1. Automated Content Classification and Tagging
AI-driven natural language processing (NLP) and machine learning algorithms automatically apply rich, consistent metadata to documents and records, reducing manual effort and improving the accuracy of information retrieval.
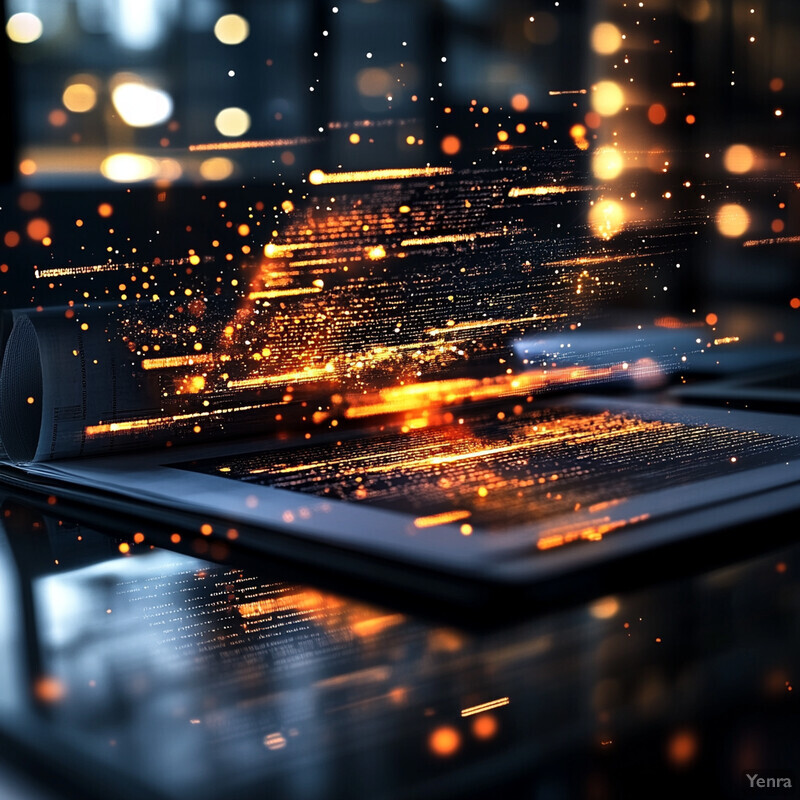
AI-driven classification systems leverage natural language processing (NLP) and machine learning models to recognize patterns within documents, emails, and other forms of enterprise content. By identifying keywords, concepts, and entities, these systems automatically assign relevant tags and metadata without the need for manual intervention. This not only improves searchability and retrieval but also ensures a consistent taxonomy across the organization. Employees benefit from faster access to information, as well-classified content reduces the time spent browsing file hierarchies or guessing at vague filenames. Over time, machine learning algorithms learn from feedback, continuously refining and improving the accuracy of the classification process, thus contributing to a more organized and navigable knowledge repository.
2. Advanced Semantic Search
Instead of relying solely on keyword matching, AI-powered search engines leverage semantic understanding to interpret the intent behind queries, delivering more relevant results and making it easier to find critical enterprise knowledge.
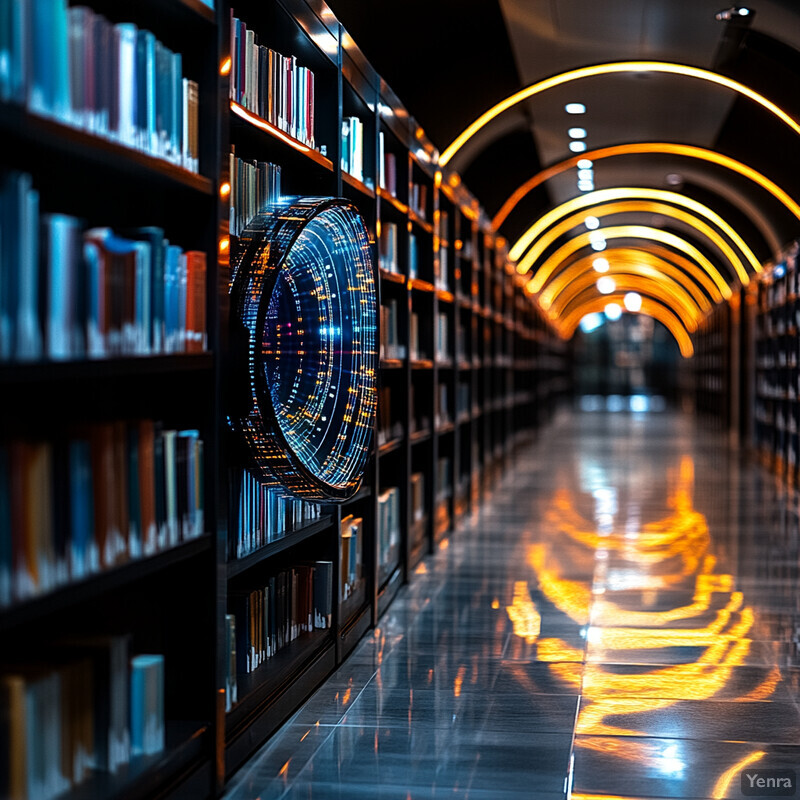
Traditional keyword-based search engines often return irrelevant or incomplete results, frustrating users who must sift through pages of content. AI-powered semantic search, on the other hand, understands the intent behind a query and the contextual relationships between terms. By capturing the nuances of language, it delivers more accurate and contextually relevant results. For example, a user searching “How can I improve manufacturing efficiency?” will see results related to process optimization, supply chain improvements, and equipment upgrades rather than simply documents containing the words “manufacturing” and “efficiency.” This semantic understanding empowers employees to quickly locate meaningful information, boosting productivity and informed decision-making throughout the organization.
3. Intelligent Knowledge Graphs
AI constructs and maintains knowledge graphs that model relationships between concepts, people, and data, enabling organizations to navigate complex informational ecosystems more intuitively and discover hidden insights.
