1. Predictive Analytics for Demand and Supply Forecasting
AI-driven predictive analytics uses historical data (sales, weather, market trends) to forecast demand and align production and logistics in food supply chains. By analyzing large datasets, these systems help prevent both stockouts and overproduction. Accurate forecasts reduce waste (e.g. perishable spoilage) and lower holding costs. These tools continuously update predictions as new data arrives (inventory levels, weather, market signals). Better forecasts improve responsiveness to sudden changes and enhance overall traceability of inventory movements.
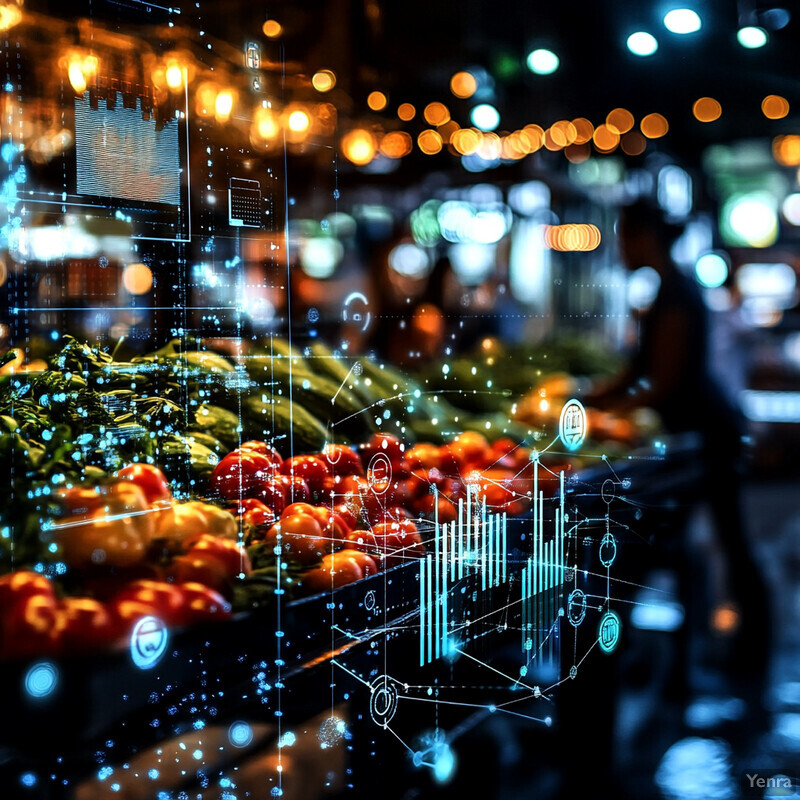
Implementing AI demand-forecasting has yielded measurable improvements in accuracy and costs. For example, one industry report notes AI-based forecasting reduced forecast errors by up to 50%. A recent case study in perishable goods showed an AI-driven two-stage forecasting model cut error dramatically (weighted RMSE dropped from 238.18 to 61.57) compared to traditional methods. Firms using AI-driven supply chains have reported 15–35% reductions in logistics costs and inventory levels due to more precise planning. These gains directly address the fact that roughly one-third of food produced globally is wasted annually; better demand forecasts help synchronize supply to actual consumption and maintain traceability of surplus and shortages.
2. Computer Vision for Quality Inspection
AI-powered computer vision automates the visual inspection of food items, identifying defects and contaminants more consistently than manual checks. Cameras and image-recognition algorithms detect issues like discoloration, incorrect shape, or foreign objects on production lines. This real-time assessment ensures substandard items are removed before packaging. Automated visual inspection scales across high volumes, improving throughput and recording quality data for traceability. Such systems also verify label accuracy or detect undercooked items by analyzing images, enhancing food safety.
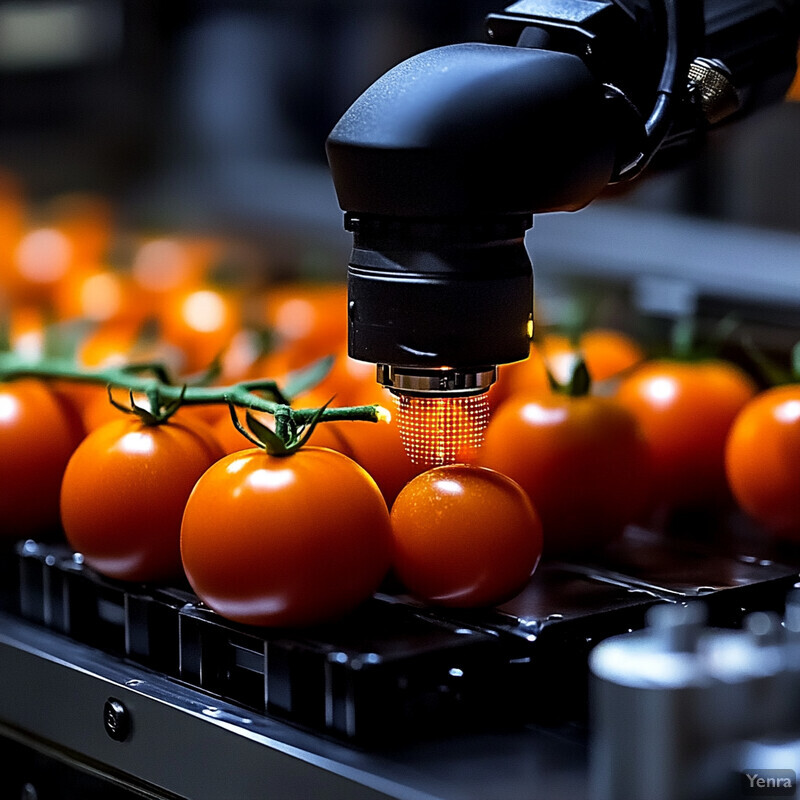
Modern computer-vision systems achieve very high accuracy in quality checks. A 2025 trial reported ~90% detection accuracy on fruits (89% for mangoes, 92% for tomatoes) using advanced vision algorithms, with deep-learning models reaching 94–95% accuracy. Automated inspection is much faster than human checks, significantly increasing throughput. For example, one analysis notes automated vision “increases throughput” and “helps maintain high quality, reduce waste”. In specialized sectors like seafood processing, over half of quality-inspection software products now use AI (including computer vision) to verify quality. Computer vision can also detect contaminants and labeling errors (e.g. missing allergen warnings) on the fly, preventing recalls and ensuring regulatory compliance.
