1. Automated Chart Review and Coding
AI-driven natural language processing (NLP) tools can automatically review clinician notes and assign the correct medical codes, reducing errors and administrative burden.
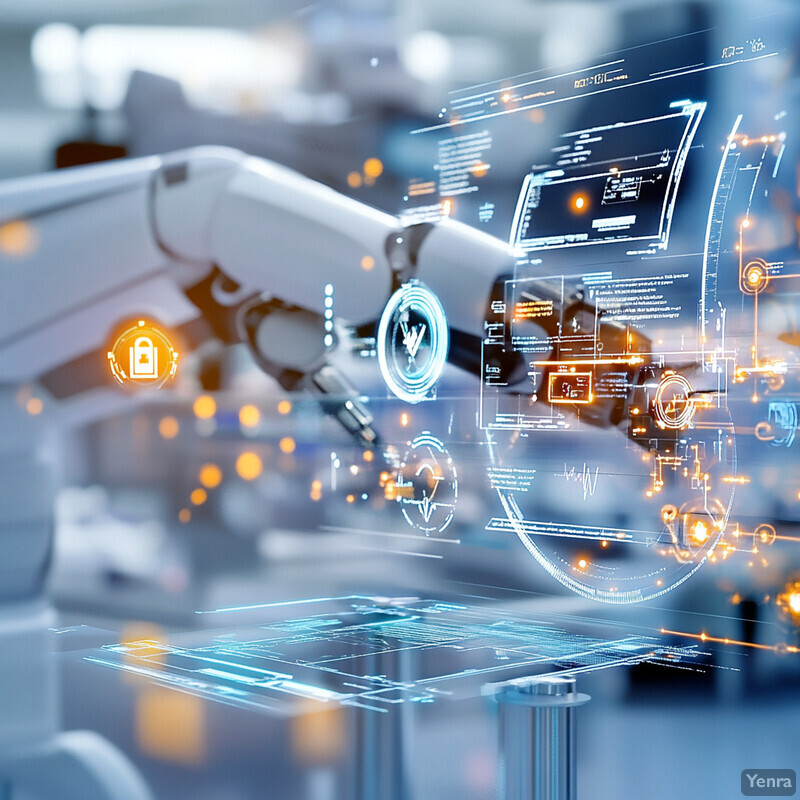
AI-driven natural language processing (NLP) and machine learning tools can dramatically reduce the administrative time and errors associated with medical coding and chart reviews. Traditionally, medical coders and billers must carefully read through physician notes, discharge summaries, and procedure reports to assign the most accurate billing codes. By deploying AI algorithms, these notes can be rapidly translated into structured data with suggested coding recommendations. This process not only helps prevent human oversight but also speeds up the revenue cycle. As a result, healthcare organizations can experience more consistent coding accuracy, improved reimbursement rates, and less burden on clinicians and administrative staff.
2. Predictive Analytics for Disease Progression
Machine learning models can analyze longitudinal EHR data to predict how a patient’s condition might evolve, helping clinicians intervene earlier.
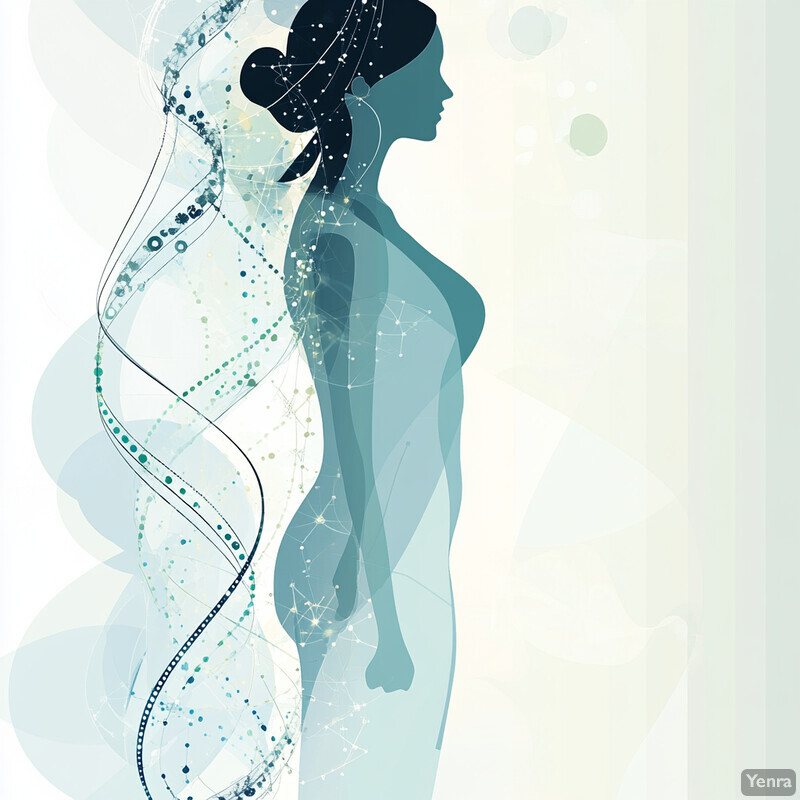
Machine learning models can analyze large volumes of longitudinal Electronic Health Record (EHR) data to identify patterns and risk factors associated with disease progression. By examining trends in laboratory values, imaging results, medication histories, and clinical notes, AI tools can predict how a patient's illness might develop over time. For instance, predictive models can indicate which diabetic patients are more likely to develop complications, or which cardiovascular patients may benefit from early interventions. This foresight empowers clinicians to act proactively, adjusting treatment plans, scheduling more frequent follow-ups, and offering preventive therapies that can improve patient outcomes and reduce long-term healthcare costs.
3. Clinical Decision Support
Intelligent algorithms can scan a patient’s entire EHR history and highlight relevant information, supporting clinicians with evidence-based recommendations at the point of care.