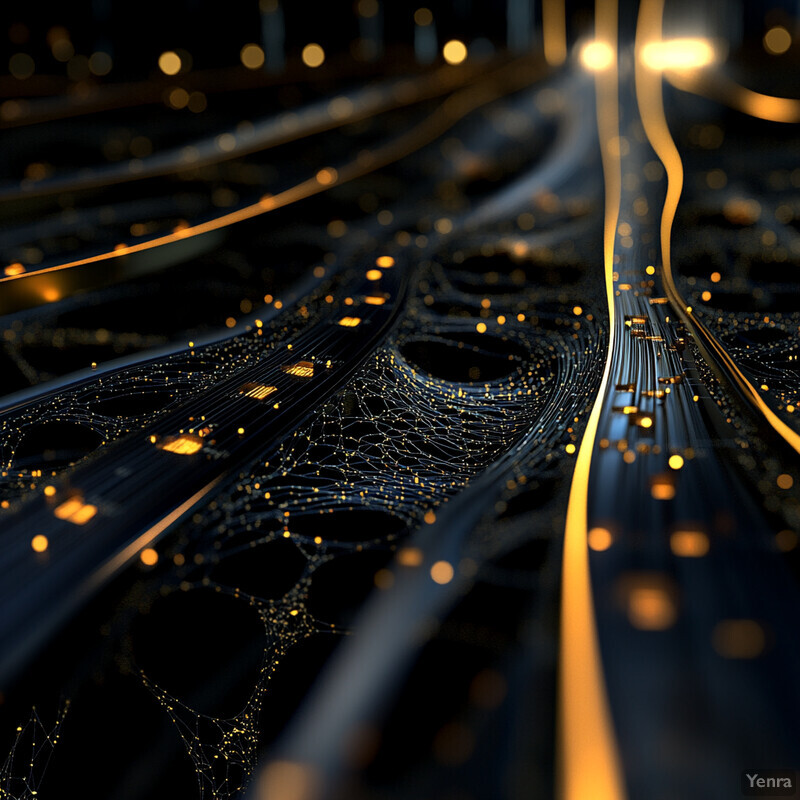
Knowledge graphs are structured representations of information that highlight the connections between entities—such as products, business units, clients, or research topics—and their attributes. AI technology helps create and maintain these knowledge graphs by analyzing relationships within massive data sets, identifying hidden links, and continuously updating the graph as new content is ingested. This visual and contextual mapping of information allows users to navigate through interconnected concepts rather than searching through isolated documents. As a result, employees discover insights and patterns that would be difficult to uncover using traditional, siloed data repositories. By offering a holistic view of enterprise knowledge, intelligent knowledge graphs enable more strategic decision-making and foster a culture of innovation.
4. Contextual Content Recommendations
Recommendation engines use pattern analysis and user behavior tracking to proactively suggest relevant documents, experts, and learning materials, ensuring that employees get the right information at the right time.
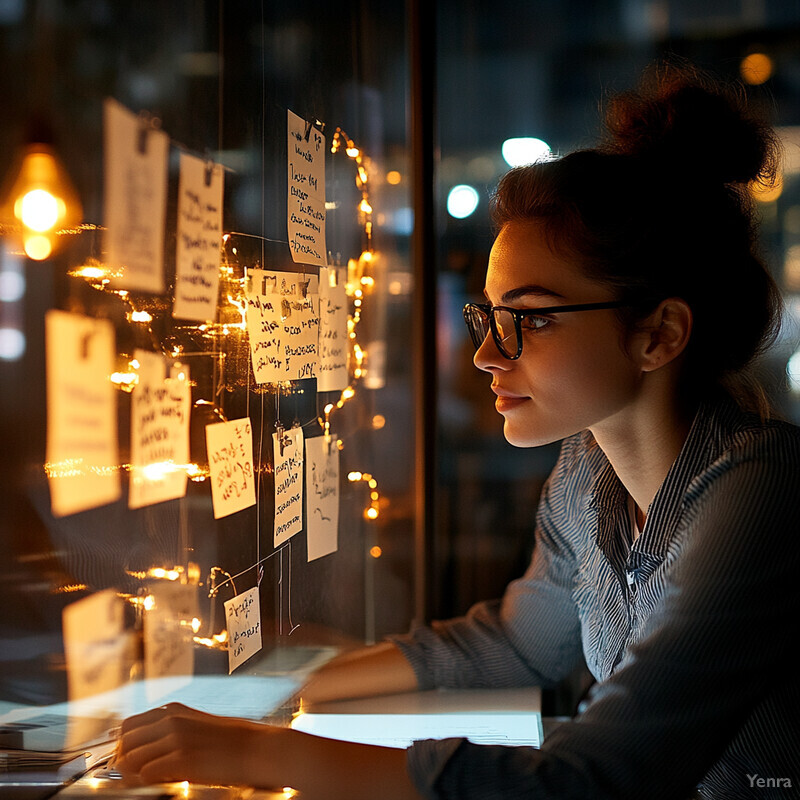
AI-powered recommendation engines leverage user behavior patterns, historical searches, interests, and contextual cues to suggest relevant documents, internal experts, or resources. For instance, if a sales representative frequently views material on a specific product line and engages with related training modules, the system can proactively suggest updated marketing collateral, pricing sheets, or relevant customer success stories. These content recommendations not only reduce the time employees spend hunting for the right information but also help them discover resources they may not have known existed. The result is a more personalized and efficient knowledge experience, leading to improved job performance and more informed interactions with colleagues and clients.
5. Automated Summarization and Content Distillation
AI systems can generate concise summaries of lengthy documents, policies, or research papers, helping users quickly grasp essential points and reduce time-to-insight.
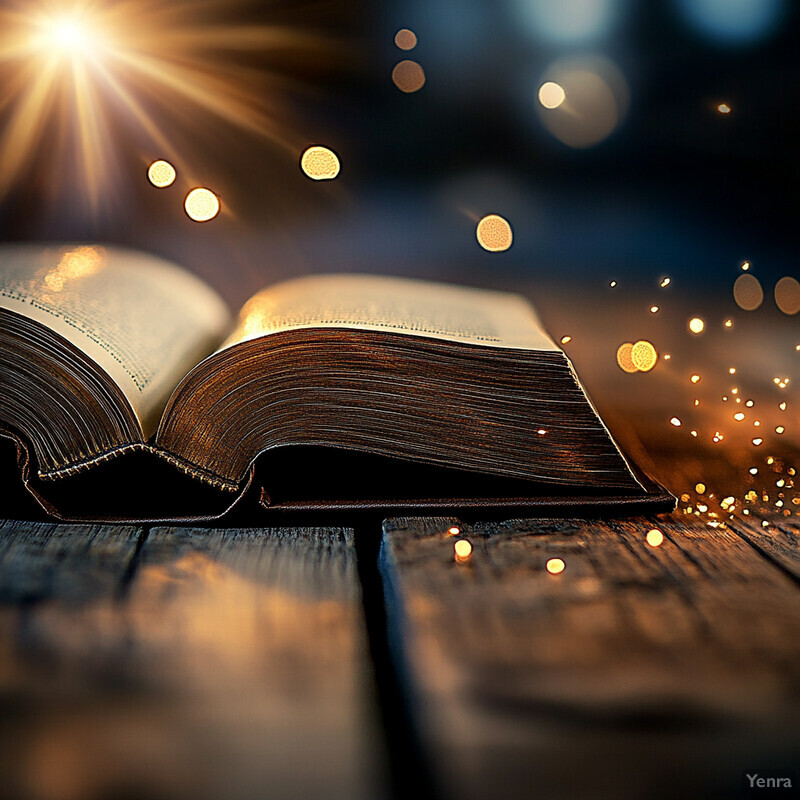
Long documents, dense reports, and extensive policy manuals can overwhelm employees trying to quickly extract essential points. AI-driven summarization tools use NLP algorithms to identify key sentences, concepts, and themes within text, then present a concise summary that captures the core message. This capability saves time by helping employees rapidly understand the content’s purpose before deciding whether to delve deeper. Instead of reading multiple lengthy documents in full, team members can rely on these automated summaries to guide their focus. As knowledge bases expand, summarization ensures that critical information remains accessible and digestible, streamlining workflow and enabling faster decision-making.
