1. Enhanced Predictive Power
Modern deep learning techniques—such as convolutional and recurrent neural networks—enable models to capture complex, non-linear relationships between species occurrences and environmental gradients, often resulting in more accurate niche predictions than traditional statistical methods.
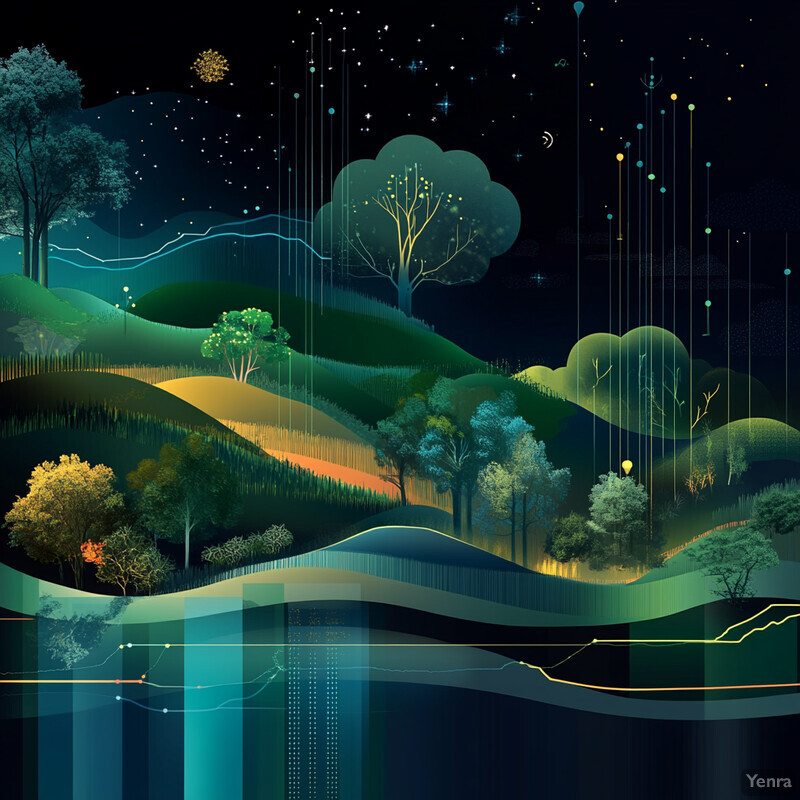
One of the most significant advancements that AI brings to Ecological Niche Modeling (ENM) is vastly improved predictive capabilities. Traditional statistical models often rely on linear or relatively simple relationships between species occurrence and environmental variables. In contrast, AI-based approaches—such as deep neural networks—are adept at capturing highly non-linear and intricate ecological relationships. These models can learn complex patterns from large and noisy datasets, improving their accuracy in predicting where species may occur. Moreover, techniques like ensemble methods and gradient-boosting help refine predictions by combining the strengths of multiple models, thus enhancing overall robustness. The result is more reliable habitat suitability maps that provide better guidance for conservation strategies, invasive species management, and biodiversity assessments.
2. Integration of Diverse Data Sources
AI can simultaneously process large, heterogeneous datasets, including climate variables, topography, soil chemistry, vegetation indices, and remote sensing imagery, thus providing a richer environmental context for niche modeling.
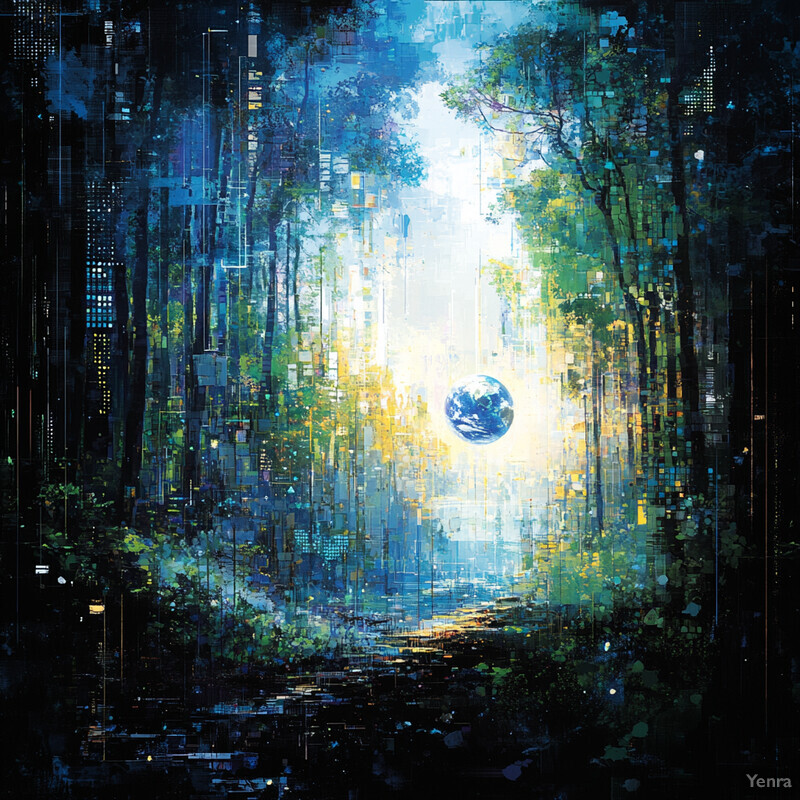
AI-driven ENM can incorporate a wide range of heterogeneous datasets simultaneously. Beyond simple environmental predictors like temperature and precipitation, these approaches can seamlessly integrate soil properties, topographic complexity, vegetation indices, land use data, genetic information, and remote sensing imagery. By doing so, the modeling process becomes more holistic, capturing the multidimensional nature of an ecological niche. As AI methods scale with increasing data complexity, ecologists gain a more nuanced understanding of the conditions that define species distributions. This richer data integration fosters more ecologically sound models, enabling researchers and managers to develop strategies grounded in a comprehensive environmental context.
3. Automated Feature Extraction
Advanced feature selection methods, including unsupervised learning and representation learning, can identify the most important environmental predictors without requiring manual selection, improving model efficiency and performance.