3. Intelligent Sensor Integration
IoT sensors (temperature, humidity, GPS, gas sensors, etc.) are integrated throughout farms, trucks, and storage facilities. AI analyzes this continuous sensor data to monitor conditions (e.g. cold chain temperatures) and trigger alerts. In fields, soil moisture and nutrient sensors guide irrigation and fertilization. In transport, embedded trackers log precise location and environment. Combining diverse sensor streams with machine learning creates a “digital nervous system” for the supply chain. This ensures that any out-of-range condition (like a broken freezer) is immediately detected, improving traceability of product conditions from origin to delivery.
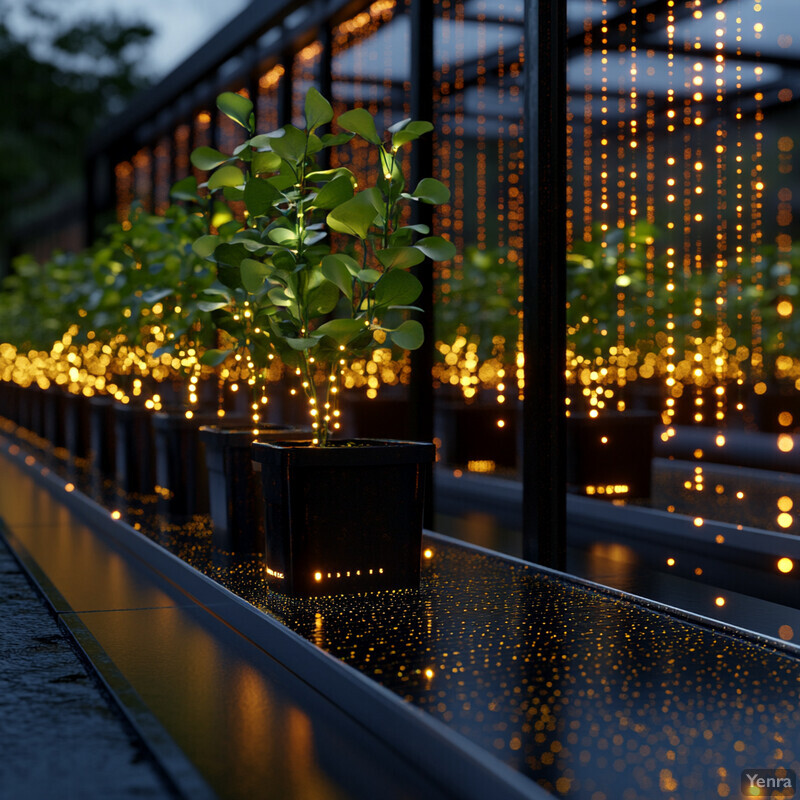
Integrating AI with IoT sensor networks has enabled large-scale monitoring and optimization. For instance, one project fused real-time data from thousands of soil moisture sensors and weather stations across wide agricultural areas, enabling AI-driven crop management at scale. In cold chains, smart sensors on pallets provide alerts if temperature or humidity drift, preventing spoilage e3s-conferences.org . IoT tracking lets companies see location and condition of goods farm-to-fork e3s-conferences.org . AI models then process this data to predict problems; e.g., machine learning applied to sensor logs can flag a likely refrigeration failure before spoilage occurs. Such systems have shown measurable benefits: studies report up to 20–30% improvements (like water savings via precision irrigation or yield gains) from AI-driven sensor insights in agriculture.
4. Blockchain Integration for Immutable Records
Blockchain provides an unchangeable ledger for all transactions in the food chain. AI complements this by automatically validating and inputting data, and by spotting suspicious entries. For example, sensor or image data can be hashed into blockchain blocks, ensuring records of origin and handling are tamper-proof. AI algorithms audit these records to detect anomalies (e.g. gaps or inconsistencies in time/location data). Together, blockchain and AI aim to offer end-to-end trust: once a record (say, a temperature log from a truck) is stored, it cannot be altered, and AI tools continuously monitor the chain for signs of fraud or error. This integration makes every handoff more transparent and automatable.
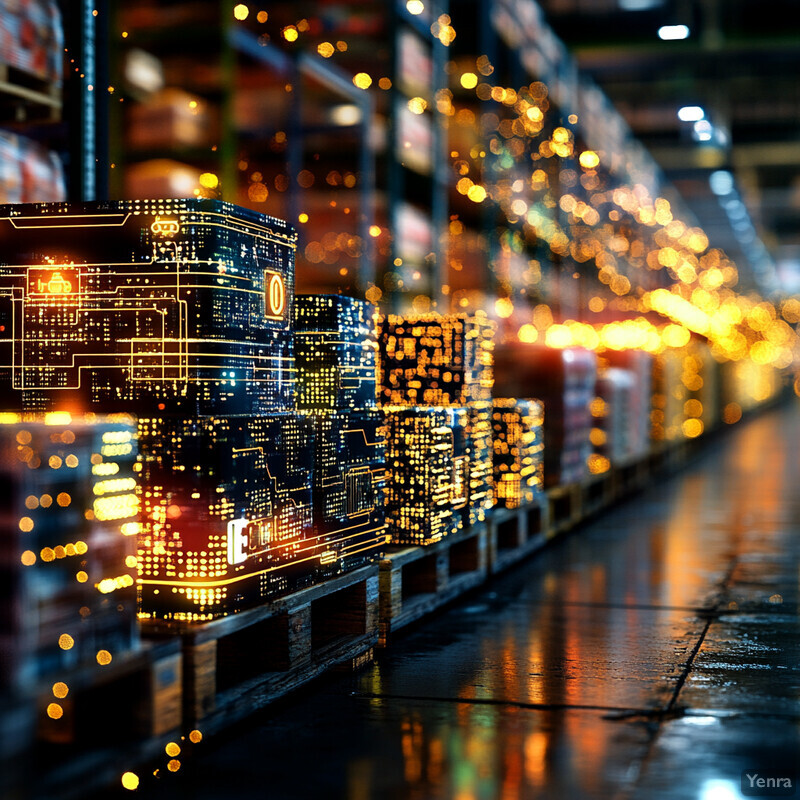
While industry interest is high, actual adoption remains modest. A recent survey finds that most food companies have yet to implement blockchain for traceability, noting “a low adoption rate of blockchain technology amongst organizations in the food supply chain”. However, early pilots demonstrate potential value. For example, Walmart’s blockchain pilot traced produce origins to 2.2 seconds (versus days manually) and had processed over 1,500 items by 2022. Other companies (e.g. in honey and coffee) are combining blockchain with AI: startups have built solutions where AI flags possible data tampering, and blockchain stores supplier documents and certificates immutably. In such systems, if AI detects, say, a mislabeled batch, the blockchain audit trail makes it easy to trace the incident back to a specific supplier or shipment. Real-world examples include TagOne’s AI+blockchain platform for authenticity verification, and Transparent Path’s sensor + AI + blockchain cold-chain tracking solution.