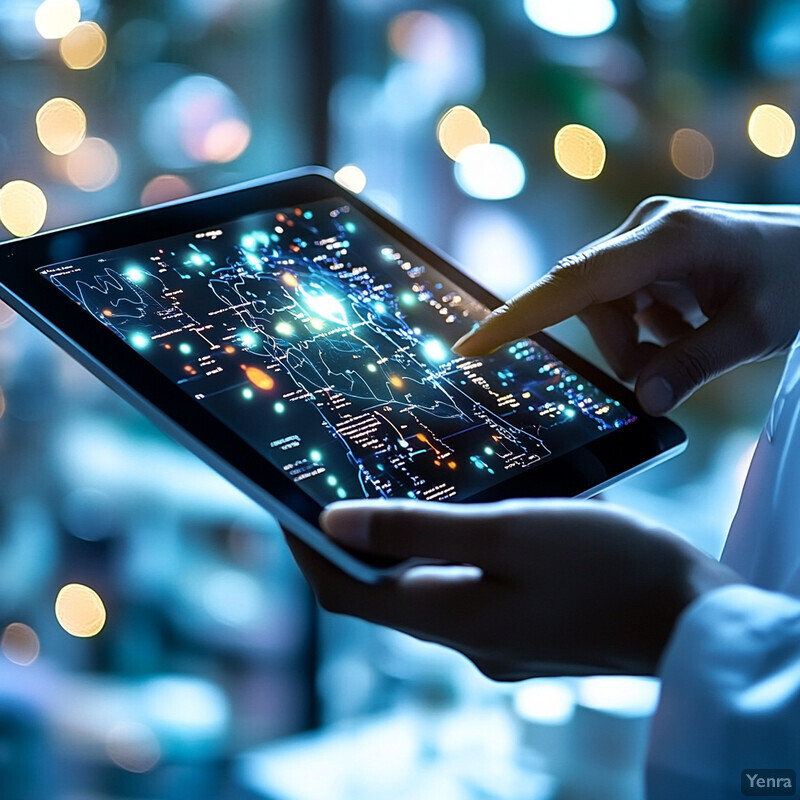
Integrating AI-driven insights into the clinician’s workflow can bolster clinical decision support (CDS) tools within EHRs. When a physician is treating a patient with multiple comorbidities, it can be difficult to recall all relevant practice guidelines or emerging research. AI-based CDS systems can review the patient’s entire medical history, highlight critical risk factors, and recommend evidence-based interventions or diagnostic steps in real-time. This helps ensure patients receive care aligned with the latest clinical guidelines and reduces the cognitive load on providers. Over time, better clinical decision support can lead to more standardized care, fewer medical errors, and improved patient outcomes.
4. Population Health Management
By analyzing large-scale EHR data sets, AI can identify population-level trends, stratify patients by risk, and inform public health strategies.
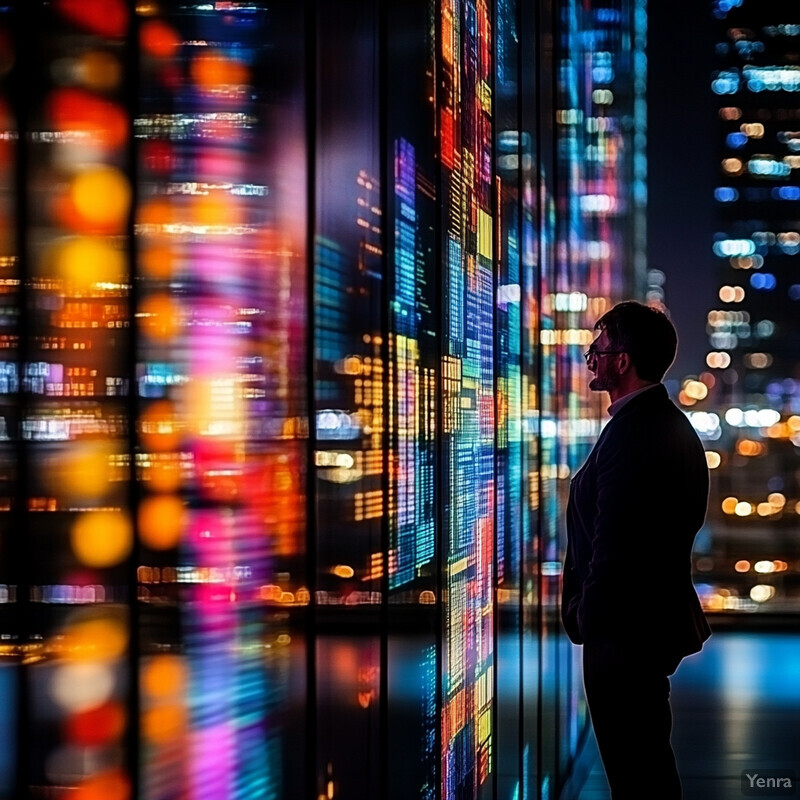
AI-assisted analysis of large-scale EHR data sets can identify trends and patterns that are not easily visible at the individual level. By aggregating data from thousands or even millions of patients, machine learning algorithms can group populations based on disease risk, social determinants of health, or healthcare utilization patterns. This allows healthcare organizations and public health agencies to design targeted interventions, allocate resources more efficiently, and monitor the effectiveness of population-level strategies. For example, identifying communities at higher risk for certain chronic conditions can guide where to focus preventive screening programs or community health initiatives, ultimately improving health outcomes on a broad scale.
5. Risk Stratification and Proactive Care
Predictive models can assess patient risk for hospital readmission, chronic disease onset, or complications, enabling targeted preventive measures.
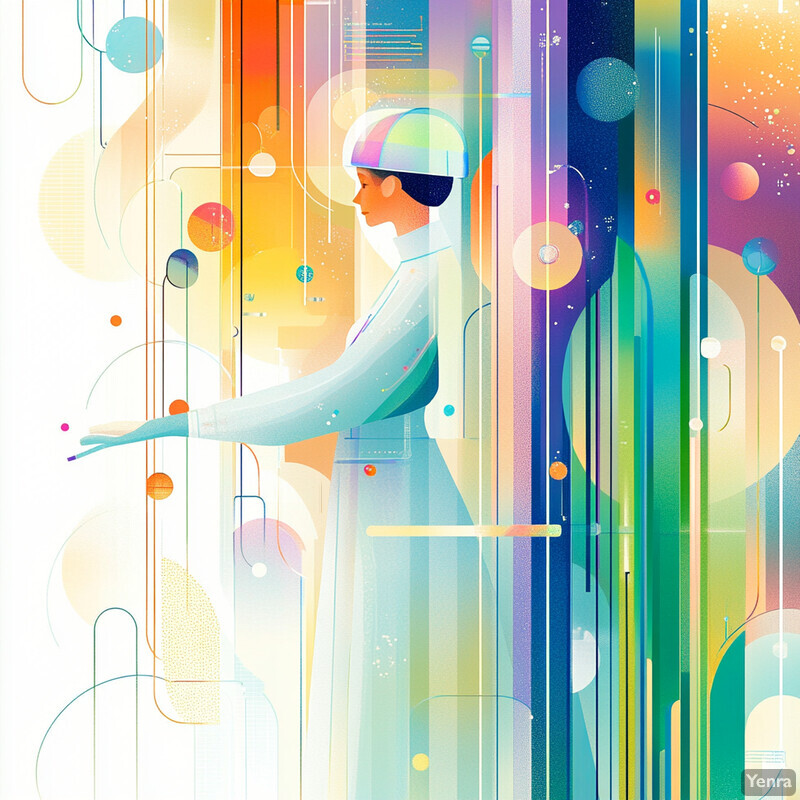
AI models excel at risk stratification—sorting patients into tiers based on their likelihood of experiencing adverse events, hospital readmissions, or disease complications. By considering a multitude of variables such as past hospitalization rates, medication adherence, and socioeconomic factors, these predictive algorithms can help care teams identify patients who may need closer monitoring or earlier intervention. With this insight, healthcare systems can proactively reach out to patients with early warning signs, adjust treatment plans, or provide additional educational resources. This shift from a reactive to a proactive model of care reduces unnecessary hospitalizations, lowers costs, and improves patient satisfaction.