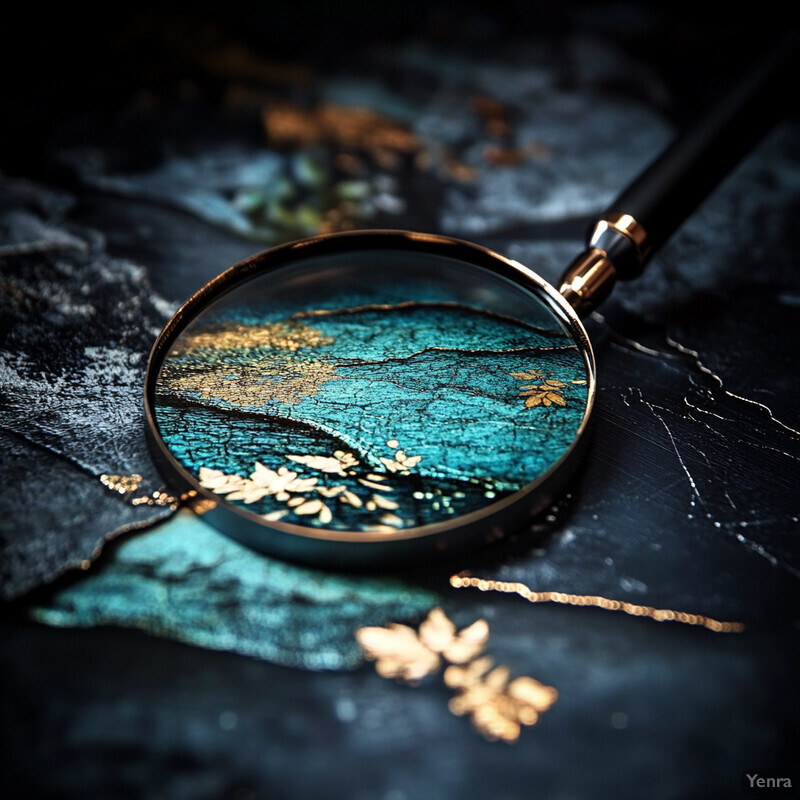
Traditional ENM techniques often require expert input to select and preprocess explanatory variables. AI, on the other hand, can perform automated feature extraction and dimensionality reduction, identifying the most relevant environmental variables without extensive manual intervention. Using methods such as autoencoders, principal component analysis enhanced by neural networks, or unsupervised clustering, AI systems reveal hidden environmental gradients and subtle ecological drivers. This capability streamlines the modeling process, reduces biases that might arise from subjective variable selection, and ensures that niche models are more data-driven and less constrained by human assumptions.
4. Handling Big Data and High-Dimensionality
AI algorithms are well-suited to manage the 'curse of dimensionality.' They can efficiently work with hundreds or thousands of predictor variables, uncovering subtle patterns and relationships previously missed by simpler models.
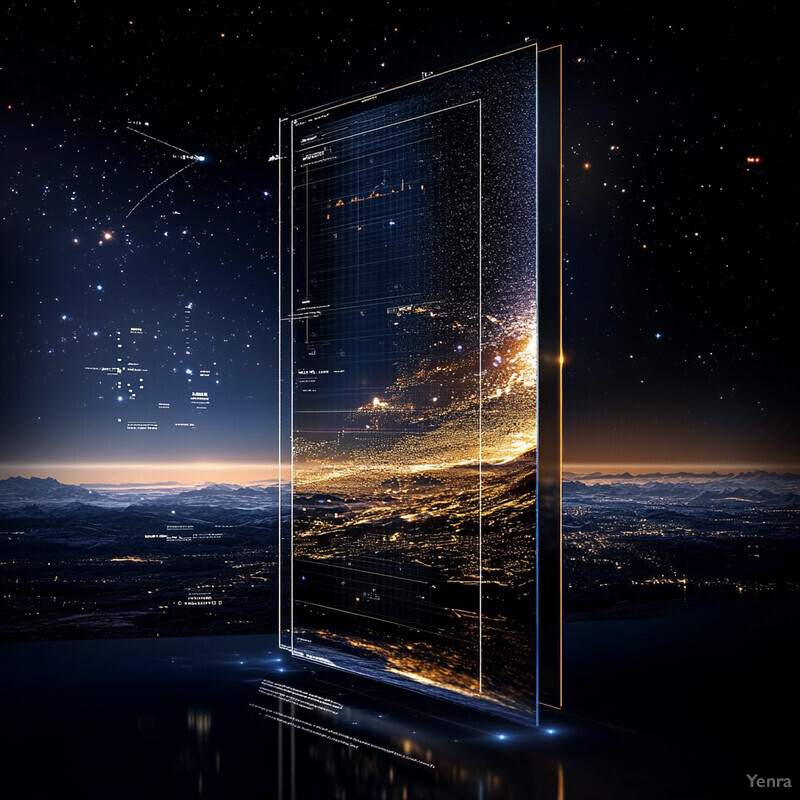
Ecological datasets are ballooning in size and complexity, often encompassing thousands of environmental variables and millions of occurrence records. AI models—especially those designed for high-performance computing environments—can efficiently handle these large, multidimensional data sets. These models can sift through vast amounts of information to detect meaningful patterns and interactions that simpler, more constrained statistical methods would miss. By managing the “curse of dimensionality,” AI-driven ENM can accommodate richer datasets and produce more fine-grained, accurate maps of species distributions at unprecedented spatial and temporal scales.
5. Improved Species Absence and Presence Data Integration
By leveraging methods like generative adversarial networks or semi-supervised learning, AI can help refine pseudo-absence generation and better integrate presence-only datasets, yielding more robust niche estimations.
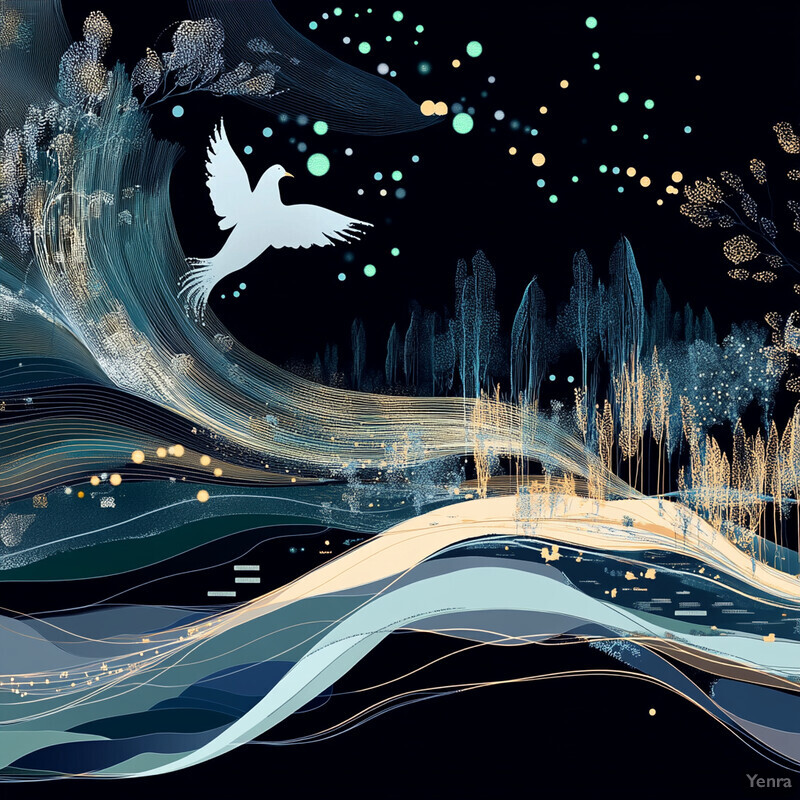
Many niche modeling projects rely on presence-only occurrence data, making it challenging to properly characterize the ecological conditions suitable for a species. AI can address this gap by employing methods like semi-supervised learning, synthetic data generation, or generative adversarial networks to create more realistic pseudo-absence datasets. These techniques help improve model calibration and performance by ensuring that the absence data used are ecologically plausible. As a result, AI-driven ENM methods deliver more robust predictions, better reflect the true ecological niche, and offer insights into conditions that limit species’ distributions.
