1. Automated Transaction Monitoring
AI-driven systems can continuously monitor and analyze large volumes of transactions, identifying anomalies or suspicious patterns more efficiently than manual reviews.
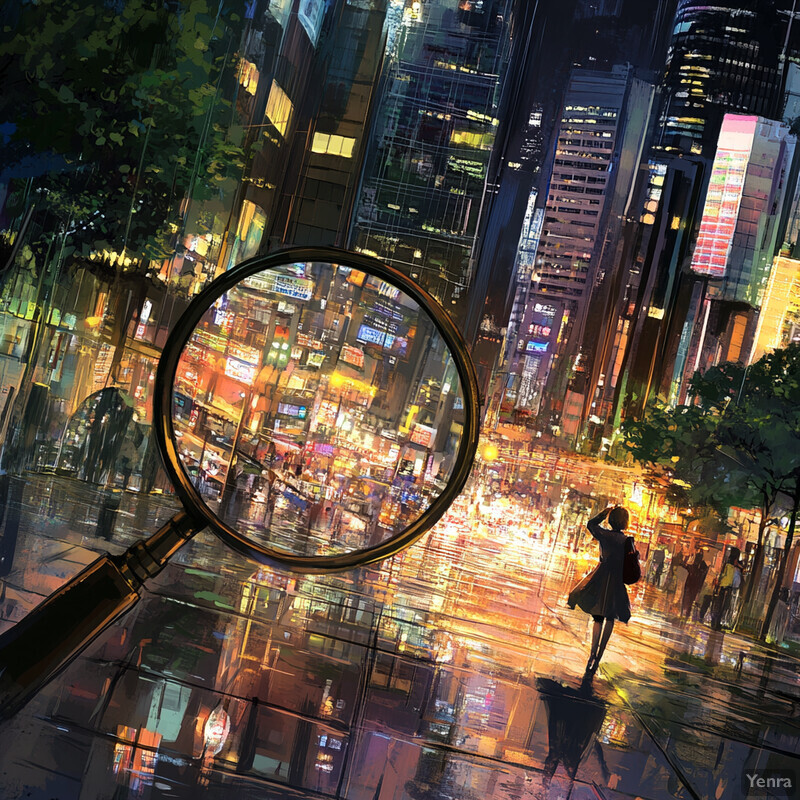
AI-driven automated transaction monitoring systems leverage machine learning algorithms and statistical models to continuously scrutinize large volumes of financial transactions, looking for irregular patterns, sudden spikes in activity, or atypical money flows. Rather than relying on manual oversight, these systems can process and analyze data in real time, identifying potential violations of regulatory rules much faster. Over time, they adapt to evolving transaction behaviors and learn to differentiate between legitimate deviations and truly suspicious activity. This reduces the burden on compliance teams, allowing them to focus on the highest-risk alerts. In turn, financial institutions can quickly address potential compliance issues before they escalate into legal or reputational problems.
2. Enhanced AML (Anti-Money Laundering) Detection
Machine learning models help flag unusual transaction patterns indicative of money laundering, improving both the speed and accuracy of AML checks.
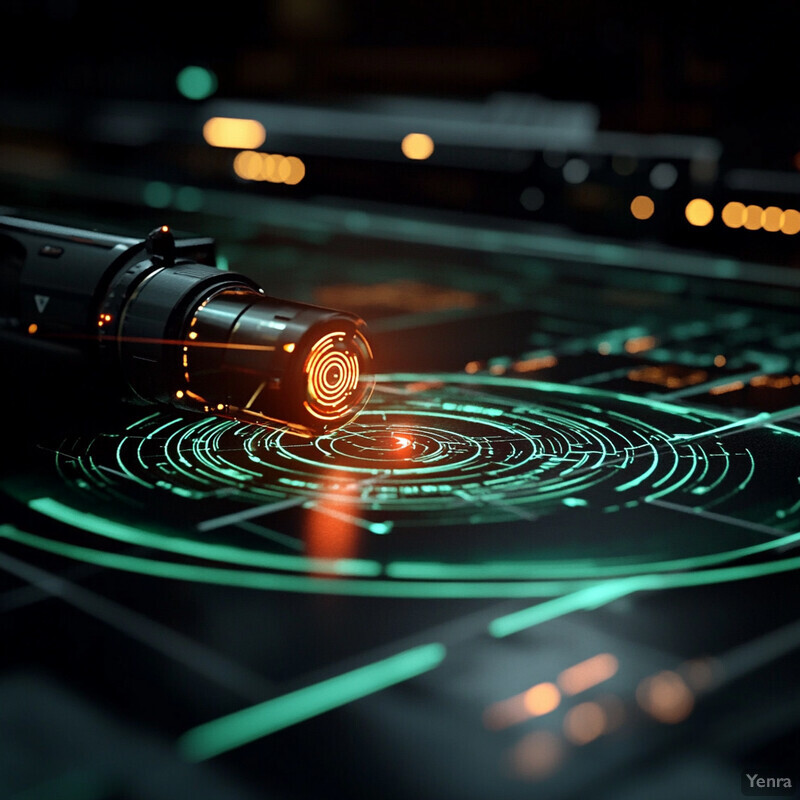
AI-powered AML systems enhance the detection of money laundering activities by examining complex transaction networks, cross-border transfers, and layered corporate ownership structures. Using advanced algorithms, these tools look for patterns consistent with known laundering techniques—such as structuring, layering, or integration—that may be difficult for human auditors to detect. They also incorporate feedback loops, refining their detection criteria based on both confirmed suspicious cases and false alarms. By scaling effortlessly with growing data volumes and rapidly changing illicit strategies, AI-driven AML solutions significantly reduce false positives, enabling more efficient and effective use of compliance resources. This leads to a more robust defense against illicit financial flows and increases confidence among regulators and customers.
3. Real-Time Fraud Detection
AI algorithms detect fraud in real time by identifying atypical user behavior, suspicious logins, or sudden changes in transaction velocity, helping financial institutions intervene quickly.
