1. AI-Driven Target Identification
Machine learning algorithms help identify previously unknown molecular targets related to certain diseases, allowing known drugs to be matched with these new targets more efficiently.
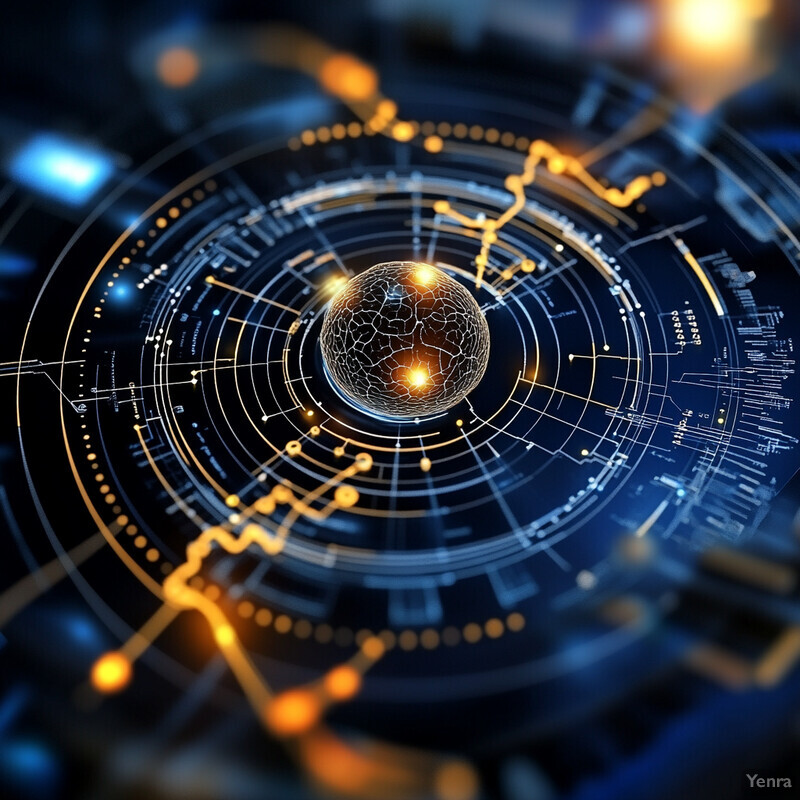
Artificial intelligence (AI) techniques, such as machine learning and deep learning, are revolutionizing how researchers identify druggable targets for repurposing. By analyzing massive datasets—from genetic profiles of diseases to structural and functional information about proteins—AI can pinpoint previously overlooked or unknown molecular targets linked to a particular disorder. For example, systems might use feature extraction methods to pick out subtle correlations between a disease’s genetic underpinnings and a drug’s known mechanism of action. By narrowing down the target space effectively, AI reduces guesswork, guiding scientists toward existing drugs that are more likely to engage these newly identified targets. This increases the odds of finding a high-value repurposing candidate without starting from scratch.
2. Multi-Omics Integration
AI models can integrate genomic, transcriptomic, proteomic, and metabolomic data to uncover hidden connections that reveal a drug's potential in new therapeutic contexts.