6. Uncertainty Quantification and Propagation
Bayesian deep learning and ensemble methods can provide probabilistic outputs, helping ecologists understand and communicate the uncertainty in niche boundaries and future projections under different climate scenarios.
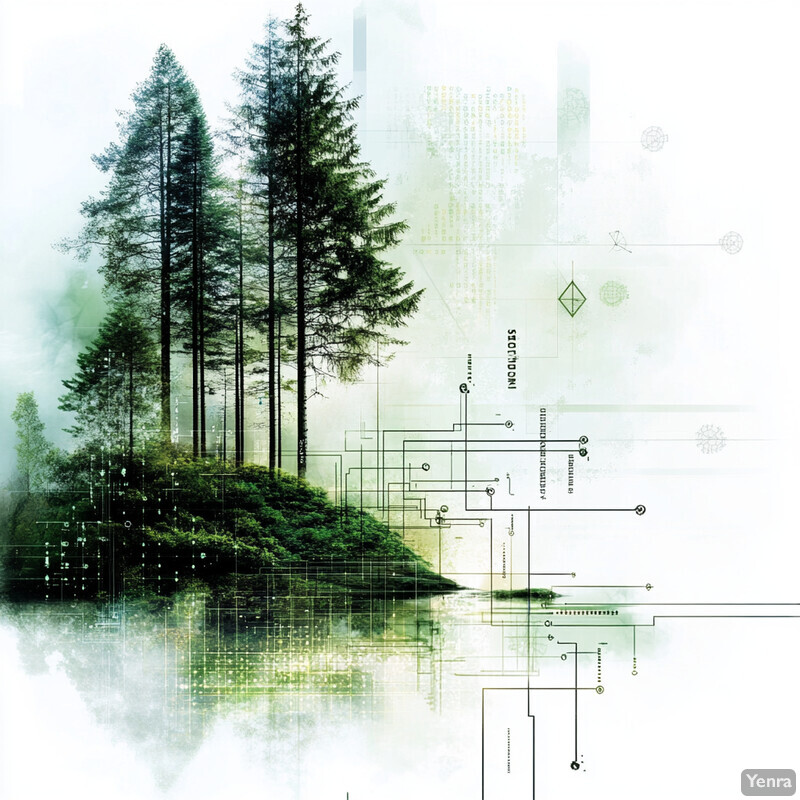
Uncertainty is a core issue in ENM, and AI provides powerful tools for quantifying and communicating it. Bayesian deep learning, quantile regression forests, and ensemble approaches generate probabilistic outcomes rather than single-point estimates. This allows ecologists to understand the confidence intervals or prediction intervals around species distribution predictions. By explicitly representing uncertainty, AI-driven ENM helps stakeholders make more informed decisions—such as identifying priority areas for conservation where model agreement is high, or pinpointing regions where more data collection is needed due to greater uncertainty.
7. Spatial and Temporal Resolution Improvements
AI models can be trained to handle spatial autocorrelation and temporal non-stationarity, leading to niche predictions that remain robust across changing environmental conditions and shifting landscapes.
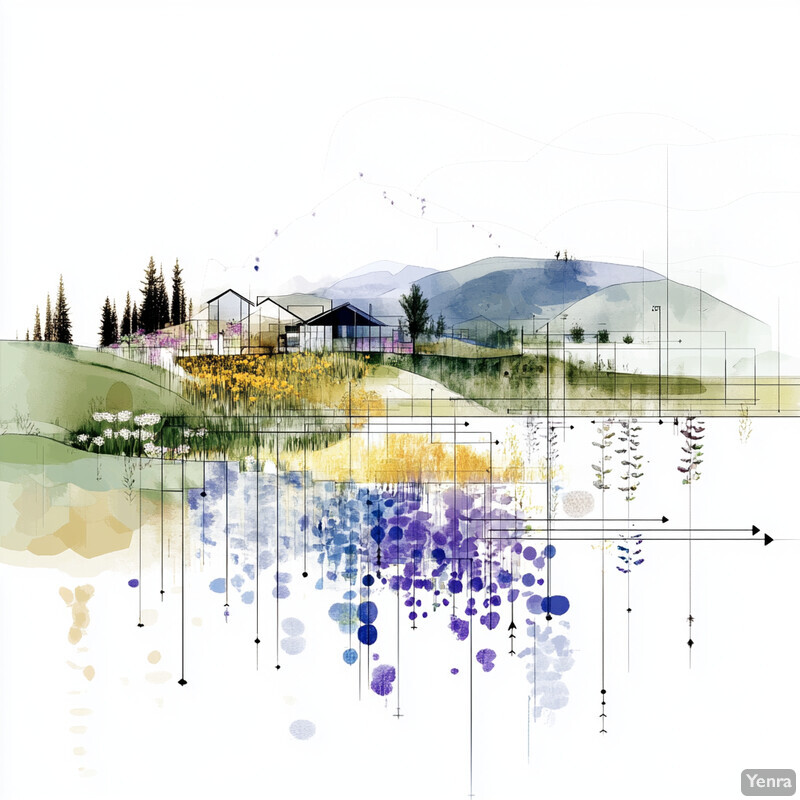
Species distributions are inherently dynamic, changing as environmental conditions shift over space and time. AI techniques can handle spatiotemporal complexity more effectively than traditional methods. Advanced spatial modeling algorithms can incorporate spatial autocorrelation, while temporal models (e.g., recurrent neural networks) can track seasonal variations, climate cycles, and historical environmental changes. These refined models capture the non-stationarity of species-environment relationships, producing niche predictions that remain valid despite environmental shifts. Such granularity is crucial for managing ecosystems in the face of climate change and land-use alterations.
8. Transfer Learning Between Species and Regions
Models pretrained on well-studied species or well-characterized regions can be adapted to less-documented taxa or habitats using transfer learning, accelerating the modeling process and reducing data requirements.
