1. Trend Prediction via Social Media Analysis
AI algorithms can scan millions of social media posts, influencer feeds, and fashion-related hashtags to identify emerging microtrends, color palettes, silhouettes, and patterns before they hit mainstream runways.
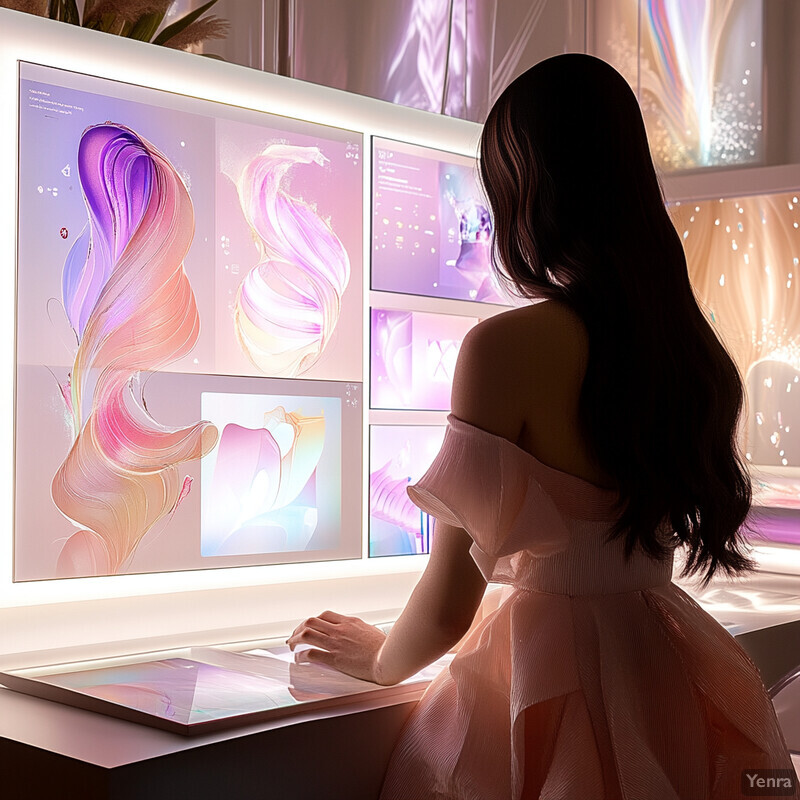
By leveraging natural language processing (NLP) and computer vision tools, AI can sift through enormous volumes of social media content to identify trends as they take shape. It examines hashtags, captions, influencers’ posts, and user interactions across platforms like Instagram, TikTok, and Pinterest. These AI-driven insights help brands recognize early-stage patterns—such as the growing popularity of a particular silhouette or the sudden interest in a certain niche aesthetic—long before they appear in runway shows or mainstream fashion magazines. This allows companies to make more informed design and merchandising decisions, speeding up product development and ensuring that their offerings align with the pulse of the digital fashion community.
2. Image Recognition for Style Identification
Advanced computer vision techniques enable automated identification of garment types, textures, and patterns from images, allowing for quicker classification of styling elements and seamless curation of lookbooks.
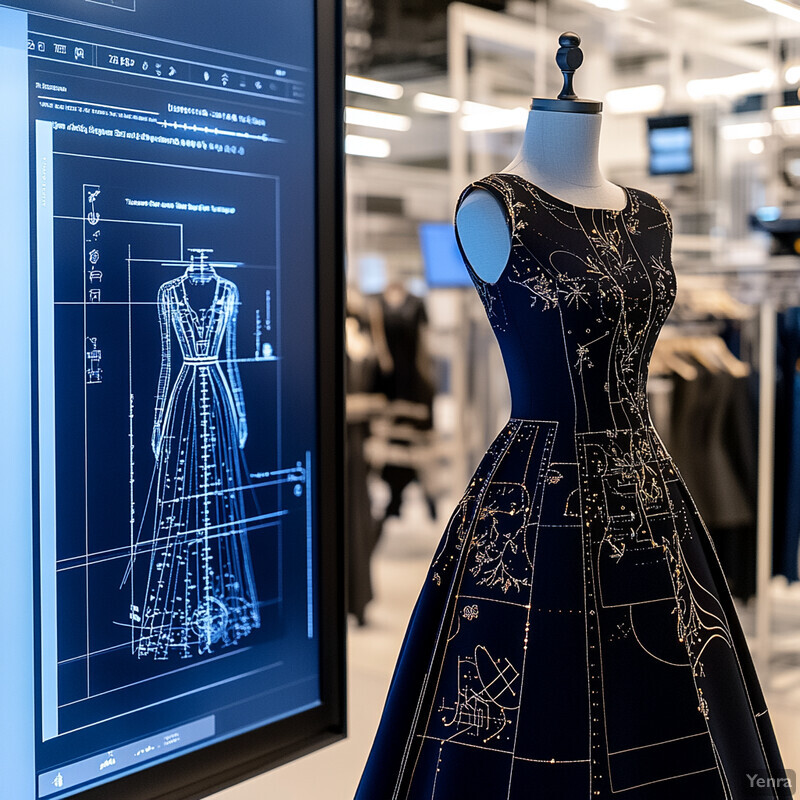
Advancements in computer vision enable AI to classify and tag garment attributes—such as sleeve length, collar style, fabric texture, and print patterns—by analyzing photographs. As a result, entire collections can be cataloged accurately without extensive human labor. This automated style identification can help retailers swiftly organize their product databases, recommend visually similar items, and even power visual search features so customers can find what they like simply by uploading an image. Over time, the system becomes more adept at recognizing subtle details and nuances, aiding in more intricate styling recommendations and boosting user satisfaction through easy navigation and discovery.
3. Personalized Outfit Recommendations
Machine learning models can analyze individual consumers’ past purchases, browsing history, and personal style preferences to recommend highly tailored outfits or accessory combinations, enhancing customer satisfaction and loyalty.