6. Enhanced Information Governance and Compliance
AI identifies sensitive information, flags compliance issues, and assists with policy enforcement, ensuring that enterprise knowledge adheres to regulatory requirements and internal governance standards.
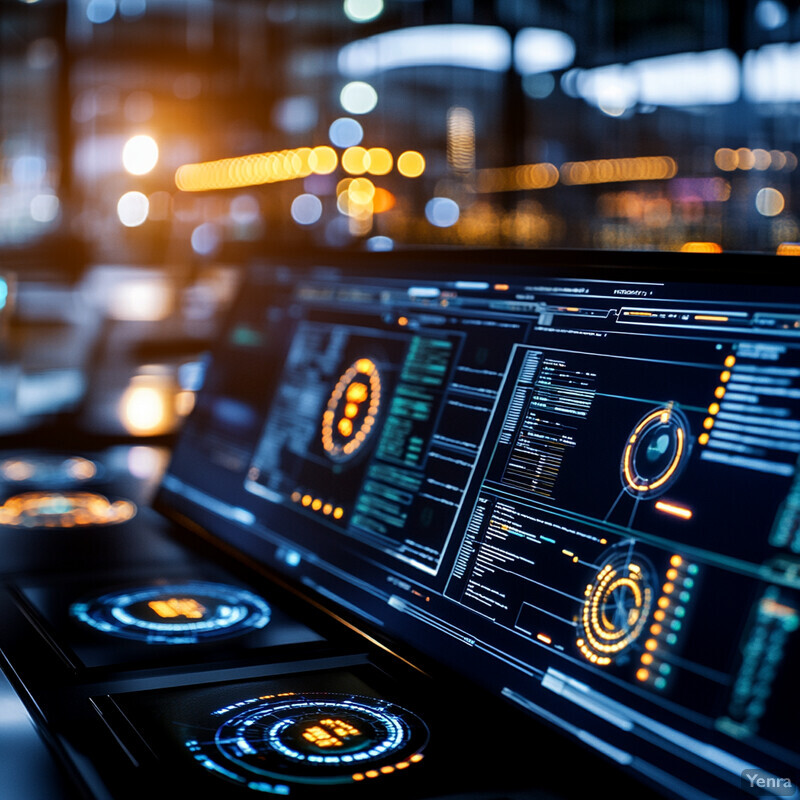
Organizations must adhere to strict regulatory requirements and internal policies governing data handling, document retention, and privacy. AI aids in this endeavor by automatically detecting sensitive information, such as personally identifiable data or intellectual property, and ensuring it is stored, accessed, and shared according to compliance rules. By identifying irregularities, AI-driven systems can flag or quarantine non-compliant content before it causes legal or reputational damage. Furthermore, these systems continuously monitor document usage, updates, and versioning, making auditing and reporting more efficient. This proactive approach to governance and compliance reduces legal risk, maintains public trust, and safeguards an organization’s reputation.
7. Real-time Language Translation and Localization
AI-driven translation tools can instantly convert content into multiple languages, bridging linguistic gaps and making enterprise knowledge globally accessible without costly and time-consuming human translation.
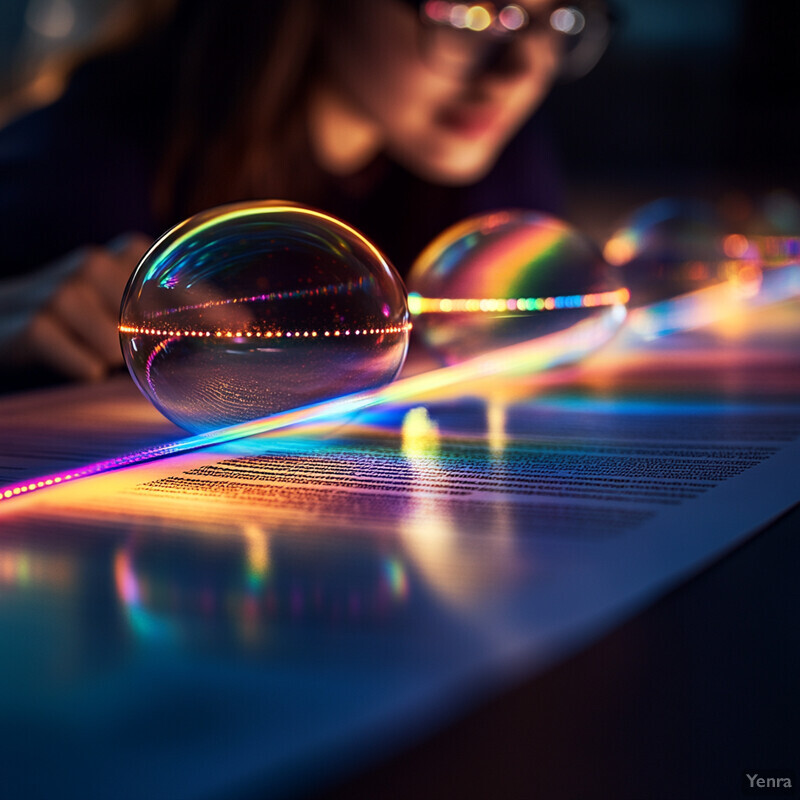
As global enterprises operate across multiple regions and languages, AI-powered translation tools facilitate seamless communication and knowledge sharing. Instead of relying solely on human translators or static language repositories, machine translation systems convert documents, intranet pages, and other knowledge assets into diverse languages in real-time. Employees across geographically distributed offices can access the same critical information, ensuring that knowledge remains inclusive and accessible. While human linguistic experts may fine-tune certain content, the immediate availability of translated material accelerates collaboration, speeds up projects, and enables a truly global knowledge environment. Over time, the AI engines improve their linguistic accuracy through user feedback, leading to even more reliable translations.
8. Expert Identification and Expertise Mapping
Machine learning algorithms analyze organizational communication and project involvement to identify subject matter experts, fostering knowledge sharing by connecting employees with the right internal authorities.