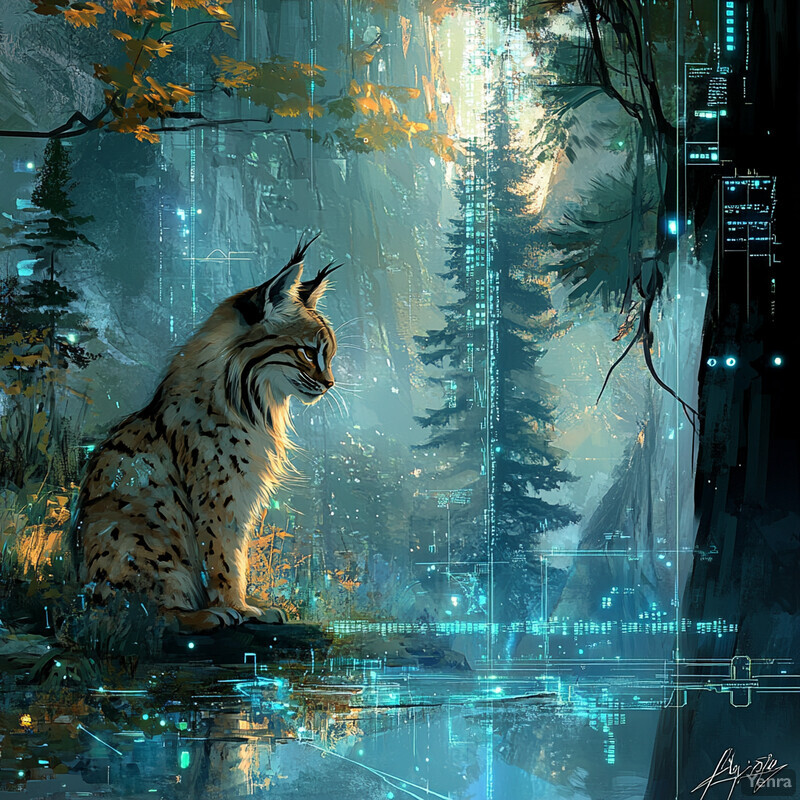
One hallmark of AI is the ability to reuse learned representations from one domain and apply them to another, a concept known as transfer learning. For ENM, this means models trained on well-studied species with rich occurrence data or well-characterized regions can be adapted to model understudied species or less-explored habitats. By leveraging shared environmental gradients or similar ecological traits, AI-driven models can rapidly improve predictions for data-poor scenarios. This accelerates the process of understanding niche requirements for a broader range of taxa and regions, ultimately supporting more comprehensive biodiversity assessments.
9. Integration with Remote Sensing Data
AI excels at extracting information from complex remote sensing imagery (e.g., hyperspectral or LiDAR data), offering insights into microhabitats and fine-scale environmental heterogeneity that improve niche delineation.
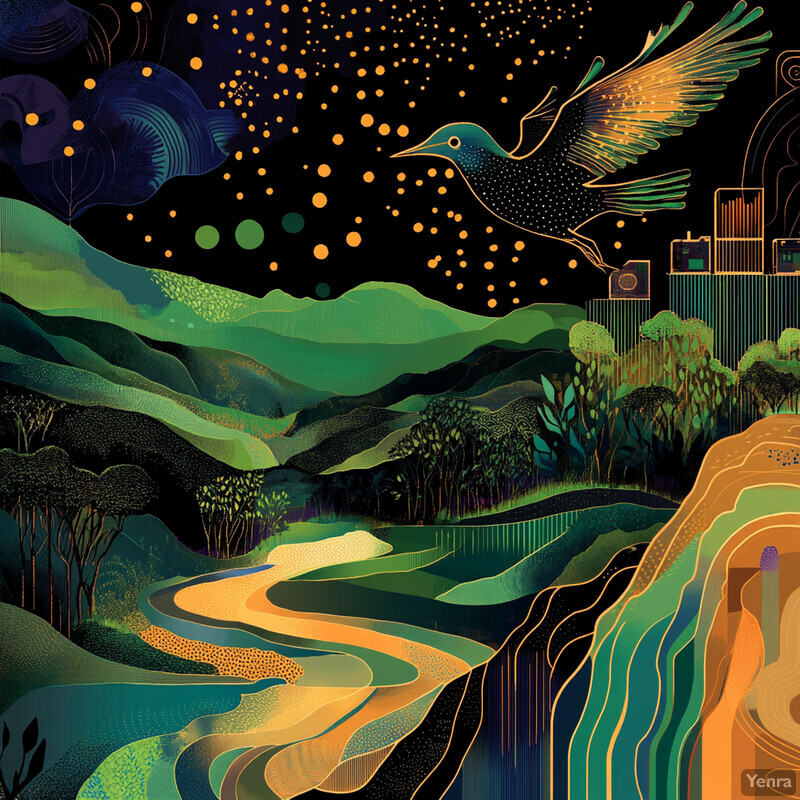
AI excels at analyzing complex imagery, making it a natural fit for incorporating remote sensing data—like multispectral or hyperspectral images and LiDAR. These datasets capture detailed habitat structure, vegetation patterns, and microclimatic variations. Deep convolutional neural networks can learn spatial patterns and features that correlate with species presence. By integrating remotely sensed data, AI-driven ENM can identify subtle habitat qualities, such as canopy density or understory composition, offering a richer, more accurate depiction of the species’ realized niche. This is particularly valuable for studying cryptic species or habitats that are difficult to sample directly.
10. Real-time and Near-real-time Predictions
With the aid of streaming environmental data and AI-based sensor fusion, niche models can be updated rapidly to reflect current conditions, aiding in dynamic conservation strategies and early detection of invasive species.
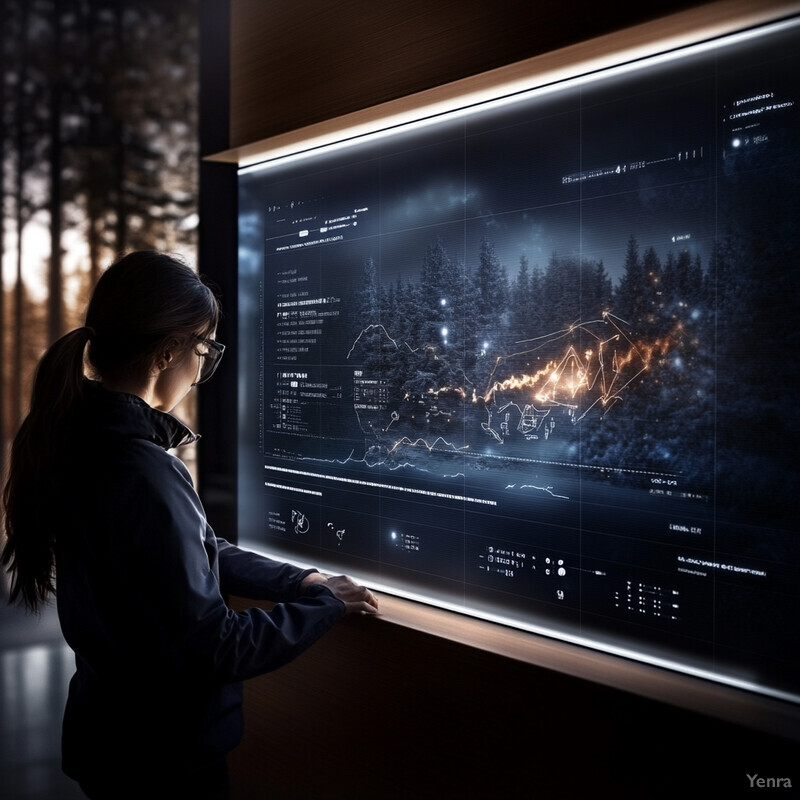
Environmental conditions and species occurrences can change rapidly, and timely predictions are vital for effective management. AI-driven ENM can leverage streaming datasets—such as climate sensor networks, citizen science observations, and satellite feeds—and update species distribution predictions on a near-real-time basis. Techniques like online learning allow models to incorporate new data continuously, ensuring that maps remain current and reflect immediate environmental conditions. This dynamic modeling capability can guide rapid decision-making during ecological crises, such as the sudden spread of invasive species or the aftermath of extreme weather events.
