1. Enhanced Return Forecasting
AI-driven predictive models use machine learning algorithms (such as gradient boosting, random forests, or deep neural networks) to better estimate future asset returns by integrating complex, high-dimensional datasets, leading to more accurate forecasts than traditional linear models.
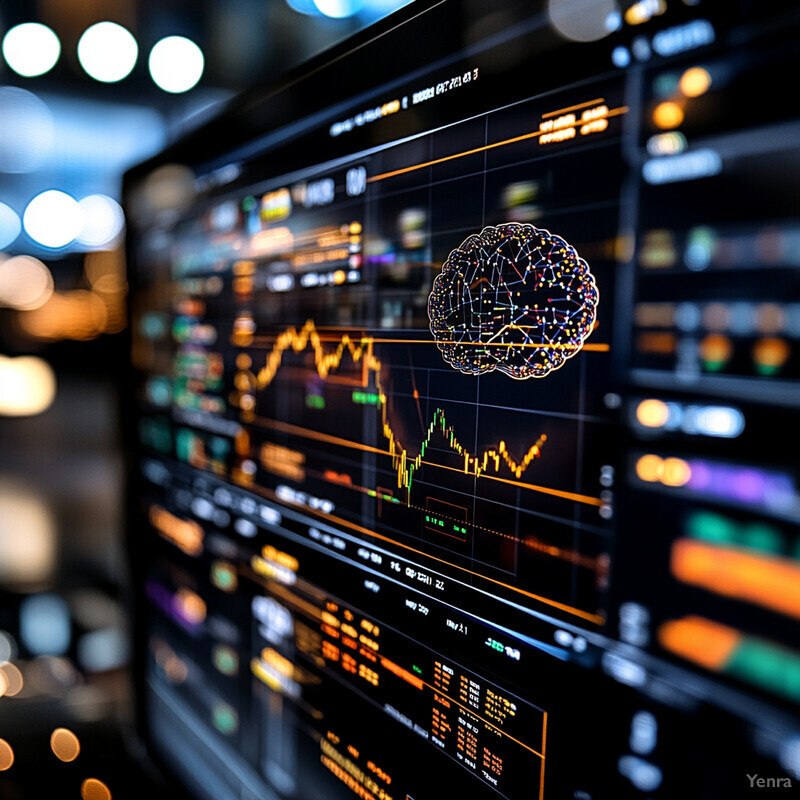
Traditional return prediction methods, often limited by linear assumptions and small feature sets, struggle to capture the complexity of modern financial markets. AI-driven models, such as gradient boosting machines, random forests, and deep neural networks, excel at detecting subtle, nonlinear relationships within vast and diverse datasets. By incorporating not only historical price and volume data but also market-wide indicators, alternative datasets, and even investor sentiment, these models can produce more accurate and robust return forecasts. This improvement in predictive power allows portfolio managers to make better-informed allocation decisions, ultimately enhancing their ability to achieve superior long-term performance.
2. Risk Modeling and Volatility Estimation
Advanced AI techniques can identify non-linear relationships and dynamic patterns in volatility and risk metrics, improving models for Value-at-Risk (VaR), Conditional VaR (CVaR), and other tail-risk measures, ultimately helping managers construct more stable portfolios.
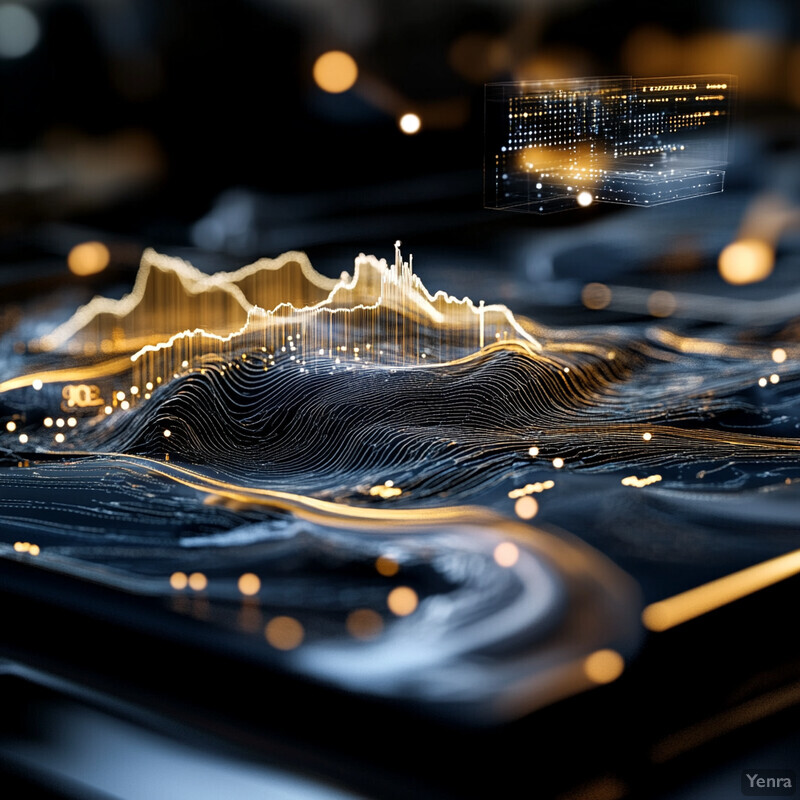
Risk is central to any investment decision, and understanding its behavior requires sophisticated tools beyond traditional variance-covariance approaches. AI-enhanced models can dynamically assess volatility, skewness, kurtosis, and tail-risk exposures by processing a wide range of inputs, including market microstructure data, macroeconomic indicators, or textual information from news sources. Machine learning algorithms can uncover complex, nonlinear patterns in volatility and can adjust their estimations in real-time as market conditions evolve. This leads to more accurate Value-at-Risk (VaR), Conditional VaR (CVaR), and other distribution-based risk measures, enabling portfolio managers to construct portfolios that are more resilient to sudden market shocks and better aligned with an investor’s risk tolerance.
3. Dynamic Asset Allocation
Reinforcement learning and adaptive algorithms can continuously learn from evolving market conditions, adjusting portfolio weights in real-time or near real-time to capitalize on market shifts and enhance performance under changing economic regimes.