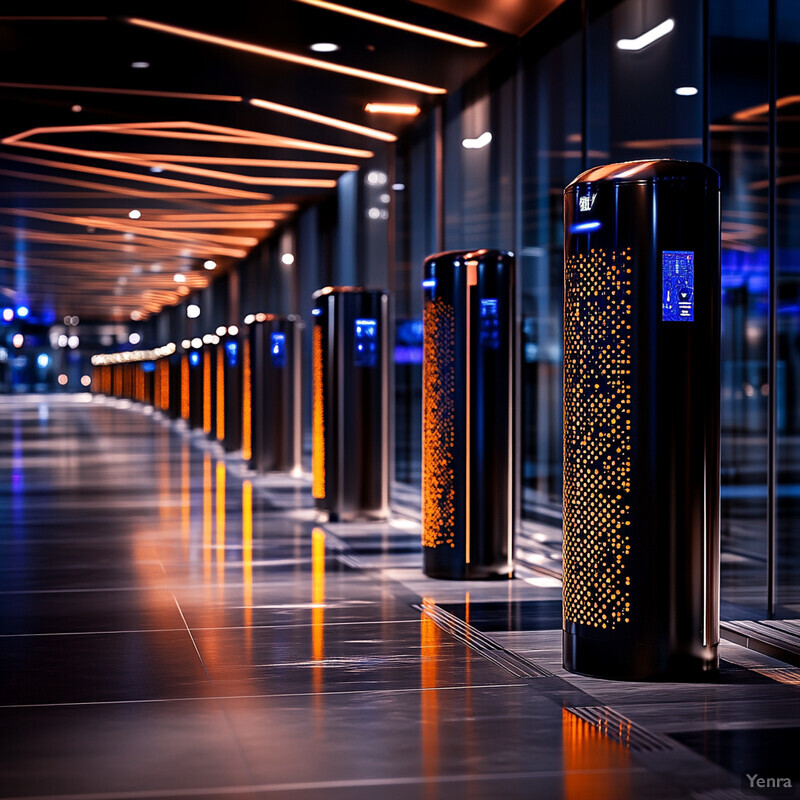
AI-driven fraud detection applies predictive analytics, anomaly detection, and behavioral modeling to flag potential fraudulent activities as they occur. This approach uses contextual information—customer purchase history, geolocation data, device identifiers, and spending habits—to determine if a transaction is legitimate. As a result, suspicious activities like account takeovers, identity theft, or unauthorized transactions can be identified and halted before any significant financial loss occurs. Moreover, self-learning models continually adapt to new types of fraud, improving detection over time. By providing timely alerts and minimizing both false positives and negative customer experiences, AI-driven fraud detection helps maintain trust in financial services.
4. Automated Sanctions and Watchlist Screening
Natural Language Processing (NLP) and machine learning models can rapidly screen entities against sanctions, politically exposed persons (PEP) lists, and blacklists, reducing false positives.
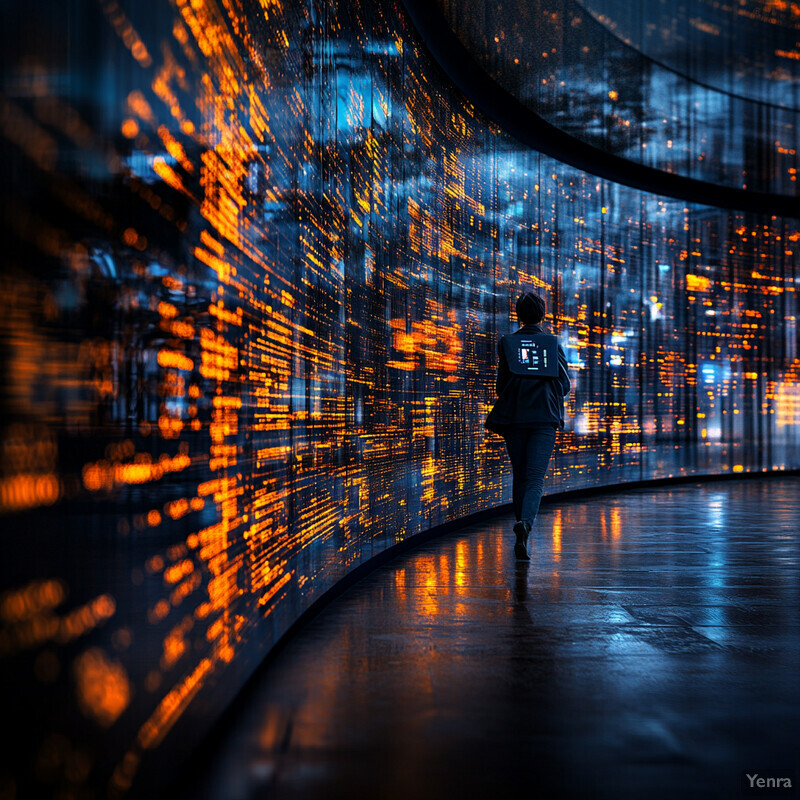
AI streamlines the labor-intensive process of screening customers and counterparties against evolving sanctions lists, PEP registers, and other watchlists. Leveraging natural language processing and entity resolution techniques, AI tools can interpret variations in naming conventions, detect minor typographical differences, and handle multi-language datasets. This reduces the reliance on manual review, lowering error rates and ensuring that financial institutions remain compliant with global regulatory requirements. Automated screening also helps to reduce the number of false matches, improving operational efficiency. Ultimately, this supports a more secure financial ecosystem by ensuring that prohibited individuals or entities are swiftly identified and blocked.
5. Intelligent Identity Verification (KYC)
AI-driven tools use facial recognition, biometric verification, and document analysis to streamline KYC processes while improving accuracy and compliance.
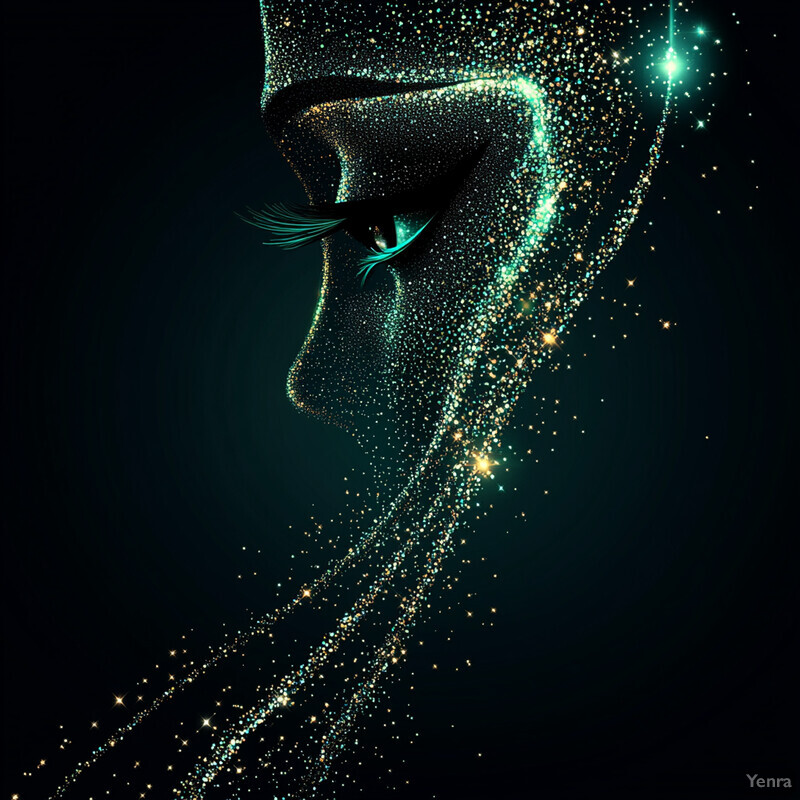
The application of AI in KYC processes ensures faster, more reliable identification and verification of new clients. Biometric verification—such as facial recognition or fingerprint analysis—coupled with document scanning and optical character recognition, checks that identification documents are authentic and untampered. Additionally, AI models cross-reference data against reliable sources, validate information accuracy, and highlight any inconsistencies. By automating these steps, financial institutions not only improve compliance accuracy but also streamline onboarding, enhancing customer experience. Over time, the system learns to detect increasingly sophisticated forgeries, making identity verification more robust.
