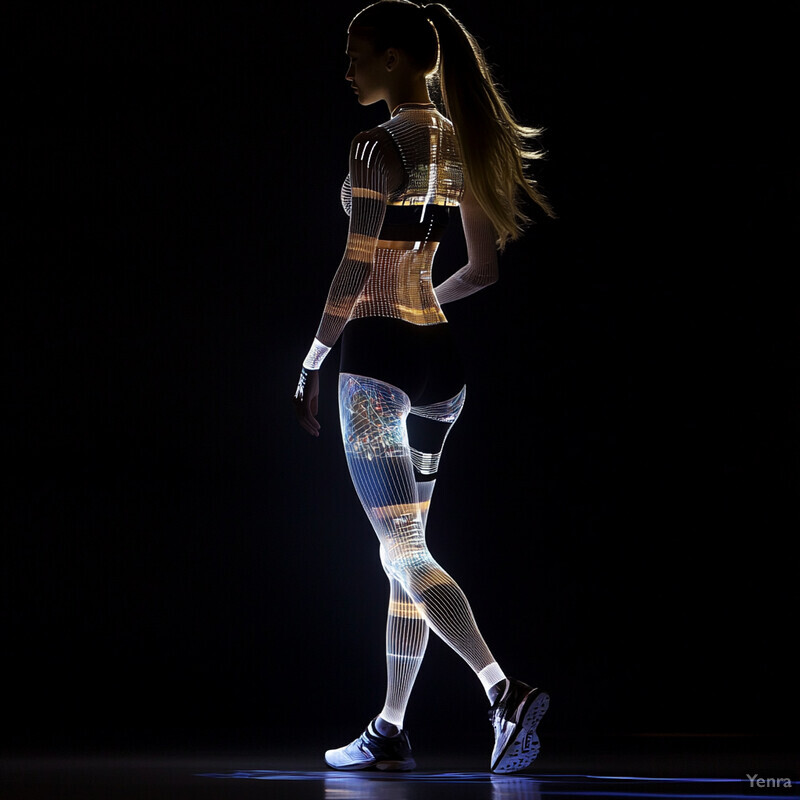
Concrete demonstrations of sensor integration show its value. One research group developed a low-cost gait measurement system that combined flexible foot pressure sensors with IMUs on the legs, all processed by an AI algorithm. Their system could accurately measure traditional gait parameters and even estimate forces and joint angles, with errors under 3% for force measurements and ~5–8% for angle measurements compared to a force plate and optical motion capture. Another example is the use of high-density electromyography (EMG) together with IMUs: by synchronizing muscle activity data with limb kinematics, AI models can identify abnormal gait muscle firing patterns that correlate with gait deviations. In prosthetics, researchers have shown that integrating a prosthetic limb’s built-in sensors (for angle and torque) with data from the user’s residual-limb IMUs allows the AI to detect gait imbalances and propose alignment adjustments. In all these cases, the AI acts as the “brain” fusing raw inputs into cohesive information – such as detecting that a patient’s weak push-off (seen in pressure data) coincides with limited ankle motion (seen in IMU data), indicating the need for calf strengthening. The successful fusion of multi-sensor data via AI means clinicians get lab-quality, multi-dimensional gait analysis from simple wearable setups.
9. Virtual Reality and Augmented Reality Feedback
AI-powered virtual and augmented reality (VR/AR) systems are being used to create immersive gait training environments. Patients can practice walking in virtual scenarios – such as navigating obstacles or varying terrains – while receiving real-time guidance augmented by AI. For example, in VR a patient might see a pathway with visual cues for foot placement, with the difficulty adapting based on their performance. These environments make therapy more engaging (“gamified”) and also safer, since challenges can be simulated without real danger (e.g., a virtual trip hazard to step over). Clinical studies indicate that VR-based gait rehabilitation can improve outcomes: patients training on treadmills with VR obstacles and AI feedback showed better improvements in balance and walking speed than conventional treadmill training. Augmented reality can also overlay guidance in the real world – for instance, smart glasses displaying prompts to lift the toes or lengthen stride, informed by AI analysis of the person’s gait. By combining AI’s analytical power with VR/AR’s interactive visuals, these systems provide an engaging, adaptive and effective way to retrain gait patterns and improve mobility confidence.
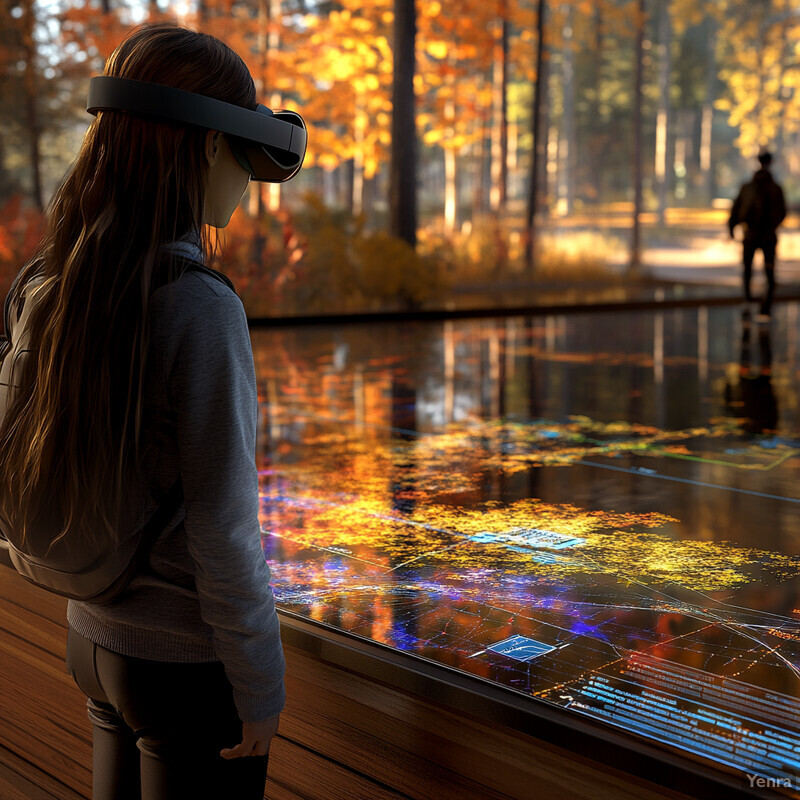
Several pilot studies highlight the benefits of VR gait therapy. In one feasibility study for Parkinson’s disease, patients used a Gamified Virtual Reality Environment (GVRE) for gait training on a treadmill. The VR system, guided by an AI engine, presented virtual environments (like a park or city street) where patients had to avoid virtual obstacles and follow prompts. The program could progressively increase task difficulty – for instance, narrowing a virtual walkway if the patient was doing well – thereby challenging the patient appropriately. The results found the VR-trained patients had improved gait velocity and stride length, and importantly, they reported higher enjoyment and motivation to train compared to traditional therapy. Another example involved post-stroke rehabilitation: a 2023 study combined a motion-capture-based AI with AR visual feedback. The patient’s steps were analyzed in real time, and an AR display projected foot placement targets and balance cues. Therapists observed that patients using this AR system were quicker to correct gait deviations (like limb circumduction or uneven step timing) during sessions. Moreover, objective assessments showed better gait symmetry after training with AR cues than without. These findings, though early, illustrate how AI + VR/AR can amplify rehabilitation – making it interactive and adaptive – leading to measurable gait improvements and potentially lower fall risk in patients.