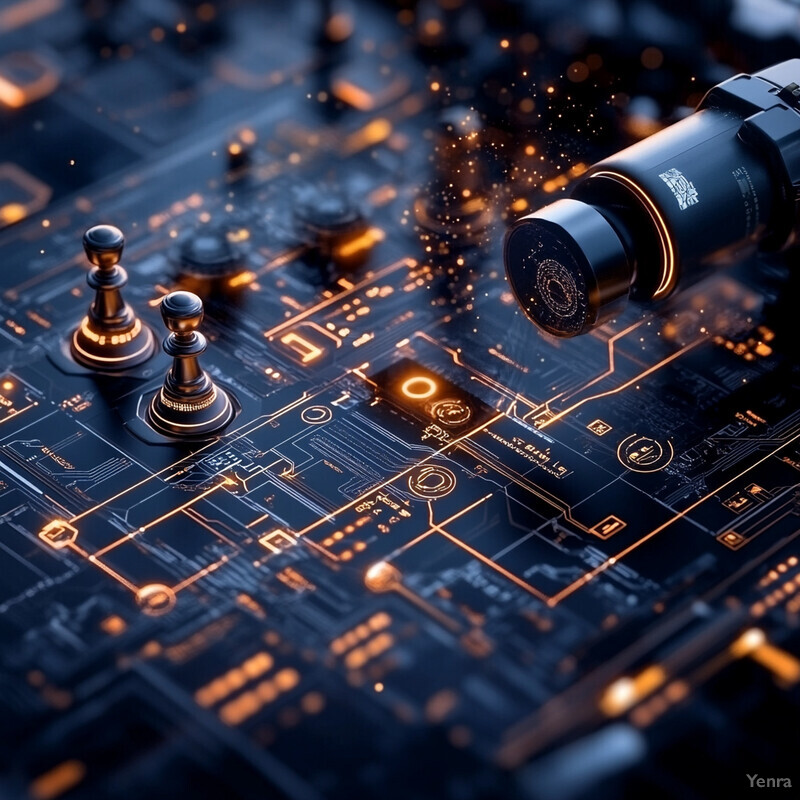
Markets are never static—they shift in response to economic cycles, political events, and investor sentiment. AI techniques, particularly reinforcement learning and adaptive machine learning models, allow for continuous adjustment of asset allocations as new information arrives. These models learn optimal allocation strategies by repeatedly interacting with a simulated or real market environment, adapting their decisions based on observed outcomes. Over time, the algorithm refines its approach to respond promptly to market regime changes, exploiting emerging opportunities while mitigating downside risk. As a result, dynamic asset allocation guided by AI can help portfolios remain more agile, optimizing returns in an ever-changing financial landscape.
4. Regime Detection and Market State Classification
AI can cluster historical market states into distinct regimes—bull, bear, high-volatility, low-liquidity, etc.—and detect transitions early, enabling portfolio managers to preemptively adjust allocations to weather regime shifts more effectively.
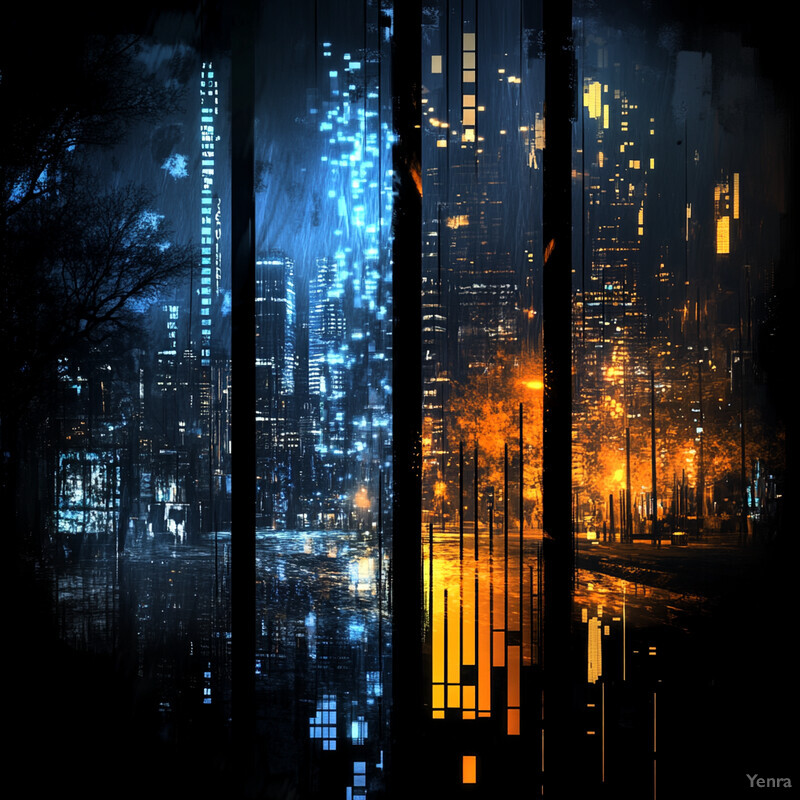
Identifying different market states—such as bull, bear, or sideways markets—is crucial for effective portfolio management. AI-driven clustering and classification algorithms can analyze historical and real-time data to detect underlying regimes that share similar characteristics, such as volatility levels, liquidity conditions, or correlation structures. By recognizing these regimes and predicting transitions, the portfolio can be proactively adjusted to account for higher risk premiums, reduced market liquidity, or changing investment opportunities. This early detection approach helps managers shield their portfolios from adverse conditions and exploit windows of opportunity that may arise during certain regimes, ultimately delivering more stable performance.
5. Multi-Objective Optimization
By leveraging sophisticated optimization frameworks, AI can simultaneously consider multiple objectives—such as maximizing returns, minimizing risk, ensuring liquidity, and incorporating ESG constraints—yielding portfolios tailored to diverse investor goals and constraints.
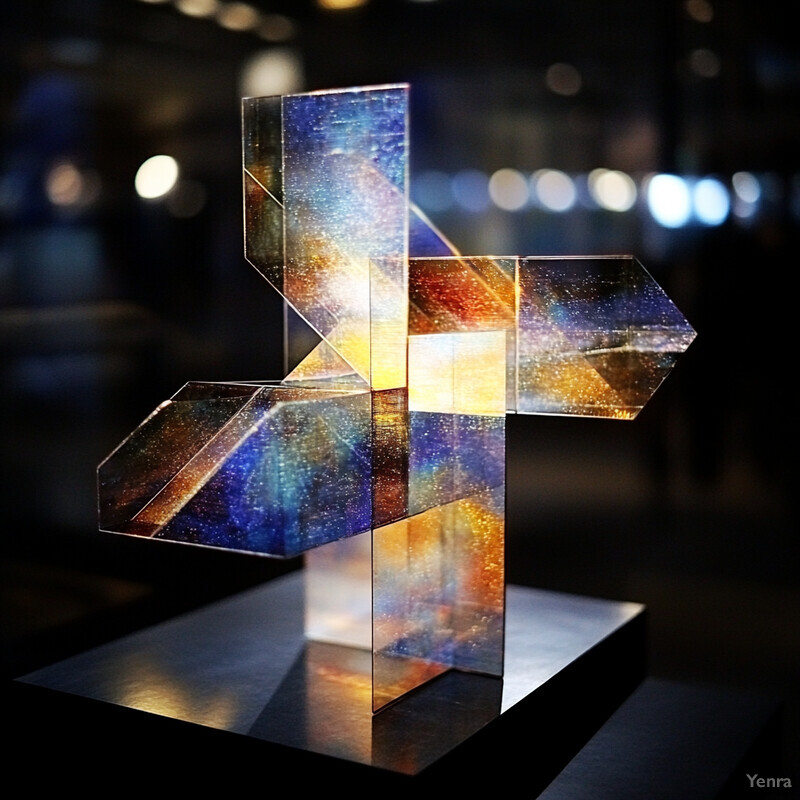
Investors often have multiple, sometimes conflicting objectives: maximizing returns, minimizing risk, maintaining liquidity, and adhering to constraints such as ESG guidelines or regulatory requirements. AI-driven multi-objective optimization frameworks can handle these complex mandates simultaneously. By leveraging evolutionary algorithms, genetic programming, or advanced optimization solvers, AI models explore a vast solution space, seeking an efficient frontier that balances these various considerations. This leads to more customized portfolios that meet investors’ nuanced goals while remaining robust to changing conditions. Over time, multi-objective optimization ensures that portfolios are not just return-oriented, but also aligned with broader strategic and ethical objectives.