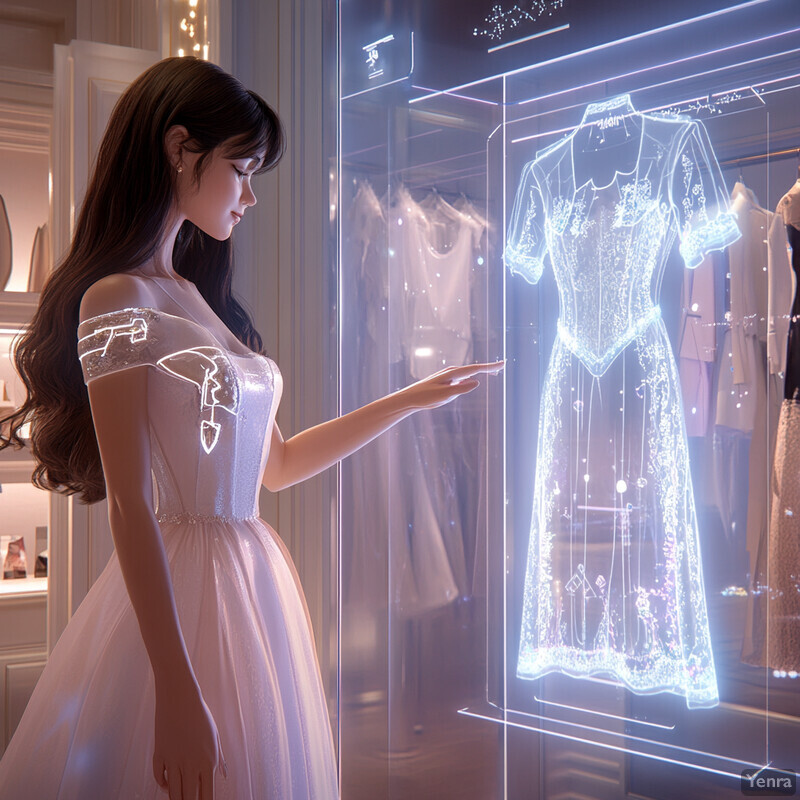
AI-driven recommendation engines leverage data on a customer’s past purchases, browsing behaviors, size preferences, and even climate considerations to provide tailored outfit suggestions. By combining factors like user demographics, historical shopping patterns, and current fashion trends, these algorithms create a curated shopping experience that feels like working with a personal stylist. Not only does this boost user engagement and satisfaction—since shoppers feel understood and catered to—it also increases conversion rates. Brands see improved loyalty as customers return to the platform for consistently relevant suggestions that evolve alongside their changing tastes.
4. Real-Time Trend Spotting
AI tools can monitor and process data from global street style photography and fashion weeks in real time, detecting subtle shifts in consumer tastes and identifying key influencers who drive emerging trends.
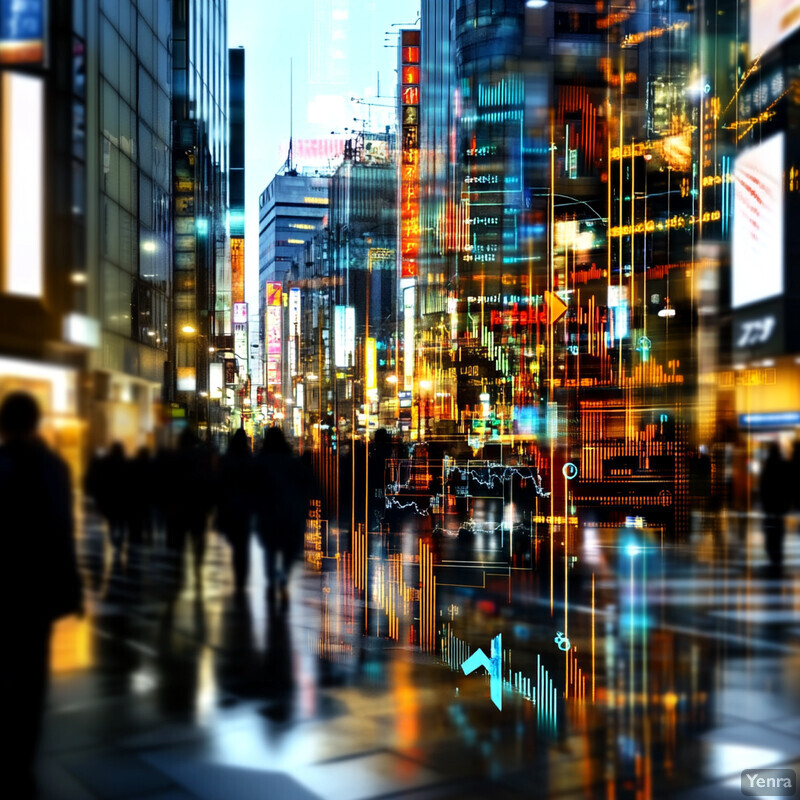
Thanks to AI, fashion houses no longer have to rely solely on retrospective analyses of past seasons to anticipate what’s next. Real-time trend detection tools comb through street style photos, influencer live streams, and reports from ongoing fashion weeks. They analyze patterns from multiple data inputs—runway looks, consumer feedback, social chatter—on a day-to-day or even hourly basis. As a result, brands can respond almost instantly to shifts in consumer preferences, quickly adjusting marketing campaigns, design concepts, and merchandising strategies. This rapid response capability helps brands stay ahead of the curve, preventing missed opportunities and ensuring relevance in a fast-paced industry.
5. Automated Styling Assistants
Conversational AI and virtual stylists can interact with customers, asking questions about their personal style and suggesting outfits that match their body type, event requirements, and personal taste—replicating the role of a human stylist at scale.
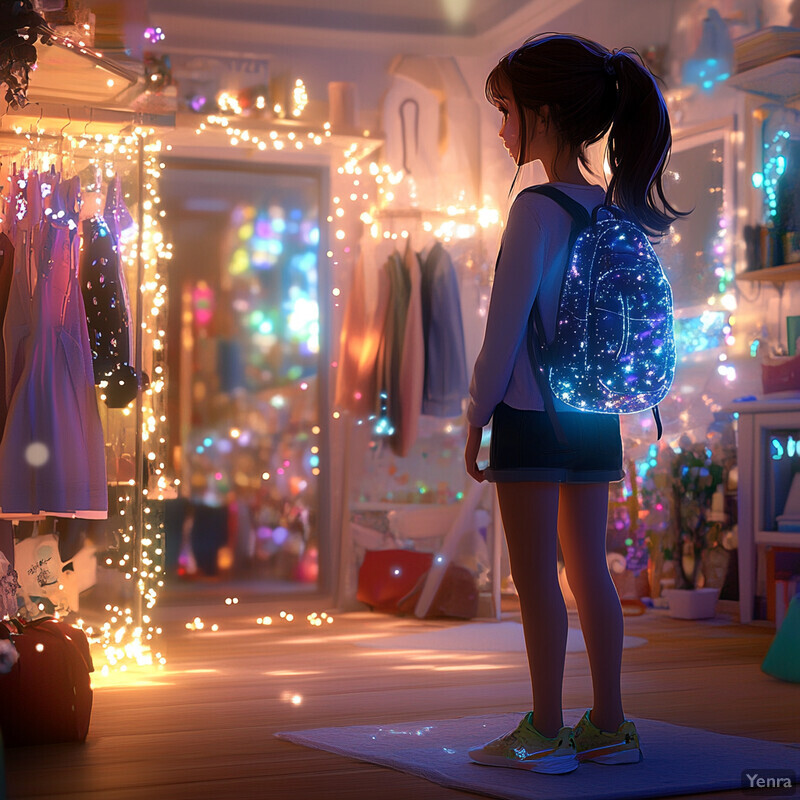
Conversational AI and virtual stylist chatbots use NLP and machine learning to understand a user’s style questions or requests. These digital assistants can ask clarifying questions—such as preferred fits, occasion types, color palettes—and then deliver curated outfit recommendations that fit the individual’s unique style profile. Unlike traditional chatbots, these AI stylists also learn from each interaction, refining their suggestions over time. By providing a personalized human-like consultation at scale, they bridge the gap between the mass market and highly personalized, boutique-level customer service. This kind of personal touch, powered by AI, helps foster deeper brand-consumer relationships.