5. Dynamic Routing and Fleet Optimization
AI optimizes delivery routes and schedules in real time, adjusting for traffic, weather, and demand fluctuations. Transportation fleets (trucks, ships, drones) are directed by algorithms that minimize distance and time. In practice, AI may reroute vehicles on-the-fly to avoid congestion or add stops if a sudden urgent order appears. This ensures faster deliveries and fresher products. Fleet sensors feed vehicle health and location data into AI, helping plan maintenance and predict breakdowns, which also contributes to on-time deliveries. Overall, dynamic routing ensures that goods move through the supply chain as efficiently as possible.
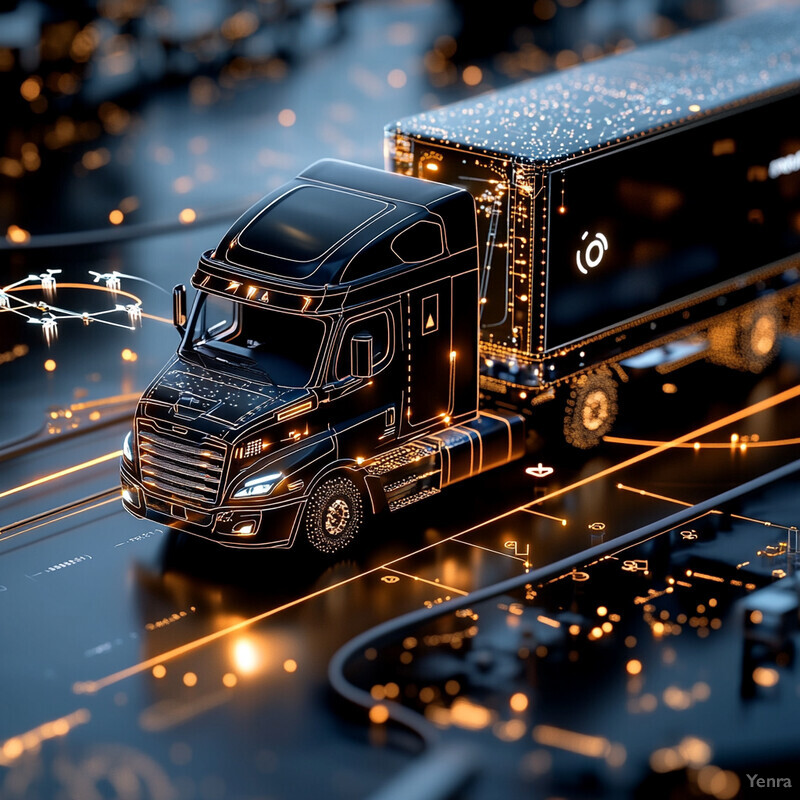
Companies see tangible benefits from AI-driven routing. One analysis reports firms using AI fleet management saw a 15–20% reduction in fuel costs and 30–40% drop in maintenance expenses. Another source notes that AI can cut fuel use by up to 15% via optimized routes. Food logistics experts highlight that AI systems ingest historical traffic and weather data to reroute trucks and avoid delays. Industry cases include Amazon and logistics providers using AI for last-mile optimization, which has improved on-time rates. Moreover, predictive routing allows fleets to increase capacity: for example, by reducing delivery delays and disruptions, companies can complete more deliveries per day and better match supply with demand.
6. Supplier Risk Scoring and Due Diligence
AI systems continuously assess supplier risk by scoring factors like financial health, compliance records, geography, and news reports. They integrate data (news feeds, sanctions lists, ESG scores, etc.) to flag high-risk suppliers (e.g. with labor or safety issues). Machine learning models assign a risk score to each supplier, prioritizing audits or alternative sourcing. Over time, these scores update automatically as new data appears. Such AI-driven due diligence helps buyers vet new partners quickly and keep tabs on existing ones, maintaining traceability of supplier performance and compliance.
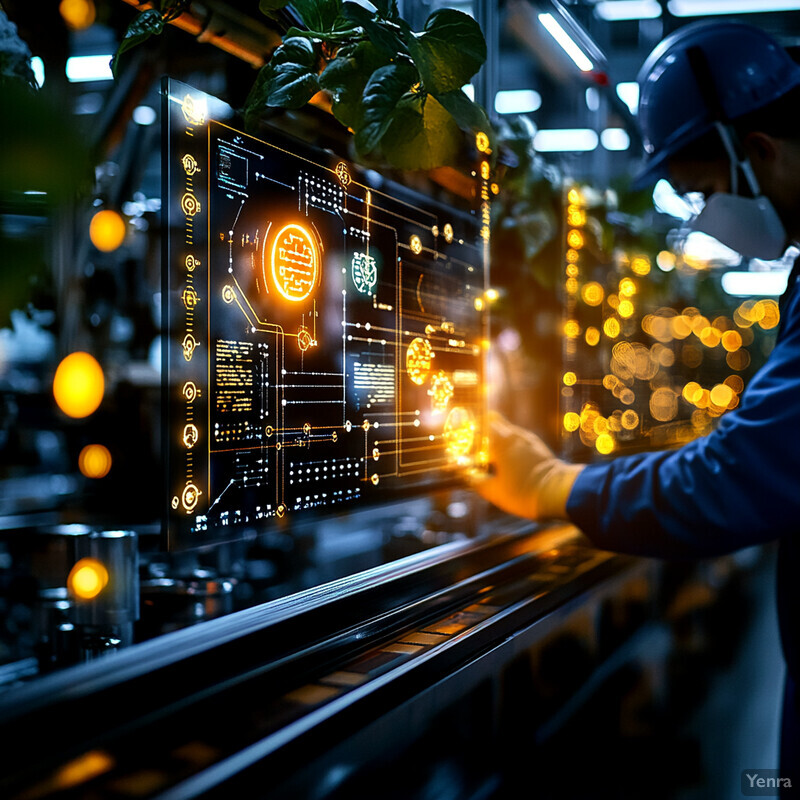
Expert analyses note that AI/ML enhances supplier risk management by enabling more refined risk scores and predictive alerts. For example, Gartner’s 2023 report highlights that embedded AI allows solutions to produce “more-refined financial risk scores” and begin predictive risk analysis for suppliers. In practice, AI-driven procurement tools are starting to mine unstructured data (like social media or news) for emerging issues. This evolution in Supplier Risk Management aims to address CIO needs for supply chain resiliency. No recent public data quantifies the improvement in risk scoring accuracy or outcomes, but industry reports agree AI is increasingly applied in supplier risk evaluation.