6. Alternative Data Integration
AI excels at extracting signals from unstructured or less conventional data sources (e.g., satellite imagery, social media sentiment, corporate earnings calls). Incorporating these signals into the portfolio optimization process can uncover hidden opportunities and enhance decision-making.
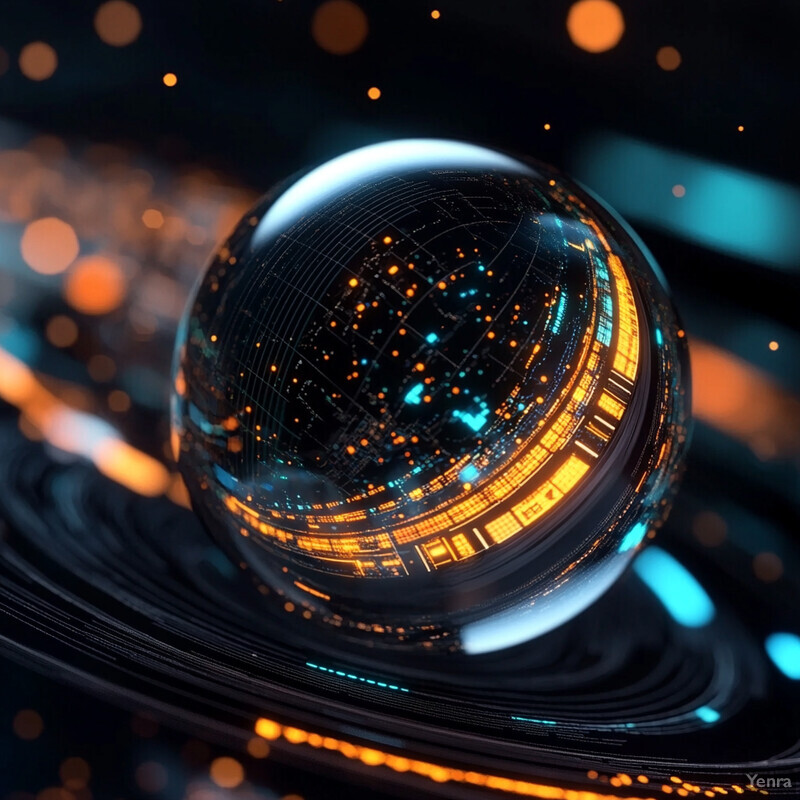
Financial markets today are influenced by more than just price and fundamental data. Unstructured and unconventional data—such as social media sentiment, satellite imagery of parking lots, climate patterns, shipping activity, and online news—can provide unique signals about a company’s health or an economy’s prospects. AI excels at processing these alternative data sources, using natural language processing, computer vision, and advanced statistical methods to derive meaningful features. Incorporating these insights into the portfolio optimization process can help uncover hidden opportunities, strengthen forecasts, and achieve a competitive edge by acting on information that remains overlooked by more traditional approaches.
7. Real-Time Stress Testing
Machine learning models can rapidly simulate how portfolios perform under hypothetical scenarios and stress conditions, identifying vulnerabilities in near real-time and helping investors rebalance or hedge positions.
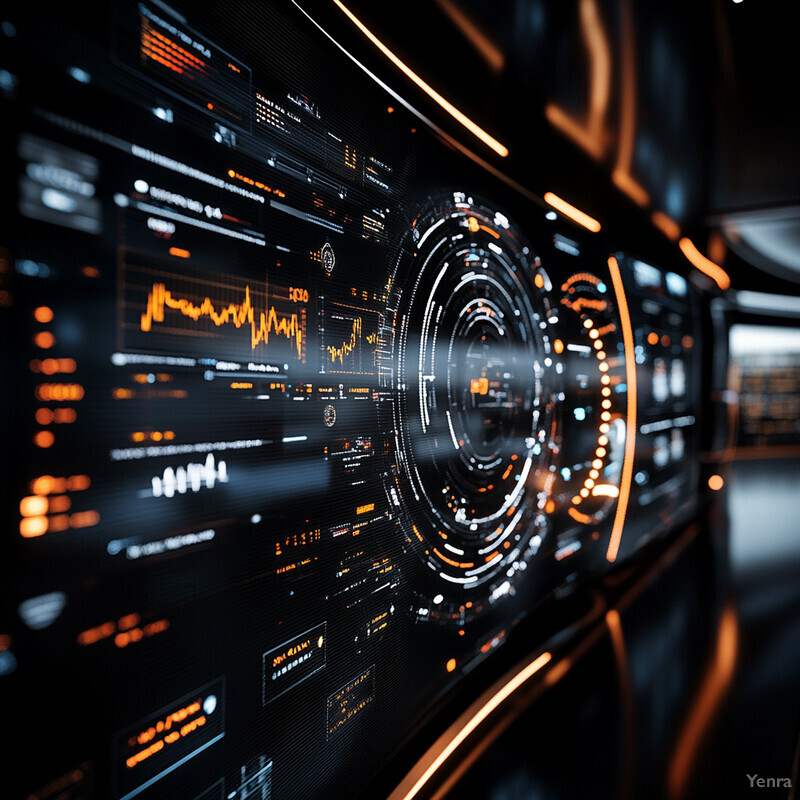
Rather than wait for periodic risk assessments, modern AI systems can simulate stress scenarios in near real-time, evaluating how a portfolio might perform under sudden shocks such as interest rate hikes, geopolitical tensions, or supply chain disruptions. Using a combination of machine learning and simulation techniques, these models incorporate a wide range of hypothetical events to identify potential weaknesses. Being able to promptly stress test the portfolio allows managers to implement timely hedging strategies, rebalance asset weightings, or adjust factor exposures before minor vulnerabilities become major losses. This proactive approach ultimately increases the portfolio’s resilience and long-term stability.
8. Adaptive Risk Budgeting
AI can dynamically allocate risk budgets across various strategies or asset classes, learning from historical performance and current market conditions to maintain optimal exposures and improve risk-adjusted returns.