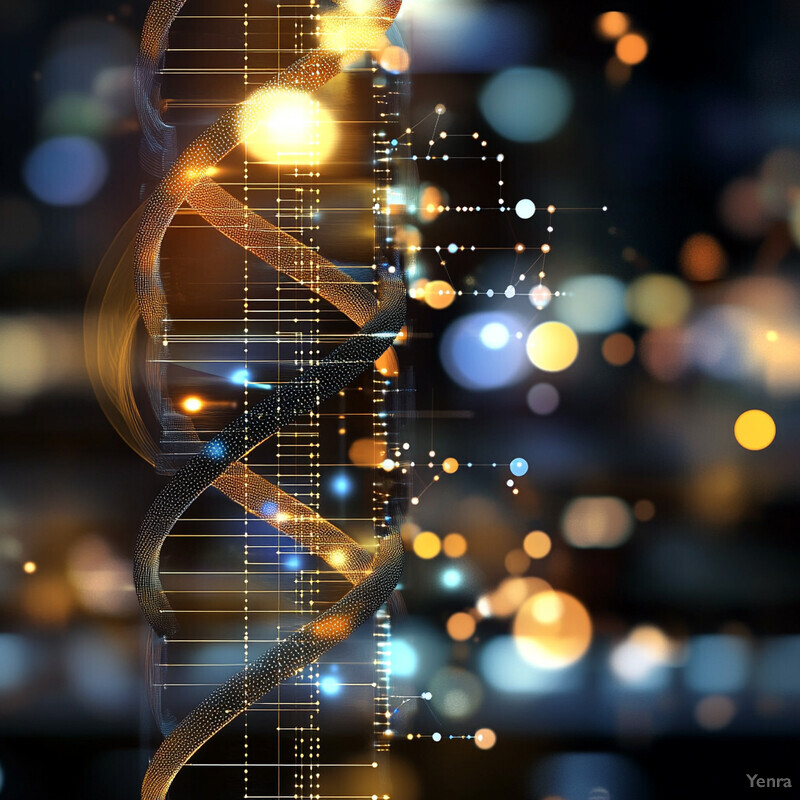
AI can integrate and interpret datasets from multiple biological layers—genomics, transcriptomics, proteomics, metabolomics—in a unified analytical framework. Previously, these data streams were studied separately, making it challenging to see how different molecular changes interconnect. Machine learning models can now correlate shifts in gene expression with changes in protein networks and metabolite levels, revealing patterns that might indicate a particular drug’s suitability for treating a different condition. This holistic, systems-level view allows researchers to uncover subtle molecular fingerprints that link a drug to a disease pathway. By fusing these diverse data sources, AI enhances the sensitivity and specificity of drug repurposing discoveries.
3. Predictive Modeling of Drug-Target Interactions
Deep learning approaches, such as graph neural networks and transformer-based models, can predict interactions between drugs and proteins with greater accuracy, revealing promising repurposing opportunities.
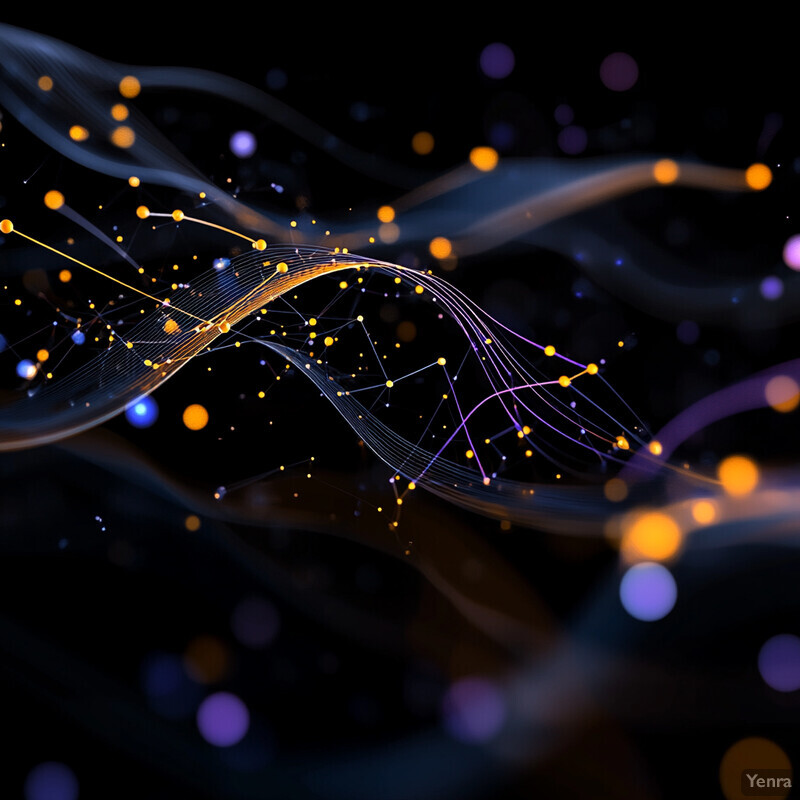
Machine learning models, including graph neural networks, deep learning architectures, and docking-based algorithms, enable more accurate predictions of how drugs will interact with biological targets. Previously, scientists relied on trial-and-error or simplistic computational models that often failed to capture the complexity of molecular interactions. Now, AI-based predictive modeling can incorporate structural, physiochemical, and conformational data to forecast drug binding affinity and specificity. These improved predictions help researchers quickly filter out unlikely candidates and focus on drugs with a high probability of engaging new targets. This capability streamlines the repurposing pipeline and saves both time and resources.