10. Remote Patient Monitoring and Telehealth
AI is allowing effective gait monitoring and rehabilitation through telehealth. Patients can be assessed and treated while at home using AI algorithms that analyze data from simple devices like smartphone cameras or wearable sensors. For example, a patient might walk in their living room while their phone records video; an AI app can compute their gait speed, stride length, and even detect abnormalities, then securely transmit these metrics to their therapist. One mobile app (“AI-enabled GaitKeeper”) has been shown to measure gait speed and variability with accuracy on par with in-clinic systems, standardizing at-home assessments. Telehealth gait analysis supported by AI means therapists can keep track of patients’ mobility between clinic visits, adjust rehab programs on the fly, and ensure safety (by spotting declines early). In addition, AI can enable virtual visits where the system gives real-time feedback to the patient (“lift your toes higher on the next step”) during a tele-rehab session. Overall, integrating AI into remote gait monitoring extends the reach of physical therapy, making high-quality gait analysis and training accessible to patients who can’t frequently travel to clinics. This continuity of care can lead to better long-term outcomes and more timely interventions.
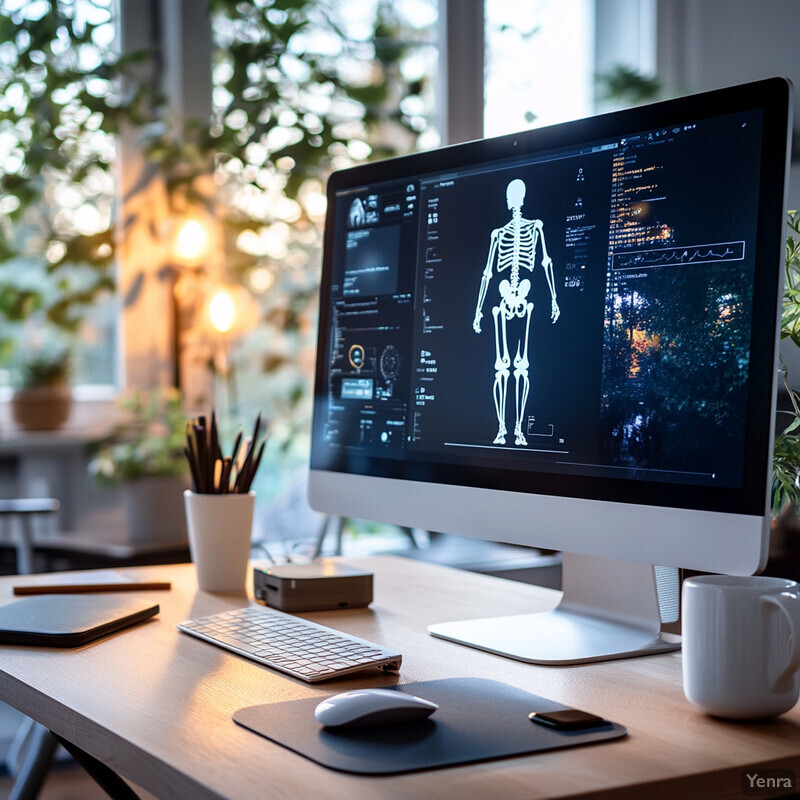
Recent developments showcase the practicality of AI in remote gait monitoring. In 2024, researchers validated a smartphone app that uses augmented reality and AI (“GaitKeeper”) to measure gait speed at home. In trials with older adults, the app’s measurements of walking speed and step length were almost identical to those from a gold-standard gait mat (concordance within a few percent), demonstrating clinical-grade precision without requiring an in-person exam. Another study developed a low-power device running an AI pose-estimation algorithm that can be mailed to patients – it uses a single camera to perform 3D gait analysis in the patient’s home, with results comparable to an in-lab exam. On the tele-rehab side, AI-driven systems have been used in video calls: one system analyzed a live video of a post-surgery patient’s walking pattern and provided the therapist with objective metrics (e.g., cadence and symmetry) during the consultation, helping them adjust the exercise program in real time. Furthermore, remote AI monitoring has been applied in fall prevention: if a home-based gait monitoring AI detects a worsening in balance or gait speed, it can alert clinicians to intervene early. These examples underline that AI can maintain the quality of gait assessment outside the clinic, effectively bringing “eyes on the patient” through any camera or sensor – enabling truly continuous, data-driven telehealth for mobility issues.
11. Early Detection of Neuromuscular Disorders
AI analysis of gait patterns is helping clinicians detect neurological or neuromuscular disorders at earlier stages than traditional exams. Certain diseases – like Parkinson’s disease, multiple sclerosis (MS), or peripheral neuropathy – cause very subtle gait changes in their initial phases (perhaps a slightly slower walking speed, a bit of asymmetry, or increased stride variability). Machine learning algorithms can be trained to recognize these minute deviations by comparing a person’s gait data to healthy norms. This means an AI system might flag “possible early Parkinsonian gait” even when the person can still walk normally in daily life, prompting further medical evaluation. For example, AI has identified early-stage Parkinson’s patients by their gait with decent accuracy (around 67–78% in research settings) when human observers saw little difference. Similarly, researchers are exploring AI gait analysis for MS, aiming to catch slight slowing or balance issues before major symptoms. By catching disorders early, treatments (medication, physical therapy, etc.) can start sooner, potentially slowing disease progression. In summary, AI’s keen pattern recognition in gait analysis provides a novel screening tool for neuromuscular conditions, offering a non-invasive way to detect diseases earlier than conventional methods might allow.