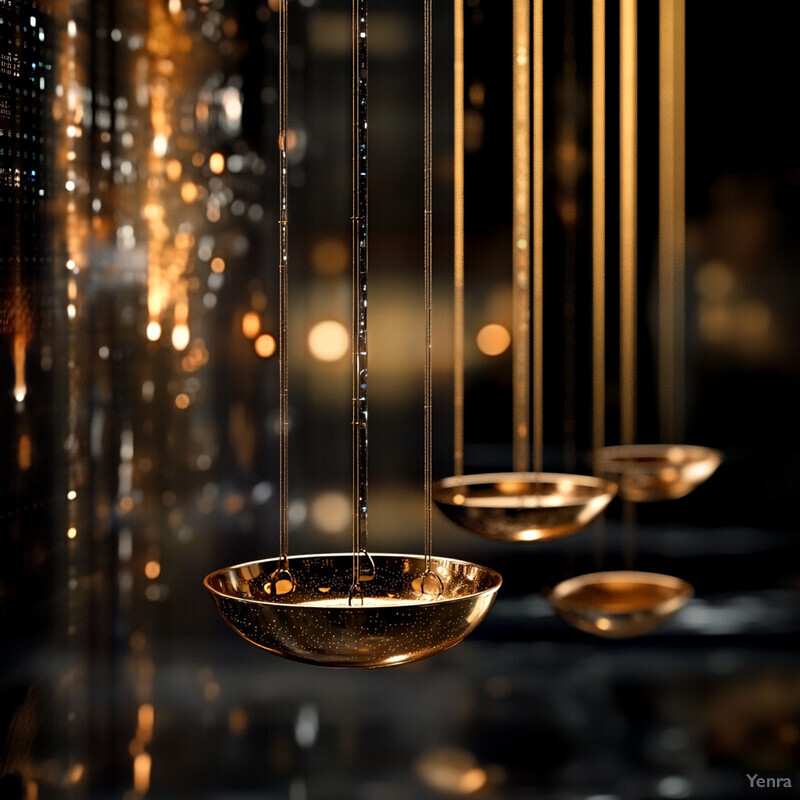
Risk budgeting involves determining how much risk to allocate to different strategies, regions, or asset classes within a portfolio. AI models can dynamically assess the evolving performance and correlation structures, continuously learning how different assets and strategies behave under shifting market conditions. By adapting these risk budgets, AI ensures that capital is deployed to strategies best suited for current conditions, rather than relying on static allocations. Over time, this adaptive risk budgeting helps smooth returns, enhance diversification benefits, and maintain risk exposures that are aligned with an investor’s changing objectives and tolerance levels.
9. Transaction Cost and Liquidity Modeling
Incorporating advanced AI models for estimating execution costs and liquidity conditions leads to improved trade scheduling and order execution strategies, thus minimizing slippage and transaction costs in portfolio adjustments.
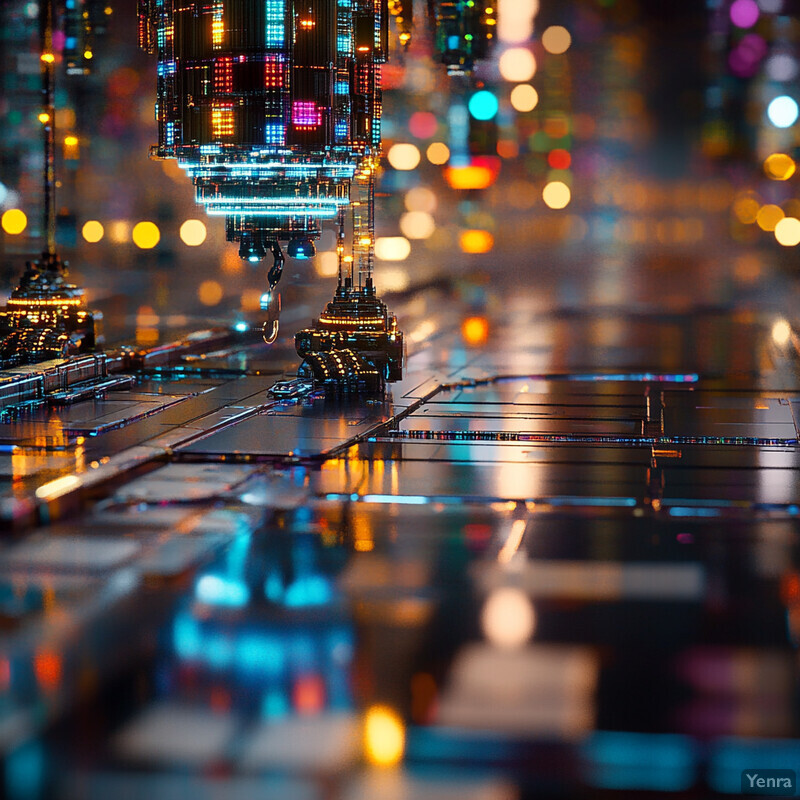
Execution costs, including spreads, commissions, market impact, and slippage, can significantly erode a portfolio’s returns. Liquidity conditions change frequently, affecting the cost of entering or exiting positions. AI-driven transaction cost modeling integrates price dynamics, order book data, market depth metrics, and historical execution records to provide more accurate predictions of real trading costs. By understanding these costs in finer detail, portfolio managers can schedule trades more efficiently and select execution venues that minimize slippage. Ultimately, improved transaction cost and liquidity modeling help ensure that the theoretical benefits of an optimal portfolio are not lost during real-world implementation.
10. Nonlinear Optimization Techniques
AI-driven optimization algorithms (e.g., evolutionary algorithms, genetic algorithms) can handle large-scale nonlinear optimization problems, discovering solutions in complex investment universes and overcoming limitations of classical mean-variance frameworks.
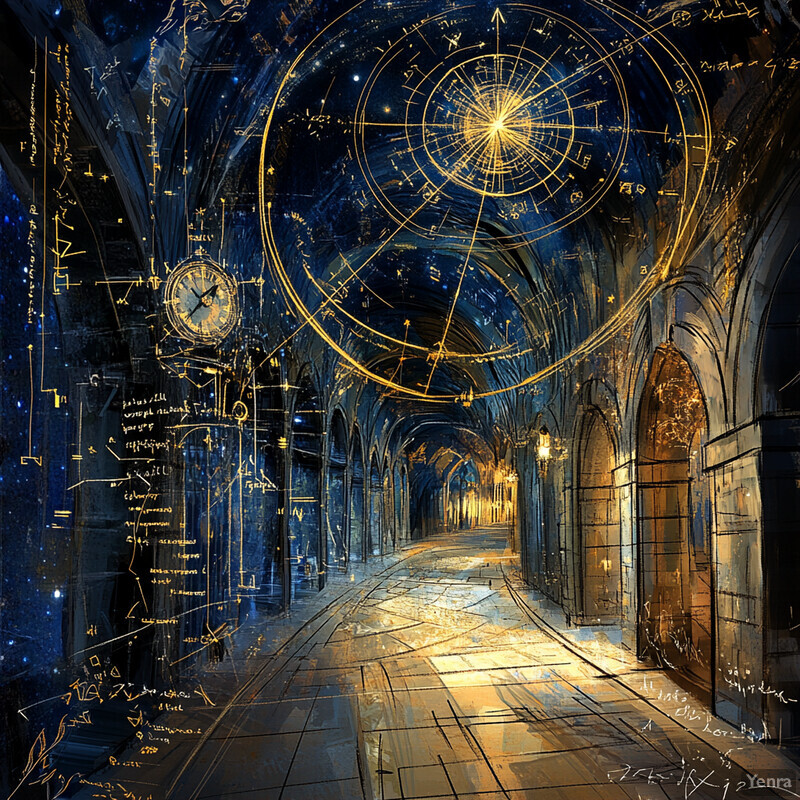
Traditional optimization techniques, like mean-variance, are limited by their assumption of normally distributed returns and linear relationships. AI-driven nonlinear optimization methods, including evolutionary algorithms and other global optimization heuristics, can navigate large, complex solution spaces. They explore portfolio configurations that traditional solvers might overlook, identifying combinations of assets that yield better risk-adjusted returns. These methods can also integrate irregular constraints and non-convex objectives, providing more flexible solutions. By embracing nonlinear optimization, portfolio managers can construct more robust, resilient portfolios that better capture the complexities of real-world markets.