7. Automated Compliance Monitoring
AI automates monitoring of regulatory and quality compliance across the supply chain. Systems scan records and sensor logs to ensure processes (like HACCP checks or temperature controls) follow standards. For instance, AI can flag if a cold-storage shipment’s log deviates from allowed ranges. It can also verify documentation (e.g. certifications, label requirements) through NLP. Automated compliance tools provide real-time dashboards and alerts, so companies fix issues as soon as they appear. By digitally tracking compliance actions, these tools create an auditable record (dates, actions) that enhances overall traceability.
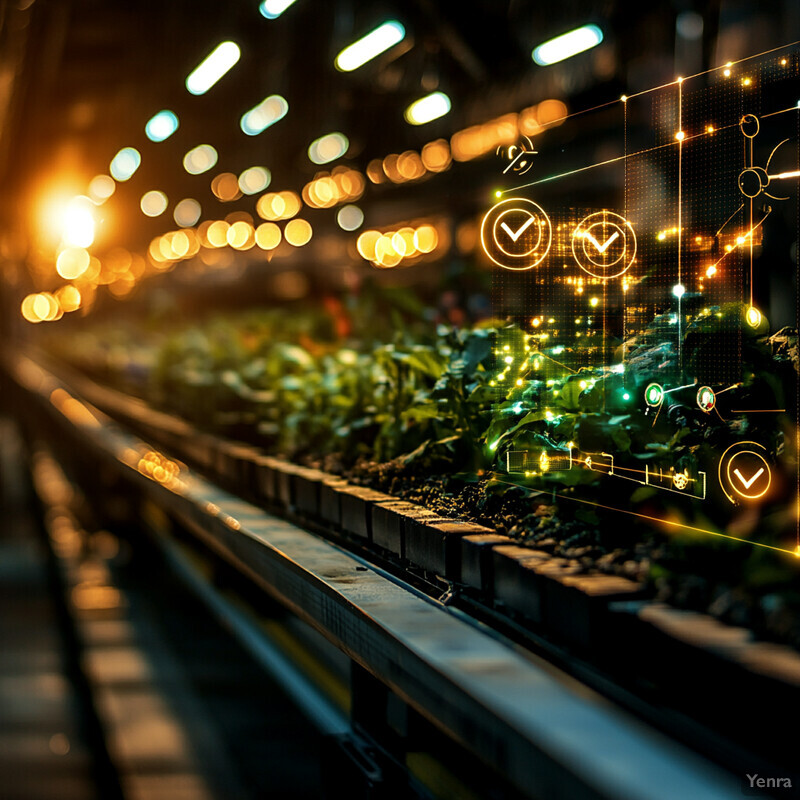
Industry platforms emphasize features such as IoT-triggered alerts for corrective actions and automated audit trails, reflecting how AI/IoT is used in practice to support compliance.
8. Precision Agriculture Data Integration
In the field, AI merges data from drones, satellites, sensors, and machinery to guide farming decisions. For example, AI analyzes satellite imagery and soil data to determine optimal planting locations or harvest times. Drones and robots equipped with sensors feed AI models data on crop health (NDVI imagery, moisture levels). This integrated data helps trace produce back to specific field conditions and farming practices. By precisely managing inputs (water, fertilizer), farmers improve yield and quality, making each batch of product more traceable to documented growing conditions.
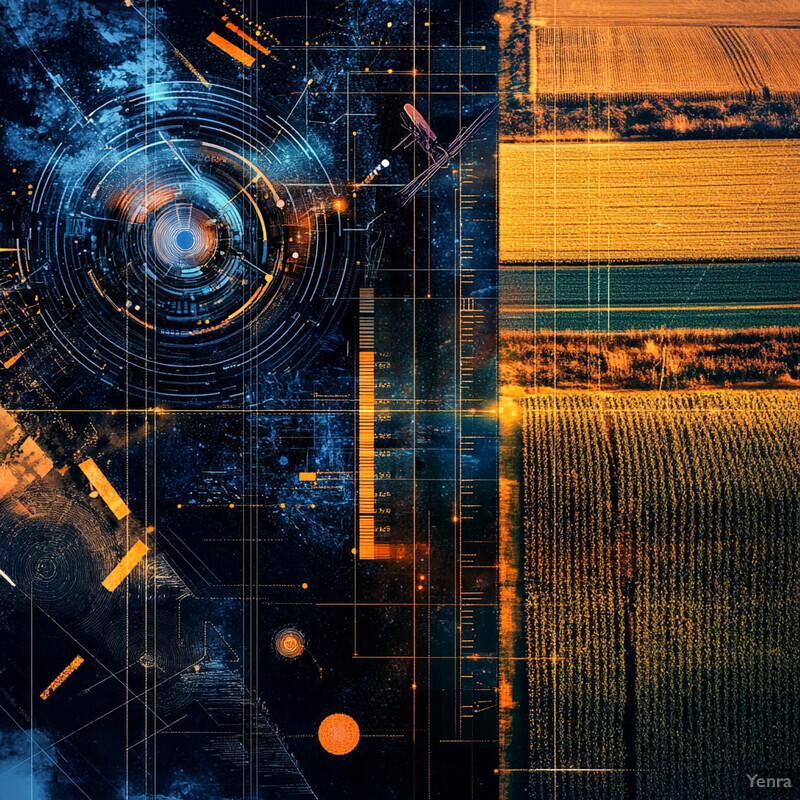
AI-driven precision ag tools can substantially improve efficiency. One analysis attributes 25–35% higher yields to AI crop monitoring (via drones/satellite and disease detection), along with reduced chemical use. Similarly, AI-controlled smart irrigation systems can cut water use by 20–30%. Other studies report AI-based yield forecasting can reduce waste by 10–20% by better matching harvest to demand. These gains demonstrate how integrating multiple data sources with AI not only boosts productivity but also creates detailed digital records of farming conditions for each harvested lot.