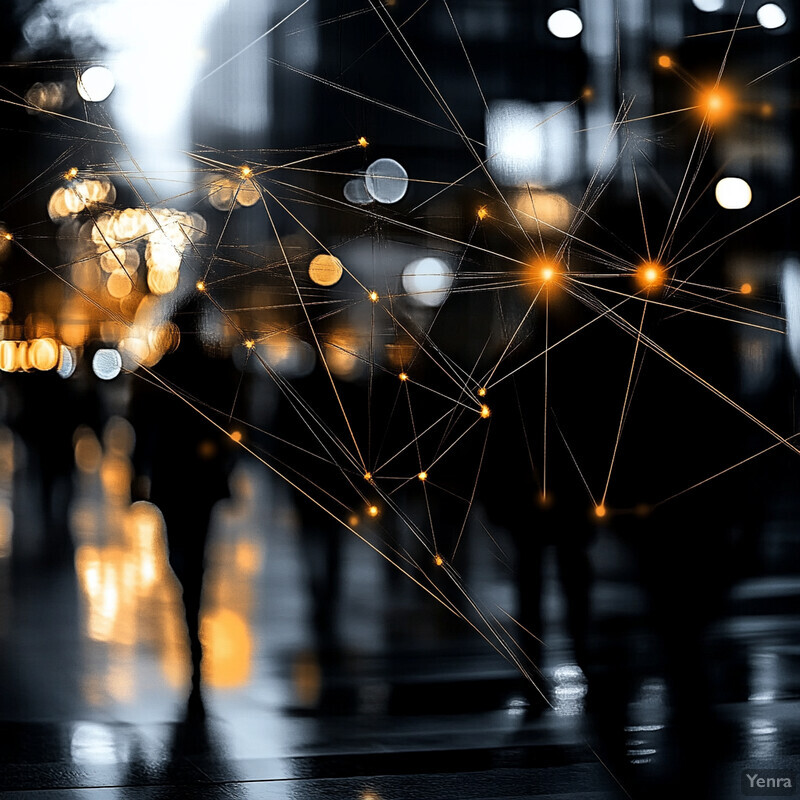
Within large organizations, locating the right expert for a project, consultation, or knowledge gap can be challenging. AI-driven analytics can examine communication patterns, contribution histories, project involvement, and skills endorsements to construct an expertise map of the enterprise. This map helps employees find individuals who have deep subject matter knowledge, relevant experience, or particular credentials. As a result, teams form faster, leverage institutional wisdom more effectively, and reduce reliance on external consultants. By breaking down silos and connecting colleagues who might never have otherwise collaborated, expertise mapping fosters a more vibrant and interconnected organizational culture.
9. Automated Knowledge Lifecycle Management
AI helps monitor content usage and relevance. It can archive outdated information, highlight gaps in available knowledge, and surface newly emerging topics, ensuring that knowledge bases remain current and useful.
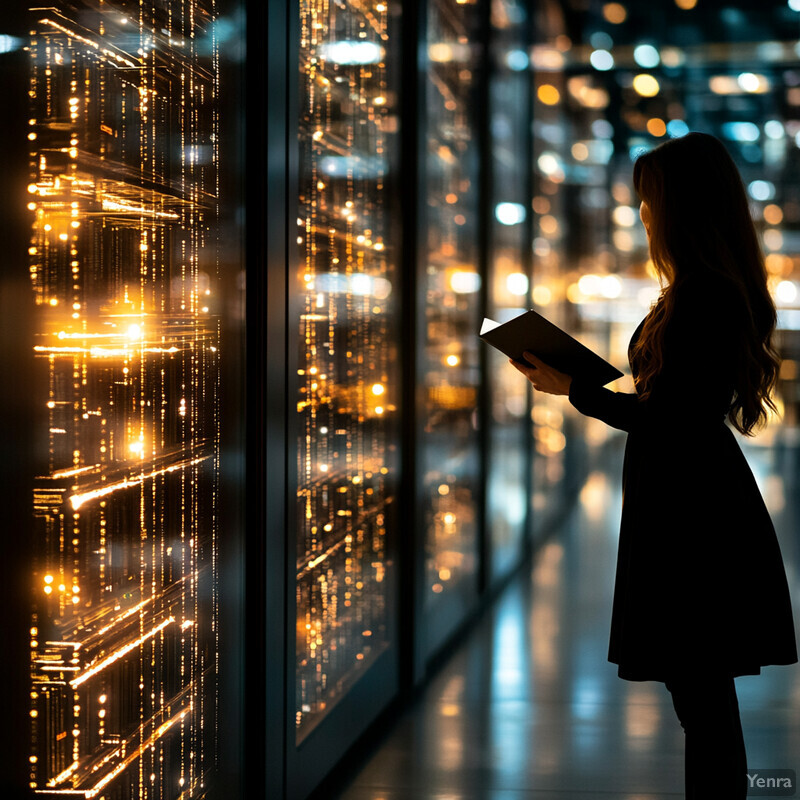
Enterprise content constantly evolves, with new information created as older materials become obsolete. AI automates the lifecycle management of knowledge assets by monitoring usage patterns, last-accessed dates, and relevance to current business operations. When content is no longer used or becomes outdated, AI can archive it, ensuring the repository remains fresh and focused. Simultaneously, the system can highlight knowledge gaps where new or updated content is needed, prompting content creators to address these deficiencies. This continuous renewal process keeps knowledge bases lean, current, and directly aligned with the organization’s strategic priorities.
10. Conversational Interfaces and Virtual Assistants
AI chatbots and voice assistants make EKM systems more approachable, allowing employees to retrieve information through natural language queries and streamline repetitive knowledge discovery tasks.
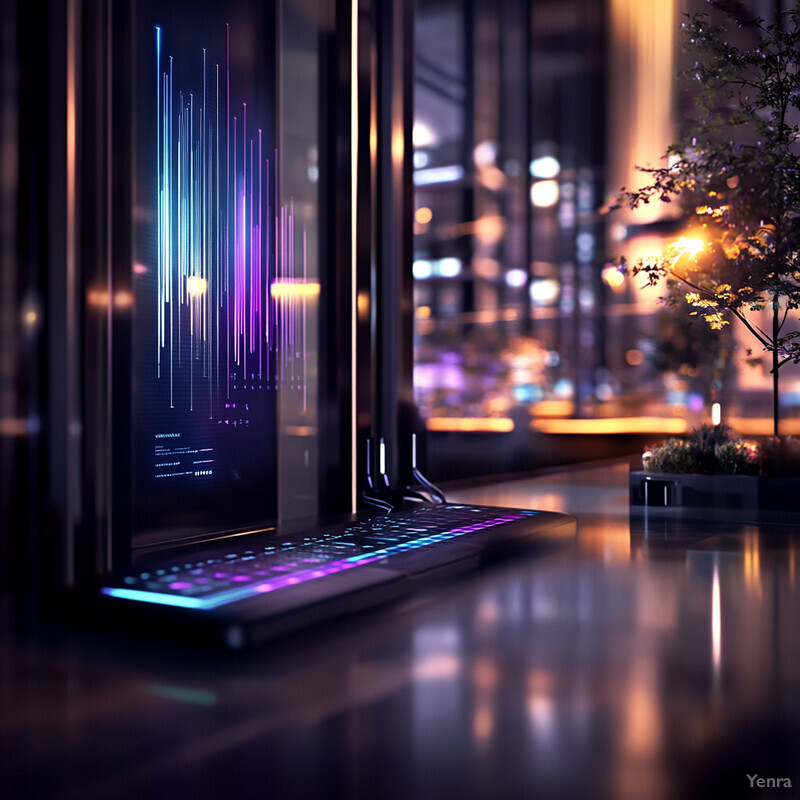
AI-powered chatbots and virtual assistants enable employees to interact with knowledge systems using natural language queries, just as they would when speaking to a human colleague. This conversational approach reduces the learning curve associated with complex search interfaces or navigating complicated directory structures. Users can simply ask, “Where can I find the latest sales performance report?” and the assistant quickly locates and presents the requested data. These tools can also integrate with other enterprise systems, completing tasks like scheduling meetings, ordering supplies, or updating CRM entries. By streamlining information retrieval and task execution, conversational interfaces enhance user productivity and overall satisfaction.