6. Data Cleaning and Normalization
AI tools can standardize and reconcile disparate data formats and terminologies across multiple EHR systems, enhancing data quality and interoperability.
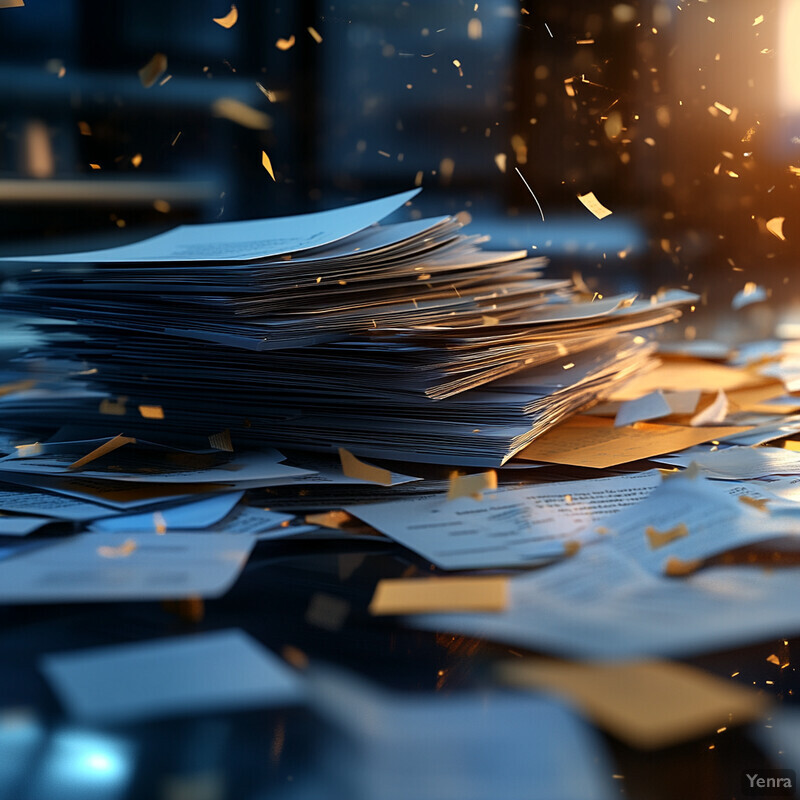
EHR data often come in heterogeneous formats, with varying codes, terminologies, and documentation styles across different healthcare systems. AI-driven data cleaning and normalization tools use NLP and ontology mapping to standardize this information, ensuring that data from multiple sources can be accurately integrated and compared. By automatically reconciling discrepancies in units, medical terms, and coding systems, these tools improve the reliability and utility of the data. As a result, researchers, clinicians, and administrators gain access to cleaner, more interoperable data sets that can fuel meaningful analytics, quality improvement initiatives, and system-wide learning.
7. Natural Language Processing of Unstructured Text
NLP can convert physician notes, lab reports, and imaging results into structured data, making them easier to search and analyze.
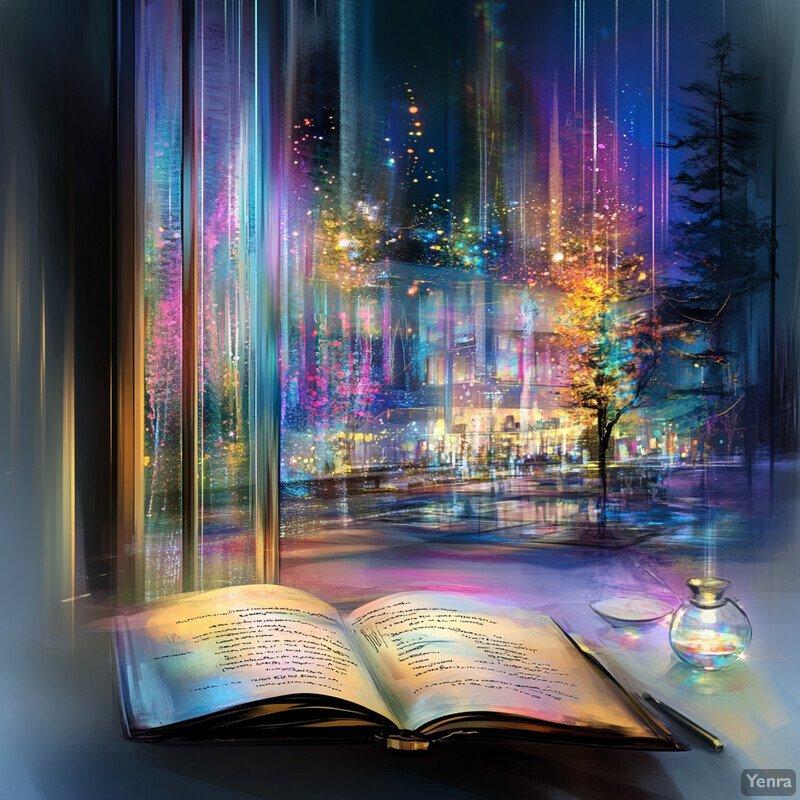
A significant portion of valuable patient information—such as descriptions of symptoms, physician impressions, and narrative diagnostic reports—is captured in unstructured text fields. NLP techniques transform these free-text entries into structured data elements that can be queried, analyzed, and integrated with other patient information. This unlocks insights previously hidden in text-based notes, enabling more holistic patient profiles. For example, NLP can detect mentions of certain lifestyle behaviors, social conditions, or early symptoms that would otherwise remain buried in narrative documents. By doing so, clinicians and researchers gain a more comprehensive understanding of patients and conditions, improving both clinical decision-making and population-level analytics.
8. Clinical Workflow Optimization
Intelligent systems can identify inefficiencies and bottlenecks in clinical workflows, suggesting improvements for scheduling, staffing, and patient throughput.