11. Enhanced Model Interpretability
New explainable AI techniques can highlight which environmental factors most influence a species’ modeled niche, providing ecological insights and making results more transparent and actionable for decision-makers.
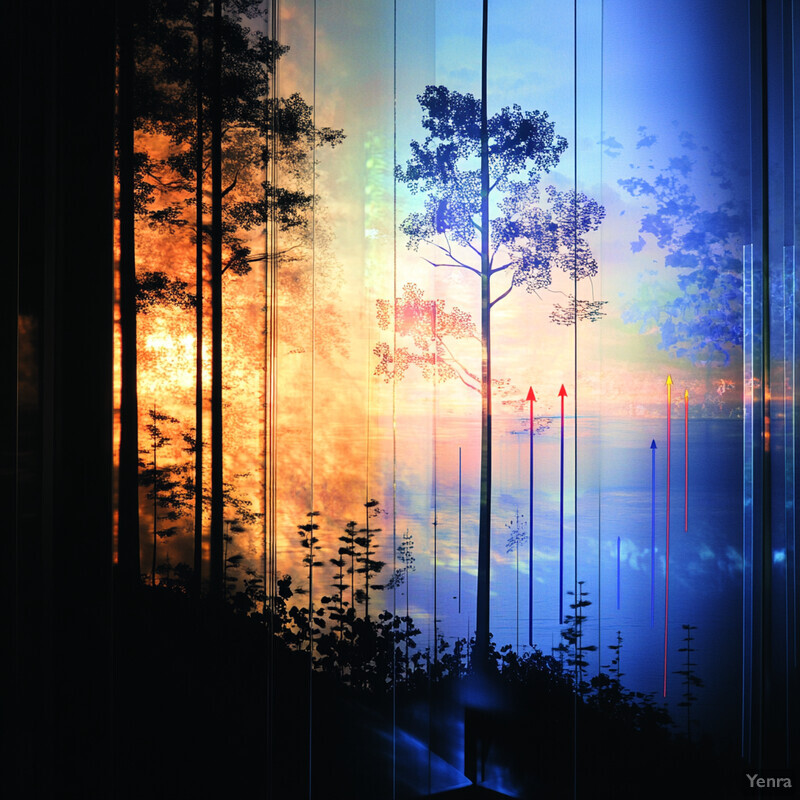
As AI models become more complex, ensuring that their predictions are transparent and interpretable has become a priority. Explainable AI methods, including feature importance rankings, partial dependence plots, and techniques like SHAP or LIME, can highlight which environmental factors most strongly influence model outputs. By providing insight into the “black box,” ecologists and conservationists can better trust and understand the predictions. This transparency fosters stakeholder acceptance, informs targeted management actions, and supports the development of more hypothesis-driven ecological research.
12. Adaptive Sampling Strategies
Reinforcement learning can guide field sampling efforts by identifying areas of greatest uncertainty, ensuring that data collection efforts are more targeted and cost-effective, which ultimately improves model quality.
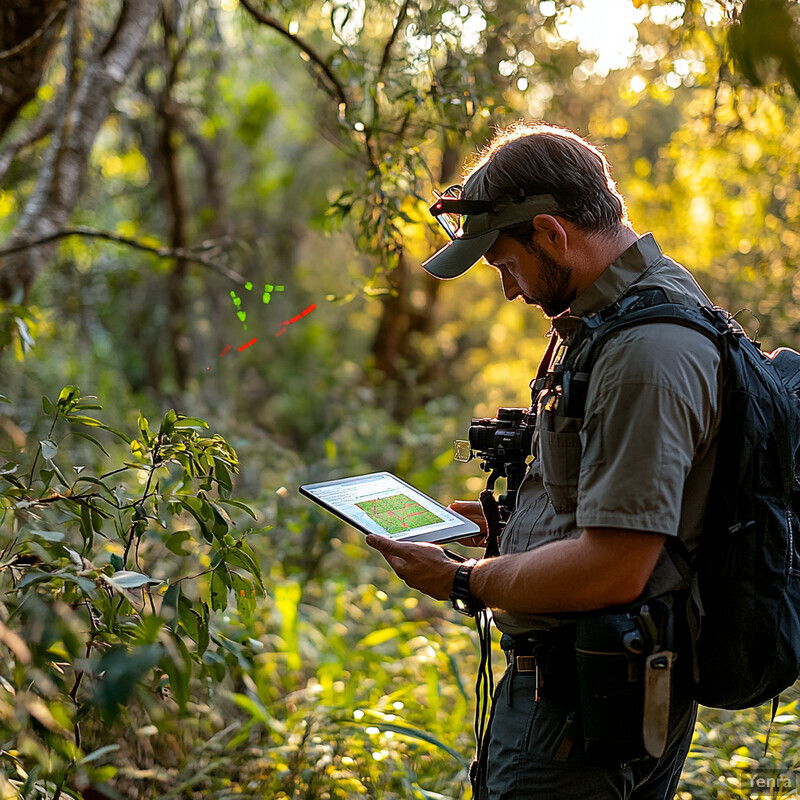
Data collection in the field can be costly and time-consuming. AI can optimize sampling efforts through reinforcement learning or active learning approaches that identify where new data would most improve the model. By guiding researchers to areas of high uncertainty, AI-driven ENM ensures that limited resources yield maximum value in terms of model refinement. Over time, these feedback loops help produce more accurate niche models, accelerate biodiversity inventories, and improve conservation planning by filling critical data gaps efficiently.
13. Climate Change Scenario Projections
AI can downscale coarse global climate model outputs to finer spatial resolutions, enabling more accurate niche projections under future climate scenarios and better informing conservation planning.