6. Predictive Inventory Management
By forecasting upcoming trends accurately, retailers can better anticipate demand for specific styles, reducing overstock or shortages and minimizing waste in the supply chain.
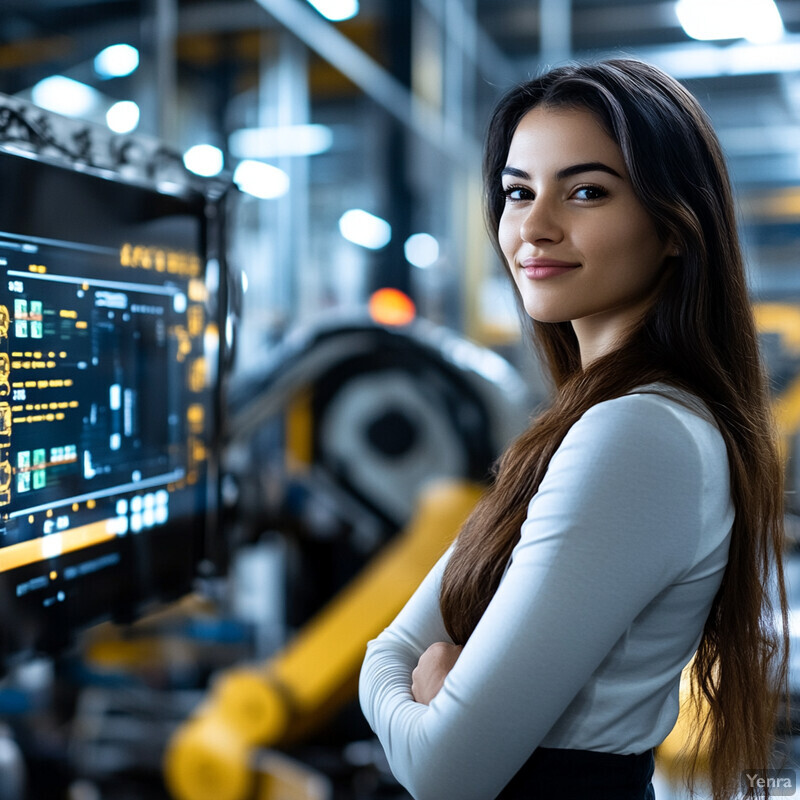
A key challenge in fashion retail is balancing supply and demand, ensuring that popular items do not run out of stock prematurely and that slow-moving inventory doesn’t lead to waste. AI-driven predictive models help by anticipating which trends will take off and when certain products will peak in popularity. These insights enable retailers to make smarter decisions about production volumes, inventory placement, and timing. As a result, companies reduce markdowns, minimize waste, and align their supply chain activities more closely with real-time demand, improving both profitability and sustainability.
7. Color and Pattern Forecasting
Machine learning algorithms can identify cyclical pattern occurrences and color prevalence over time, helping designers and brands predict which hues and motifs will resonate in future collections.
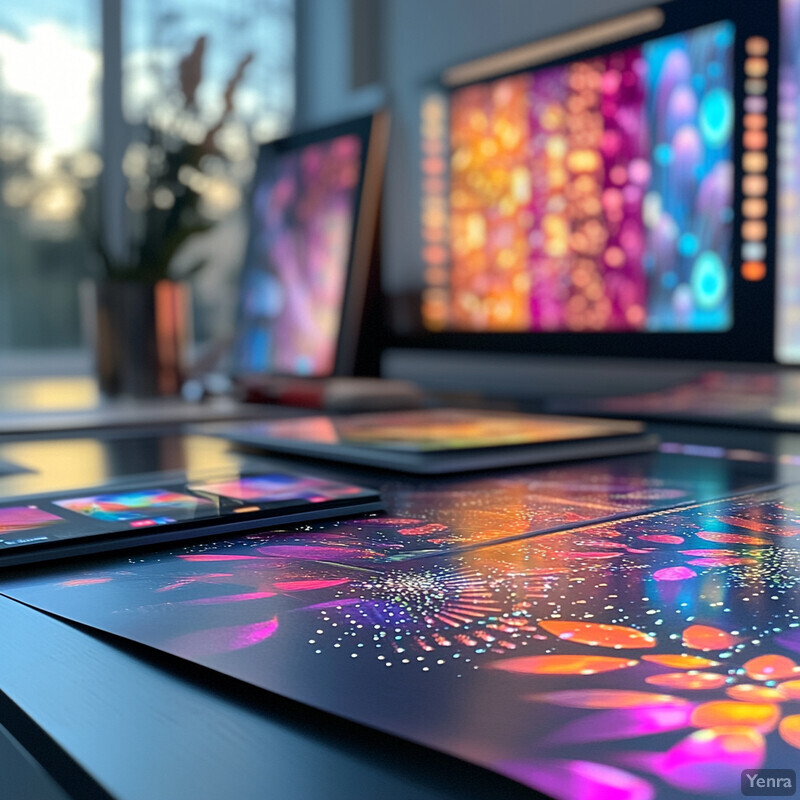
Colors and patterns are central to defining each season’s aesthetic identity. AI trend forecasting models use historical data, runway imagery, and emerging consumer signals to predict which hues, motifs, and textures are likely to gain traction. By understanding these patterns ahead of time, designers can incorporate the right elements into future collections, ensuring that their lines resonate when they hit the market. Additionally, these predictions help textile manufacturers and suppliers plan their materials, enabling the industry to operate more efficiently and reduce the risk of stocking unpopular fabrics or prints.
8. 3D Virtual Fitting and Try-Ons
AI-powered virtual reality (VR) and augmented reality (AR) solutions allow consumers to virtually try on clothing and accessories, giving brands immediate feedback on fit and style preferences.