4. Natural Language Processing for Literature Mining
AI-powered NLP tools rapidly scan and interpret the biomedical literature, extracting insights on off-label drug use, side effect profiles, and molecular interactions that can hint at potential repurposing avenues.
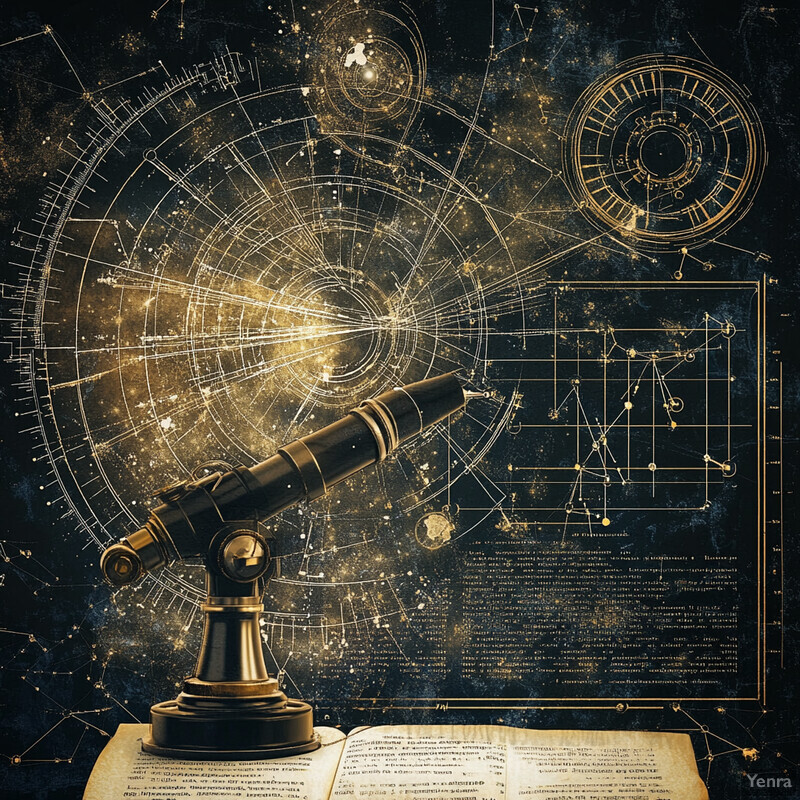
AI-driven NLP tools excel at extracting actionable insights from the vast and continuously expanding body of biomedical literature. Instead of manually sifting through countless papers and clinical trial reports, NLP systems can semantically analyze text, detect patterns, and generate summaries about drug efficacy, side effects, and relevant molecular pathways. By automating literature review, NLP reduces human error and accelerates the hypothesis generation process for repurposing. It can identify off-label uses and unexpected correlations that would be easy to overlook. Integrating these insights with other data sources allows researchers to build more robust rationales for repurposing candidates.
5. Knowledge Graphs and Reasoning Engines
AI-driven knowledge graphs integrate heterogeneous biomedical data, making it easier to navigate relationships between diseases, pathways, and compounds.