9. Automated Shelf-Life Prediction
AI models predict the remaining shelf life of perishable products by analyzing environmental data and product quality indicators. Sensor data (temperature history, gas concentrations, humidity) from storage or transport are fed into machine learning models trained on spoilage experiments. Computer vision can also assess appearance (color, texture) to gauge freshness. These predictions let supply chain operators make dynamic decisions: for instance, expediting shipments of items nearing expiration or routing them to nearby markets. This proactive approach preserves safety and quality while enhancing traceability of how long items have been in the chain.
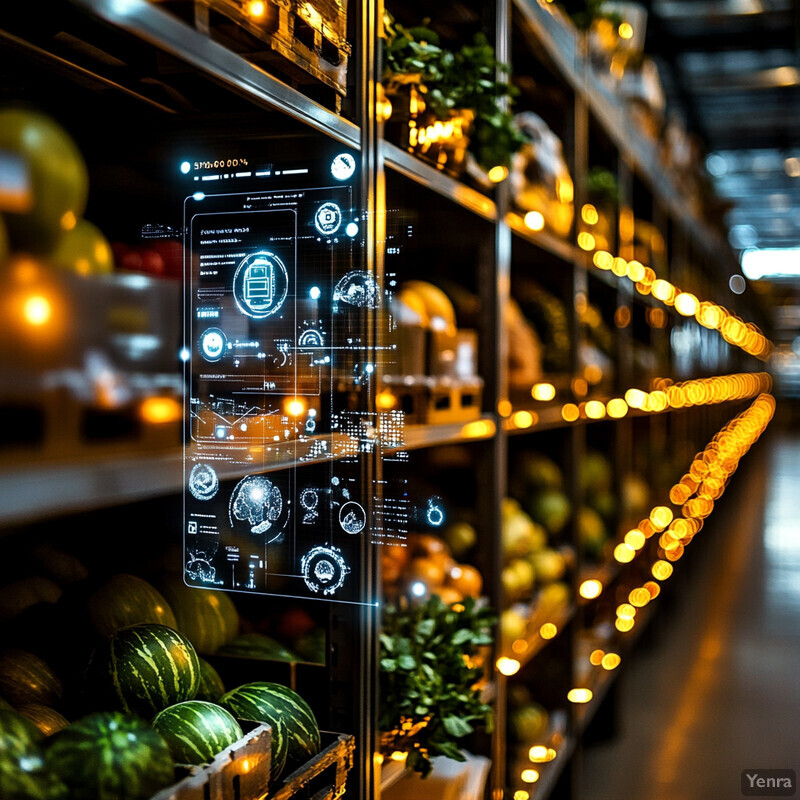
Recent trials show high prediction accuracy using AI. For example, machine learning models have predicted shelf life for produce with 78–96% accuracy under test conditions. In blueberries, various algorithms achieved 90–94% accuracy in forecasting freshness based on environment sensors. One study reported AI could predict fruit expiry within about 0.9 days of actual shelf life with nearly 89% accuracy. Such results indicate AI can effectively estimate degradation rates.
10. Enhanced Recall Management
AI streamlines product recalls by rapidly identifying affected lots and notifying stakeholders. When a contamination is detected, AI uses traceability data (batch numbers, shipment logs) to pinpoint which products might be at risk. Automated workflows then notify distributors, retailers, and consumers via digital channels. Machine learning can also analyze past recall incidents to improve future recall plans. Through simulation, AI helps determine which products to remove and the optimal recall strategy, minimizing time and scope while ensuring all tainted items are accounted for.
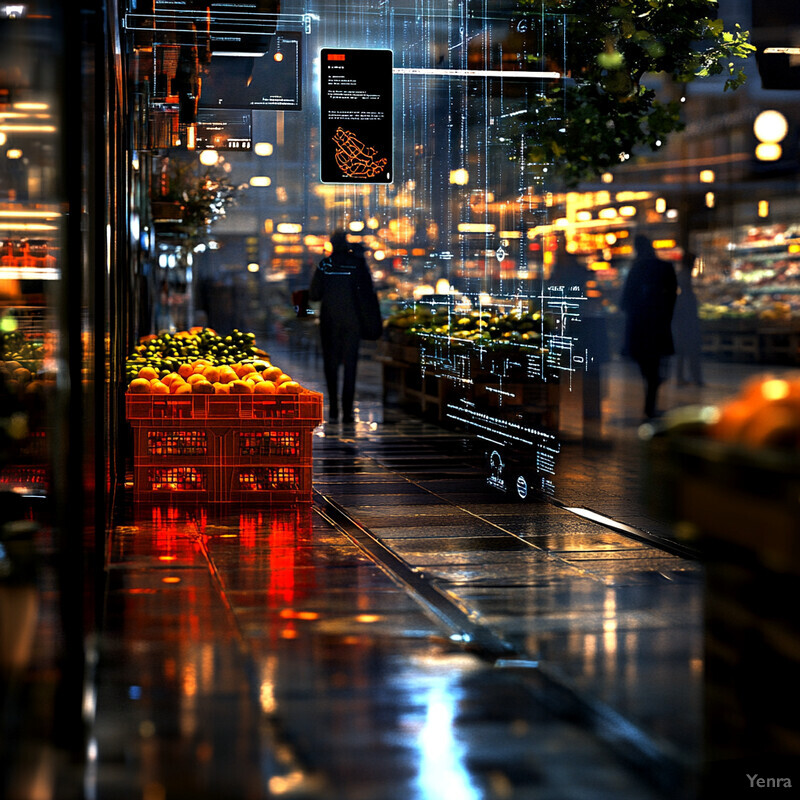
Food recalls have surged, emphasizing the need for smarter management. Recent reports show recalls in food (and other industries) rose over 20% from 2020 to 2023, reaching a seven-year high in 2023. Over 70% of manufacturers have experienced a recall in the last five years, with each recall averaging ~$100 million in cost. Analysts attribute part of this increase to better detection via AI and digital tools; for example, advanced traceability and analytics make it easier to detect issues like pathogen contamination quickly. AI-enabled recall systems can therefore significantly cut the time needed to identify and isolate affected products, though exact time-savings metrics for 2023–24 have not been publicly quantified.