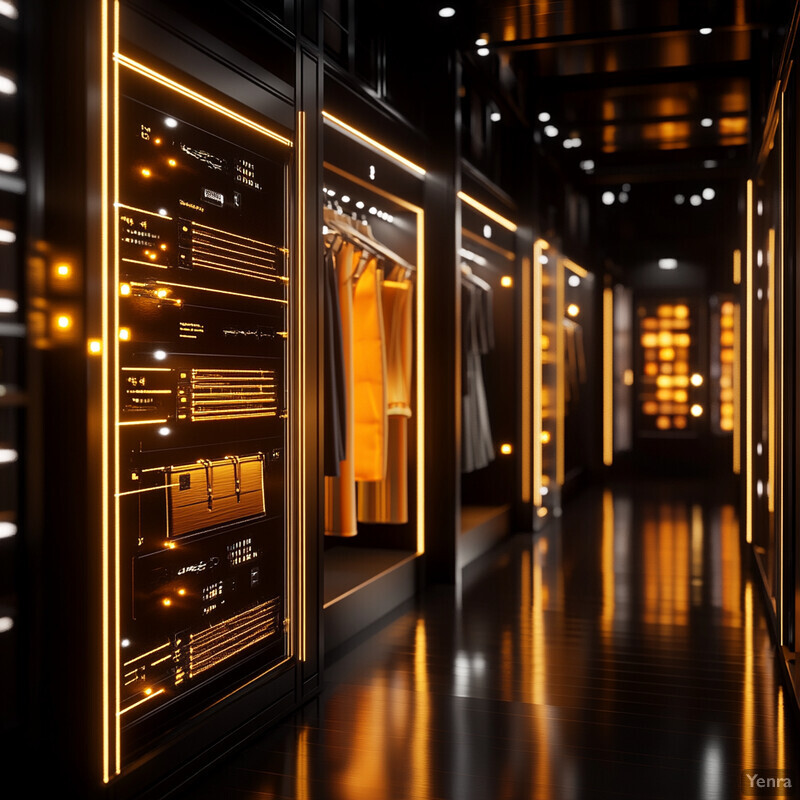
The integration of augmented reality (AR) and virtual reality (VR) technologies allows consumers to try on garments in a digital environment, reducing returns and improving the shopping experience. Advanced AI models simulate how different fabrics drape and move, while virtual body modeling technology accounts for individual body shapes and sizes. This not only empowers consumers to make confident purchase decisions but also offers brands immediate feedback on fit and style preferences. Over time, brands can refine their sizing charts and product designs based on this data, ultimately improving product-market fit and reducing return rates.
9. Fabric and Material Innovation
Using AI to analyze properties of various textiles and historical performance data, designers can predict which fabrics are best suited for certain styles and forecast the materials consumers will prefer for comfort and sustainability.
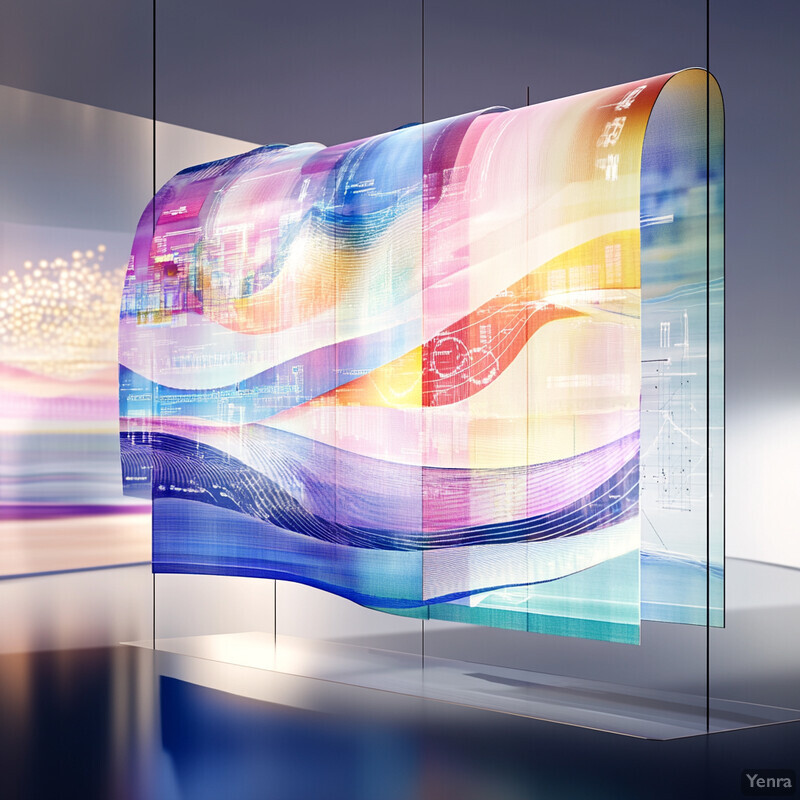
AI tools can analyze the historical performance of various fabrics, comparing their durability, comfort, and overall customer satisfaction ratings. With these insights, designers can select materials that not only align with forthcoming stylistic trends but also meet consumer expectations for quality and sustainability. Such data-driven decision-making reduces trial and error in fabric sourcing and can encourage innovation—leading to the adoption of cutting-edge materials that have the right feel, cost profile, and environmental footprint. Over time, this approach contributes to a more sustainable and forward-thinking fashion ecosystem.
10. Customer Preference Clustering
Advanced clustering algorithms can segment customers into style-based groups, helping retailers understand unique market niches and design collections that speak directly to those segments.
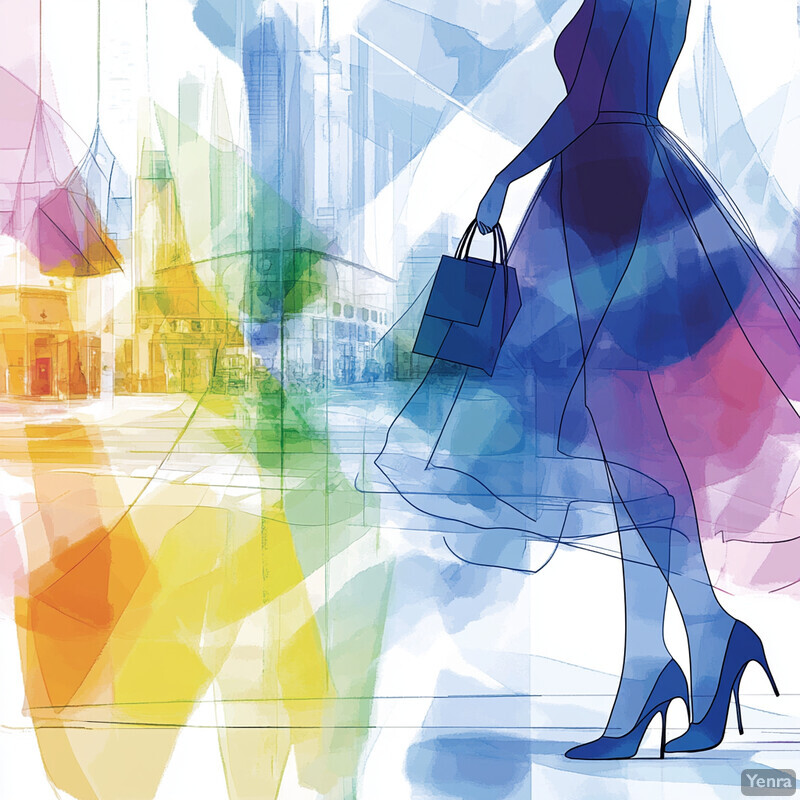
AI-powered clustering algorithms group similar consumer profiles based on their shopping behaviors, style preferences, and engagement levels. By understanding these natural segments—such as athleisure enthusiasts, vintage aficionados, or luxury seekers—brands can tailor their product lines and marketing campaigns more effectively. This granular understanding allows them to speak directly to each group’s core desires, offering personalized collections and communications. In turn, customers feel more recognized and served, which strengthens brand loyalty and encourages repeat visits, ultimately boosting overall sales.