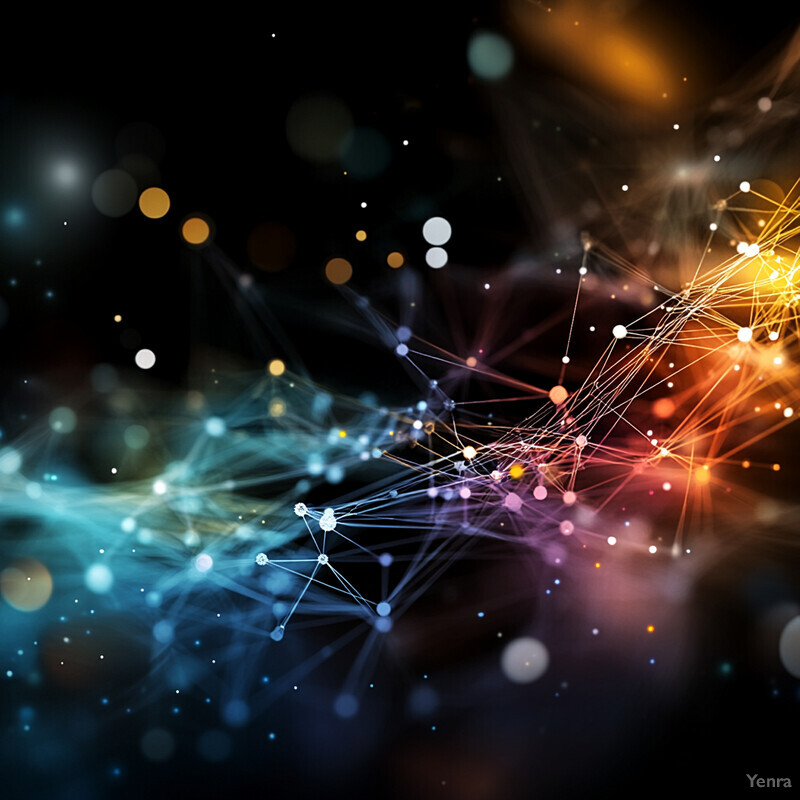
Building and interrogating complex knowledge graphs allows AI to represent the relationships among drugs, diseases, genes, proteins, and pathways in a structured, interconnected manner. Reasoning engines can navigate these graphs to infer previously unrecognized links, such as a known drug having potential activity against a disease-associated network of interacting proteins. This approach goes beyond isolated data points, letting researchers follow chains of evidence through multiple layers of biological complexity. By applying logical inference and machine learning techniques on top of these graphs, AI can highlight new hypotheses for drug repurposing that are grounded in a rich tapestry of biomedical knowledge.
6. High-Throughput Virtual Screening
Machine learning can accelerate the virtual screening of large drug libraries against disease-associated targets, improving the speed and accuracy of identifying promising repurposing hits.
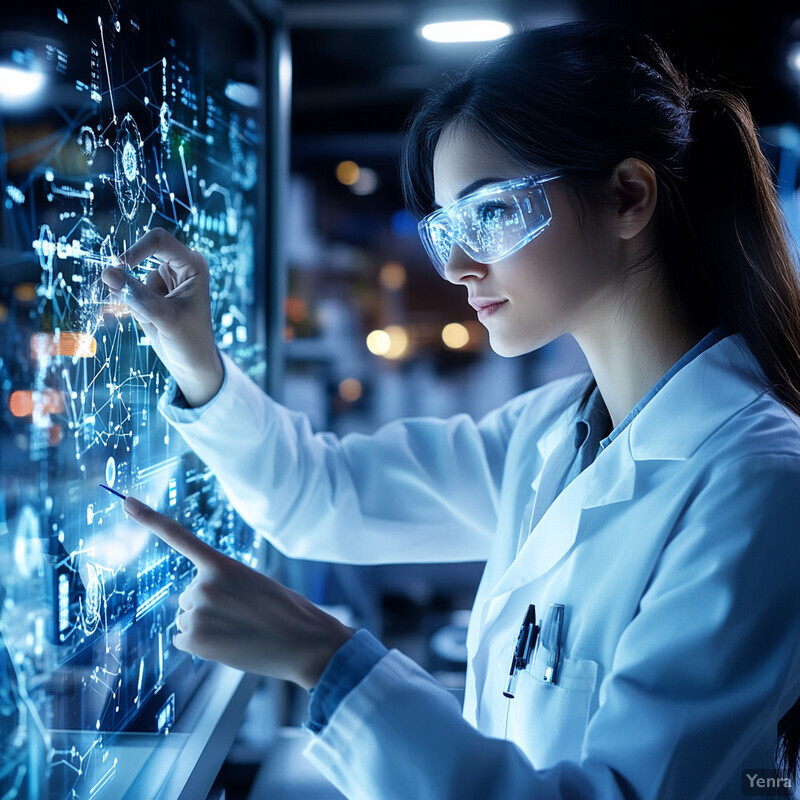
AI significantly speeds up virtual screening by rapidly evaluating the potential interactions between large compound libraries and disease targets. Traditional virtual screening could be computationally expensive and limited in scope. Machine learning models optimize this process by learning patterns in molecular structures and target binding sites, allowing them to quickly eliminate unpromising candidates. This approach not only reduces the computational burden but also improves hit rates. Efficient high-throughput virtual screening enables researchers to identify repurposing opportunities more swiftly, supporting a more agile approach to drug discovery.