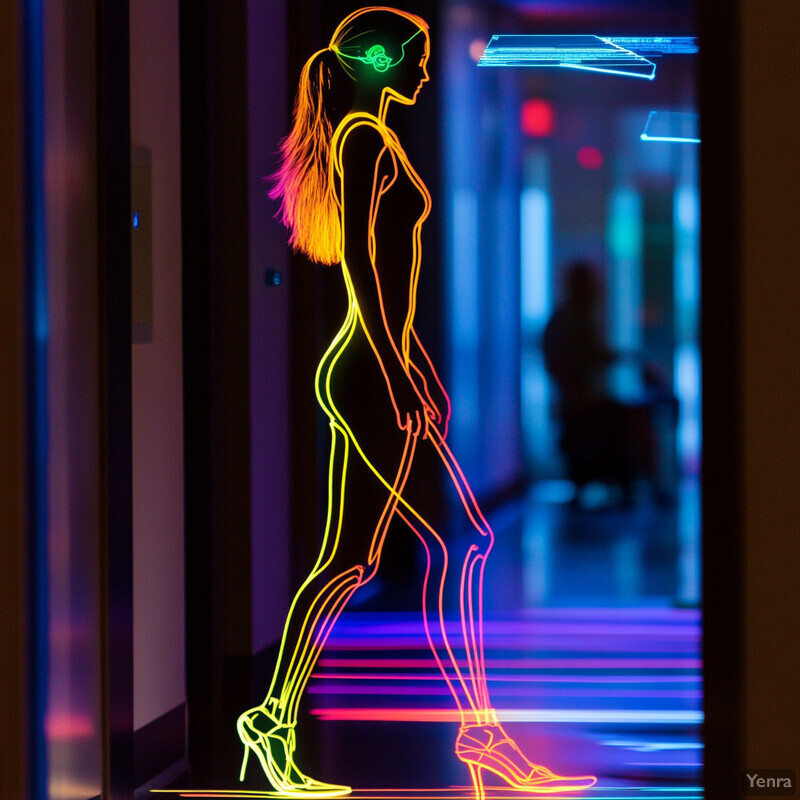
One striking example comes from Parkinson’s disease research. In a 2025 study, scientists used an AI model to analyze detailed spatiotemporal gait features (like stride length and variability at various walking speeds) from 178 individuals, some with early Parkinson’s, some with moderate Parkinson’s, and healthy controls. The AI learned to separate early Parkinson’s gait from normal gait – notably, it found that an abnormally short stride at a faster walking pace and an increased variability in stride length at a slow pace were telltale early signs. Using just two gait metrics, the model could distinguish early Parkinson’s patients with about 67% accuracy (which, while modest, is significant given clinical exams often can’t detect such early gait changes at all). For moderate-stage vs early-stage, accuracy was ~70%. This demonstrates AI’s ability to detect mild gait impairment associated with early neural deficits. Beyond Parkinson’s, other work has shown AI can classify subtle balance and gait changes in diabetic neuropathy patients versus healthy peers, which could serve as an early warning for nerve damage. In MS, preliminary results indicate that machine learning can pick up slight slowing and increased sway in walking that correlate with very early MS lesions on MRI. While still evolving, these findings suggest AI gait analysis could become a valuable addition to routine screenings – for instance, an older adult’s annual physical might include a quick AI gait test to check for emerging neurological issues that merit further testing.
12. Objective Outcome Measurements
AI-driven gait analysis provides objective metrics to evaluate patient progress, reducing reliance on subjective observation. In traditional rehab, therapists often rate gait quality or improvement based on visual judgments or coarse measures (like “walked 10 meters 2 seconds faster”). AI now delivers precise, quantifiable outcomes – for example, “stride length increased by 5 cm” or “gait symmetry improved by 15%,” or even composite gait quality scores. These consistent measurements allow for standardized tracking of outcomes across sessions and clinicians. If one therapist measures a patient’s walking speed with a stopwatch and another uses their judgement of smoothness, they might disagree; but an AI system analyzing sensor data will produce the same result for walking speed or smoothness, whoever operates it. This consistency builds trust in the data. In practice, objective outcomes mean clinicians and patients can clearly see improvements (or detect plateaus) in hard numbers. Studies have noted that automated gait metrics like cadence, stance time, and symmetry index have high reliability and sensitivity to change, making them excellent outcome measures for rehabilitation trials. By adopting AI-based objective metrics, therapy teams can make data-informed decisions (e.g., ready to progress from walker to cane because gait stability score has improved to a safe level). Overall, AI ensures that outcome measurement in gait rehab is quantitative, repeatable, and free of personal bias.
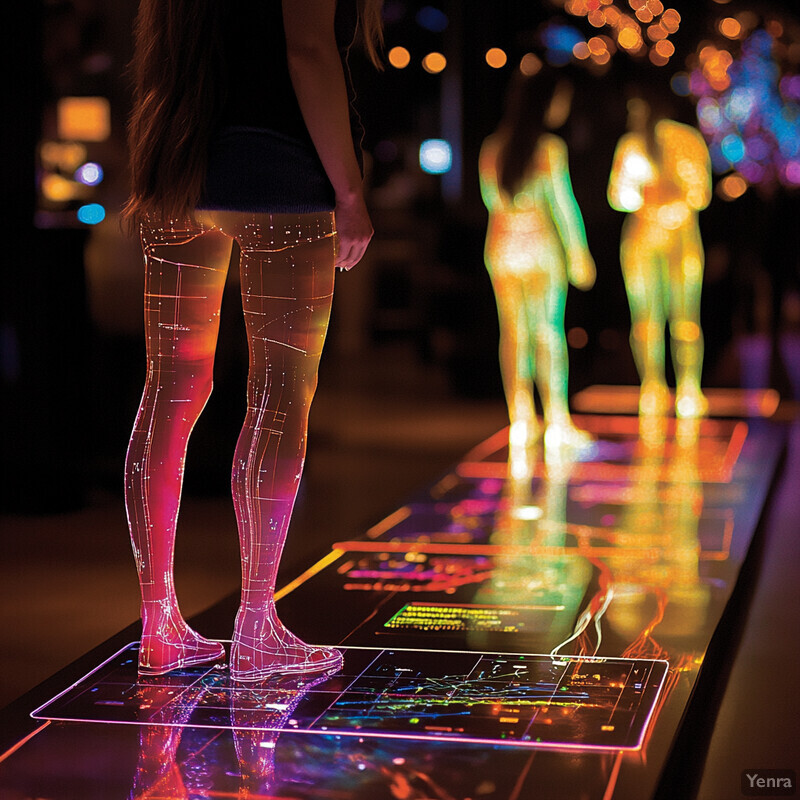
The impact of objective measures is evident in clinical settings using AI gait tools. In one mobility clinic, patients began being assessed with an AI motion analysis app before each appointment. Rather than notes like “gait somewhat better” in the chart, the system generated concrete data – for example, a patient’s walking speed increased from 0.8 m/s to 1.0 m/s after 4 weeks of therapy, and their left-right step time asymmetry decreased from 10% to 4%. These numbers gave both the therapist and patient clear evidence of improvement, whereas previously they might rely on a general impression. In trials, objective gait measures have improved the evaluation of interventions: a 2024 study on powered prosthetics introduced a “gait quality index” derived from force and motion sensors to quantify how well the prosthesis was tuned. This index detected improvements that were missed by subjective tuning assessments, proving its value in outcome measurement. Additionally, regulatory bodies and payers are interested in hard endpoints – AI provides exactly that. For instance, an AI-based gait speed measure is being considered as a digital biomarker for drug trials in Parkinson’s, because it can be consistently measured across sites and observers. As another example, an AI algorithm’s output of “percentage of time spent in balanced gait” has been used to document how much a patient recovered stability after vestibular therapy, offering a more nuanced outcome than just a balance questionnaire. Across the board, the infusion of AI is turning subjective gait analysis into objective outcome science, improving transparency and decision-making in rehabilitation.
13. Machine Learning-Based Data Reduction
Gait analysis produces a huge amount of data (multiple angles, forces, timings), and AI helps distill this complexity into key indicators. Using techniques like principal component analysis (PCA) or feature selection algorithms, machine learning can reduce the dimensionality of gait data – essentially compressing many correlated variables into a few meaningful factors. This makes clinical interpretation easier: instead of trying to interpret 20 different gait parameters, a therapist might look at 2–3 composite features or an overall gait deviation index. For example, in cerebral palsy gait analysis, researchers have used PCA to combine kinematic deviations at various joints into a single “Gait Deviation Index” score that summarizes overall gait abnormality on a 0–100 scale. AI can automate and refine these reductions, ensuring that the most informative patterns are captured. A recent systematic review noted that many studies applied dimensionality reduction to gait datasets to focus on the most relevant features, improving the efficiency of analysis and often the accuracy of classification models. In sum, machine learning-based data reduction turns the high-dimensional, noisy gait data into digestible metrics or scores, without losing essential information. This allows clinicians to grasp patient status at a glance and track progress with simplified yet comprehensive indices.