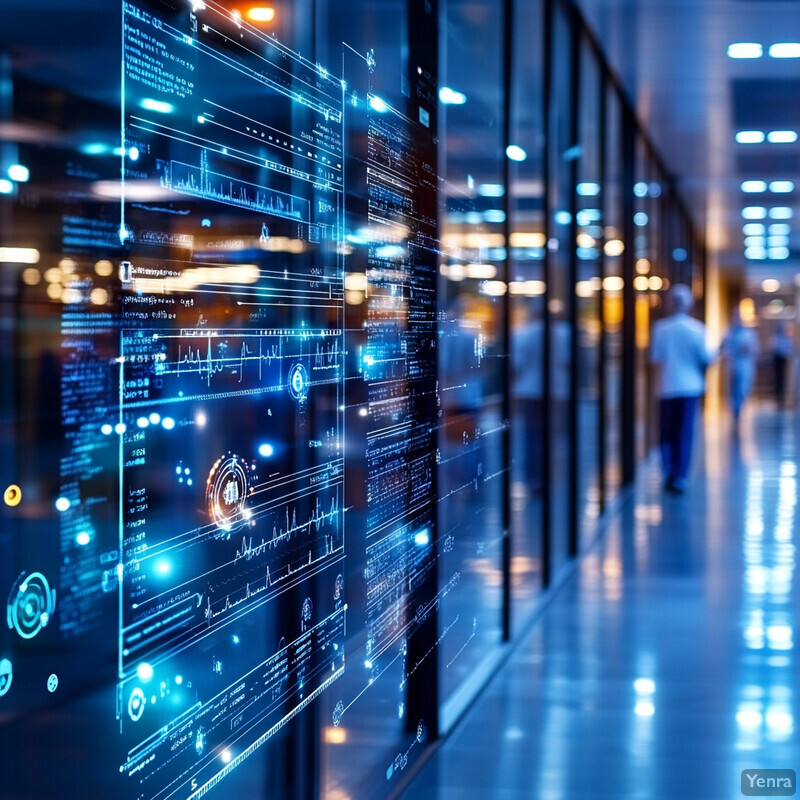
By analyzing patterns in patient flow, resource utilization, and staff scheduling, AI can identify inefficiencies in clinical workflows. These algorithms may uncover that certain types of appointments consistently run over time, that certain diagnostic tests are frequently delayed due to equipment availability, or that patient wait times spike during specific hours. With these insights, administrators can make targeted improvements—such as adjusting staffing levels, reordering how appointments are scheduled, or adopting new triage protocols. Over time, these changes can help reduce patient wait times, increase staff productivity, enhance care quality, and ultimately lead to improved patient and clinician satisfaction.
9. Quality and Outcomes Measurement
AI can monitor treatment protocols, adherence to guidelines, and patient outcomes, offering insights on how to improve quality metrics and reduce variation in care.
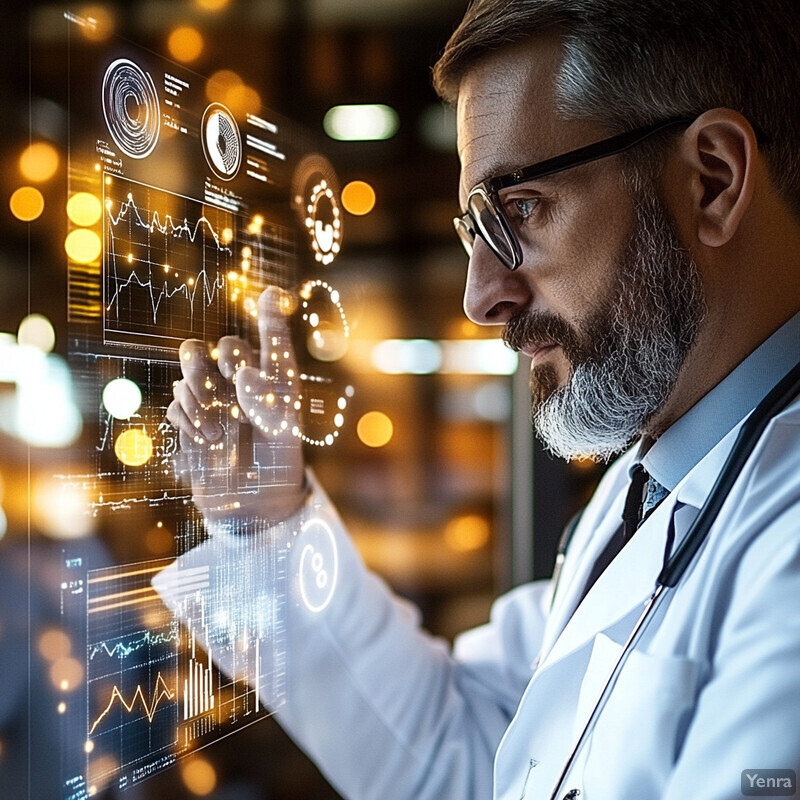
AI-driven analytics can help healthcare organizations monitor adherence to clinical guidelines, measure patient outcomes, and assess the quality of care delivery. By continuously scanning EHR data, these tools ensure that best practices are followed, identify where protocols are not being met, and highlight areas for improvement. For example, a hospital might use AI to track how frequently its providers are following recommended care pathways for managing sepsis. The data can then guide educational initiatives, policy changes, or targeted interventions to improve compliance. Over time, these efforts translate into better patient outcomes, more consistent care, and enhanced performance on key quality metrics.
10. Early Warning Systems for Patient Deterioration
Predictive models can detect subtle changes in vital signs or lab results, alerting clinicians to patients who may be at risk for rapid decline.
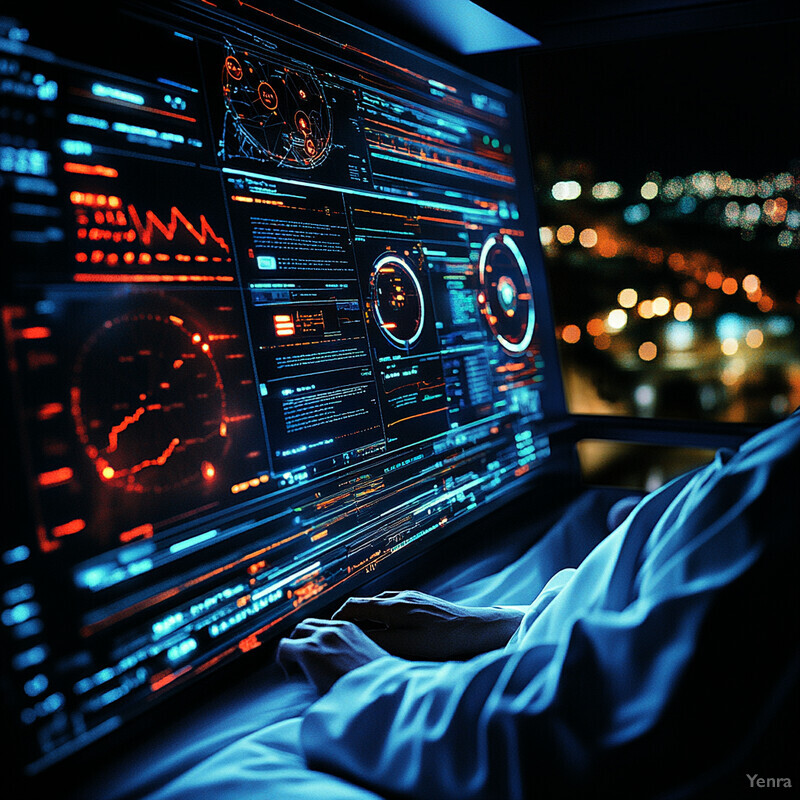
Hospitalized patients can experience sudden clinical deteriorations that are challenging to predict. AI-based early warning systems continuously analyze vital signs, lab results, nursing notes, and other EHR inputs to detect subtle patterns that may signal impending instability. For instance, a combination of slightly dropping blood pressure, rising heart rate, and specific lab abnormalities may alert the system to a risk of sepsis. This early notification allows clinicians to intervene sooner, potentially preventing serious complications. By catching these signals early, healthcare teams improve patient safety, reduce the likelihood of adverse events, and promote more timely and effective interventions.