11. Explainable AI Models for Investment Decisions
Recent advances in model interpretability allow investment teams to understand the rationale behind AI-driven portfolio adjustments, building trust and aiding in compliance.
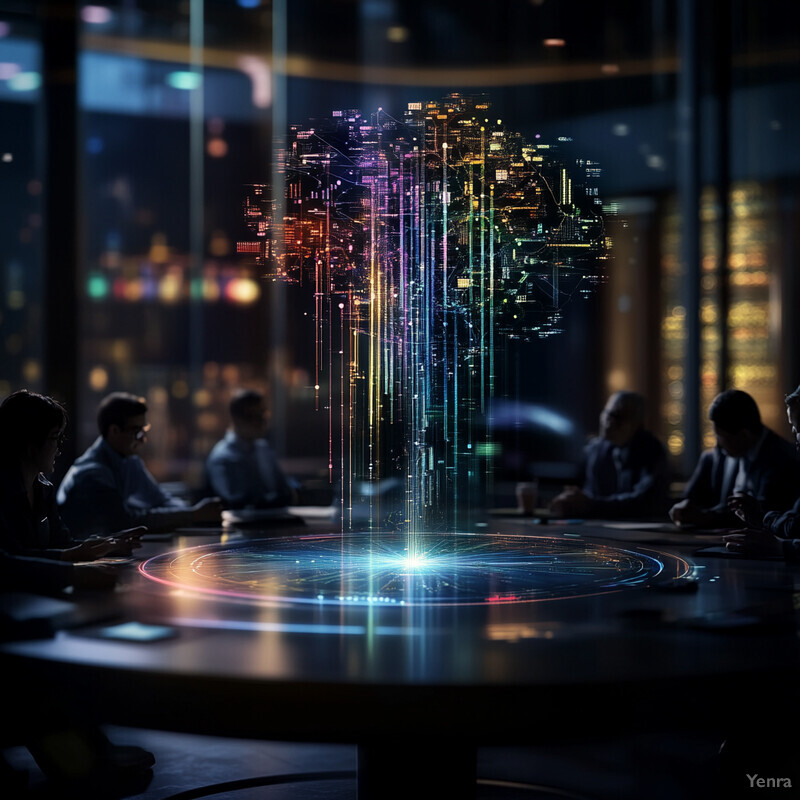
With the growing complexity of AI models, interpretability and explainability have become critical. Explainable AI (XAI) techniques help portfolio managers, compliance officers, and end investors understand the logic behind model-driven decisions. These methods provide insights into which features influenced a certain allocation or why the model is forecasting higher risk in a given sector. Improved transparency fosters trust, allowing stakeholders to appreciate the rationale behind strategic shifts. It also ensures alignment with regulatory requirements and best practices in governance. By making AI models more interpretable, investment teams can combine the strengths of advanced analytics with human judgment.
12. Continuous Learning and Model Updating
AI models can continuously update themselves as new data flows in, improving the timeliness and quality of forecasts and aligning with the most current market dynamics.
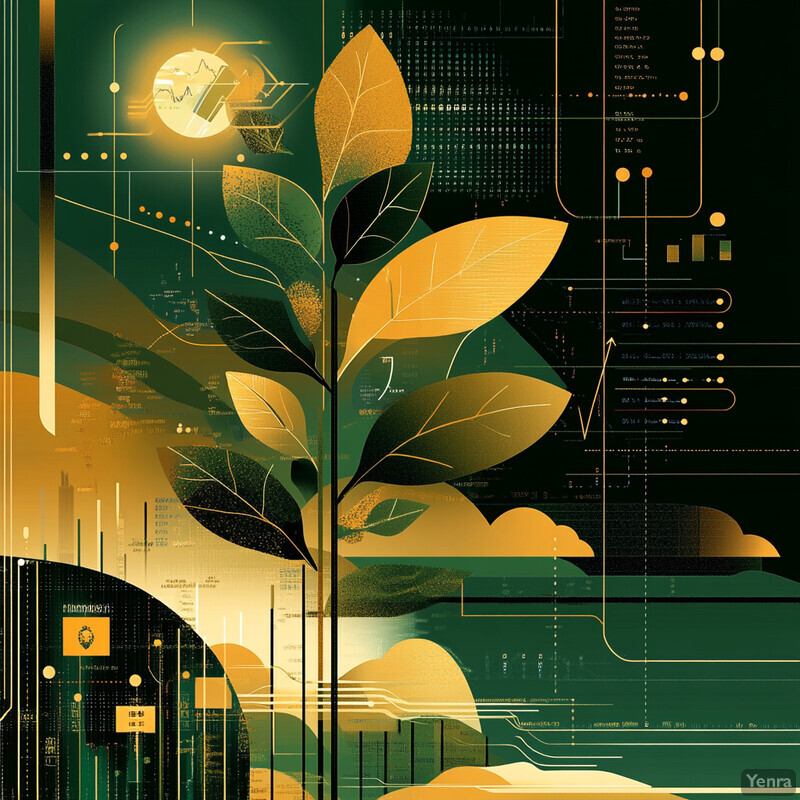
Financial markets are dynamic, and model parameters that worked well in one period may fail in another. AI models equipped with continuous learning capabilities update themselves as new data arrives, always reflecting the latest market conditions. This adaptability enables them to refine their signals, incorporate fresh economic indicators, and correct for recent forecasting errors. The result is a system that does not become outdated or static. Over time, continuous learning ensures that the portfolio optimization framework remains relevant, proactively managing shifts in correlations, risk premia, and asset characteristics to deliver more stable returns.
13. Integration of Macro and Micro Signals
AI models can simultaneously process macroeconomic indicators, corporate fundamentals, technical signals, and alternative datasets, merging top-down and bottom-up approaches to achieve more holistic and accurate portfolio insights.