6. Predictive Risk Scoring
Advanced analytics models predict regulatory and compliance risks before they materialize, allowing firms to proactively adjust controls or reporting strategies.
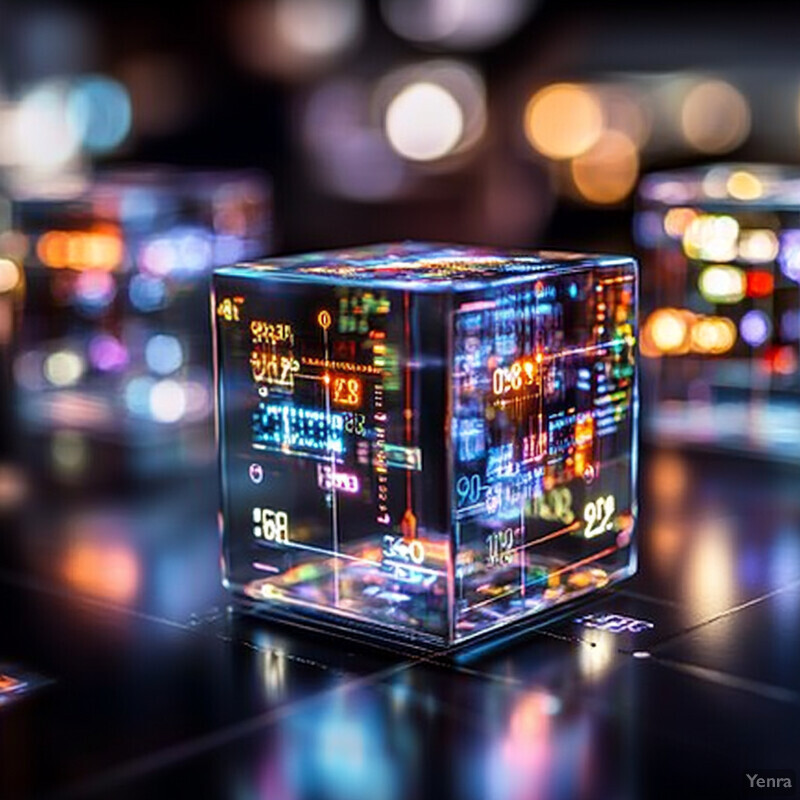
AI-driven predictive risk scoring assigns risk levels to clients, transactions, or business lines based on historical data, current market conditions, and behavioral indicators. Unlike static, rules-based approaches, machine learning models can uncover subtle correlations and anticipate emerging risk factors. This enables compliance teams and risk managers to focus their attention on higher-risk areas before issues materialize. Predictive scoring also supports scenario planning, helping institutions adjust their policies and controls to align better with regulatory expectations. As a result, companies gain a proactive, rather than reactive, stance in maintaining compliance and reducing potential regulatory fines.
7. Adaptive Regulatory Reporting
AI helps financial institutions adapt swiftly to ever-changing regulations by parsing new rules, interpreting their implications, and automatically updating compliance checklists.
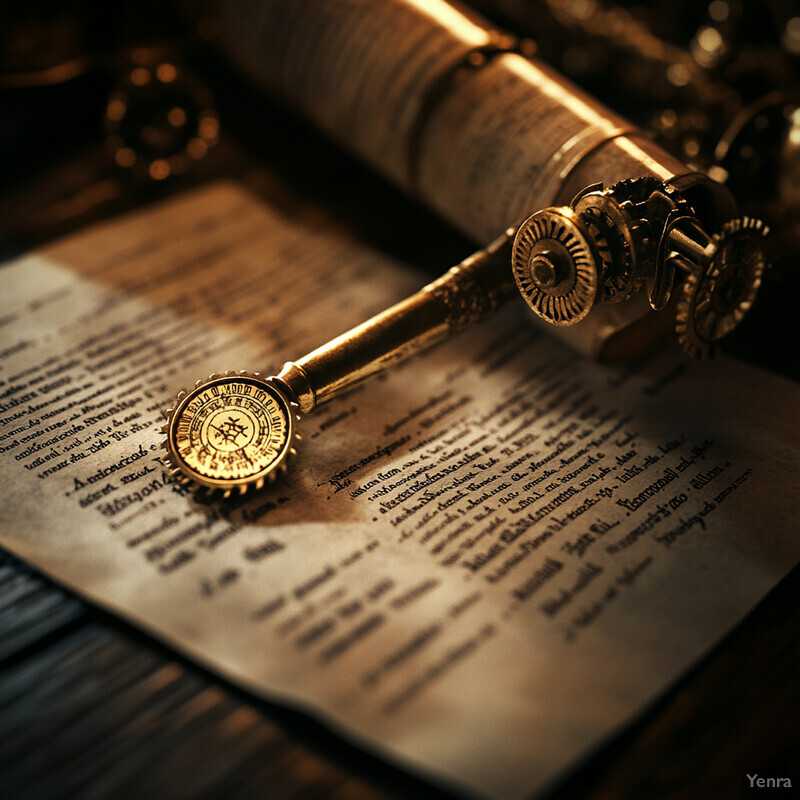
With frequent regulatory updates and region-specific rules, staying current with reporting obligations can be challenging. AI-driven solutions parse through new and updated regulatory texts, identify relevant clauses, and automatically update reporting frameworks or templates. As these systems integrate seamlessly with internal data repositories, they can extract the required information for regulatory disclosures with minimal human intervention. This flexibility allows institutions to quickly adapt to new rules, reduce the cost of compliance management, and avoid penalties for late or incomplete submissions. Ultimately, adaptive regulatory reporting leverages AI to ensure that organizations remain aligned with evolving compliance landscapes.
8. Automated Regulatory Text Analysis
NLP tools interpret complex regulatory documents, extracting critical compliance requirements and summarizing obligations for quick reference.