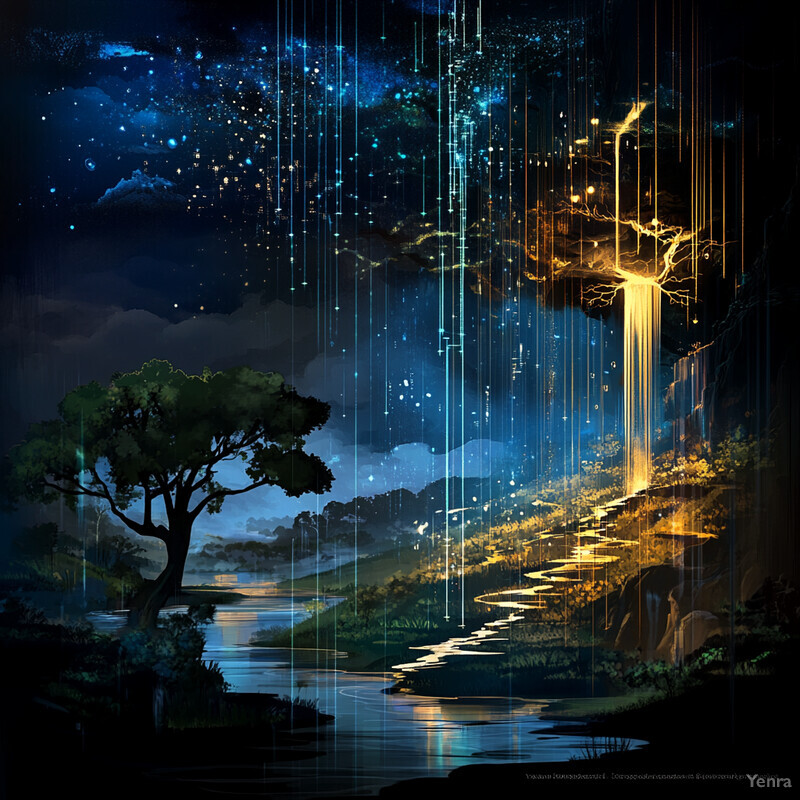
Predicting how species distributions will shift under future climate scenarios is a critical application of ENM. AI can downscale coarse global climate model projections to finer spatial resolutions and incorporate these detailed environmental forecasts directly into niche models. By leveraging advanced interpolation and pattern recognition techniques, AI offers more precise scenarios of how changing temperatures, precipitation patterns, and extreme weather events will alter suitable habitats. With improved scenario modeling, conservationists can prioritize habitats for protection, restoration, and assisted migration, ultimately supporting proactive biodiversity management strategies.
14. Hybrid Modeling Approaches
AI can bridge the gap between purely statistical and process-based ecological models by integrating mechanistic understanding (e.g., physiological constraints) with data-driven predictions, yielding more ecologically realistic outcomes.
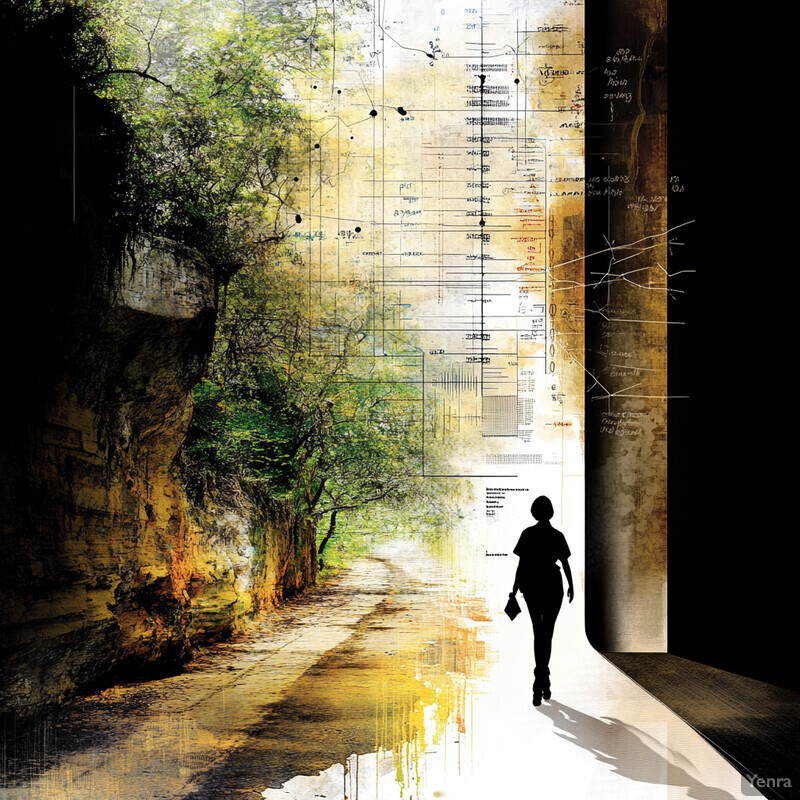
Purely statistical ENM techniques can overlook underlying biological mechanisms, while purely mechanistic models may miss real-world complexities. AI can bridge this gap by integrating mechanistic constraints—such as physiological limits on temperature tolerance—with data-driven predictions. This hybrid approach ensures that models are both biologically plausible and empirically grounded. The result is more ecologically realistic niche estimates that can guide ecological restoration, inform climate adaptation strategies, and improve our understanding of species’ fundamental vs. realized niches.
15. Accounting for Phenological and Behavioral Complexity
Time-series analysis and sequence models (e.g., LSTMs) can incorporate seasonal changes, migration patterns, and other temporal factors into niche models, capturing dynamics that static methods overlook.
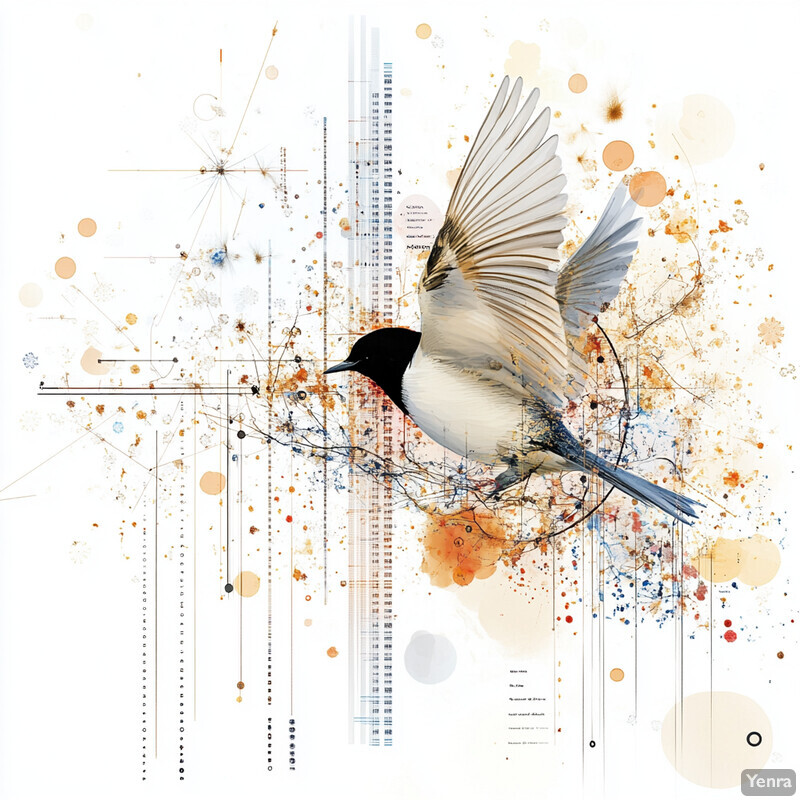
Seasonal migrations, breeding seasons, dormancy periods, and other temporal patterns influence where and when species can thrive. Advanced AI methods, such as recurrent neural networks or temporal convolutional networks, can directly model time series data to capture phenological cues and behavioral shifts. By considering temporal dynamics, these models provide a more complete picture of species niches across different life stages and climatic phases. This temporal sophistication is crucial for designing protected areas, predicting invasion timing, and understanding how species may adapt (or fail to adapt) to evolving climate conditions.