7. Automated Hypothesis Generation
AI platforms can autonomously generate testable hypotheses about how certain marketed drugs could be beneficial in treating other conditions, streamlining the early discovery phase.
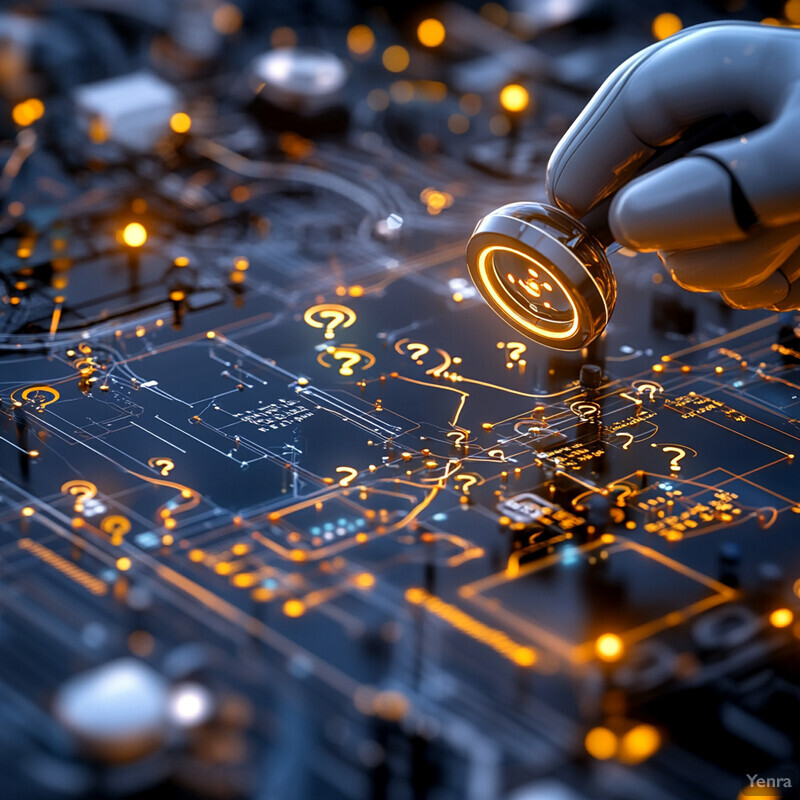
AI platforms can autonomously propose new hypotheses about potential drug-disease relationships. By continuously scanning emerging literature, patient data, and molecular studies, these systems identify patterns and suggest which existing drugs might help treat other conditions. This removes the initial “shot in the dark” associated with traditional repurposing efforts. Instead of relying solely on human intuition and serendipity, researchers gain a systematic pipeline that can generate testable ideas at scale. Such an AI-driven approach fosters innovation and accelerates the discovery process, ensuring that valuable repurposing opportunities don’t remain hidden.
8. Mechanistic Insight into Drug Actions
By analyzing data at molecular and pathway levels, AI helps elucidate the mechanisms by which a known drug might ameliorate a different disease, supporting rational repurposing strategies.
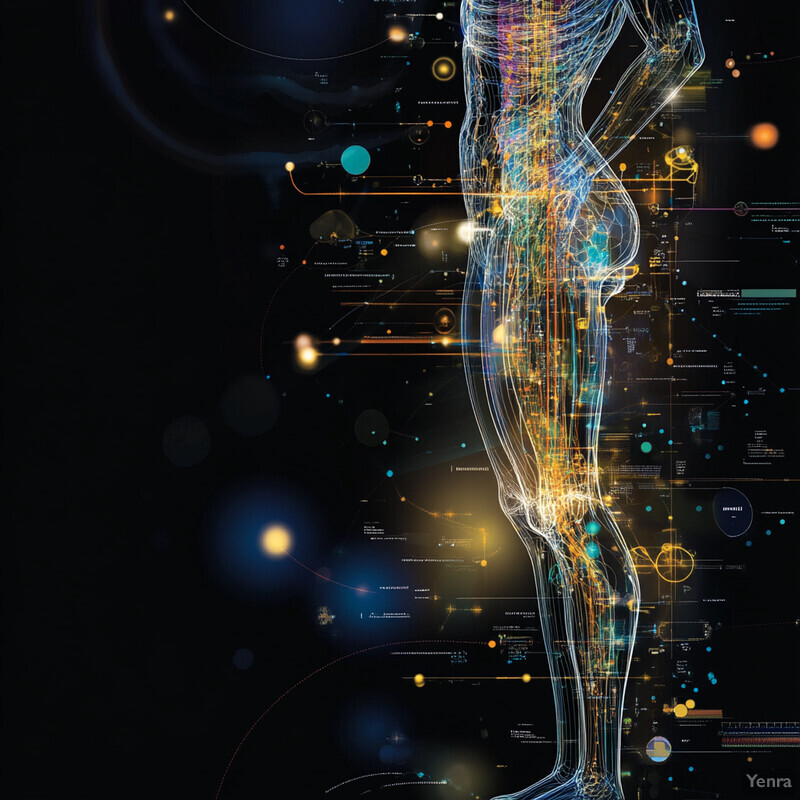
One of the key bottlenecks in repurposing is understanding why a drug might work in a new indication. AI can unravel the mechanistic details by mapping a drug’s known interactions onto disease-specific molecular pathways and gene regulatory networks. Machine learning algorithms can help