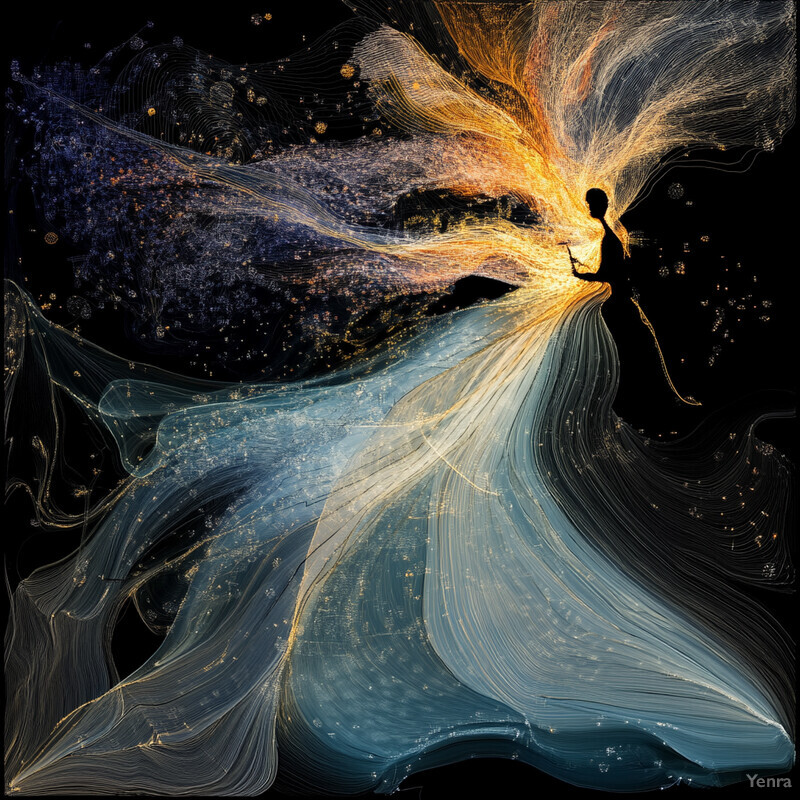
Concrete implementations highlight how data reduction enhances gait analysis. One study on pediatric cerebral palsy gait data used an AI approach to identify the top principal components (PCs) describing gait variance among patients. The first PC in their analysis captured a combination of knee and hip motion patterns that explained over 70% of the variability in gait – effectively summarizing the gait impairment severity with one value. By focusing on that PC score, surgeons could better compare pre- vs post-operative gait changes in these children. Another investigation in stroke survivors employed an autoencoder (a form of neural network) to compress full-body kinematic gait data into just two dimensions, which corresponded to “overall gait symmetry” and “temporal coordination.” These compressed features were then used to successfully cluster patients by level of impairment. Moreover, feature selection algorithms are used in machine learning gait models to trim irrelevant or redundant inputs. In one 2024 study, researchers initially had 25 gait features from IMUs; an AI recursive feature elimination identified the 3 most informative features (a symmetry index and two timing variables) that achieved 93–94% classification performance – using all 25 did not improve accuracy. This shows that AI can not only reduce data for interpretation but also for model efficiency. The outcome is streamlined gait analysis that retains critical insights: clinicians get simpler scores (like “gait quality = 78/100”) that still reflect intricate underlying measurements, thanks to AI’s data reduction prowess.
14. Automated Progress Tracking
AI tools can automatically track a patient’s gait improvements (or regressions) over time by comparing current gait data to prior data. Rather than relying solely on periodic exams, these systems continuously or periodically measure gait parameters and detect trends. For instance, an AI algorithm might notice that a stroke patient’s step length has increased by 10% over the last two weeks and their walking speed by 0.2 m/s – clear signs of progress – even if the changes are too subtle for a casual observer to notice day-to-day. Conversely, the system might detect if a Parkinson’s patient’s gait is slowing or becoming more variable, signaling a need to adjust treatment. By quantifying change, AI enables data-driven adjustments to rehab plans (e.g., advancing to more difficult exercises once plateaus are identified). This also provides motivation to patients, who can see objective proof of improvement on charts or dashboards. Studies in rehab settings have shown that ML-based analyses can sensitively pick up gait changes: some algorithms can distinguish an improvement as small as a few percentage points in symmetry or a few centimeters in step length that clinicians might have overlooked. In essence, automated progress tracking ensures no improvement or decline goes unnoticed, thereby optimizing the rehabilitation trajectory and outcomes.
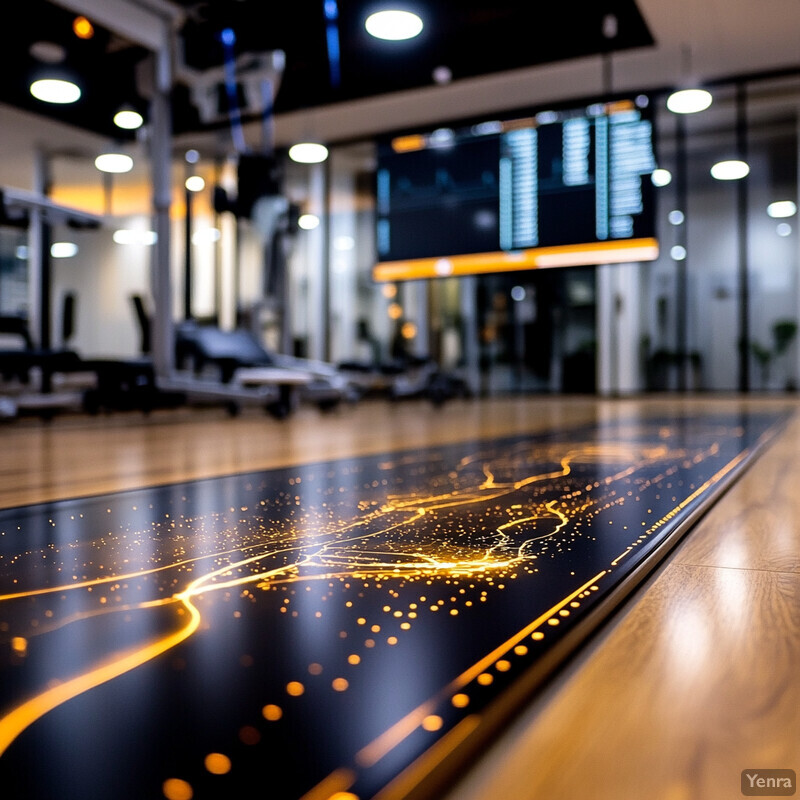
Implementation of automated tracking is evidenced in research and practice. In one clinical trial for orthopedic rehab, patients recovering from knee surgery wore a sensor that fed gait data to an AI platform daily. The AI produced weekly summaries indicating changes in metrics like weight-bearing symmetry and stride smoothness. These summaries showed a steady improvement for most patients, but importantly, it flagged two patients whose gait symmetry stagnated – prompting clinicians to provide extra interventions for those individuals, ultimately improving their outcomes compared to historical norms. A 2024 systematic review found that among 63 machine-learning gait studies, a common goal (in over 20% of them) was to monitor how gait patterns change with interventions or over time. For example, one study used clustering algorithms on gait data from multiple therapy sessions to automatically group strides by quality; as the patient improved, more strides fell into the “normal gait” cluster vs the “impaired gait” cluster, objectively marking progress. Another interesting use: gamified rehab systems with AI not only adapt exercises (as mentioned earlier) but also record performance metrics each session – the AI can then chart these metrics, showing trends like “patient’s balance score increased from 60 to 75 in 3 weeks.” This ongoing analysis helps therapists decide when goals are met or when to update the care plan. The outcome is a shift from snapshot evaluations to continuous monitoring, enabled by AI, ensuring timely and personalized care adjustments for optimal recovery.