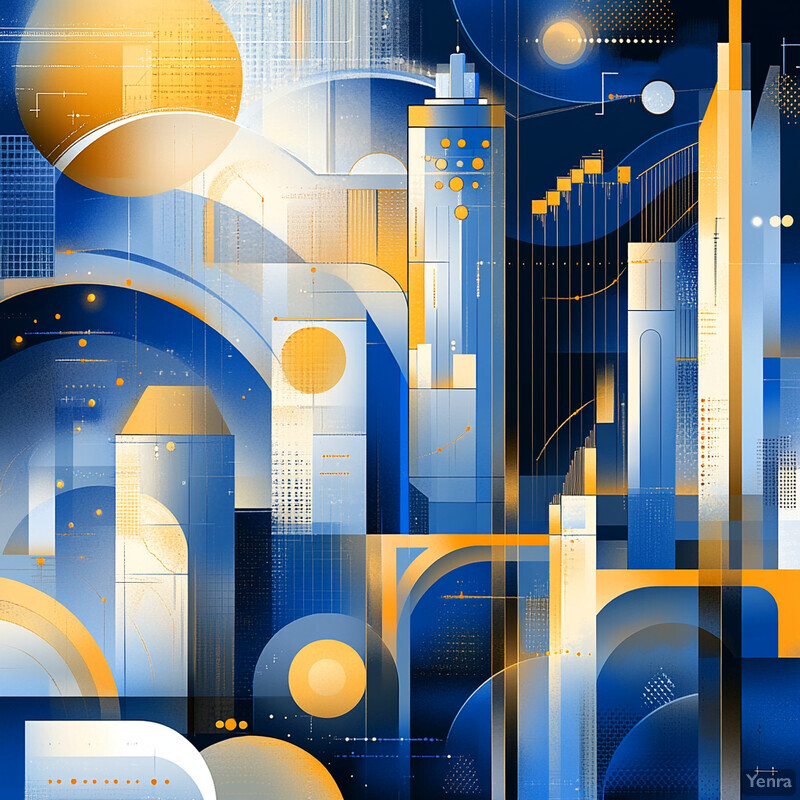
Building an effective portfolio often requires reconciling top-down macroeconomic perspectives—such as interest rates, GDP growth, and inflation trends—with bottom-up micro-level signals like company earnings, management quality, and sector health. AI excels at integrating diverse data streams, enabling a comprehensive view of the market. By modeling how macroeconomic shifts influence the behavior of specific companies or sectors, AI can uncover nuanced relationships and more precisely estimate return drivers. This holistic approach prevents information silos and leads to investment decisions that incorporate both the “big picture” and the granular details, ultimately improving the quality of portfolio construction.
14. Customized Investor Profiles
By applying clustering and personalization algorithms, AI can segment investors into groups with distinct risk tolerances, investment horizons, and objectives, enabling tailored portfolios that better suit individual preferences.
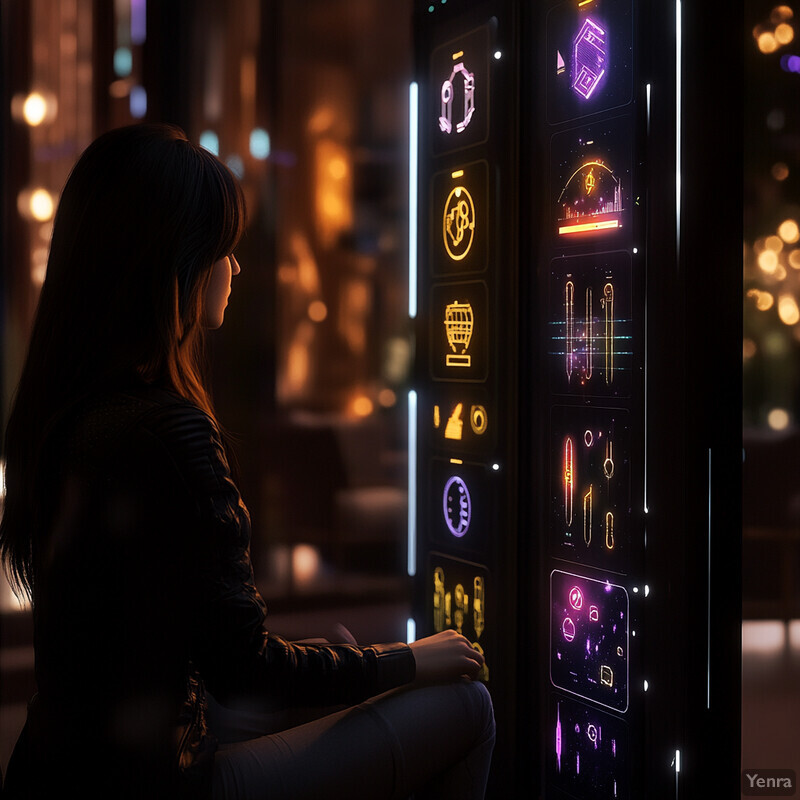
Not all investors share the same goals, time horizons, or risk preferences. AI-driven segmentation methods, such as clustering and personalization algorithms, can group investors based on their unique characteristics—be it retirement goals, ESG preferences, liquidity needs, or appetite for volatility. This personalization allows portfolio managers to tailor investment strategies to each client’s specific circumstances. For example, a risk-averse investor nearing retirement might receive a more stable, income-focused portfolio, while a young investor with a longer time horizon might be offered a growth-oriented strategy. By delivering a more individualized experience, AI enhances investor satisfaction and long-term engagement.
15. Detection of Style Drift
AI can identify unintended shifts in a portfolio’s style or factor exposures over time, allowing managers to rebalance to maintain style integrity and alignment with strategic mandates.
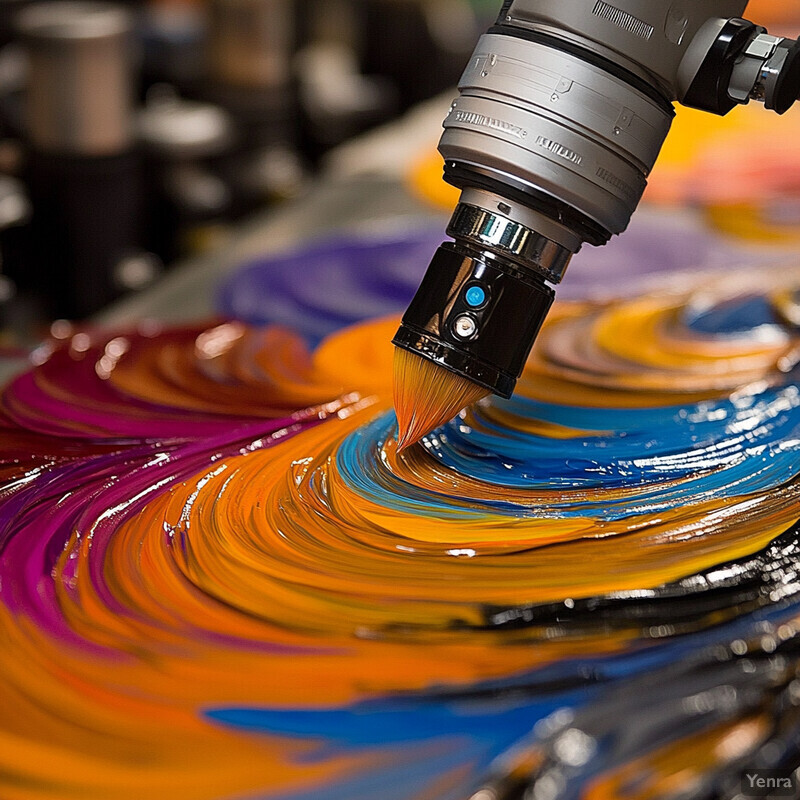
Over time, a portfolio might unintentionally deviate from its intended investment style or factor exposure. For instance, a fund marketed as “value-oriented” could shift toward “growth” characteristics if left unchecked. AI models monitor portfolio composition, factor loadings, and risk exposures continuously, using machine learning to detect subtle style drifts. Once identified, managers can take corrective action, rebalancing the portfolio to restore its intended style. Maintaining style integrity aligns the investment with its stated objectives, ensures transparent communication with clients, and prevents style drift from eroding the trust that investors place in a given strategy.