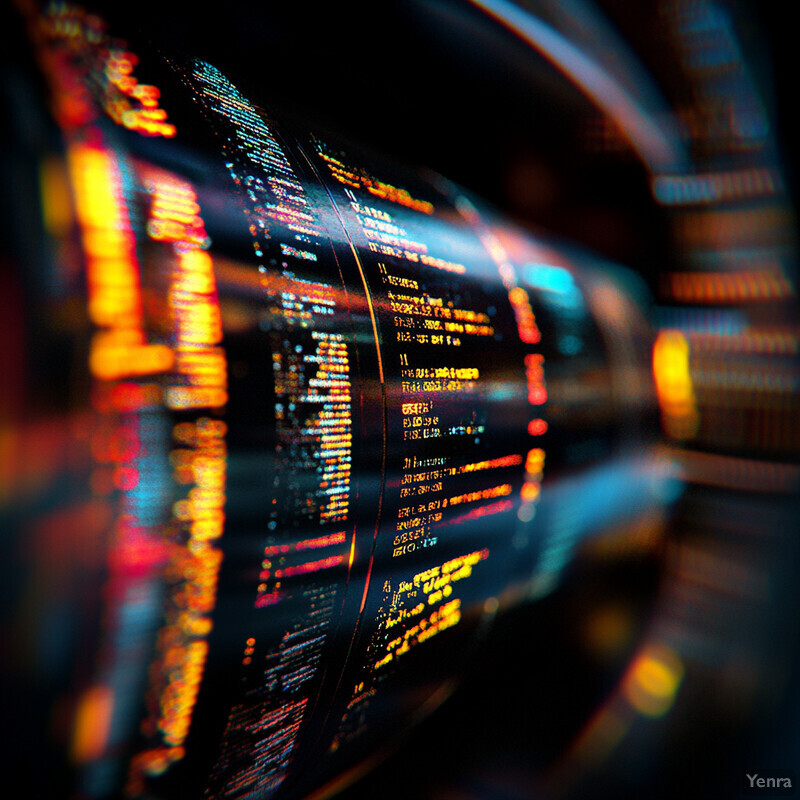
AI-driven Natural Language Processing (NLP) tools can rapidly ingest and interpret complex regulatory documents—often hundreds of pages long—identifying key requirements, obligations, and timelines. Rather than manually combing through dense legalese, compliance teams can rely on summarized extracts, highlights of critical changes, and direct links between regulations and internal policies. Over time, these NLP models improve their understanding of domain-specific language and become more adept at extracting nuanced meaning. By automating this analysis, financial institutions stay informed about evolving standards and can act promptly to implement necessary controls. The result is a more informed and agile compliance function.
9. Workflow Automation and RPA
Robotic process automation (RPA) with AI enhances routine compliance tasks—such as form completion, data entry, and record-keeping—reducing error rates and labor costs.
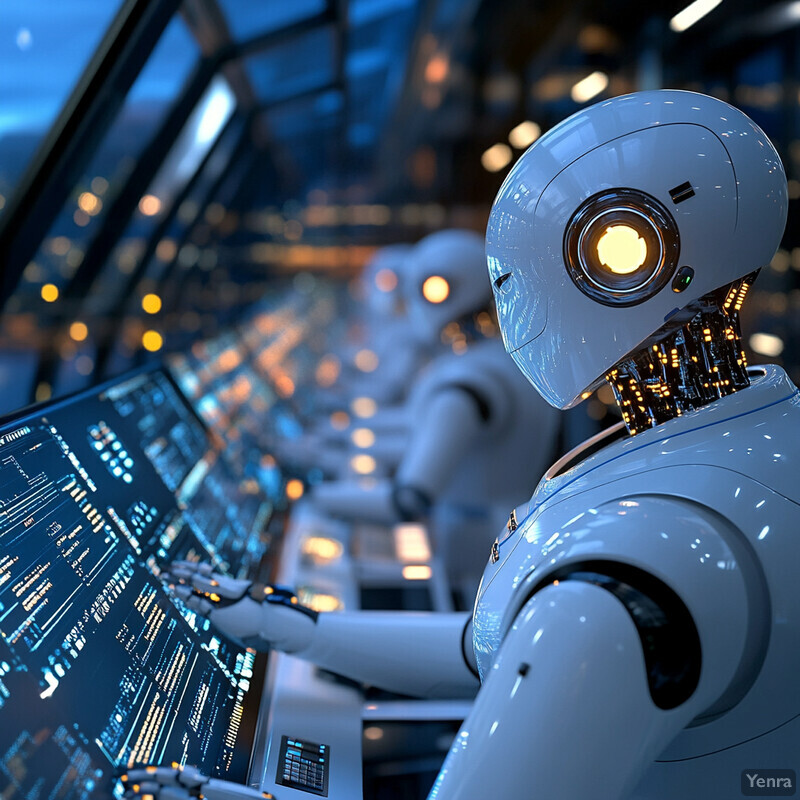
By integrating AI with RPA, financial institutions can automate repetitive and time-consuming compliance tasks such as data entry, form completion, record updates, and basic due diligence checks. Machine learning enhances RPA by allowing bots to make conditional decisions and learn from outcomes, improving over time. This reduces human error, speeds up routine processes, and frees compliance officers to focus on complex, strategic issues. Improved efficiency leads to more reliable regulatory reporting and streamlined compliance operations. Ultimately, this combination of AI and RPA elevates overall productivity and reduces compliance-related costs.
10. Data Quality and Cleansing
AI-driven data governance tools identify inconsistencies, missing fields, or incorrect values in large data sets, ensuring higher-quality input for compliance-related analytics.
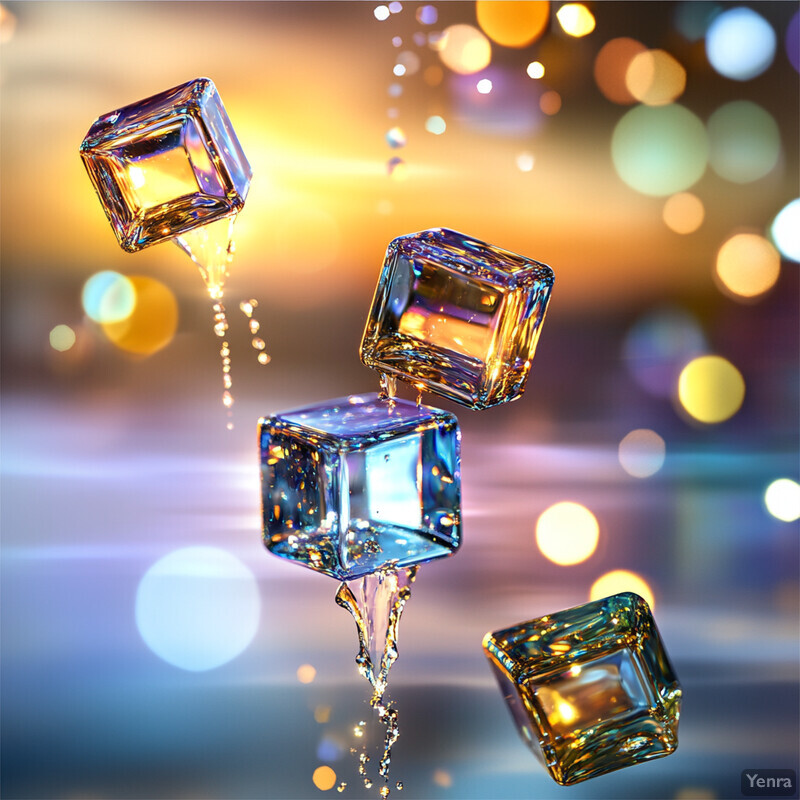
Ensuring accurate and reliable data is crucial for effective compliance. AI-powered data quality tools use machine learning models to identify duplicate records, detect missing or inconsistent information, and flag anomalies in large datasets. By applying pattern recognition, these tools can cleanse data repositories and maintain a single source of truth. The result is more reliable input for compliance analytics, lower incidence of false positives in alerts, and reduced investigative workloads. Additionally, consistent and accurate data sets empower more effective decision-making and enhance overall compliance governance.