15. Optimized Prosthetic and Orthotic Fitting
AI is improving how prosthetic limbs and orthopedic braces (orthotics) are fitted and tuned to patients by analyzing gait data. Traditionally, prosthetists adjust alignment and components largely by trial-and-error and visual gait observation. Now, AI can assist by modeling how different adjustments would affect a patient’s gait and recommending optimal settings for comfort and performance. For example, an AI could analyze a transfemoral (above-knee) amputee’s walking pattern and suggest fine-tuning the prosthetic knee resistance or foot alignment to reduce an asymmetry in step length. In orthotics, AI can help design personalized braces (like an ankle-foot orthosis) that perfectly match the patient’s anatomy and gait needs, perhaps via 3D printing. This data-driven approach often results in better gait outcomes – patients walk more naturally and with less discomfort when their devices are tuned using quantitative feedback. Early studies in this realm have reported that AI-designed socket shapes for prosthetic limbs can achieve fit and pressure distribution comparable to expert-crafted sockets, but with far less manual effort archives-pmr.org. By shortening the fitting process and iterating designs digitally, patients receive their devices faster and typically require fewer adjustments. Overall, AI is making prosthetic/orthotic fitting more precise, efficient, and tailored to each individual’s biomechanics, which translates into smoother gait and higher satisfaction for assistive device users.
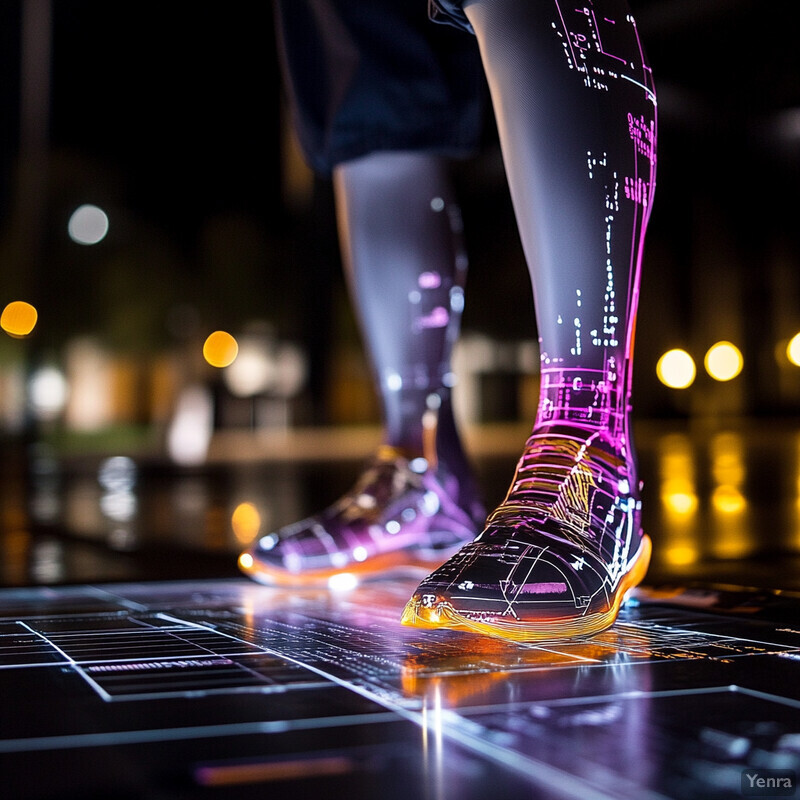
A concrete breakthrough is in prosthetic socket design. In 2024, researchers published results of an AI algorithm that generates transtibial (below-knee) prosthetic socket shapes based on a database of expert-designed sockets and the patient’s residual limb 3D scan. The AI-designed sockets were manufactured and tested: pressure sensor maps showed that these sockets distributed loads on the limb as well as the clinician-crafted ones, and patient comfort ratings were similar, demonstrating successful personalization by AI. Another study introduced an AI-driven system for prosthetic alignment tuning. It used gait sensor inputs (from a smart prosthetic foot) to adjust the alignment parameters in real time during a patient’s walking trial – the result was an objectively more symmetrical gait and reduced limp, achieved in a fraction of the time a manual tuning session would take. In orthotics, AI-based modeling has been used to optimize the stiffness of AFOs (ankle-foot orthoses) by predicting how different materials and geometries affect gait dynamics. One team employed a machine learning model to recommend an AFO design that minimized abnormal knee flexion in mid-stance; when built and tested, that AI-recommended brace indeed improved the patient’s gait more than the standard design. These examples underline that by leveraging gait data and computational models, AI can refine device fit and function beyond what subjective methods can do. Patients end up walking more efficiently (e.g., lower energy cost, closer to normal kinematics) when their prostheses or orthoses are fine-tuned with AI insights. As these technologies mature, we expect to see prosthetic fittings that once took weeks of appointments shortened to maybe a single session with AI guidance, giving patients a quicker return to mobility.
16. Enhanced Rehabilitation Gamification
AI is powering more engaging, game-like rehabilitation exercises for gait training, which keeps patients motivated. Gamification means turning rehab tasks into game challenges – for example, scoring points for good steps, or completing virtual missions by walking a certain distance or improving speed. AI comes in by adjusting the game difficulty to the patient’s level in real time and ensuring the “game” targets the therapeutic goals. If a patient is improving quickly, the AI might speed up the game or introduce new obstacles to keep them challenged; if they are struggling, it can simplify tasks to avoid frustration. This dynamic tailoring maintains an optimal level of difficulty, often referred to as the “challenge point,” which maximizes learning and enjoyment. Clinical pilots have shown that gamified gait rehab leads to better adherence: patients are more likely to complete their exercise programs and do extra practice because it’s fun and rewarding. For instance, a gait training game might involve collecting tokens by stepping with proper form, and patients often get immersed in achieving high scores. The use of AI ensures the game remains neither too easy nor too hard over the course of therapy. Overall, by merging therapy with gaming and using AI to personalize the experience, rehabilitation becomes more engaging without losing effectiveness – in fact, gamification tends to increase effectiveness by boosting patient effort and consistency.