11. Predictive Maintenance of Handling Equipment
AI monitors equipment health (refrigeration units, conveyors, forklifts) to predict failures before they occur. IoT sensors track vibration, temperature, and usage hours; AI analyzes this to schedule proactive maintenance. This reduces unplanned downtime and avoids spoilage from equipment failure (e.g. freezer breakdown). In warehouses and farms, such predictive maintenance systems generate work orders automatically when a potential fault is detected. Over time, AI learns equipment patterns to optimize maintenance intervals. This ensures that traceability isn’t broken by unexpected downtime that could disrupt tracking processes.
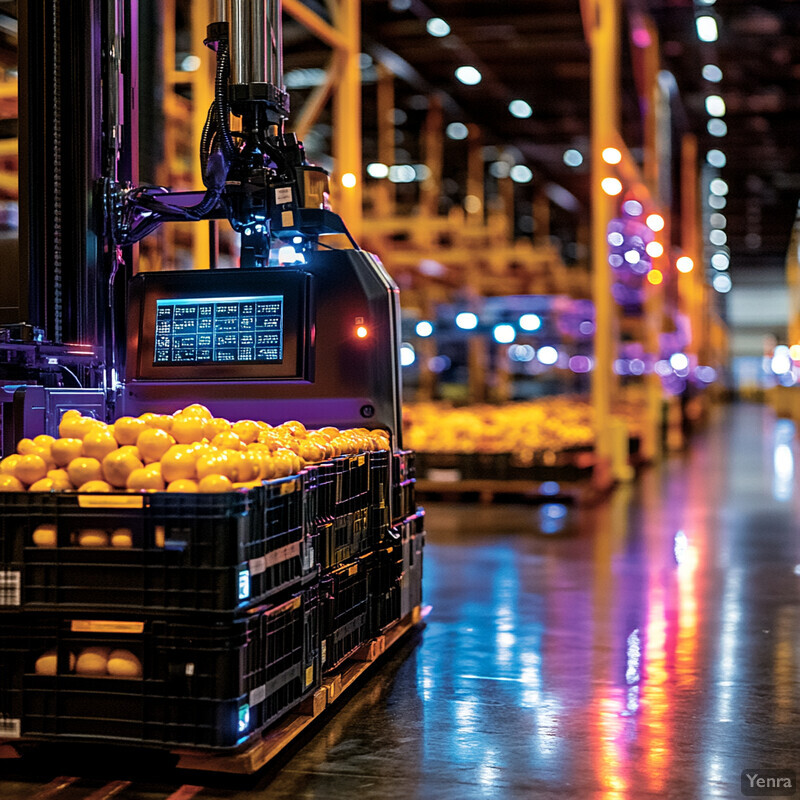
Companies report substantial downtime reductions with predictive AI. For example, one Siemens case study showed 20% less unplanned downtime after implementing AI-based monitoring of production equipment. In cold chains, AI-enabled controls trigger maintenance alerts (e.g. if a refrigerator’s defrost cycle is abnormal), preventing losses. Industry analyses also highlight growing investment: the global predictive maintenance market (covering various sectors) was estimated at $10.2 billion in 2023, reflecting broader use of AI in equipment monitoring. Specific to food logistics, IoT analytics prevent product damage by forecasting equipment failures ahead of time.
12. Better Inventory Management
AI continuously balances inventory by integrating sales data, forecasts, and supplier lead times. Systems recommend optimal reorder quantities and timing, preventing overstock or stockouts. In food retail and warehousing, AI-driven demand sensing adjusts inventory dynamically, for example by creating orders when sales spikes are predicted. Real-time integration with warehouse systems ensures perpetual inventory tracking. This leads to leaner stock levels and faster stock turns, all while maintaining traceability of product movements (each batch is logged as it arrives and departs).
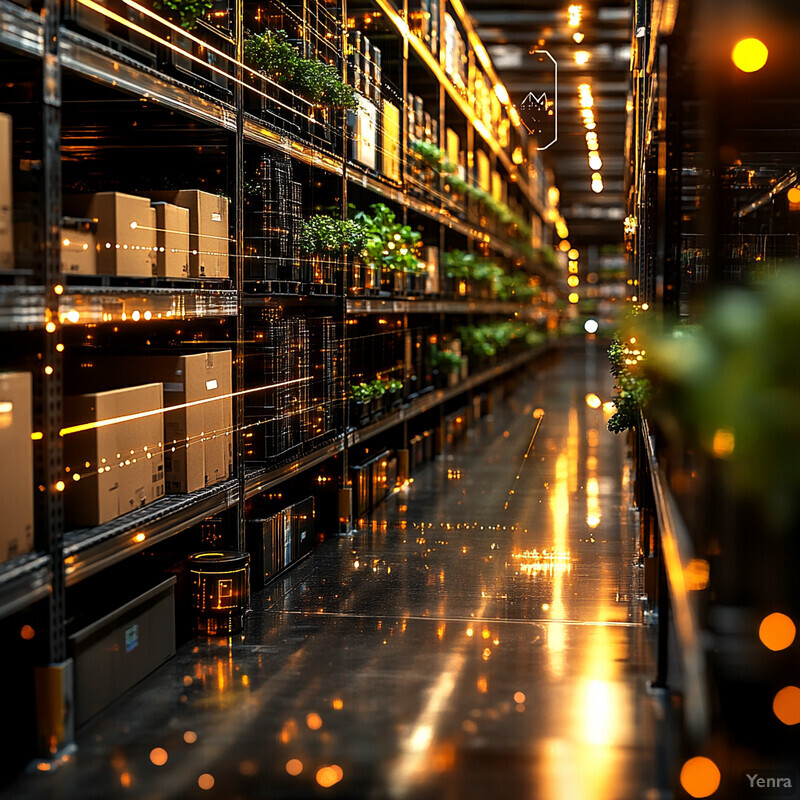
AI-assisted inventory has shown clear benefits. Firms implementing AI planning have reported up to a 35% improvement in inventory efficiency, partly by avoiding excess stock. AI forecasting also helps minimize waste: a Cogent Infotech analysis notes that by predicting demand more accurately, AI systems “ensure timely availability” of products and reduce expired waste. In practice, retailers using AI have achieved higher on-shelf availability and lower spoilage – though exact figures vary by case. Overall, companies can match stock to demand more precisely, cutting carrying costs while still tracking every item through the supply chain.