11. Intelligent Case Management
AI prioritizes and classifies compliance alerts, enabling investigators and compliance officers to focus on the most pressing issues first and streamline their workflows.
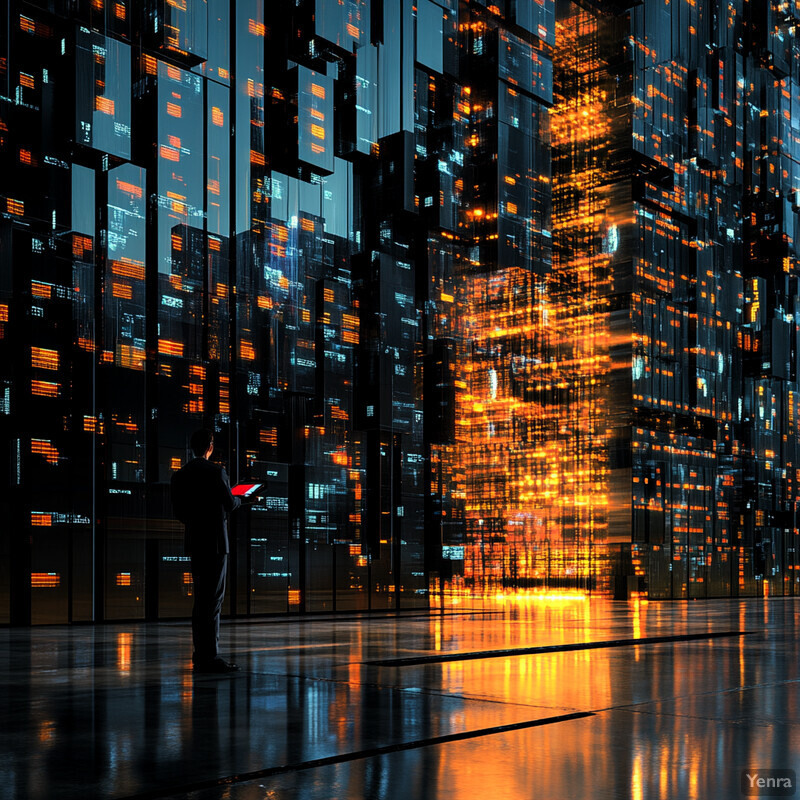
AI-driven case management systems classify, prioritize, and route alerts or incidents to the appropriate compliance specialists. By learning from historical resolutions and investigator feedback, these systems can predict which cases are most urgent and require human review, helping to allocate resources efficiently. Automated summarization of case details and suggested next steps reduces the preliminary investigative burden. Over time, the process becomes more streamlined, ensuring that compliance officers focus their attention on critical issues rather than sifting through low-risk alerts. As a result, case backlogs diminish, and overall compliance performance improves.
12. Continuous Monitoring of Communication Channels
NLP-based tools can monitor emails, chats, and voice calls for non-compliant language, insider threats, or unethical behavior to ensure regulatory adherence.
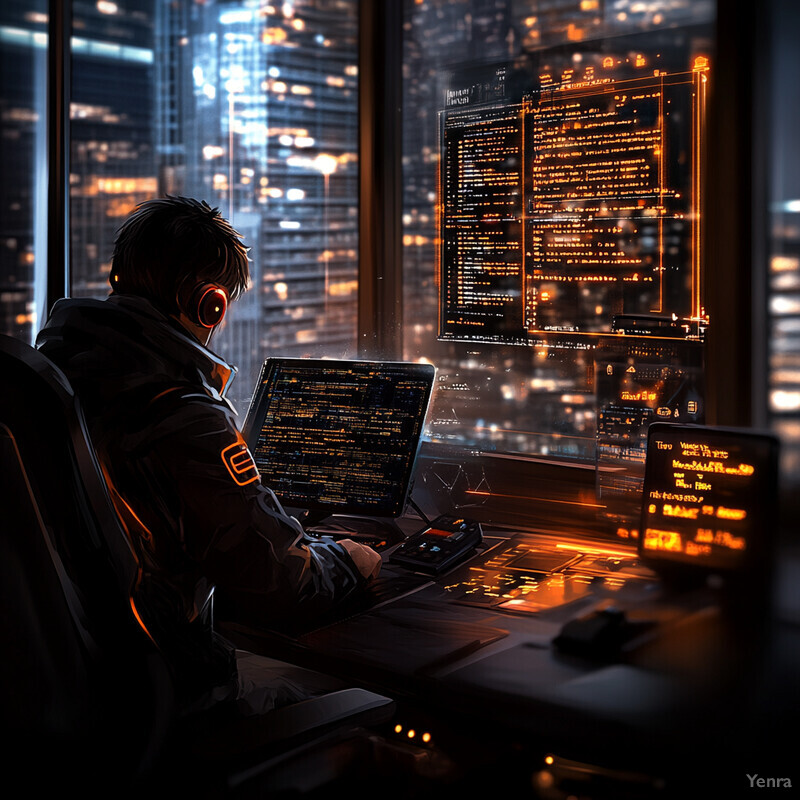
Monitoring internal and external communications—including emails, chat messages, and voice calls—is a key component of preventing misconduct and ensuring compliance. AI-driven NLP solutions can detect phrases, patterns, or sentiments that suggest insider trading, collusion, or other illicit activities. By analyzing communication content in real time, these tools provide early warnings to compliance teams. The detection capability extends across languages, channels, and communication styles, increasing the likelihood of intercepting potential violations early. This proactive approach reduces operational, reputational, and legal risks for financial institutions.
13. Enhanced Audit Trails and Traceability
AI systems create detailed, immutable logs of compliance activities and decision-making processes, facilitating smoother regulatory audits and examinations.