11. Predictive Knowledge Needs
By analyzing historical usage patterns, AI can predict what information a team or user will need in the future, proactively surfacing relevant documents, guidelines, or best practices before they are explicitly requested.
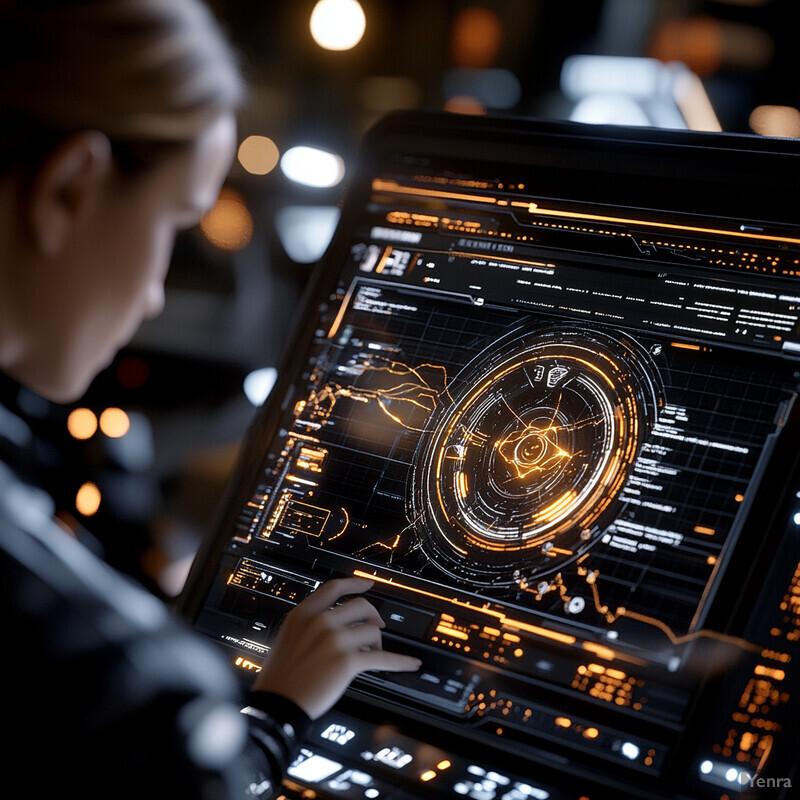
AI analytics can identify trends in user behavior, department queries, and ongoing projects to predict which information employees will need next. For example, if a development team frequently references certain compliance guidelines before product releases, the system can anticipate those needs and surface the relevant documents proactively. This predictive approach reduces search time and cognitive load, allowing employees to focus on higher-value tasks. Over time, the system refines its recommendations, becoming more attuned to workflow rhythms and seasonal patterns. Ultimately, anticipating knowledge needs drives efficiency, ensures timely decision-making, and keeps teams operating at peak productivity.
12. Quality Assurance and Consistency Checks
AI-driven quality checks ensure that enterprise documentation maintains consistent terminology, formatting, and style, improving the overall clarity and reliability of stored knowledge.
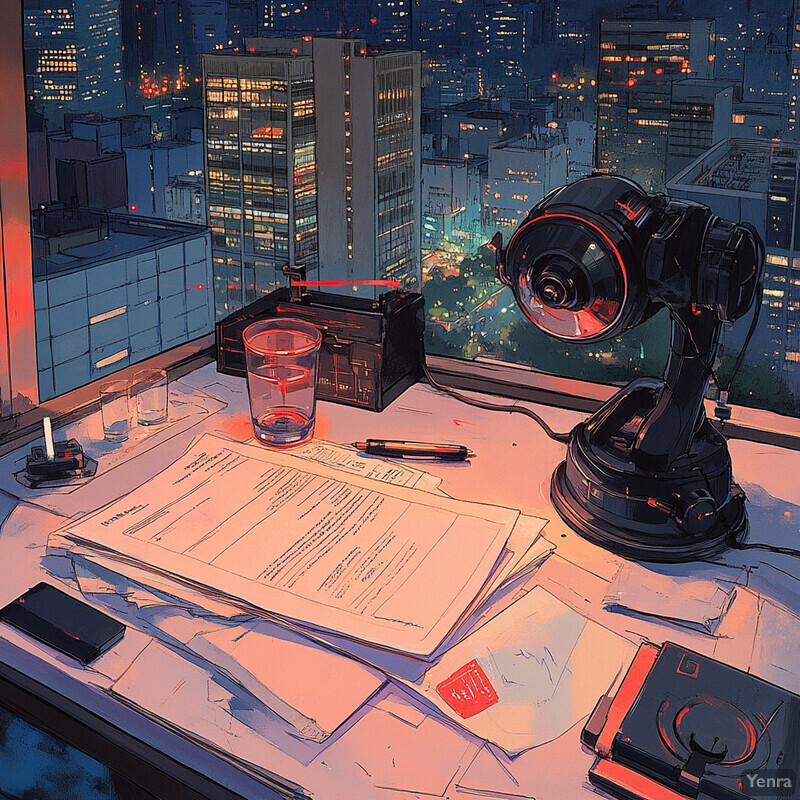
Maintaining consistent language, formatting, and style across enterprise content is a challenge, especially in large organizations where multiple authors contribute. AI-based quality assurance tools scan documents for discrepancies, outdated terminology, incomplete references, and conflicting information. They alert content creators to these inconsistencies and recommend corrections, ensuring that everyone accesses accurate and coherent information. By standardizing how knowledge is presented, the enterprise delivers a more professional image and reduces misunderstandings caused by inconsistent or erroneous content. In the long run, this improves overall trust in the knowledge repository and ensures that materials remain a reliable source of truth.
13. Intelligent Knowledge Ingestion from Unstructured Data
AI techniques, such as computer vision and NLP, extract structured insights from images, scanned documents, and audio/video files, enriching the knowledge repository with previously untapped information.