16. Data Quality Control and Outlier Detection
AI-based anomaly detection can flag suspicious occurrence points or environmental records, improving the reliability of the data feeding niche models and reducing erroneous predictions.
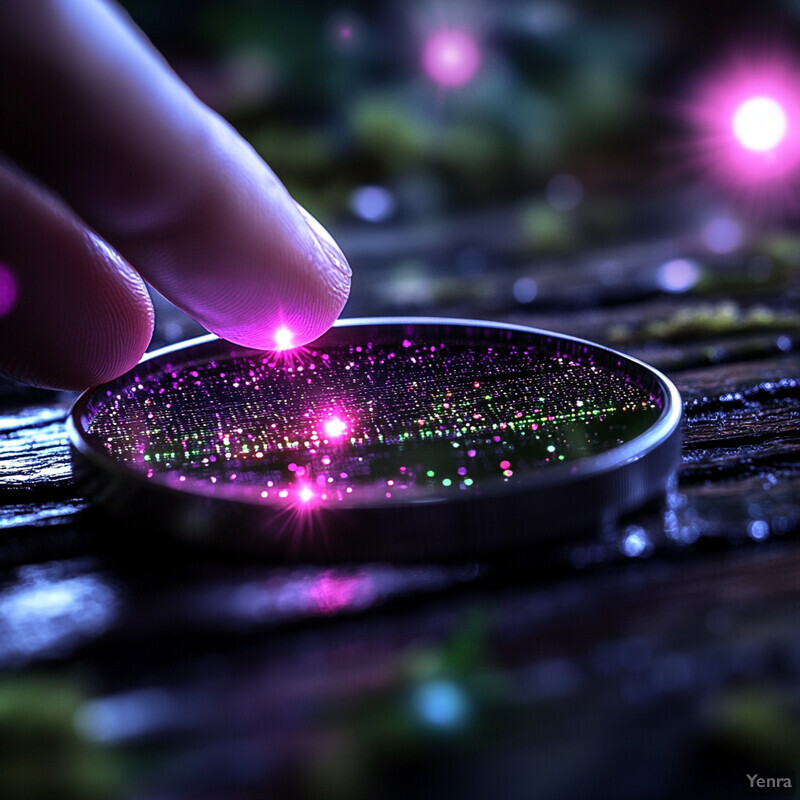
ENM success hinges on data quality. AI-driven anomaly detection techniques can flag suspicious occurrence points, environmental records, or sensor outputs. Unsupervised learning methods, for instance, can identify atypical patterns that may indicate misidentified species, erroneous GPS coordinates, or instrument calibration issues. By refining the input data before model training, AI ensures that the resulting niche predictions are based on reliable and accurate information. This leads to more trustworthy maps of species distributions and reduces the risk of misguided management actions based on faulty datasets.
17. Multi-species and Community-level Analyses
By modeling multiple species simultaneously and using techniques from community-level machine learning, AI can identify emergent patterns in biodiversity, facilitate ecological network analyses, and reveal interspecific interactions.
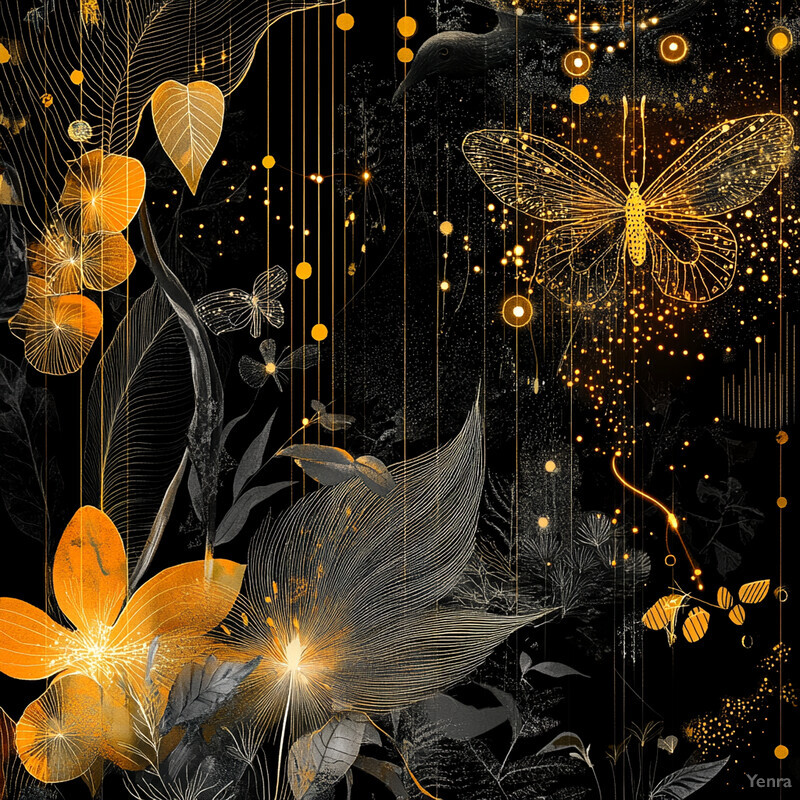
Instead of modeling each species independently, AI methods can handle multi-species modeling tasks simultaneously. Deep learning architectures that consider community-level patterns can reveal interspecific interactions, such as competition, predation, or symbiosis. Such community-level ENM approaches foster a richer understanding of ecosystem functioning and can illuminate how changes in one species’ distribution might cascade through the community. This broader ecological perspective is vital for biodiversity conservation strategies that account for complex network dynamics and ecosystem services.
18. Improving Rare Species Modeling
For species with scarce occurrence data, AI methods can make the most of limited information through data augmentation techniques, synthetic data generation, and clever prior distributions to achieve more stable niche estimates.