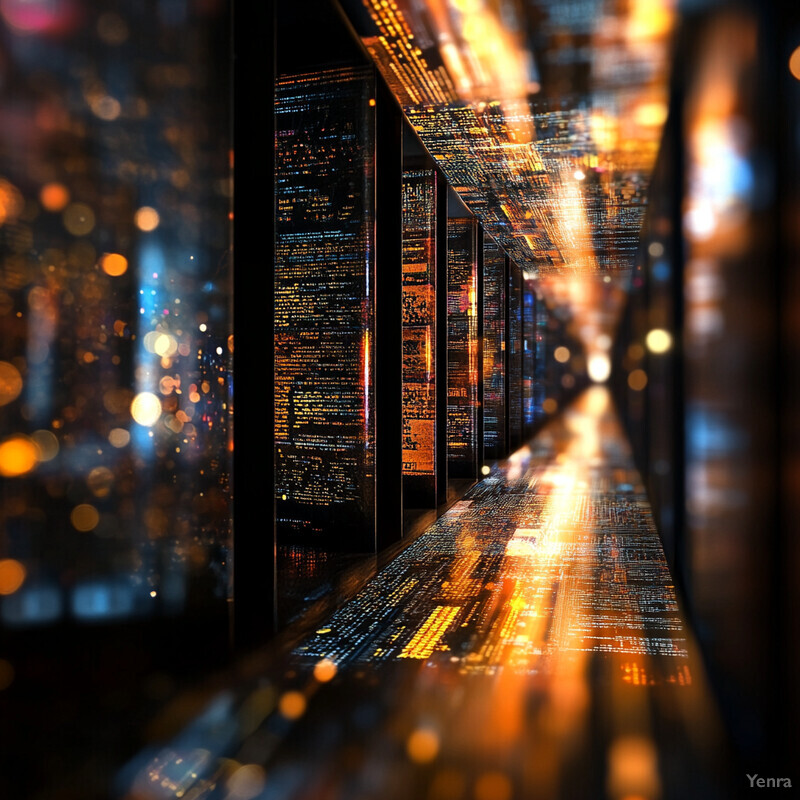
Regulatory audits demand a clear record of decision-making, actions taken, and documentation reviewed. AI can automatically create detailed, timestamped logs of all compliance activities, including changes to policies, updates to systems, and the reasoning behind specific alerts. This level of traceability makes it easier for internal and external auditors to verify that compliance protocols were followed. By ensuring that every decision is documented and easy to trace, institutions can demonstrate transparency and accountability. This fosters trust with regulators and stakeholders and mitigates the risk of non-compliance sanctions.
14. Regulatory Gap Analysis
AI compares internal policies and procedures with evolving regulations, identifying discrepancies and recommending necessary changes or additional controls.
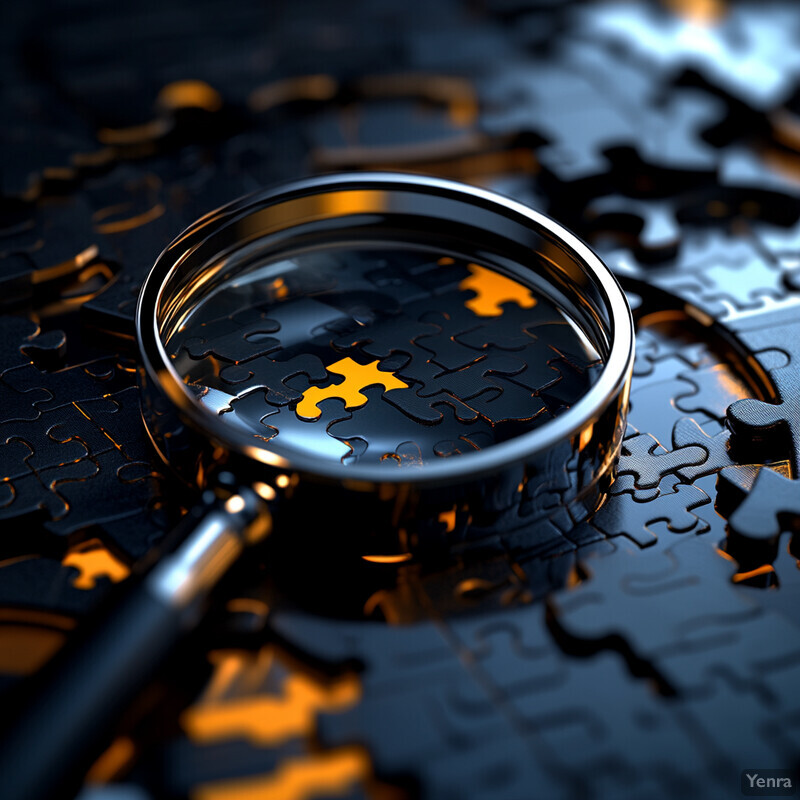
Identifying gaps between an institution’s current policies and newly enacted regulations can be complex. AI-driven regulatory gap analysis tools scan internal policies, procedures, and controls, comparing them against updated legislative or regulatory texts. By highlighting discrepancies, the tools guide compliance teams in refining existing controls, drafting new procedures, or implementing training programs for staff. As a result, institutions can address potential compliance weaknesses before they are flagged by regulators. This proactive approach enhances regulatory readiness and reduces the likelihood of costly remediation efforts.
15. Scenario Testing and Stress Testing
Machine learning models can simulate various market, geopolitical, or compliance scenarios, forecasting impacts on regulatory adherence and suggesting mitigation strategies.
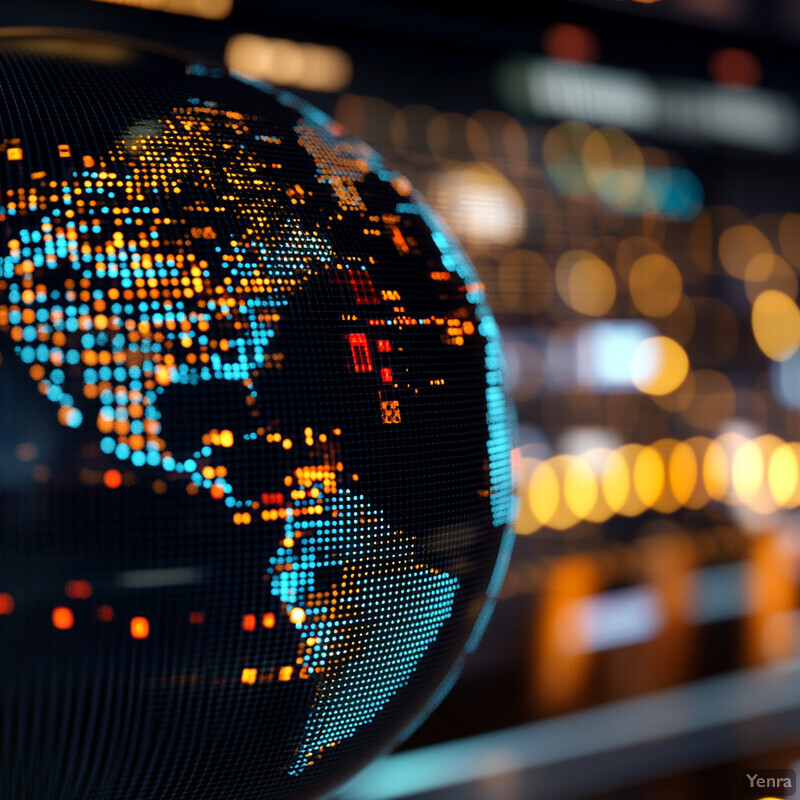
AI-based models can simulate a range of scenarios—such as market downturns, geopolitical unrest, or cybersecurity incidents—to determine how compliance frameworks hold up under pressure. By evaluating how regulations would be met in these hypothetical situations, institutions can identify potential compliance shortfalls and develop contingency plans. These tests enable a forward-looking compliance strategy, ensuring that policies are robust enough to handle unexpected changes. As models learn from actual outcomes and near misses, scenario testing becomes increasingly accurate and valuable. Ultimately, this leads to more resilient and future-proof compliance programs.