11. Personalized Treatment Recommendations
Advanced analytics can integrate genetic, clinical, and social determinants of health data to recommend tailored interventions and therapies.
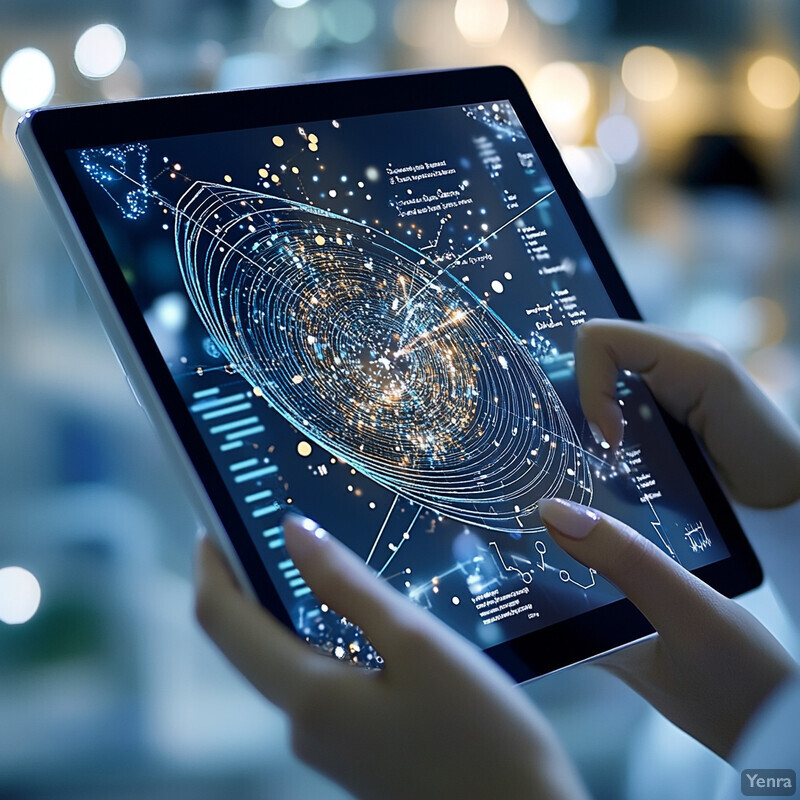
AI can integrate clinical data with genetic information, imaging results, social determinants of health, and patient preferences to formulate individualized treatment plans. Instead of applying a one-size-fits-all approach, healthcare providers can use AI-driven insights to determine which therapies are most likely to be effective for a particular patient. For instance, certain medications or treatment strategies may work well for one subgroup of patients but not another. By leveraging advanced analytics, clinicians can offer more personalized care, ultimately improving treatment outcomes, reducing side effects, and enhancing patient satisfaction.
12. Drug Safety and Pharmacovigilance
Machine learning techniques can detect adverse drug events by analyzing medication histories, lab values, and clinical narratives, improving patient safety.
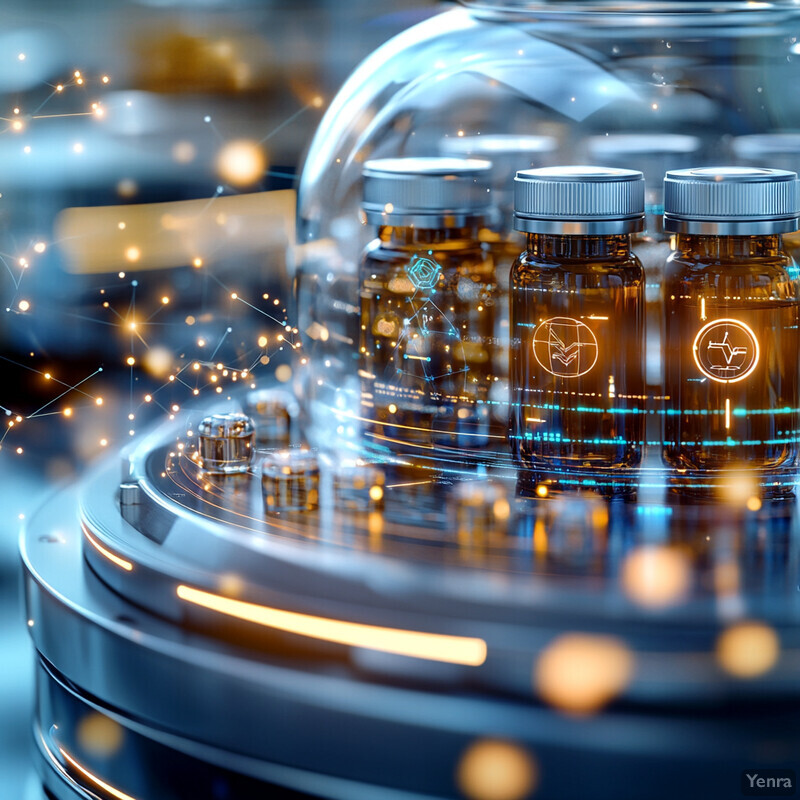
Adverse drug events (ADEs) are a significant source of patient harm and healthcare cost. AI can analyze medication lists, laboratory values, and clinician notes to detect potential ADEs before they manifest into critical issues. These tools can also identify patients most at risk for drug-drug interactions or medication non-adherence. By proactively flagging high-risk patients or suspicious prescribing patterns, AI enhances pharmacovigilance efforts, reduces medication errors, and safeguards patient well-being. This leads to improved patient safety profiles, fewer hospital readmissions related to medication problems, and better overall outcomes.
13. Clinical Trial Recruitment
AI can sift through EHRs to identify patients meeting trial criteria, accelerating enrollment and ensuring better trial matching.