11. Influencer Impact Analysis
AI can quantify and qualify the influence of fashion bloggers, vloggers, and Instagram personalities, correlating their styles with subsequent consumer purchasing behaviors, thus guiding brand partnerships and collaborations.
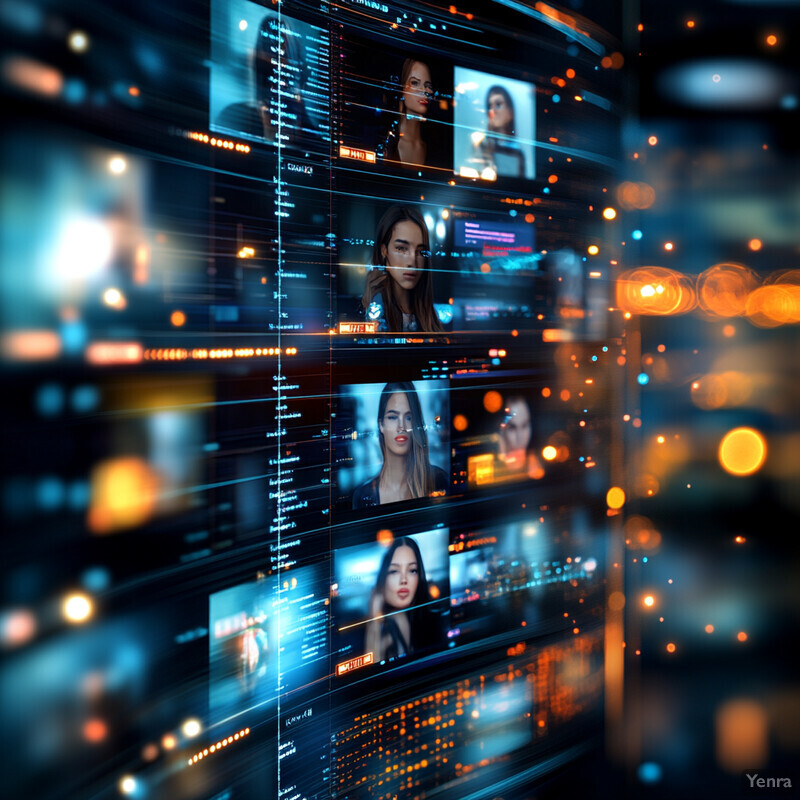
Influencers play a vital role in shaping consumer perceptions and purchasing patterns. AI-driven tools measure the true impact of influencers by analyzing followers’ engagement, conversions from influencer-driven campaigns, and subsequent shifts in style preferences. By correlating these metrics, brands identify which influencers best align with their target demographics and aesthetic values. This helps them invest more strategically in influencer partnerships, ensuring maximum return on marketing spend and strengthening the authenticity and reach of their brand message.
12. Social Listening for Early Signals
By monitoring online forums, comments, and user-generated content, AI can identify subtle shifts in sentiment toward certain trends, styles, or brands long before sales data catches up.
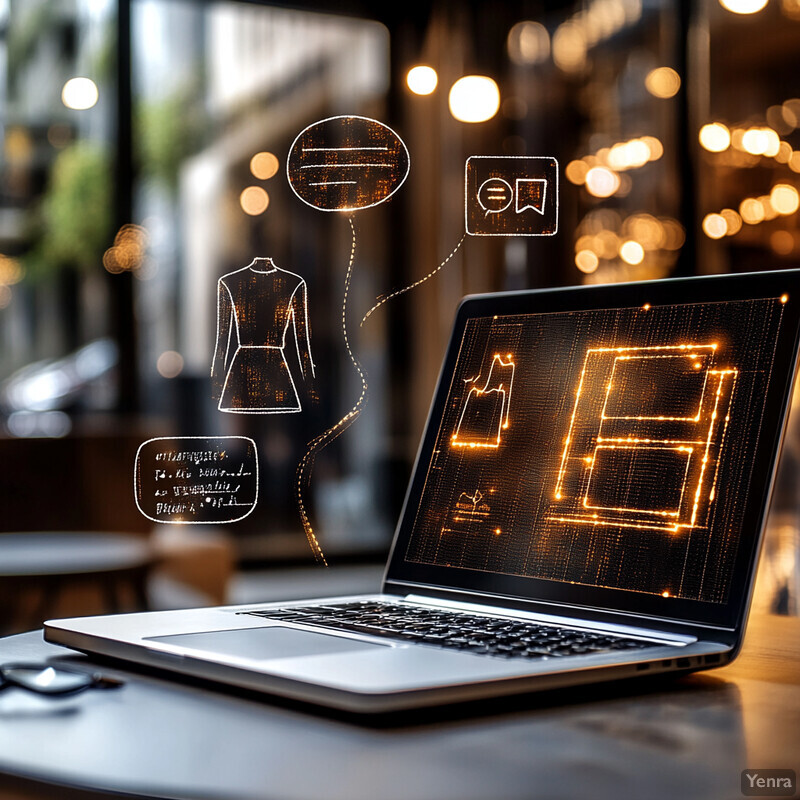
Beyond explicit trend signals, subtle shifts in consumer sentiment—captured through comments, reviews, and forum discussions—offer valuable clues about emerging preferences and potential pain points. AI-driven social listening tools can identify these subtle changes earlier than traditional methods. This allows brands and designers to course-correct before a small issue becomes a widespread problem. For example, if consumers start voicing concerns about sustainability, companies can pivot toward eco-friendly materials or adjust their messaging to emphasize ethical production. The result is a more proactive, consumer-responsive approach to fashion creation and promotion.
13. Fashion Show Analysis and Summaries
AI can quickly process runway footage to identify dominant silhouettes, recurring color themes, and fresh design elements, producing data-driven recaps of each season's highlights.