16. Event-Driven Portfolio Adjustments
Leveraging natural language processing and news sentiment analysis, AI can rapidly respond to market-moving events, adjusting allocations more quickly and strategically than traditional approaches.
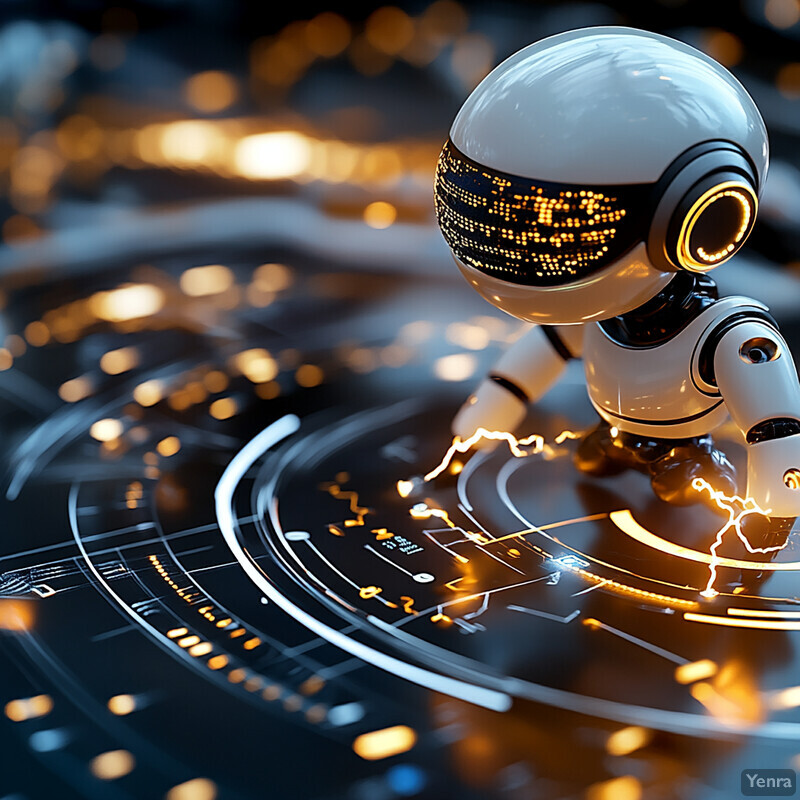
Sudden events—corporate earnings surprises, mergers and acquisitions, regulatory changes, natural disasters, or geopolitical tensions—can rapidly shift the investment landscape. AI-powered natural language processing and sentiment analysis techniques instantly parse news, transcripts, and social media feeds, converting text into actionable signals. With this capability, the portfolio can be quickly recalibrated to reflect new information. For example, positive sentiment around a key earnings report might justify increasing exposure to a certain sector, while negative geopolitical news might prompt a reduction in emerging market holdings. By integrating event-driven insights, AI helps portfolios remain responsive and opportunistic in volatile environments.
17. Optimization Under Complex Constraints
Many portfolios are subject to intricate regulatory, ESG, or liquidity constraints, and AI-based solvers can handle these more efficiently, finding solutions that might be missed by conventional methods.
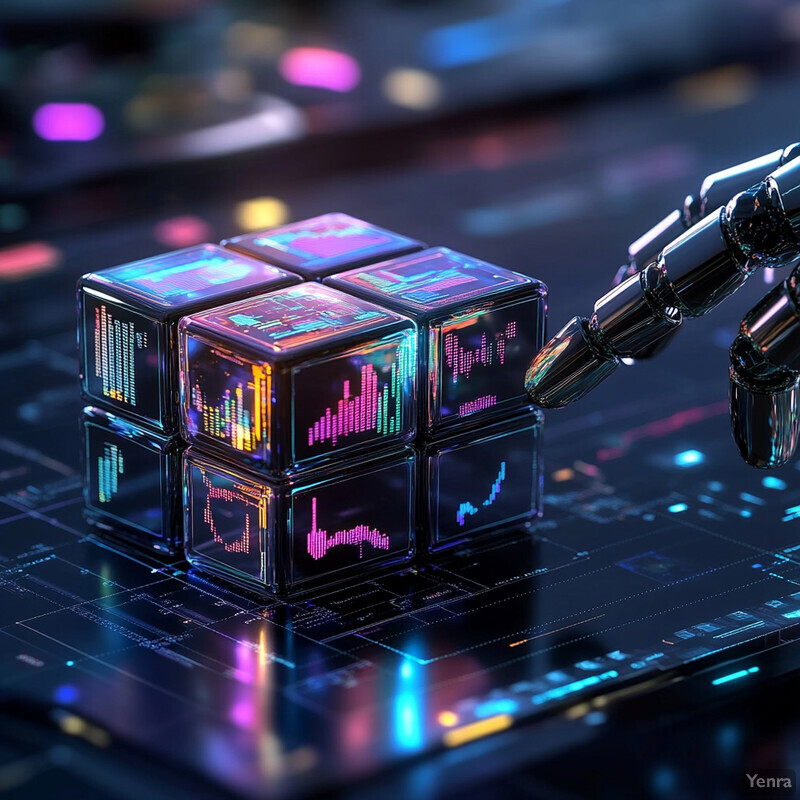
Portfolio construction often involves intricate constraints—liquidity thresholds, regulatory requirements, ESG mandates, concentration limits, and tax considerations. AI-based optimization tools can handle these complexities more efficiently than classical methods. They use advanced solvers and heuristic search techniques to find portfolios that respect all given constraints while still aiming to maximize returns and minimize risk. This flexibility allows for more sophisticated customization, ensuring that the final solution is both practical and aligned with the investor’s broader considerations. As a result, portfolio managers can confidently navigate a wide range of real-world conditions that extend well beyond simplistic optimization frameworks.
18. Improved Factor Modeling
By identifying latent factors and nonlinear relationships among assets, AI can improve traditional factor models, helping portfolio managers understand the underlying drivers of returns and optimize exposures accordingly.