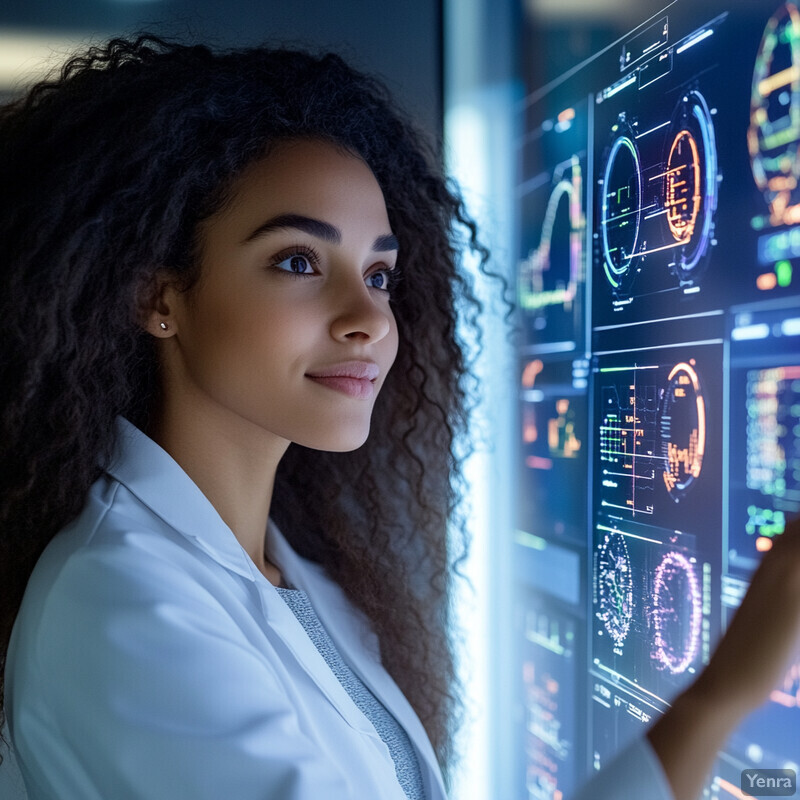
Identifying and enrolling eligible patients into clinical trials is a time-consuming and often inefficient process. AI solutions can swiftly scan EHR data, correlating patient characteristics, diagnoses, lab results, and demographic factors against clinical trial eligibility criteria. This streamlined approach helps research coordinators and clinicians find suitable candidates faster, increasing the likelihood that trials are completed on schedule. Patients also benefit, as it expands their access to cutting-edge therapies and interventions. Improved trial recruitment accelerates the development of new treatments, ultimately improving the quality and availability of medical advances.
14. Cohort Selection for Research
Researchers can use AI to quickly assemble patient cohorts that match specific inclusion/exclusion criteria, enhancing the speed and accuracy of studies.
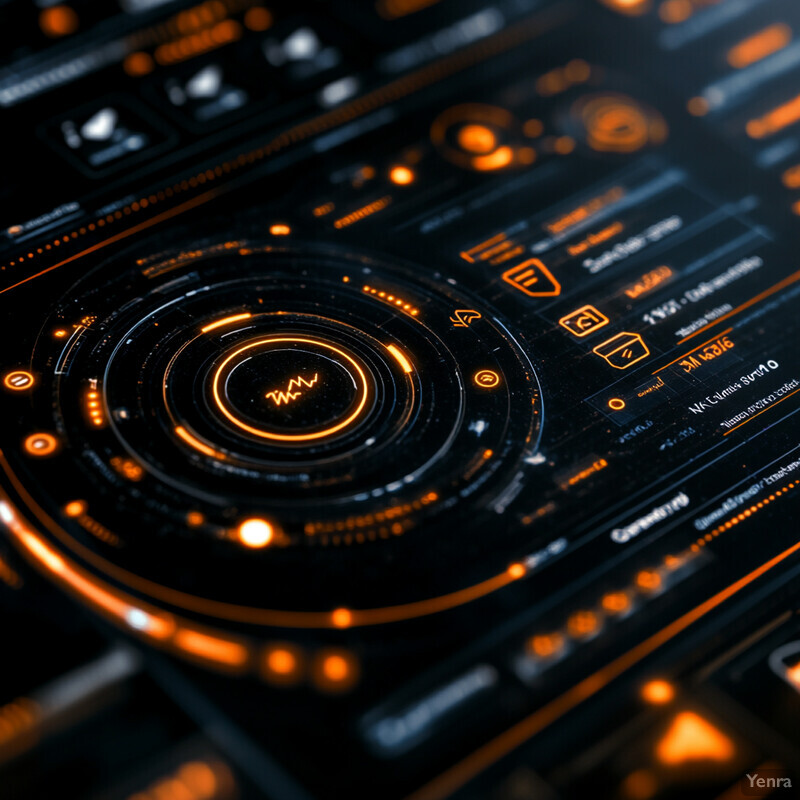
Medical researchers often need to assemble large cohorts that match precise inclusion and exclusion criteria for observational studies, quality improvement initiatives, or retrospective analyses. AI tools expedite this task by extracting and comparing relevant EHR data points (such as diagnostic codes, procedure notes, and medication regimens) across thousands of patients. Researchers can rapidly identify the right patient subsets, reducing the time and labor typically required for manual chart reviews. The resulting cohorts are more accurate, ensuring that studies are based on high-quality, representative samples of the patient population.
15. Reducing Administrative Burden
Automated clinical documentation assistants can help clinicians input structured data directly into the EHR, reducing administrative tasks and freeing up time for patient care.
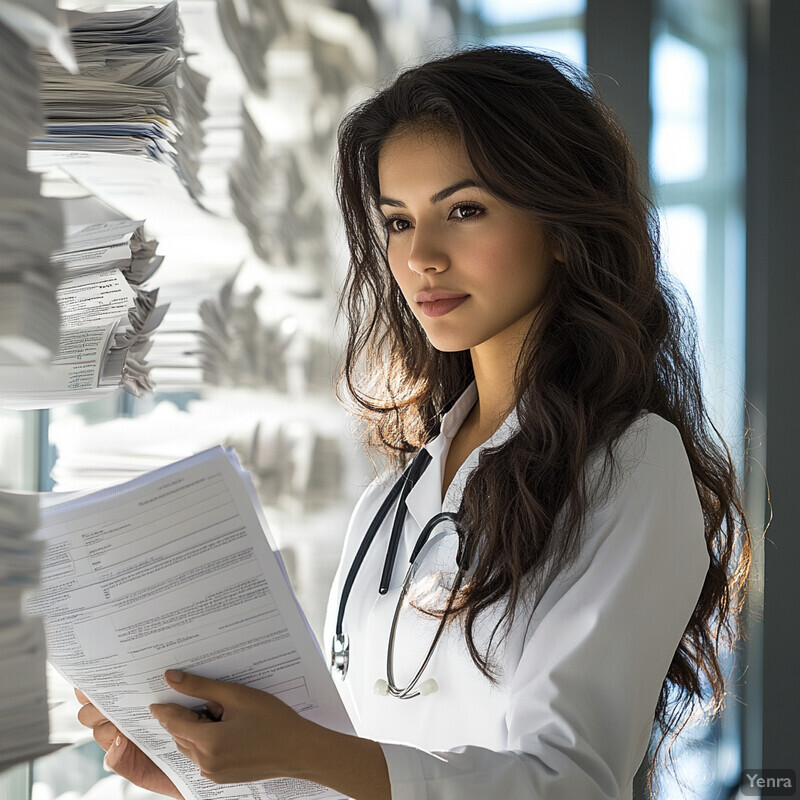
Clinicians today spend a significant amount of their time documenting patient encounters and inputting data into the EHR. AI-powered clinical documentation assistants help automate these tasks by converting voice narratives into structured notes and suggesting relevant templates or codes. This technology decreases the time providers spend on paperwork, allowing them to focus more on direct patient care. Over time, reduced administrative burden leads to better job satisfaction among clinicians, improved patient-provider relationships, and a decrease in documentation-related burnout.