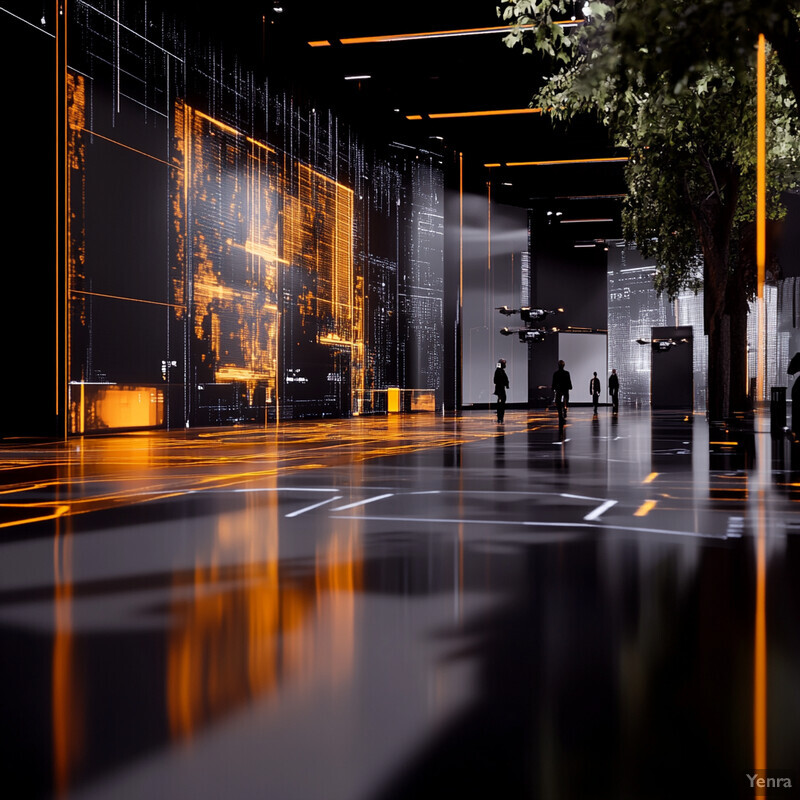
Runway shows generate a rich visual dataset of future trends, but manually analyzing each look across multiple shows is time-consuming. AI solutions can rapidly break down each outfit, identifying key silhouettes, colors, and textures and comparing them across designers and seasons. This results in structured insights that highlight common themes and where designers are diverging. By delivering data-rich summaries, AI empowers trend forecasters, buyers, and creatives to understand which directional shifts are likely to catch on, enabling informed decision-making for future collections.
14. Automated Mood Boards
Generative AI can produce thematic mood boards and style guides by pulling from a variety of images and inspirations, helping designers conceptualize new collections more efficiently.
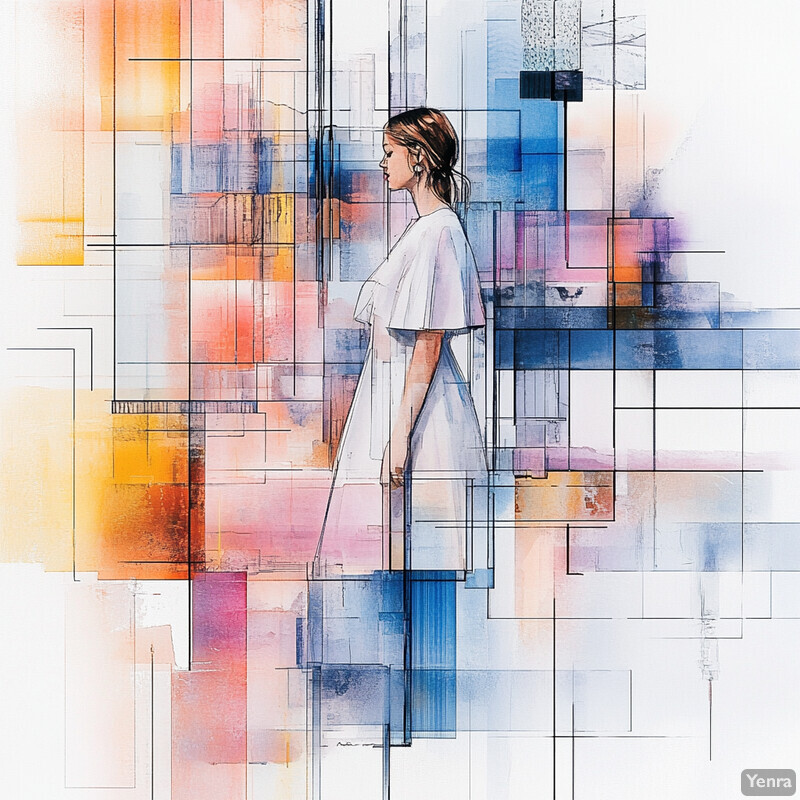
Mood boards help designers conceptualize their creative visions, yet assembling them manually can be time-intensive. Generative AI tools can produce thematic collections of images, textures, and color palettes based on user prompts. By blending user inspiration with AI-curated imagery, the process of ideation becomes more efficient and exploratory. Designers receive instant visual references aligned with their conceptual brief, helping them reach a refined aesthetic direction faster. Ultimately, this accelerates the design cycle and encourages more experimentation and innovation.
15. Demand Forecasting Across Markets
By comparing online search trends, local cultural factors, and climate patterns, AI can help brands predict which styles will be popular in different regions, guiding product localization and targeted marketing.
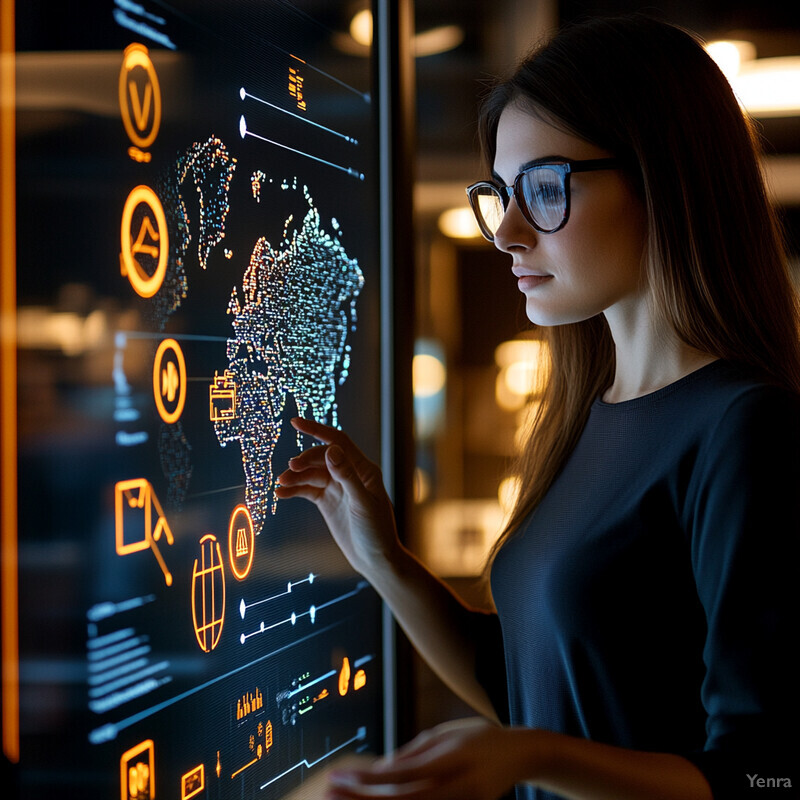
Fashion preferences differ vastly by geography, climate, and cultural influences. AI-powered demand forecasting tools consider these variables, along with online search trends and local events, to predict which styles will resonate in different regions. These localized insights allow brands to tailor their product assortments, marketing messages, and pricing strategies for each target market. Such precise customization ensures that consumers find relevant, culturally appropriate options, boosting brand appeal and increasing sell-through rates.