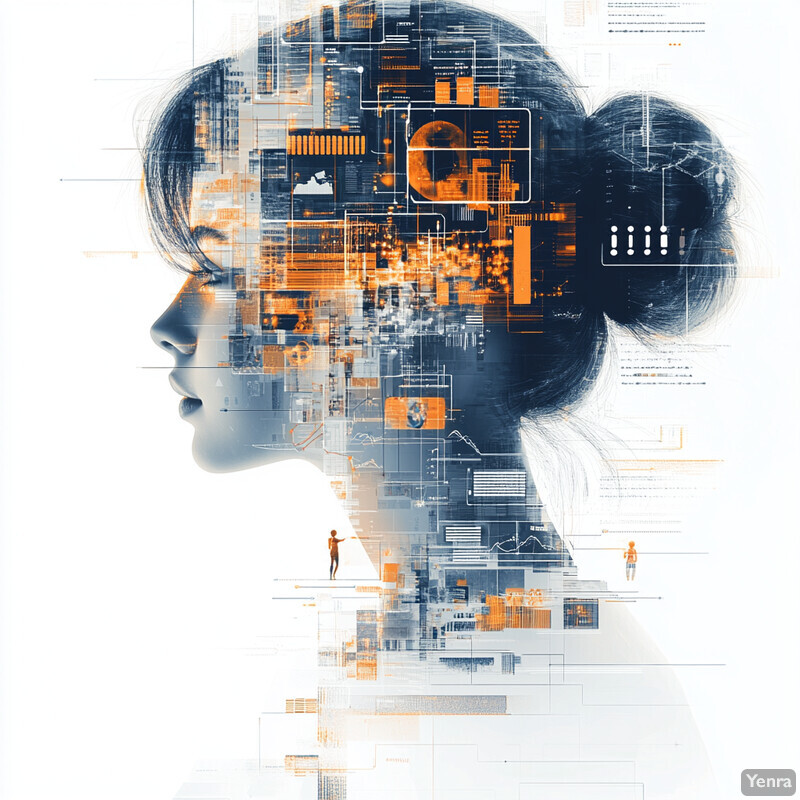
Enterprises accumulate vast amounts of unstructured data—images, PDFs, scanned documents, audio files, and videos—that traditionally remain underutilized. AI-driven extraction techniques, such as optical character recognition (OCR) for text in images or speech-to-text for audio, convert these assets into machine-readable formats. Once processed, natural language understanding models can identify key topics, sentiments, and entities, enriching the knowledge base with previously untapped insights. As this unstructured data is integrated, it augments the overall completeness of enterprise knowledge. Employees can then search, analyze, and connect this new data with existing information, providing a more comprehensive understanding of organizational operations and opportunities.
14. Enhanced Content Personalization
Machine learning tailors the discovery process based on user roles, skill levels, departments, and past behavior, ensuring that employees only see the most relevant enterprise knowledge for their needs.
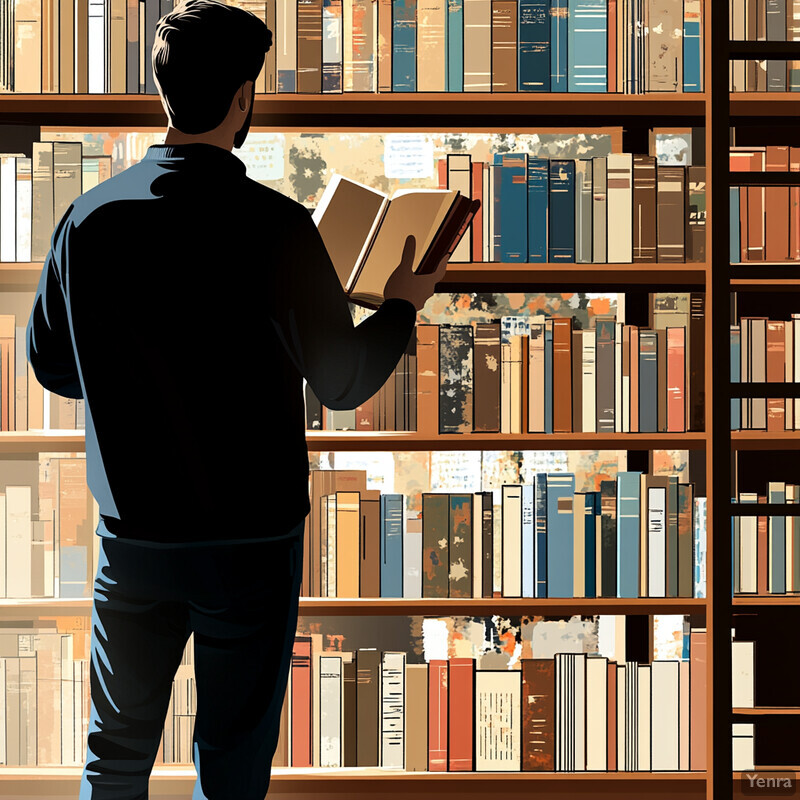
Different roles, departments, and regions within a company have distinct information needs. AI-powered personalization ensures that content delivered to each user is tailored to their responsibilities, past behavior, skill level, and real-time context. A marketing manager might see the latest product briefs and brand guidelines, while a financial analyst is shown updated revenue reports and risk assessments. This customization streamlines the user’s interaction with the knowledge system, improving engagement and reducing information overload. Ultimately, enhanced personalization ensures that each employee quickly accesses the most pertinent data, making them more effective and confident in their roles.
15. Adaptive Learning and Training Programs
AI-driven learning systems adjust training content in real-time based on employee performance and knowledge gaps, making corporate education more efficient and personalized.
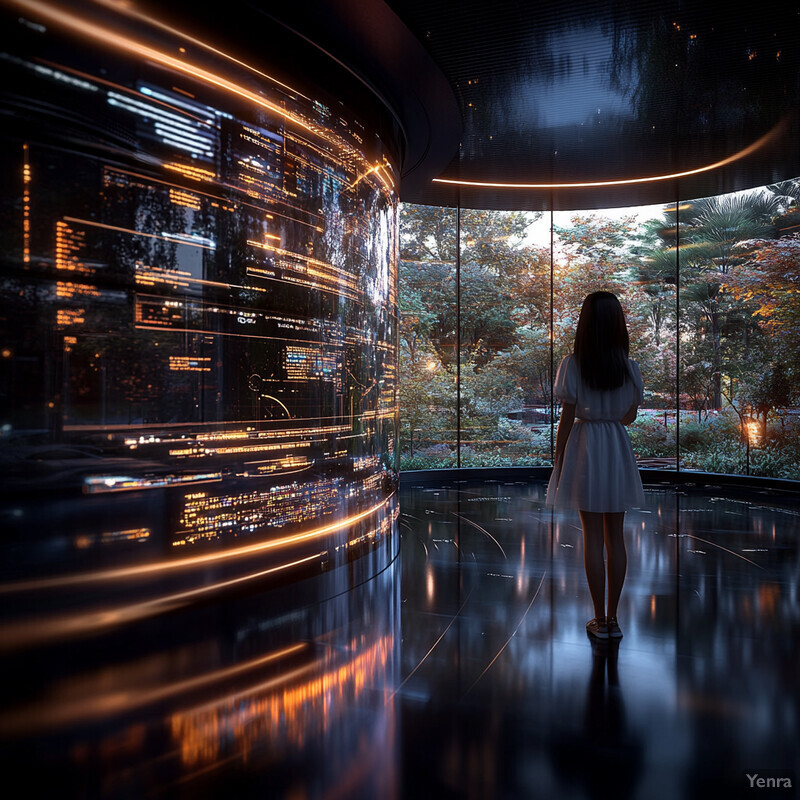
Corporate training and onboarding materials often fail to meet learners’ varying needs and learning styles. AI adapts educational content in real-time based on the learner’s progress, areas of confusion, and performance metrics. For instance, if a trainee struggles with a particular concept, the system can present alternative explanations, interactive examples, or additional exercises. Over time, this personalized approach to learning enables employees to develop competencies more efficiently, improving overall workforce skill levels. By continuously adjusting the difficulty and pacing of training content, adaptive learning systems ensure a more productive and satisfying experience for employees at all stages of their careers.