16. Data Mining for Undiagnosed Conditions
Complex pattern recognition can uncover hidden correlations, helping detect underdiagnosed or rare conditions that might be missed by human review.
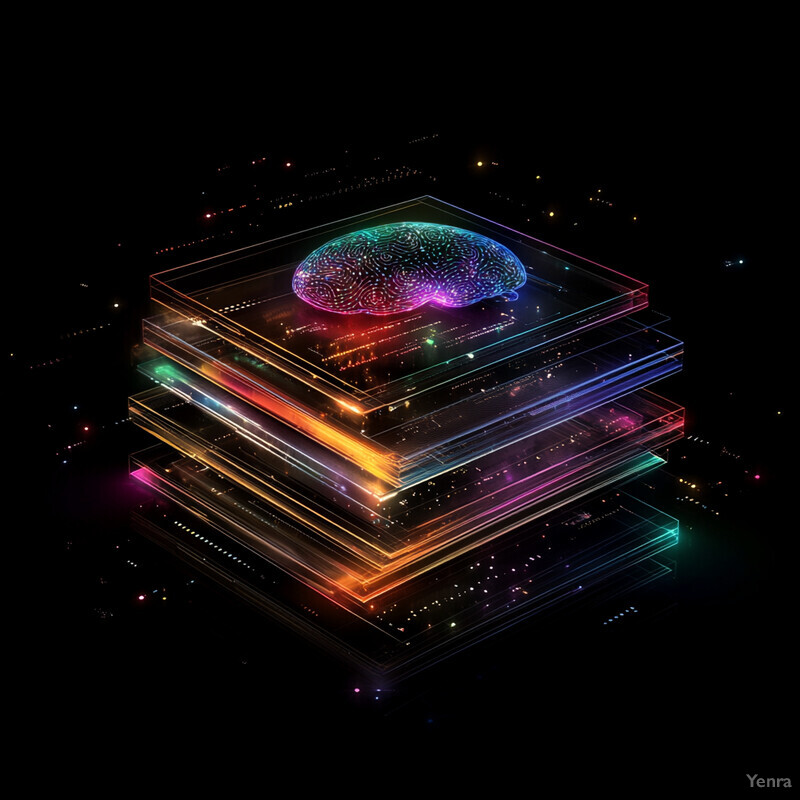
Complex diseases and rare conditions can be challenging to diagnose early. AI can mine vast amounts of patient data to detect subtle patterns that clinicians might overlook. For example, certain obscure symptom clusters combined with specific lab value trends could indicate the presence of a rare genetic disorder. By surfacing these correlations, AI helps clinicians consider conditions they might not have otherwise suspected. Early identification and diagnosis improve the chances of timely interventions, better prognoses, and more effective treatments.
17. Integration with Wearable and IoT Data
AI facilitates the seamless incorporation of data from wearable devices and home monitoring systems into EHRs, providing a fuller clinical picture.
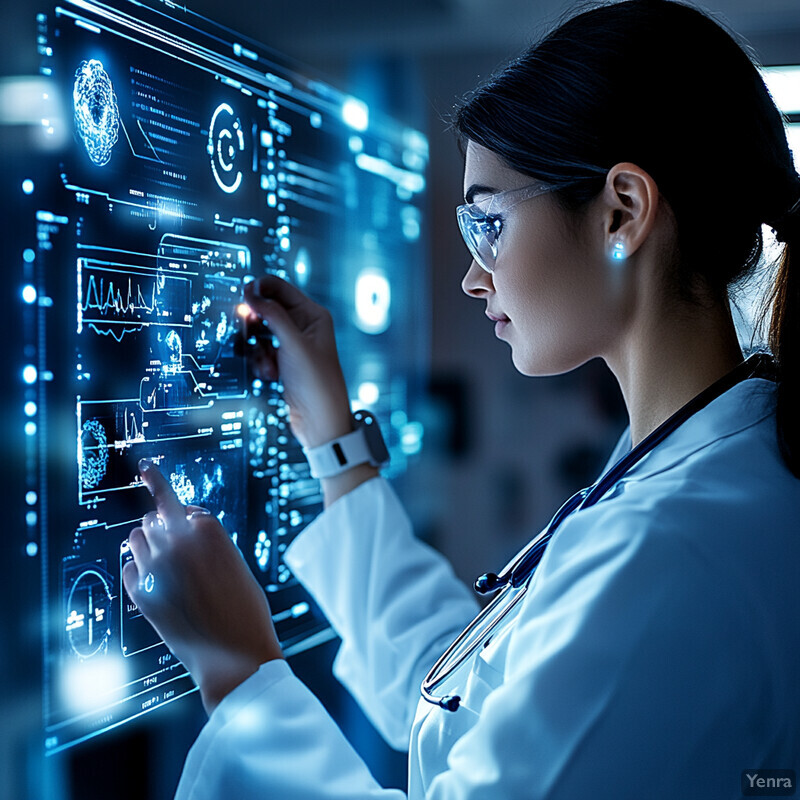
The explosion of wearable devices and Internet of Things (IoT) sensors in healthcare generates continuous streams of patient data—from heart rate monitors and blood glucose trackers to at-home blood pressure cuffs. AI facilitates the aggregation and analysis of this data alongside traditional EHR information. This comprehensive view allows clinicians to monitor patient health outside of hospital settings, identify changes early, and implement remote interventions. As a result, patients can enjoy more flexible, personalized care, and health systems can reduce hospital visits, ultimately improving patient convenience and outcomes.
18. Decision Support for Imaging and Pathology
By correlating radiology and pathology findings with patient records, AI can help identify patterns that lead to more accurate diagnoses.