16. Competitive Intelligence
AI tools can analyze competitor product lines, price points, promotional strategies, and consumer engagement to inform a brand’s strategic decisions on future styling and marketing directions.
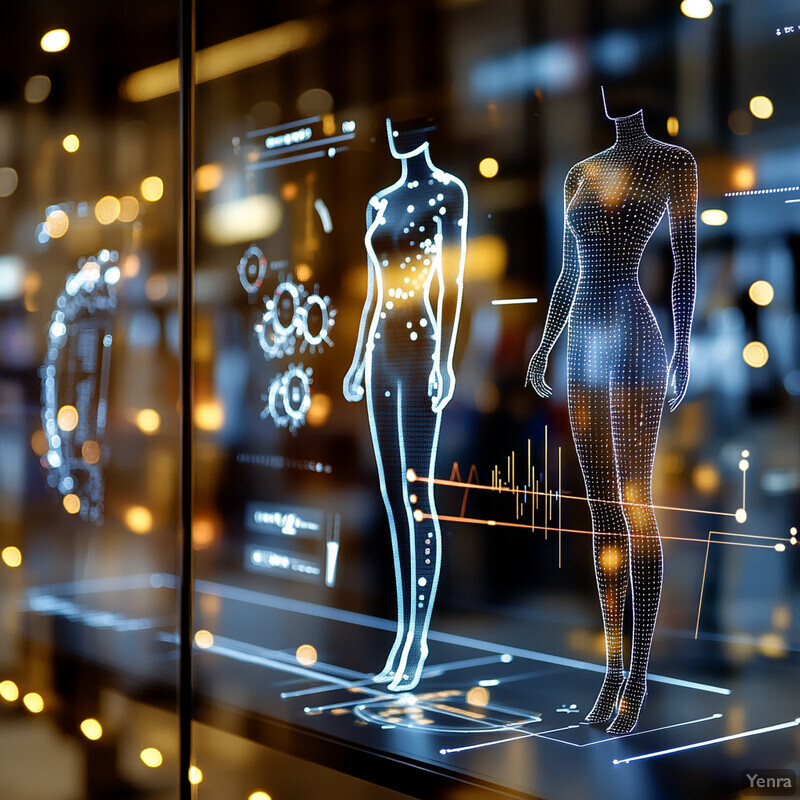
Staying ahead in the fashion industry often means keeping a close eye on competitors. AI-driven competitive intelligence tools analyze rival brands’ offerings, pricing strategies, inventory movements, and promotional campaigns. By correlating these findings with internal performance metrics, brands can identify gaps in their product lines, respond swiftly to price changes, and anticipate new trend directions prompted by competitor moves. In essence, AI transforms competitive analysis into a continuous, data-informed process, enabling businesses to adjust their strategies preemptively and maintain a competitive edge.
17. Sustainability Analytics
Trend forecasting integrated with sustainability metrics helps predict which eco-friendly materials, recycled fabrics, or zero-waste production methods will become more appealing, guiding ethical decision-making in fashion.
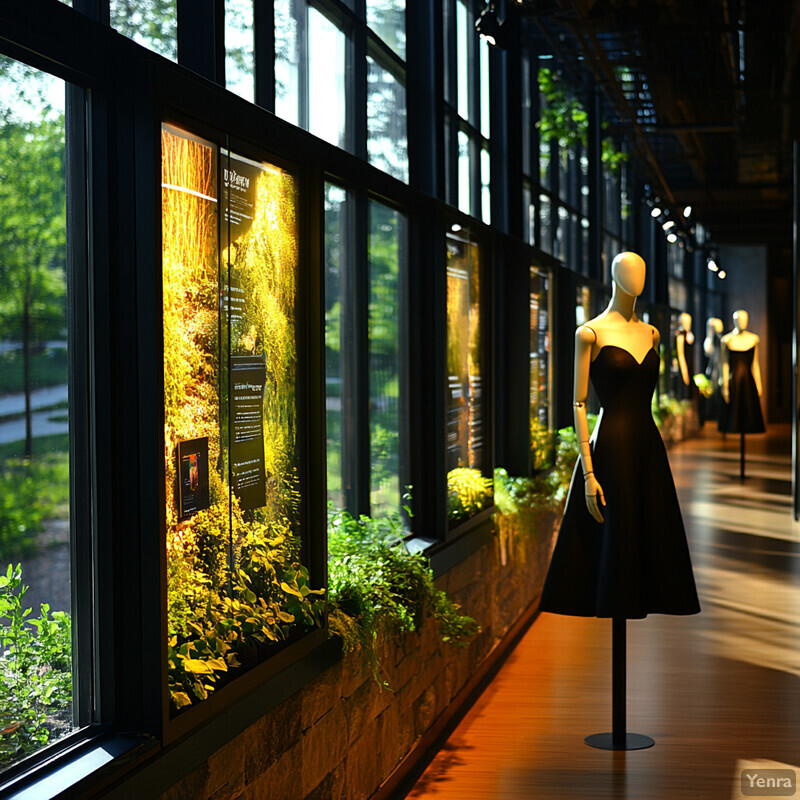
Consumer demand for ethically produced, eco-friendly fashion continues to rise. AI can integrate environmental impact assessments and sustainability ratings into trend forecasting models, highlighting which sustainable materials, production methods, and circular business practices are likely to gain popularity. By proactively aligning with these insights, brands can position themselves as leaders in responsible fashion, strengthening customer trust and loyalty. This strategic approach to sustainability not only meets evolving consumer expectations but also helps ensure long-term viability in a more environmentally conscious marketplace.
18. Historical Data Mining
Machine learning can ingest decades of historical fashion data to detect cyclical trends, providing insights into when certain silhouettes or color palettes might resurge.