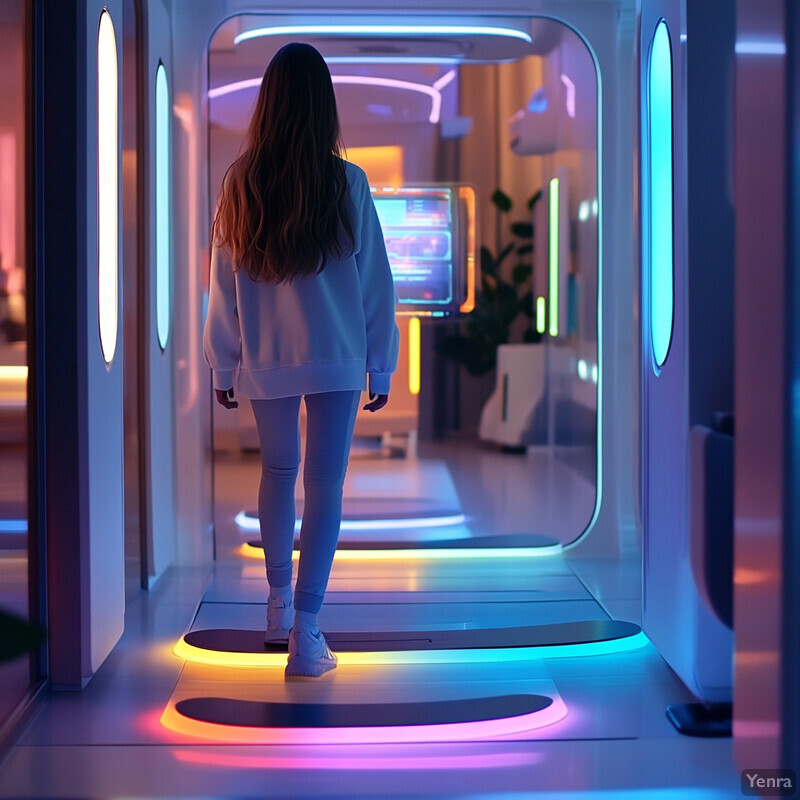
Practical examples of gamified gait rehab are emerging. A recent study co-created a treadmill-based game for Parkinson’s patients: as patients walked, they entered a virtual park environment where they had to follow specific paths and avoid obstacles, effectively practicing complex gait tasks. An AI algorithm monitored their performance (speed, obstacle hits, etc.) and adapted the game – for example, if a patient consistently succeeded, the next round the walking path would become narrower or the obstacles would move slightly faster. Feasibility results showed patients were highly engaged, and some even requested longer sessions because they enjoyed the game, something unheard of in conventional therapy. Another system called “Strolll” uses augmented reality glasses to overlay a game on the real world for people with Parkinson’s: the AI in that system provides auditory cueing and visual targets to step on, turning a daily walk into a mission (e.g., “stomp the alien circles on the floor”). Early reports indicate that using Strolll at home improved patients’ gait initiation and reduced freezing episodes, presumably because the interactive cues and game incentives encouraged regular practice. Additionally, researchers have quantified the benefits of gamification: a systematic review found that across multiple studies, gamified rehab not only improved physical outcomes (motor function and gait speed) but also significantly improved patients’ emotional state and motivation to continue therapy. The inclusion of AI means these games can be finely tuned to each patient, making rehabilitation both effective and enjoyable – a win-win for recovery.
17. Integration With Robotic Exoskeletons
AI is enhancing robotic exoskeletons used in gait rehabilitation by enabling these devices to adapt to the user in real time. Robotic exoskeletons (wearable powered braces for legs) can assist individuals with impaired walking (like spinal cord injury or post-stroke patients) to move their limbs. With AI control, the level of assistance provided by the exoskeleton can be continuously tuned: the system can give more support when the patient is struggling and back off when the patient initiates better movement on their own. This ensures the patient always gets an “optimal challenge” – enough help to complete the motion safely, but not so much that it does all the work. For example, if the exoskeleton’s sensors (perhaps measuring muscle effort or motion smoothness) detect that the patient is beginning to rely too heavily on the device, the AI can slightly reduce motor assistance to encourage the patient’s own muscles to engage more. Conversely, if fatigue sets in and performance declines, the AI can increase support to prevent bad habits or falls. Studies with AI-driven exoskeleton algorithms show patients achieve more natural gait patterns and greater voluntary effort compared to fixed assistance protocols. Additionally, AI can allow exoskeletons to automatically switch modes (say, from level-ground walking to stair-climbing assistance) by recognizing the environment. In summary, integrating AI makes robotic gait training devices smarter, safer, and more effective by personalizing the assistance in real time to each user’s needs.
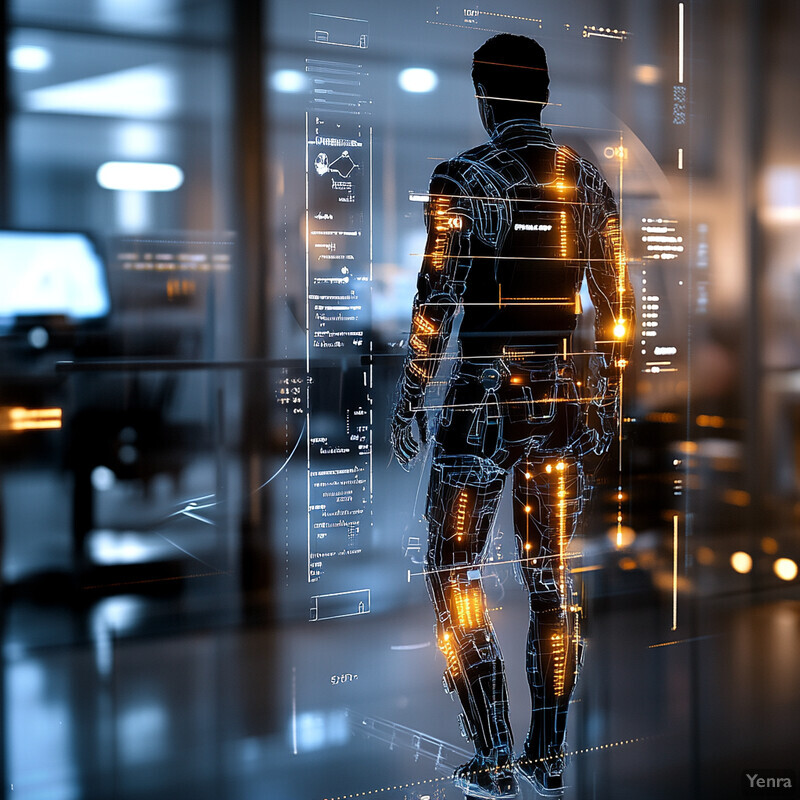
Cutting-edge research demonstrates these capabilities. In a 2023 publication in Science, engineers presented an AI-driven lower-limb exoskeleton that uses machine learning to detect gait phases and walking conditions, and dynamically adjust assistance type and level. For instance, when a user started walking on an incline, the AI controller seamlessly increased knee and hip power output to help uphill, then reduced power on flat ground to avoid doing all the work. The system also monitored the user’s effort (via EMG sensors) and modulated help – if the user’s muscle activation indicated they could handle more on their own, the exoskeleton faded its assistance, effectively acting like a therapist “weaning” the patient off support. In trials with individuals with mobility impairments, this AI-adaptive exoskeleton led to improvements in gait speed and reduced compensation movements compared to using a fixed-assistance exoskeleton. Another study introduced an adaptive control strategy for a hip exoskeleton using physiological feedback: it used the wearer’s heart rate and gait data to adjust how much torque to apply, ensuring the exercise stayed challenging but not overly exhausting. Those wearing the AI-controlled hip exoskeleton had significantly lower heart rates for the same walking task over time, indicating improved efficiency and endurance building. These examples reflect the trend: AI allows exoskeletons to evolve from rigid machines to responsive co-trainers, automatically tuning settings (e.g., support force, joint trajectory) to optimize the rehabilitation session or mobility experience for each user. Such intelligent exoskeletons are already showing better outcomes in clinical research, and they hold promise for wider use in restoring independent walking.