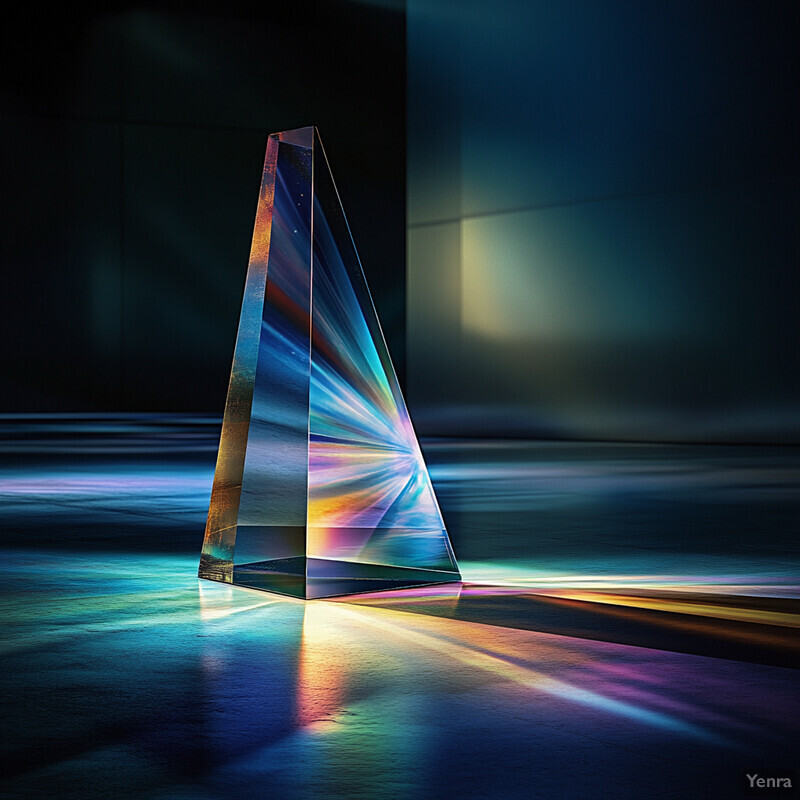
Factor investing relies on identifying key drivers of returns—such as value, momentum, quality, or low volatility. AI can enhance factor modeling by uncovering latent factors and nonlinear relationships that classical methods miss. Through complex pattern recognition, machine learning models isolate subtle signals that help explain asset price movements. Moreover, these models dynamically adjust factor loadings as market conditions evolve, ensuring that the factor exposures remain relevant. By improving factor models, AI refines the framework through which portfolio managers understand return drivers, resulting in more targeted allocation decisions and enhanced risk-adjusted performance.
19. Risk-Parity and Robust Portfolio Construction
Machine learning methods can enhance risk-parity strategies and robust optimization approaches, ensuring portfolios remain balanced across different risk sources, even as correlations and volatilities fluctuate.
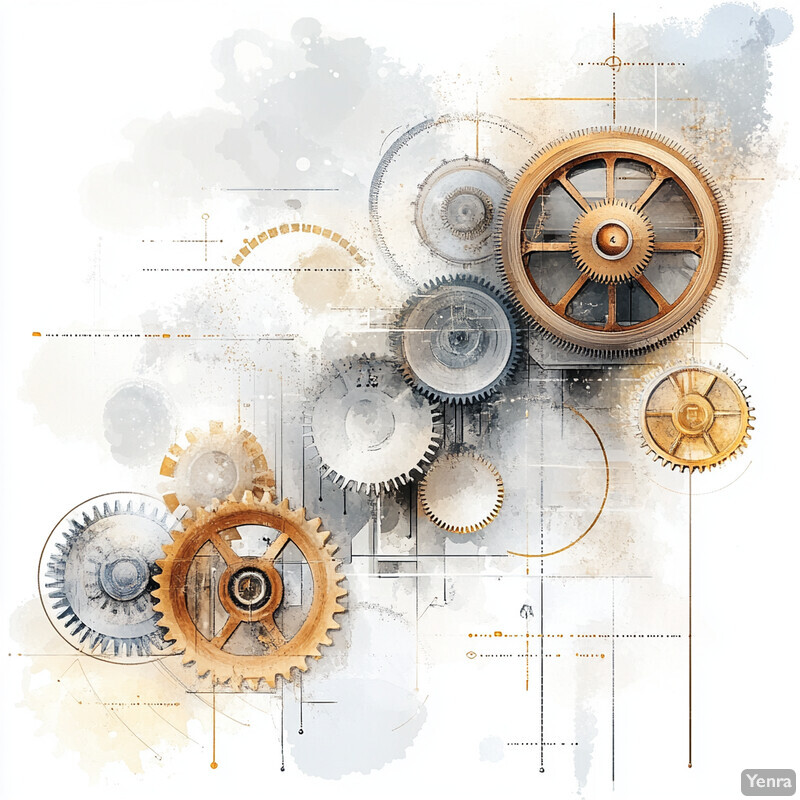
Risk-parity strategies seek to balance risk contributions from different assets, ensuring that no single asset dominates the portfolio’s risk profile. AI enhances these approaches by continuously monitoring correlations and volatility patterns, adjusting allocations as relationships shift. Moreover, robust optimization techniques, powered by AI, prepare the portfolio for adverse conditions by minimizing sensitivity to model errors and parameter uncertainty. This helps maintain a stable risk profile and safeguard against unexpected shocks. With AI’s assistance, risk-parity and robust portfolio methodologies become more adaptive, providing a stronger line of defense in turbulent markets.
20. Scenario Generation and Simulation
AI can rapidly generate realistic market scenarios that reflect non-stationarity and complex dynamics, providing a richer set of possible futures against which portfolios can be tested and optimized.
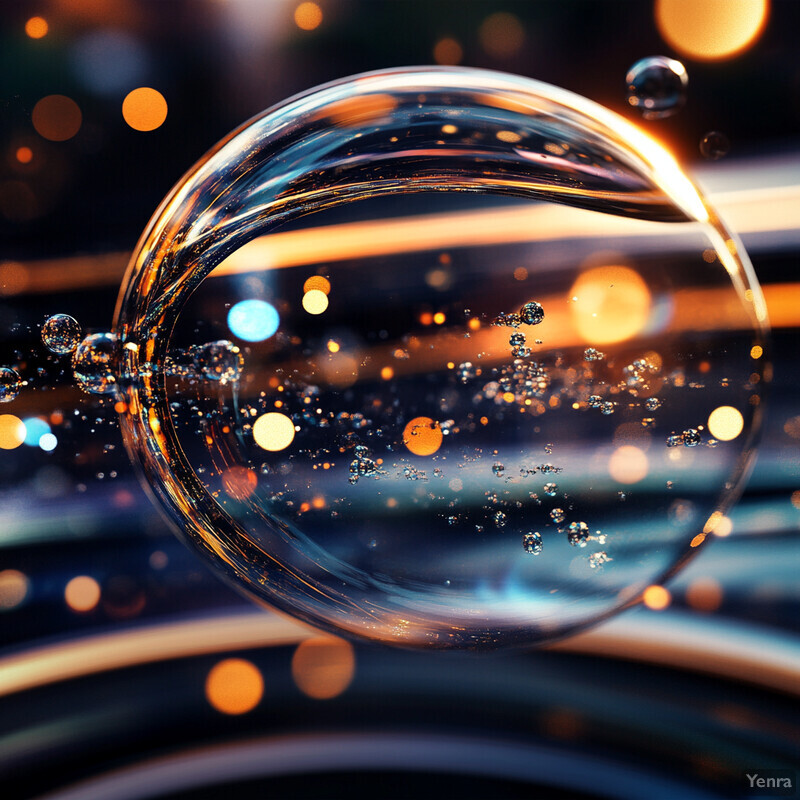
Building resilience into a portfolio requires anticipating a range of potential future states. AI excels at generating realistic, forward-looking scenarios that reflect complex, nonlinear market dynamics. By combining historical data with sophisticated simulation techniques, AI can produce a diverse set of plausible outcomes—spanning various macroeconomic environments, regulatory changes, or disruptive innovations. Portfolio managers can then optimize their allocations against this richer landscape of possibilities, improving the portfolio’s preparedness for uncertainty. Over time, these AI-generated scenarios lead to portfolios that are not only well-positioned for expected conditions but also robust against unexpected events, creating a stronger foundation for long-term success.