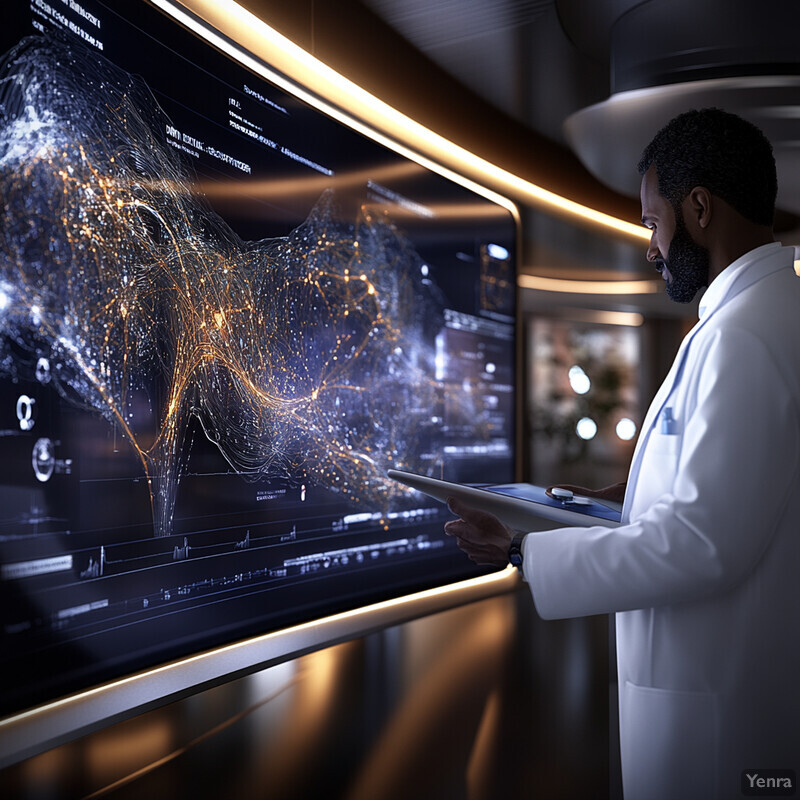
Combining EHR data with advanced image analysis algorithms can help radiologists and pathologists make more accurate diagnoses. AI-driven image recognition tools can highlight suspicious lesions on imaging studies or identify subtle cellular changes in pathology slides. By correlating these findings with a patient’s overall clinical context from the EHR, clinicians receive a more holistic picture, enabling more confident, data-driven decision-making. Enhanced diagnostic accuracy reduces the likelihood of misdiagnosis, minimizes unnecessary follow-up tests, and leads to more effective and timely treatments.
19. Fraud Detection and Compliance Monitoring
AI can spot unusual billing patterns or discrepancies in documentation, helping maintain regulatory compliance and prevent fraud.
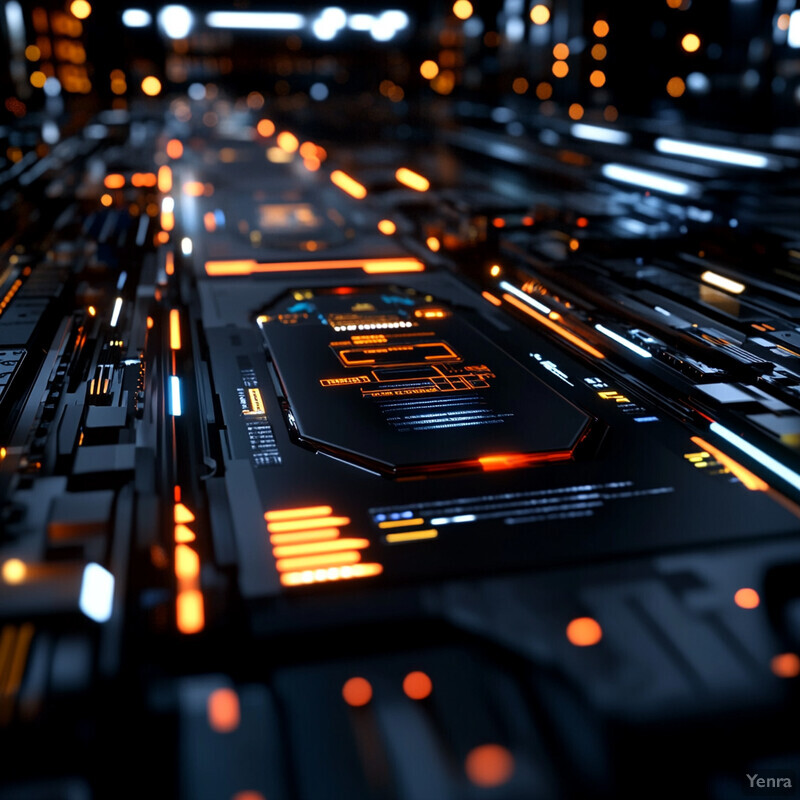
Healthcare fraud and billing discrepancies are persistent challenges that can drain resources and increase costs. AI algorithms can detect unusual patterns in billing records, identify suspicious coding practices, and flag discrepancies that might indicate fraudulent activity. By cross-referencing claims data with EHR information, these systems ensure providers adhere to regulatory standards and help maintain the financial integrity of healthcare organizations. Over time, reducing fraud and non-compliance translates into cost savings, better resource allocation, and enhanced public trust in the healthcare system.
20. Privacy-Preserving Analytics
Advances in federated learning and differential privacy enable meaningful analysis of EHR data without compromising patient confidentiality.
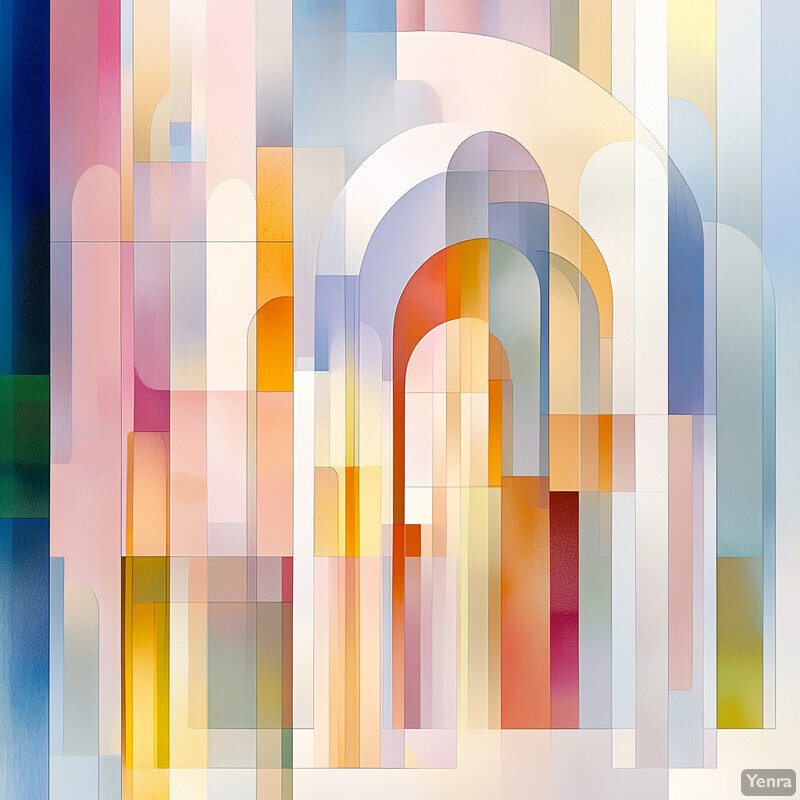
While AI-driven insights offer tremendous benefits, protecting patient privacy remains paramount. New approaches like federated learning and differential privacy allow healthcare institutions to perform analytics on distributed EHR data without compromising confidentiality. These methods enable models to train on data that never leaves the source institution, or to incorporate noise into the dataset so individual patient identities remain anonymous. Such privacy-preserving techniques allow organizations to collaborate on large-scale research, share insights, and improve care quality, all while maintaining stringent patient privacy and ethical standards.