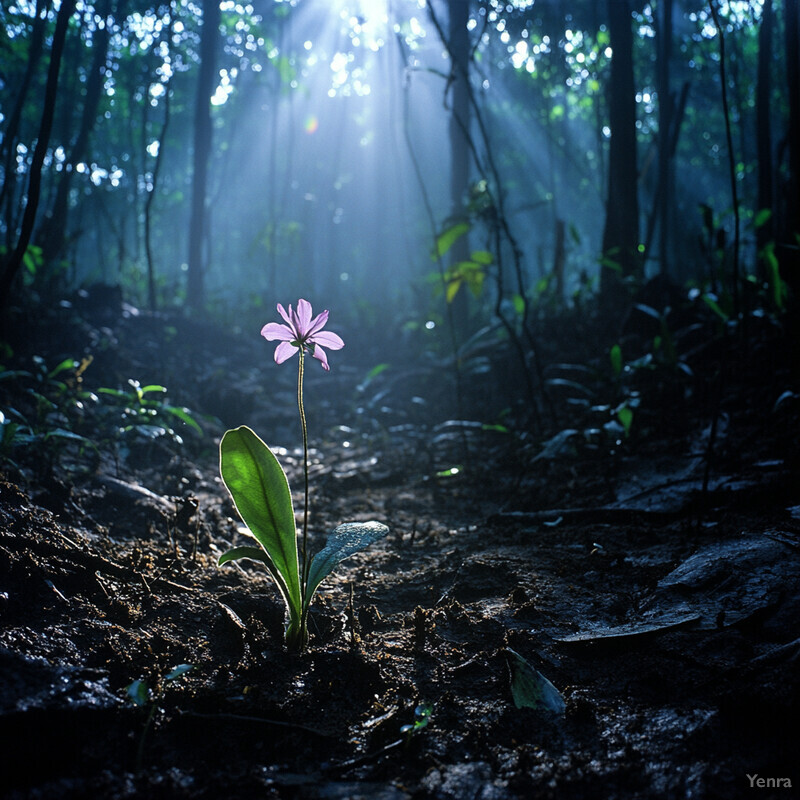
Rare or data-poor species present a major challenge in ENM due to scant occurrence records. AI can compensate by employing data augmentation strategies, transfer learning from related species, or probabilistic modeling techniques that account for small sample sizes. These approaches improve predictive stability and reduce biases that might incorrectly exclude rare species from conservation plans. As a result, AI-driven ENM ensures that even the most elusive taxa are not overlooked, supporting a more comprehensive and equitable approach to biodiversity protection.
19. Accelerated Computation and Scalability
High-performance computing platforms and distributed AI frameworks can run large-scale niche modeling studies more quickly, enabling comparisons across large regions or multiple species without prohibitive computational costs.
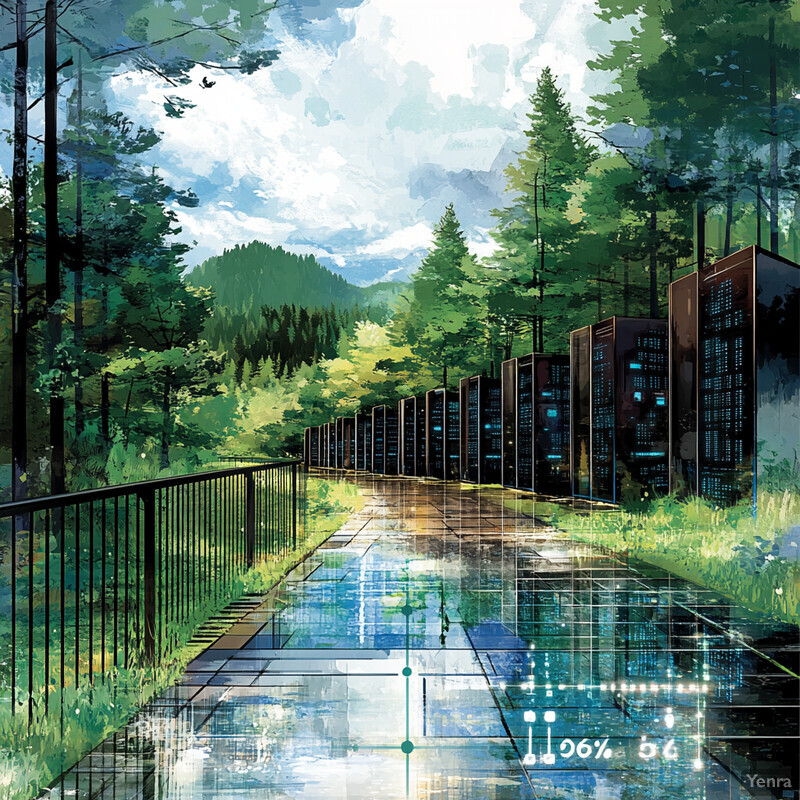
AI models thrive in parallel computing environments and can run on powerful graphics processing units (GPUs) or cloud-based infrastructures. As a result, extensive ENM projects that might have taken weeks or months with conventional methods can now be completed more rapidly. This increase in computational efficiency enables global-scale comparisons, repeated model runs for sensitivity analyses, and extensive scenario testing. By shortening feedback loops, AI-driven ENM empowers researchers to respond more swiftly to emerging ecological threats and to refine their models iteratively as new data become available.
20. Decision-support Tools Integration
AI-driven niche modeling outputs can be seamlessly integrated into decision-support systems, web-based platforms, and conservation planning tools, making the results more accessible and directly usable by policymakers, resource managers, and conservation practitioners.
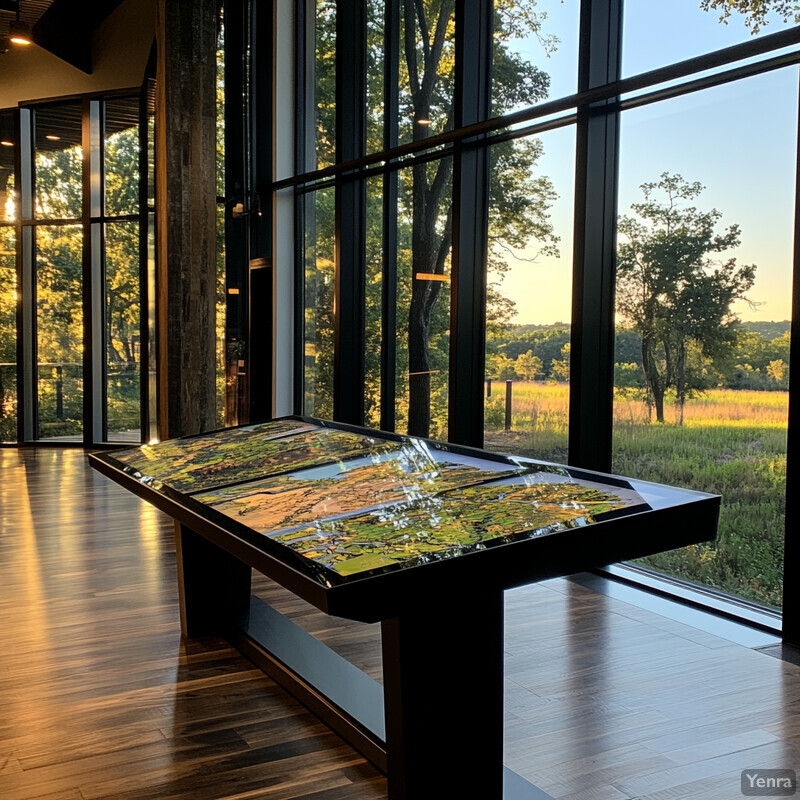
Ultimately, the value of ENM lies in its practical utility for conservation, land-use planning, and resource management. AI allows for the seamless integration of complex niche modeling outputs into user-friendly decision-support tools. Web-based interfaces, mobile applications, and interactive dashboards can present AI-derived predictions in accessible formats for policymakers, conservation practitioners, and the public. By delivering actionable insights directly to those who manage and protect natural habitats, AI-driven ENM ensures that cutting-edge modeling efforts translate into on-the-ground results, enhancing the impact of ecological research and guiding informed decision-making.