16. Cognitive Search for FAQs and Troubleshooting Guides
AI-driven systems help generate dynamic, context-aware FAQs and troubleshooting guides that adapt as new products, processes, and policies come online.
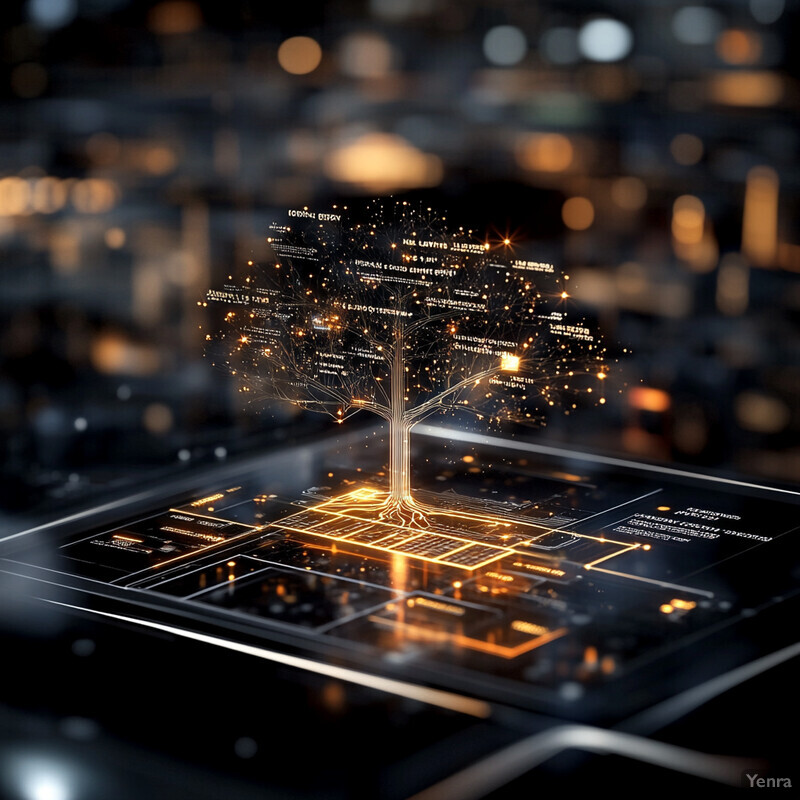
As products and processes evolve, maintaining up-to-date FAQs and troubleshooting guides is essential. AI-powered cognitive search systems use contextual understanding and semantic analysis to generate dynamic help content. When users type a question, the system matches their query with the most relevant solutions, even if they don’t phrase it exactly as documented. By analyzing historical queries, it can also identify common issues and proactively update FAQs or highlight necessary training materials. This responsive approach transforms static repositories into living resources, reducing customer support load, improving internal help desk efficiency, and empowering employees and customers to solve problems independently.
17. Automated Knowledge Gap Analysis
By examining usage patterns and user feedback, AI identifies areas where information is incomplete or unclear, prompting the creation or improvement of content to fill those gaps.
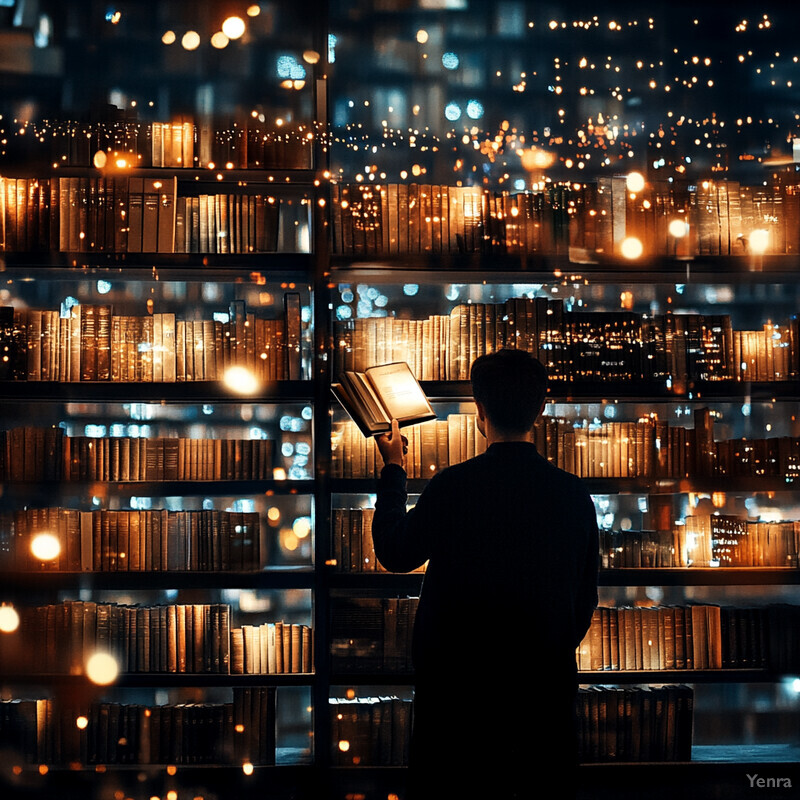
Even comprehensive knowledge bases may have blind spots. AI-driven analytics evaluate user search queries, access logs, and feedback to pinpoint subjects that lack sufficient documentation or clarity. Identifying these gaps encourages content creators to produce new articles, guides, or training materials that address unmet needs. As the system continually monitors activity, it ensures the knowledge base evolves alongside the organization’s changing priorities and challenges. Over time, this iterative enhancement of content availability reduces frustration, streamlines project execution, and supports better-informed decision-making across the enterprise.
18. Metadata-Enriched Document Retrieval
Deep-learning-based classifiers add rich metadata and context to stored documents, making it simpler to locate specific pieces of information—even when the original request is vague.