13. Identifying Sustainability and Ethical Sourcing
AI analyzes geospatial and environmental data to verify that sourcing practices meet sustainability standards. Satellite imagery and AI models detect deforestation or illegal land use at a supplier’s farms. For labor practices, AI examines news and compliance databases for child/forced labor risk. These insights score suppliers on ESG criteria. By continuously monitoring factors like carbon footprint or water use (via IoT or supply-chain data), AI helps companies source more ethically. Whenever a supplier falls out of compliance, the traceability system logs it, allowing rapid substitution with an approved alternative source.
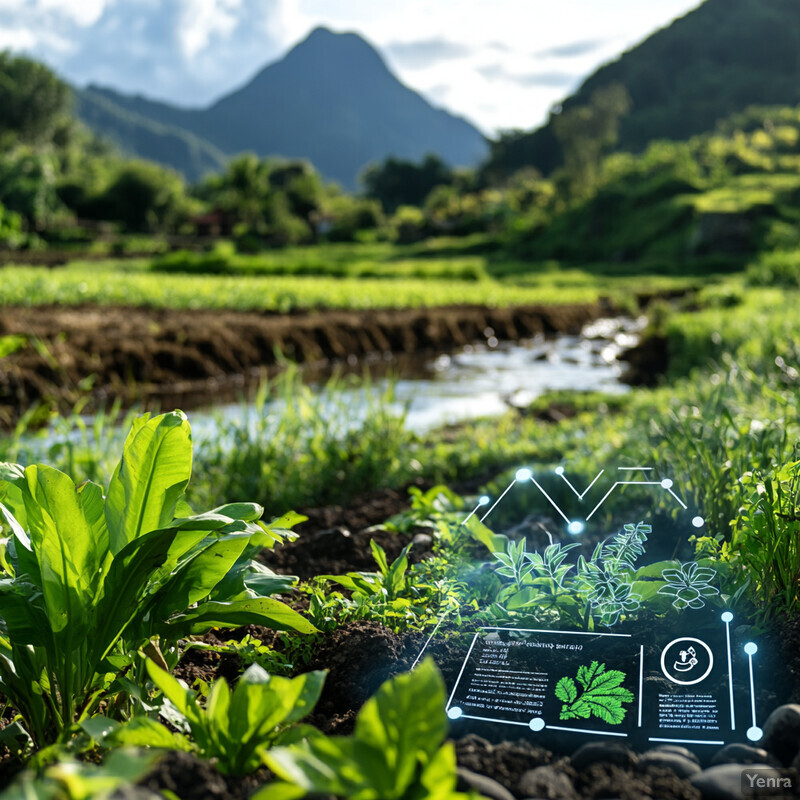
AI-driven sustainability tools are now vital under new regulations. Agriculture drives 70–80% of deforestation globally, so food companies face EU rules requiring proof of deforestation-free sourcing. In response, firms deploy AI with satellite data to audit supply chains. For instance, a new platform (TRACT) uses AI to validate farm geolocation data and check for deforestation, aiding compliance with the EU Deforestation Regulation. The platform even integrates alerts from Global Forest Watch and labor-risk data from universities, applying AI to flag non-compliance. Such tools are in active use by major commodity companies to ensure sustainable timber, soy, coffee and cocoa sourcing.
14. Continuous Supplier Performance Monitoring
AI continuously evaluates supplier KPIs (delivery times, quality defects, audit scores) to monitor performance. Data feeds from logistics and ERP systems allow AI to detect degradation in supplier reliability or quality. Dashboard analytics highlight trends (e.g. a supplier’s on-time rate falling). Some platforms use AI to benchmark suppliers against peers. By scoring suppliers on service, quality and sustainability metrics, companies can trace how supplier performance evolves over time. This enables proactive management: if a key supplier shows signs of risk, the system can automatically generate alerts or suggest backup plans, preserving continuity of the traceable chain.
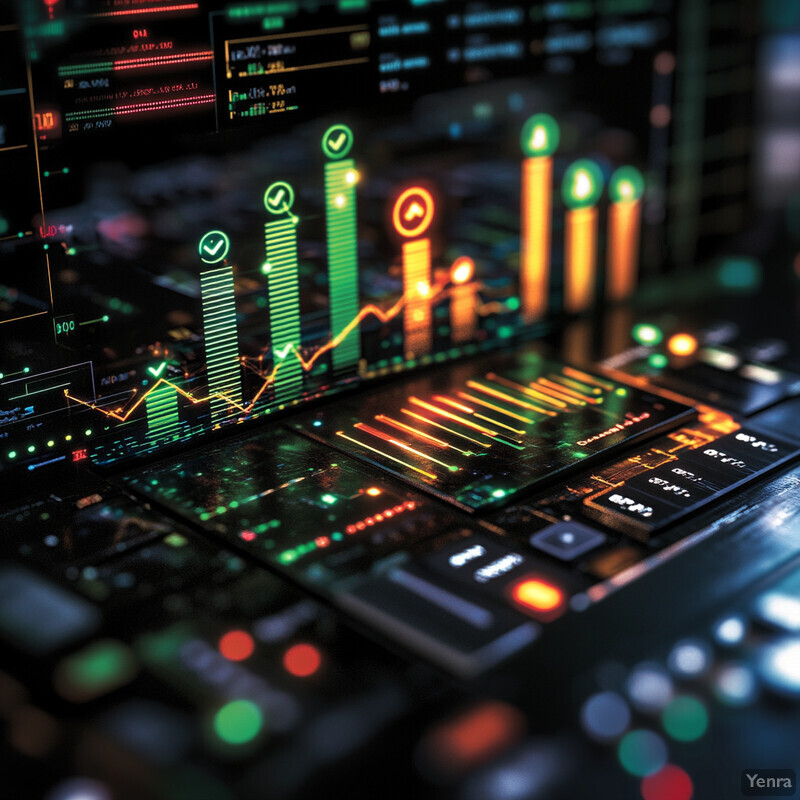
Industry experts emphasize AI’s role in supplier monitoring. For example, a supply management blog notes that AI can “analyze various data points, including supplier performance metrics and compliance records” to give companies timely insights on their suppliers. Predictive analytics also help by forecasting which suppliers might fail to meet demand or standards. While specific performance gains (e.g. percentage of on-time deliveries improved) are not publicly reported, surveys indicate over 70% of executives now expect AI to enhance visibility and collaboration with suppliers. In short, AI-enabled systems make supplier scorecards more dynamic and precise, although published studies quantifying these improvements are limited.