16. Compliance Chatbots and Virtual Assistants
Intelligent agents assist compliance officers with on-demand answers to complex regulatory questions, pulling from a wealth of structured and unstructured data sources.
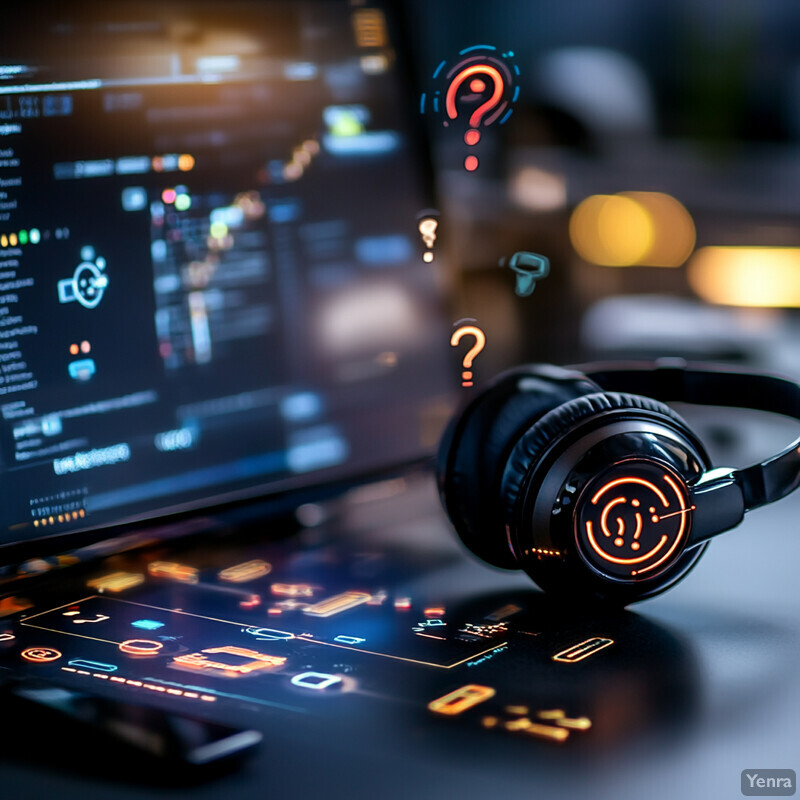
Intelligent virtual assistants can provide immediate, on-demand guidance to compliance officers, employees, or even customers who need clarification on regulatory matters. By drawing from internal policy repositories, external rulebooks, and past compliance cases, chatbots can deliver quick, accurate answers. They can also guide users through complex procedures, ensuring that proper steps are followed. Over time, these virtual assistants learn from user queries, becoming more adept at providing context-specific advice. By making compliance knowledge more accessible, chatbots help instill a stronger culture of compliance throughout the organization.
17. Entity Resolution and Network Analysis
AI-based entity resolution helps uncover hidden relationships between customers, vendors, and transactions, revealing intricate networks that might indicate misconduct.
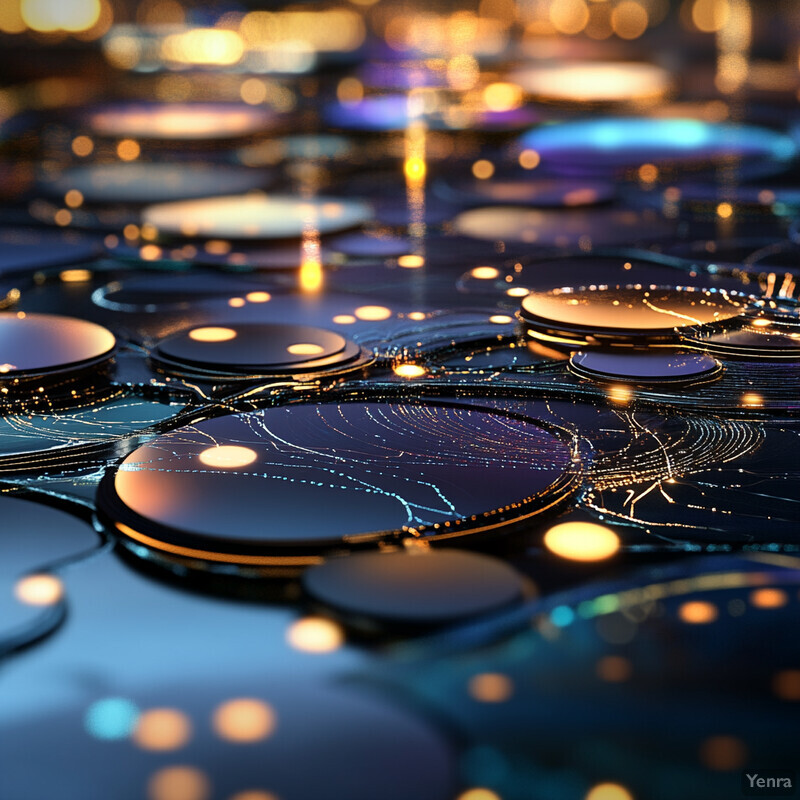
AI-driven entity resolution tools consolidate information from disparate systems and data sets, accurately linking accounts, customers, counterparties, and transactions. By revealing complex ownership structures or indirect relationships, these solutions can uncover hidden networks and patterns of suspicious activity. Applying graph analysis and machine learning, the tools detect entities linked by unusual payment flows or shared characteristics that may indicate collusion or money laundering. Improved entity resolution gives compliance teams a more holistic view of risk, enabling them to address potential issues more effectively. This network-level insight is invaluable for proactive compliance investigations.
18. Dynamic Threshold Setting
Machine learning helps set smarter thresholds for alerts and exceptions, reducing false positives and false negatives, and ensuring that compliance teams focus on truly risky cases.