18. Data-Driven Clinical Decision Support
AI is increasingly used to support clinicians in making evidence-based decisions during gait rehabilitation. By analyzing large databases of patient gait metrics and outcomes, machine learning systems can offer treatment recommendations or predictions that inform therapy planning. For example, an AI tool might predict that a stroke patient with a certain gait speed and symmetry early in rehab is on track to recover independent walking in 8 weeks, or conversely, needs a different intervention if progress is lagging. Such insights help therapists decide when to progress a patient’s therapy or change strategies. AI can also synthesize complex data into simple visual dashboards – showing trends like improving balance scores or flagging risk factors (e.g., “this patient’s gait variability remains high, associated with fall risk”). In practice, this means clinicians get a “second opinion” grounded in big data: the AI might suggest, for instance, more balance training based on patterns seen in thousands of past cases with similar profiles. Ultimately, data-driven decision support aims to complement clinical judgment with objective analytics, ensuring patients receive interventions with the highest likelihood of success according to current evidence.
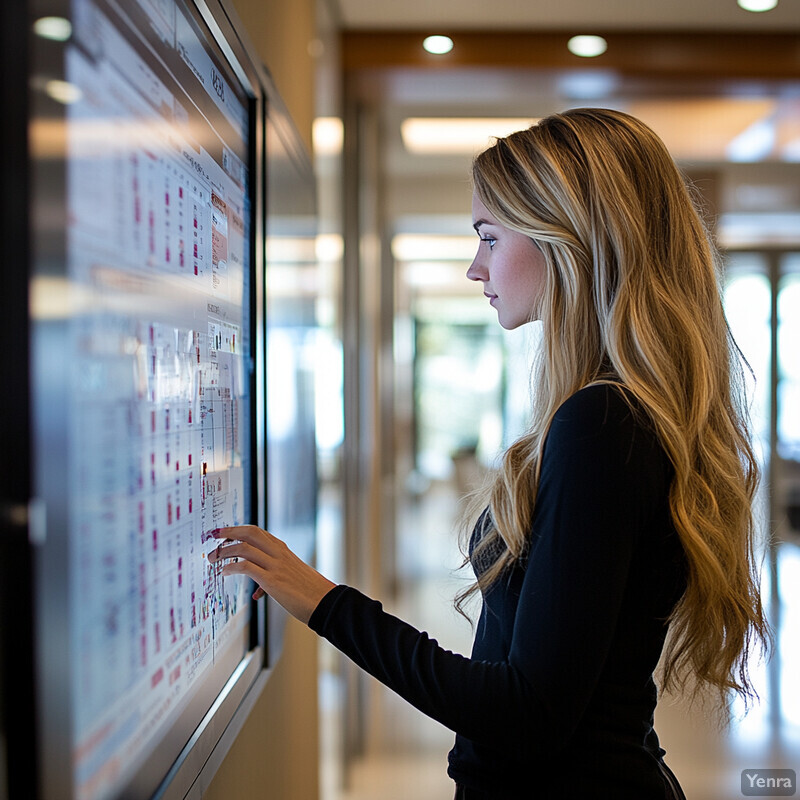
Early implementations illustrate how AI can guide gait rehab decisions. In one study, researchers deployed a decision-support algorithm that learned from over 500 stroke rehabilitation cases. The AI could predict each patient’s likely 6-minute walk distance after a course of therapy, using initial gait parameters and patient characteristics. If the prediction fell short of goals, the system would suggest specific adjustments – for instance, adding ankle dorsiflexion exercises if it detected limited foot clearance, because data showed that helped similar patients improve walking distance. Therapists following these AI suggestions found that some patients made faster gains than expected, validating the approach. Another example comes from Parkinson’s disease care: an AI model integrated gait decline markers (like slowing cadence) with medication data to alert clinicians when a patient might need a therapy change. In a pilot trial, use of this AI alert system led to earlier modifications of treatment and improved mobility outcomes compared to a control group with standard care. Importantly, clinicians retained final say – the AI’s role was to crunch the numbers from myriad past cases and present a data-backed recommendation. As these systems evolve, they are incorporating more factors (strength, balance, cognitive status, etc.) to provide comprehensive guidance. Hospitals are beginning to adopt such AI dashboards in multidisciplinary rehab meetings, where the team reviews the computer’s analysis alongside their clinical impressions. The result is often high confidence in decision-making and a more personalized, evidence-aligned rehabilitation plan for each patient.
19. Motion Quality Scoring Systems
Beyond basic parameters like speed or stride length, AI enables scoring of gait quality on standardized scales. These systems assess how well a person walks in terms of smoothness, symmetry, and stability, and then assign numerical ratings. For example, an AI might output a “gait smoothness score” from 0 to 100 or rate symmetry on a percentage scale, with 100% being perfectly symmetrical walking. This gives therapists an objective way to pinpoint specific deficits (like a low score in limb symmetry) and track improvements. Gait quality scores condense complex biomechanical data into clinician-friendly indexes – similar to how blood pressure provides a snapshot of cardiovascular health, a composite gait score provides a snapshot of locomotor function. In practice, a therapist might see that a patient’s Stability Score improved from 60 to 75 after balance training, reflecting tangible progress. Scoring systems also allow quick comparison to normative data: for instance, indicating a patient’s gait quality is 1.5 standard deviations below healthy peers. With AI handling the heavy data crunching (analyzing joint angles, forces, and timing relationships), these scores can be generated automatically during an assessment. This standardization helps in setting goals (e.g., achieve a symmetry score above 80) and objectively determining when someone’s gait has normalized enough for discharge. In summary, AI-driven gait scoring provides a clear, quantifiable measure of movement quality that enhances clinical insight and communication with patients.