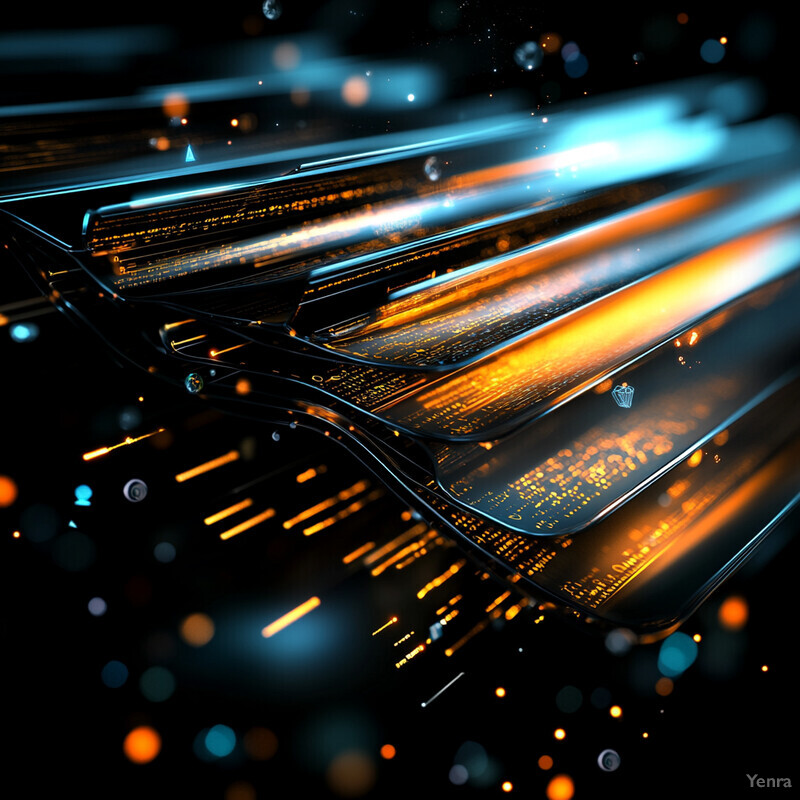
Generic file names and sparse metadata often complicate document retrieval. AI solutions enhance metadata by extracting meaningful properties from content—such as topics, authors, creation dates, and internal references. This enrichment provides users with multiple pathways to find the right document: by concept, associated project, relevant department, or related terms. Rich metadata transforms the enterprise repository into a flexible, multidimensional search environment rather than a flat file directory. Employees can discover content more intuitively, resulting in less wasted time, reduced duplication, and more efficient knowledge workflows.
19. Intelligent Content Lifecycle Insights
Through analytics dashboards enhanced by AI, enterprises gain insights into how knowledge evolves over time, which documents are most frequently consulted, and where to invest in new knowledge creation.
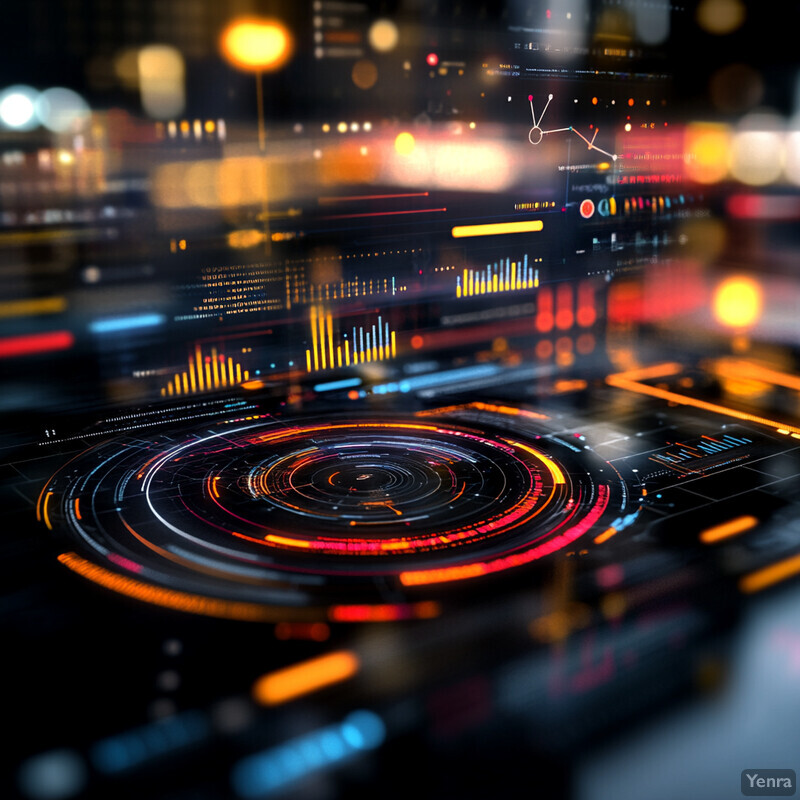
By analyzing usage metrics, version histories, and user feedback, AI offers insights into how enterprise knowledge changes over time. Leaders can see which documents are most frequently consulted, which pieces of content fail to engage users, and which sections are consistently flagged as outdated or unclear. These insights guide strategic decisions about what types of content to invest in, what to retire, and how to improve the quality of existing materials. Armed with this information, organizations can focus on continuous improvement, ensuring their knowledge base aligns with evolving business objectives and user expectations. This data-driven approach to content management enhances overall knowledge relevance and efficiency.
20. Scalable and Adaptive Knowledge Repositories
As organizations grow and data volumes increase, AI ensures that EKM systems remain scalable, adaptive, and responsive, handling ever-expanding content repositories without degrading user experience.
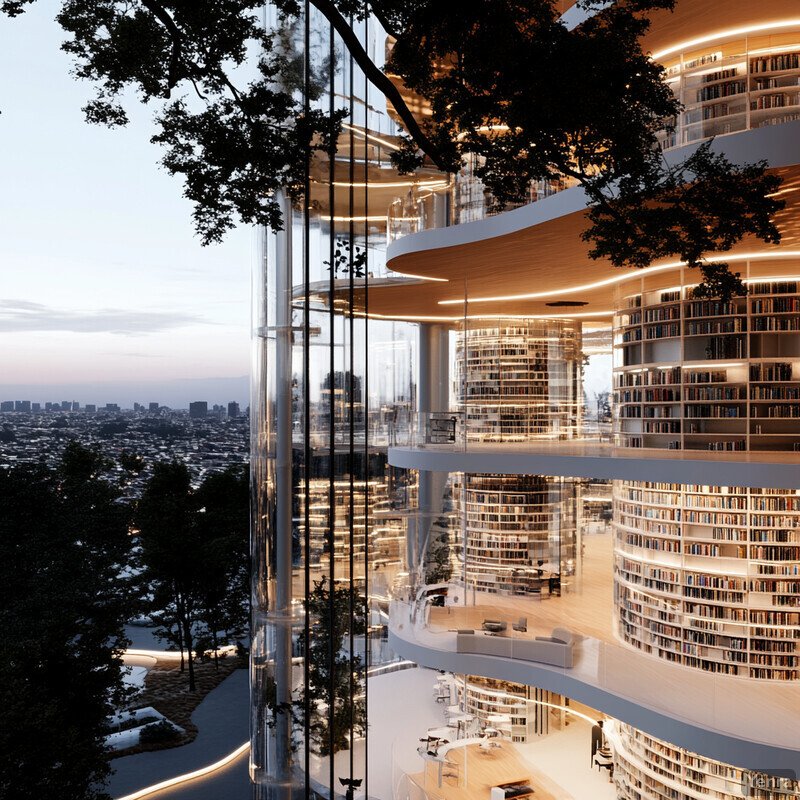
As organizations grow and accumulate massive volumes of data, maintaining a responsive, user-friendly knowledge management system becomes challenging. AI-driven EKM solutions scale effortlessly, handling increasing loads of content and users without performance degradation. As new business units form, products launch, or markets expand, the AI adapts classification models, search algorithms, and recommendation engines to reflect these changes. This ensures that knowledge delivery remains efficient and relevant regardless of organizational complexity or data size. By dynamically responding to growth and transformation, AI-enabled repositories protect the value of enterprise knowledge over the long term, ensuring they remain powerful resources well into the future.