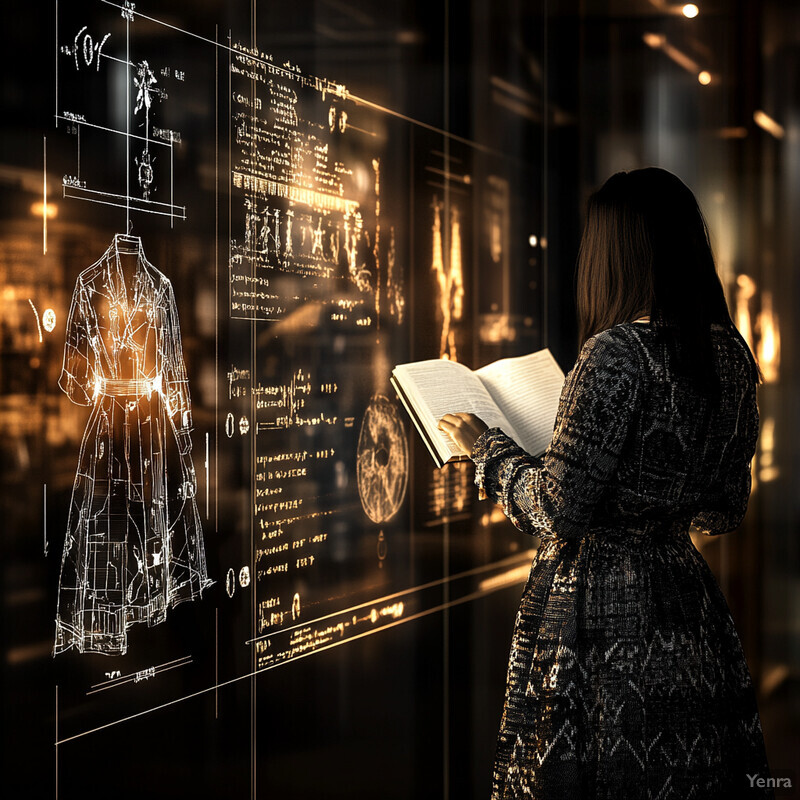
Fashion trends are cyclical, with styles from previous decades often re-emerging. Machine learning models trained on decades of fashion archives can predict when certain silhouettes, prints, or accessories might return to prominence. By correlating these historical insights with current consumer preferences, brands can bring back classic looks at precisely the right time. This reduces guesswork in the design process and allows for informed nostalgia-driven marketing, ensuring that vintage revivals feel current rather than dated.
19. Customized Brand Aesthetics
Through style transfer algorithms, designers can experiment with various aesthetics—ranging from minimalist and avant-garde to sporty and vintage—to refine a brand’s signature look based on predicted market reception.
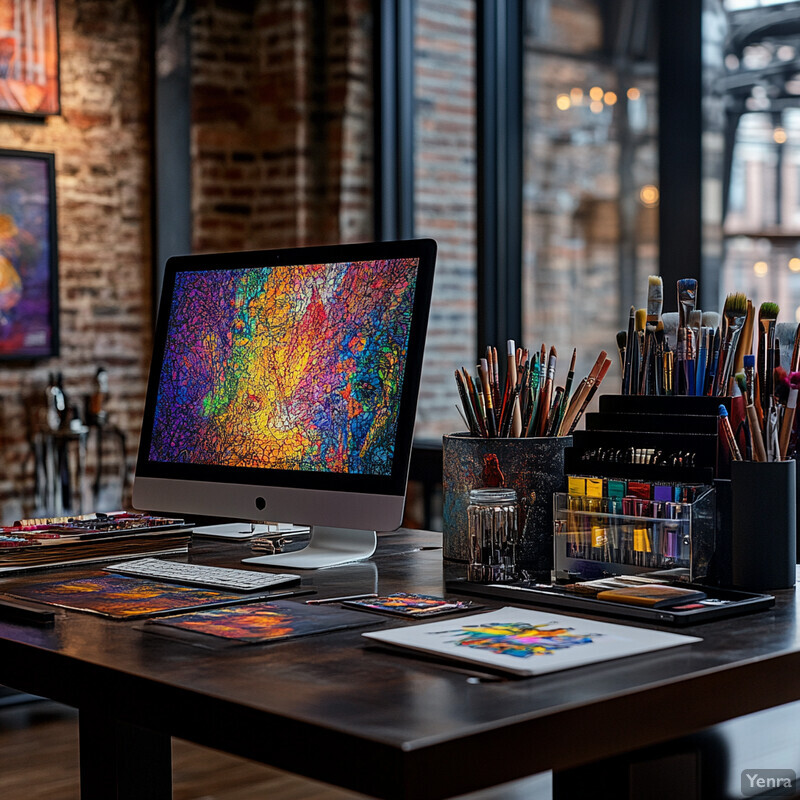
AI can assist designers in experimenting with various aesthetics—mixing and matching elements from different style genres to produce fresh brand signatures. Through style transfer algorithms and generative models, designers can quickly visualize how certain aesthetics, color schemes, or motifs would look when applied to their brand identity. This allows for rapid prototyping of signature looks that resonate with target audiences and can guide long-term brand evolution. Ultimately, it empowers creative teams to iterate more dynamically and adapt their brand’s visual language based on data-backed insights.
20. Rapid Prototyping of Concepts
AI-driven generative design tools can create multiple fashion sketches, prints, or accessory concepts rapidly, allowing designers to test the waters, gather feedback, and iterate toward trend-aligned products swiftly.
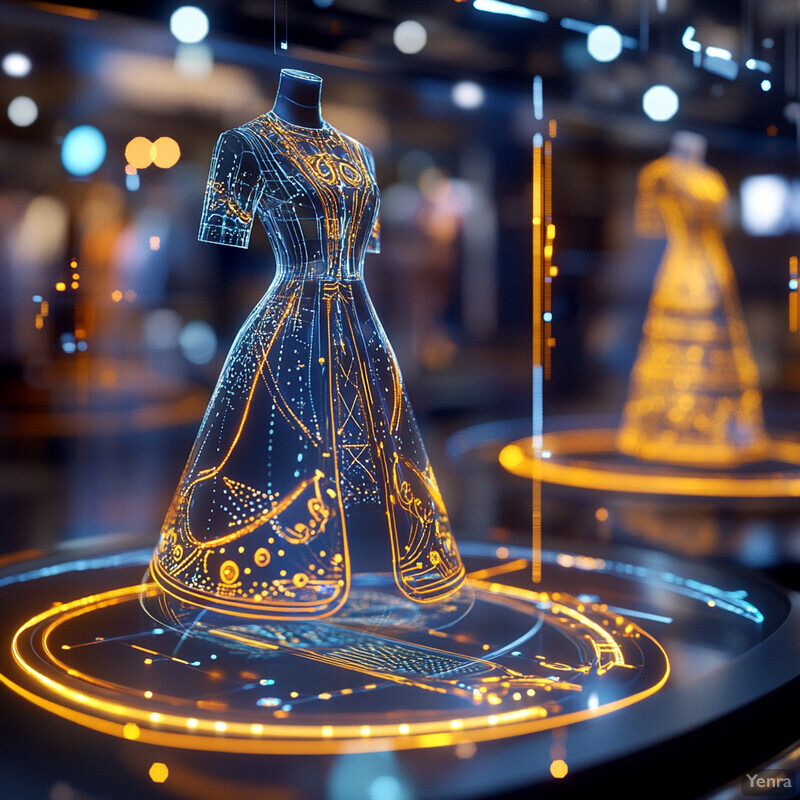
Using generative AI, design teams can produce multiple sketches, patterns, or even entire virtual line-ups of garments within hours rather than days. These prototypes can then be tested—either internally, via focus groups, or through virtual showrooms—to gauge consumer reactions. By using AI in the early stages of design, brands can weed out weaker concepts and focus resources on developing products that are more likely to succeed. This rapid ideation-to-feedback loop boosts innovation, cuts down on time-to-market, and ultimately supports a more agile and consumer-driven product development process.