15. Smart Packaging Integration
Packaging is becoming “smart” by embedding sensors and digital IDs. Examples include QR codes linked to databases, RFID tags with temperature or gas sensors, and even intelligent inks that change color if conditions deviate. AI in packaging reads these signals – for instance, image recognition can scan a package label to confirm the contents match the digital record. Sensors in the package can stream condition data (e.g. temperature history) which AI analyzes to verify the product remained in spec. This makes each package itself a node in the traceability network, carrying its own data that can be decoded at every checkpoint (factory, warehouse, retailer, consumer).
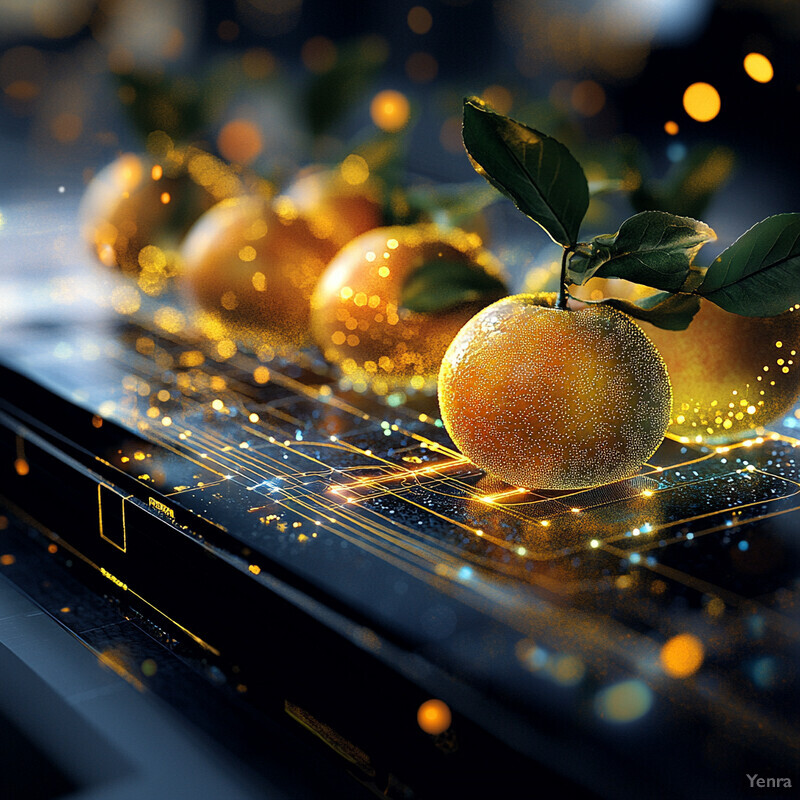
The smart packaging market is growing rapidly, reflecting wider adoption of these technologies. The global smart packaging market was valued at about $21.3 billion in 2023, with an expected compound annual growth rate around 6–8%. This includes innovations like sensor-enabled labels and blockchain-linked QR codes. Industry reports cite cases where brands use intelligent labels to provide consumers with traceability info (e.g. a scan revealing origin farm and batch). Early pilots in cold-chain logistics have shown that sensor-equipped packages can catch temperature excursions in transit, though precise impact data (like spoilage rate reduction) from 2023–25 trials is still emerging.
16. Geospatial Intelligence and Localization
AI-powered geospatial analysis uses satellite and mapping data to localize supply chain operations and assess environmental risks. Companies overlay supply routes and facilities on maps to evaluate climate and geopolitical factors. For instance, AI models predict how a hurricane could disrupt a port of entry, allowing rerouting. Other applications include mapping deforestation hotspots or water stress areas to avoid sourcing from those locations. Localization also means aligning products with local regulations and risks: AI can analyze zoning and infrastructure (e.g. port capacities) to optimize logistics and ensure all network nodes are mapped and monitored.
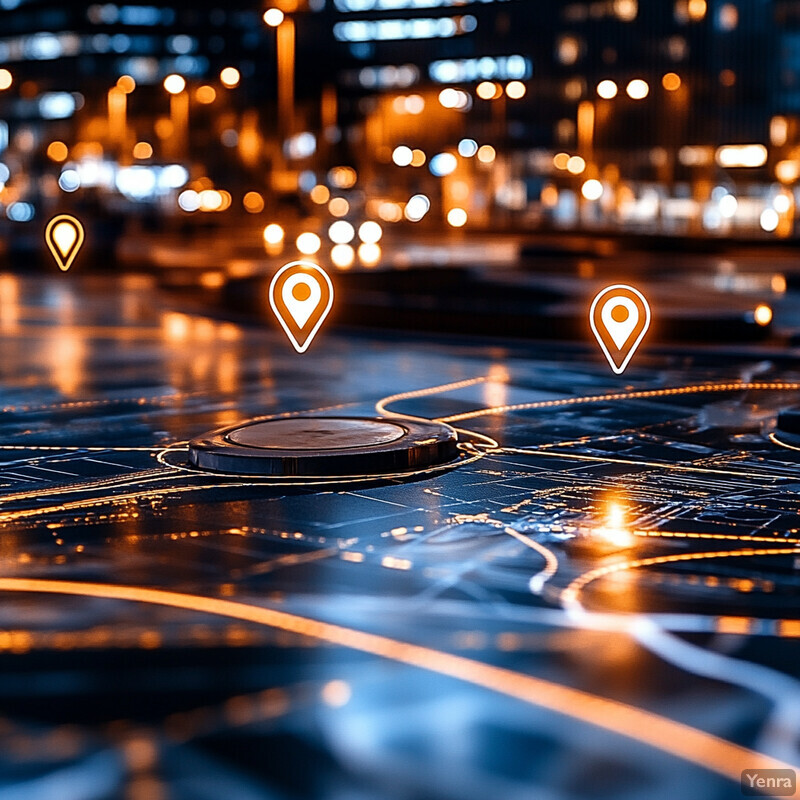
Few companies have fully matured supply-chain geospatial programs. One report finds only 10% of firms reach a high level of “supply chain maturity” by using advanced analytics and location intelligence. However, leading adopters demonstrate the potential: for example, the IMF’s PortWatch uses GIS and satellite data to track over 100,000 ships at more than 1,400 ports, giving real-time alerts on port congestion or weather impact. Major firms also use geospatial AI: a global pulp and paper company uses satellite imagery and AI to monitor its timber suppliers’ forests for sustainable logging. These cases illustrate how location data coupled with AI enhances traceability of goods through environment and geography.