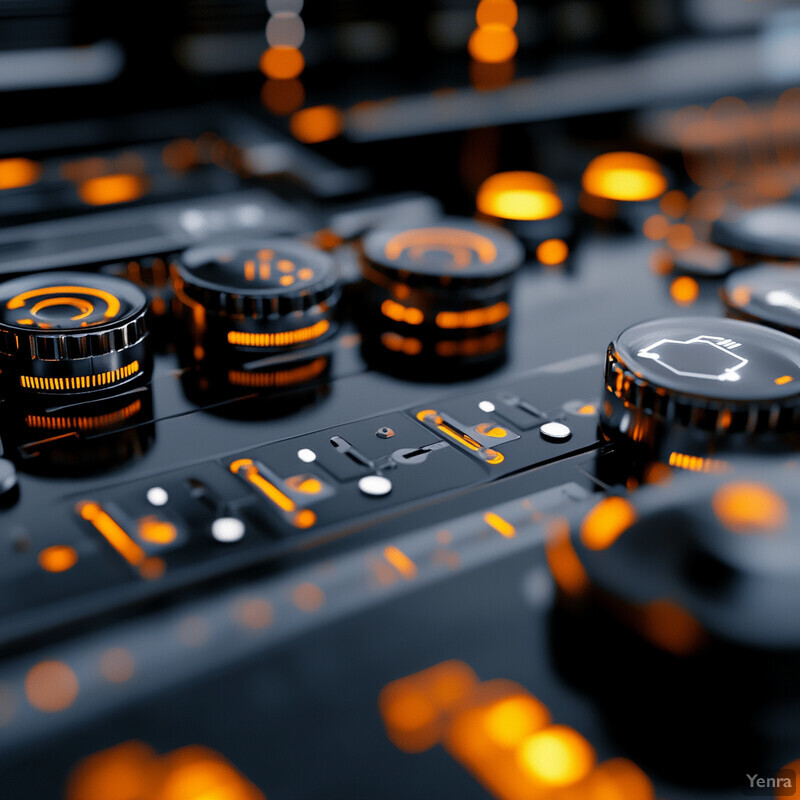
Traditional compliance systems rely on static thresholds for flagging anomalies, often leading to either too many false positives or missed threats. AI models can dynamically adjust alert thresholds based on evolving transaction volumes, customer profiles, and historical behavior. By continuously learning from feedback, these models improve their sensitivity to genuine risks and reduce unnecessary alerts. Over time, this results in a more efficient allocation of investigative resources and less investigative fatigue. Dynamic thresholds ensure that compliance efforts remain effective and finely tuned to changing business realities.
19. Predictive Benchmarking
AI tools benchmark an institution’s compliance posture against industry standards, highlighting potential weaknesses and guiding strategic improvements.
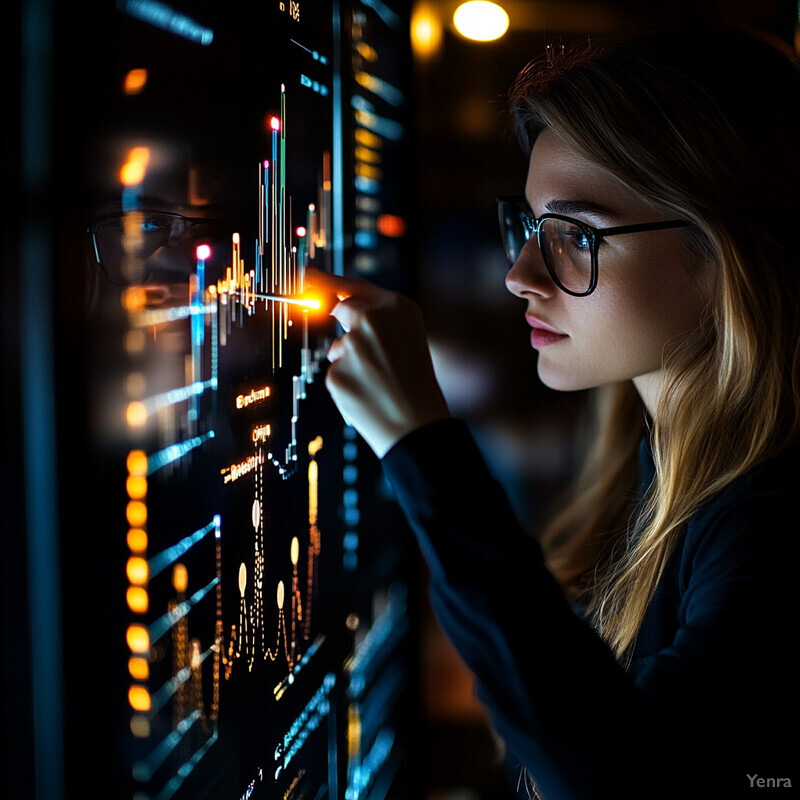
AI-enabled benchmarking compares an institution’s compliance metrics against industry standards, peer performance, or historical trends. By identifying where a firm stands relative to best practices, these analytics highlight areas for improvement and guide strategic investments in compliance infrastructure. Predictive models can also forecast future regulatory environments and estimate how well the institution would adapt to new rules. This forward-looking insight helps prioritize compliance initiatives, ensuring that resources are used optimally and that institutions stay ahead of regulatory curves. Benchmarking ultimately drives continuous improvement in compliance standards.
20. Explainable AI Models
Advanced explainability methods ensure that the reasoning behind compliance decisions made by AI models is transparent, satisfying regulatory requirements for accountability and fairness.
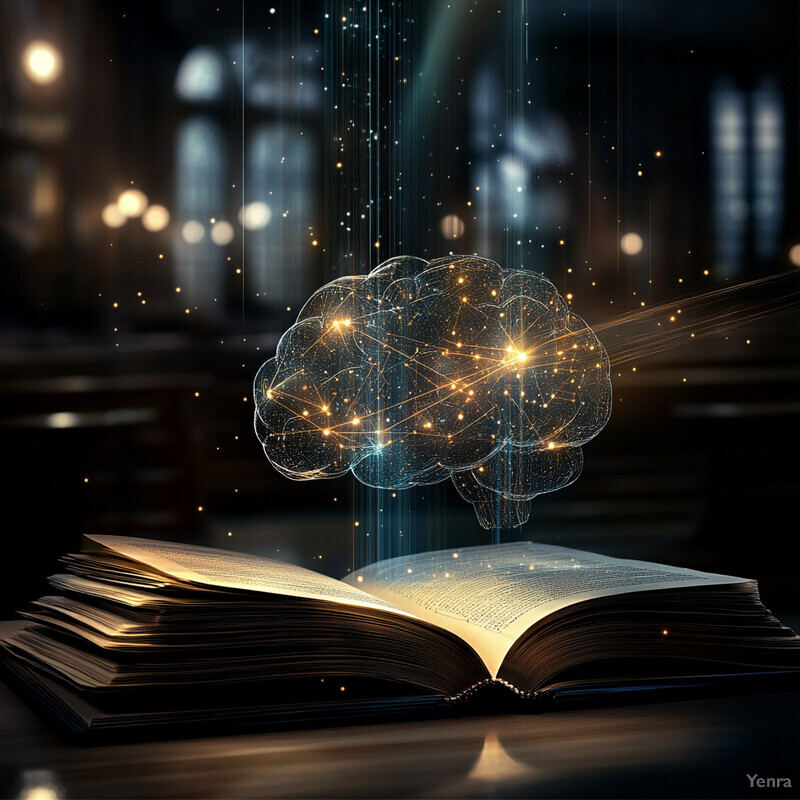
Regulators increasingly demand transparency in the decisions made by AI systems. Explainable AI techniques, such as model-agnostic explanations or feature importance insights, clarify how complex models arrive at their conclusions. This ensures that compliance officers and auditors can understand the basis for alerts, risk scores, or policy recommendations. By providing interpretable reasoning, explainable AI not only meets regulatory requirements but also builds trust in AI-driven compliance tools. As a result, institutions can confidently deploy advanced analytics while maintaining accountability, fairness, and ethical standards in their compliance processes.