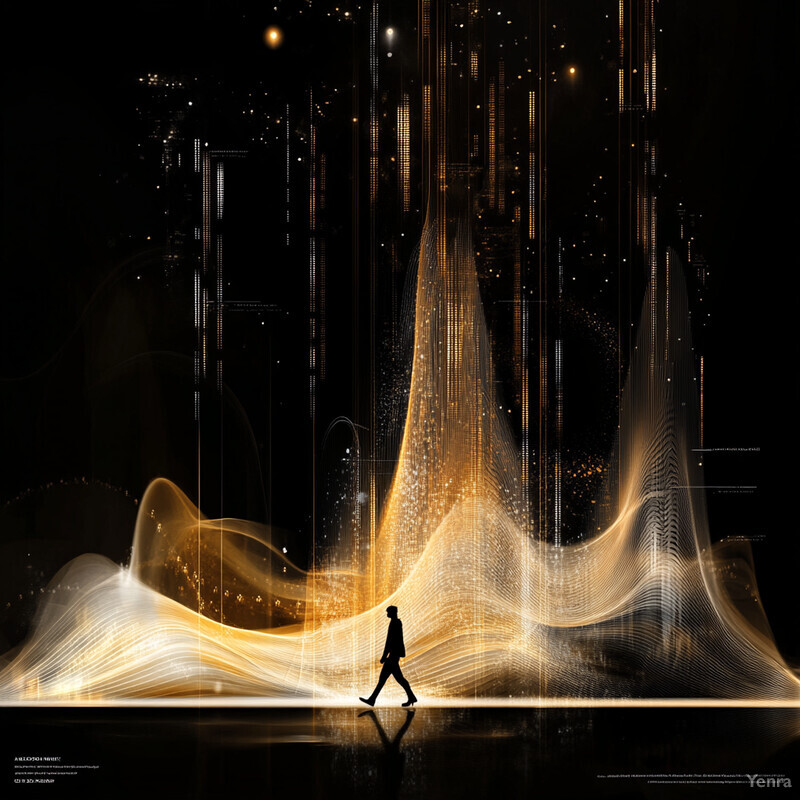
Researchers have developed several gait quality indices using AI and statistical modeling. One example is the Gait Deviation Index (GDI), which combines numerous kinematic deviations into a single number – originally created for children with cerebral palsy, it’s now computable by software in seconds. A high GDI indicates near-normal gait, whereas a low GDI signifies significant pathology. AI algorithms have further refined such indices; for instance, a 2024 study introduced a force-based “gait quality score” for prosthetic leg user】. They measured eight metrics (including weight-bearing symmetry and timing of force application) in amputee patients walking with a powered prosthetic foot. The AI then identified which metrics best distinguished a well-tuned prosthesis from a poorly tuned one, and combined them into a single Gait Quality score. When patients’ prostheses were optimally tuned, their score improved markedly, correlating with smoother and more symmetric force pattern】. In another case, scientists at a gait lab created a “Shriners Gait Index (SGI)” tailored for pediatric patients, using machine learning to weigh various kinematic errors; in validation, the SGI closely tracked expert clinicians’ judgments of gait improvement on a numeric scale, but with far greater consistency. These scoring systems are already being applied in practice: for example, rehab centers sometimes report a composite gait score in addition to traditional measures, which helps both therapists and patients appreciate overall progress. As AI allows these scores to be calculated objectively and repeatedly, clinicians gain a reliable outcome measure for gait quality – making it easier to document improvements (like “quality score up 20 points”) and to compare the effectiveness of different treatments in research and clinical audit】.
20. Population-Level Insights and Predictive Modeling
By aggregating gait data from thousands of individuals, AI can uncover broad patterns and improve protocols for patient care. On a population level, machine learning can identify which gait factors are the strongest predictors of certain outcomes – for instance, finding that reduced gait speed and high step-time variability together strongly predict future falls in older adults. These insights inform public health and clinical guidelines (such as fall screening criteria). Additionally, analyzing large datasets allows AI to stratify patients into subgroups that respond differently to treatments, guiding personalized medicine at scale. For example, an AI might reveal that stroke patients with very asymmetric gait early on benefit more from one type of walking therapy, whereas those with slow-but-symmetric gait do better with another – such knowledge helps develop decision trees for clinicians managing new patients. On the research side, pooling multi-center gait data (across countries and demographics) via AI yields normative references and expected recovery trajectories, which clinicians can use as benchmarks. Predictive modeling on population data can even forecast healthcare needs: authorities could predict how many people will require gait assistance devices in 5 years based on current gait trends in the population. In summary, AI applied to big gait datasets provides high-level insights that refine best practices, shape preventative strategies (like community fall prevention programs targeting those flagged by models), and ensure that clinical protocols evolve based on the latest evidence drawn from diverse patient populations.
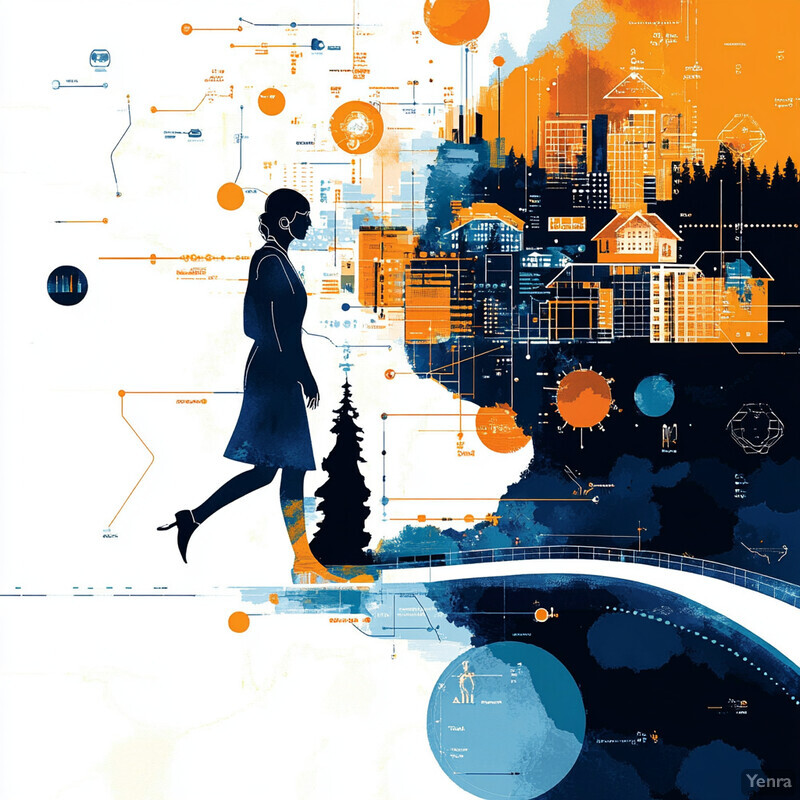
Large-scale initiatives illustrate these benefits. One prominent project is the European Mobilise-D consortium, which is collecting continuous gait data from 2,400+ participants across multiple conditions (Parkinson’s, COPD, MS, etc.) using wearable sensor】. Their machine learning analyses have already provided important insights – for instance, they found that a 10% decline in real-world walking speed over a year was associated with a significantly higher risk of hospitalization in COPD patients, information now being used to enhance monitoring guideline】. In another example, researchers pooled gait and outcomes data from six global cohorts (part of a major validation study) and used AI to establish cut-off values for digital gait metrics that predict clinically meaningful event】. One result was a recommended threshold for daily walking activity (measured by wearable) below which older adults had markedly increased fall rates – these findings are influencing fall prevention screening recommendations internationally. Moreover, population data allows predictive modeling for health economics: a recent machine learning study using national survey data predicted that a modest improvement in average gait speed among seniors could correlate with millions in healthcare savings by reducing fall injuries, guiding public health initiatives to encourage walking programs. These examples demonstrate that when AI analyzes gait at the population level, it not only advances scientific understanding (revealing how gait links to broader health outcomes) but also directly translates into improved protocols and preventive measures that benefit entire communities.