identify which signaling cascades or biological processes are modulated by the drug in the context of the new disease. With deeper mechanistic insight, researchers can better predict side effects, refine dosing strategies, and design more effective clinical trials. This knowledge-driven approach elevates repurposing efforts from guesswork to evidence-based decision-making.
9. Real-World Evidence Extraction
AI techniques can mine electronic health records (EHRs) and insurance claims to detect patterns of drug usage and disease improvement, highlighting repurposing candidates with real-world validation.
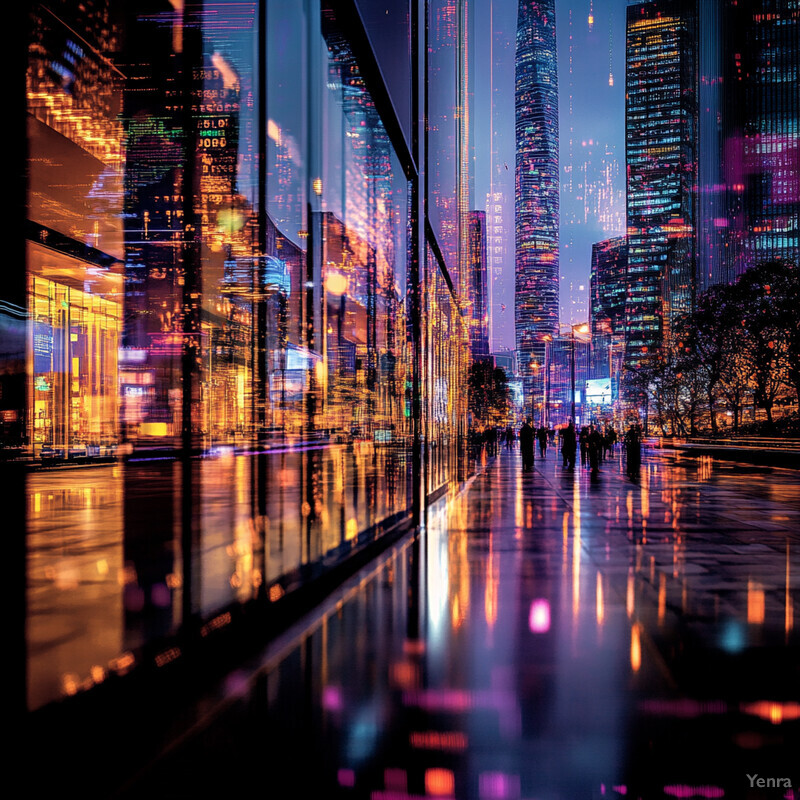
Electronic health records (EHRs) and other real-world data sources contain a wealth of information about how drugs perform outside controlled clinical trial settings. AI can mine these records to identify patterns—such as patients with certain comorbidities improving on a given medication—suggesting repurposing opportunities. By correlating treatment outcomes, patient demographics, and medication histories, machine learning models can highlight drugs that have unsuspected benefits. This direct-from-clinic insight complements lab-based discoveries and can accelerate the prioritization of drugs for formal repurposing trials.
10. Adverse Event Analysis as Clues
Machine learning can spot patterns in adverse event reports that suggest a secondary beneficial effect for a drug, guiding researchers to explore its repurposing potential.
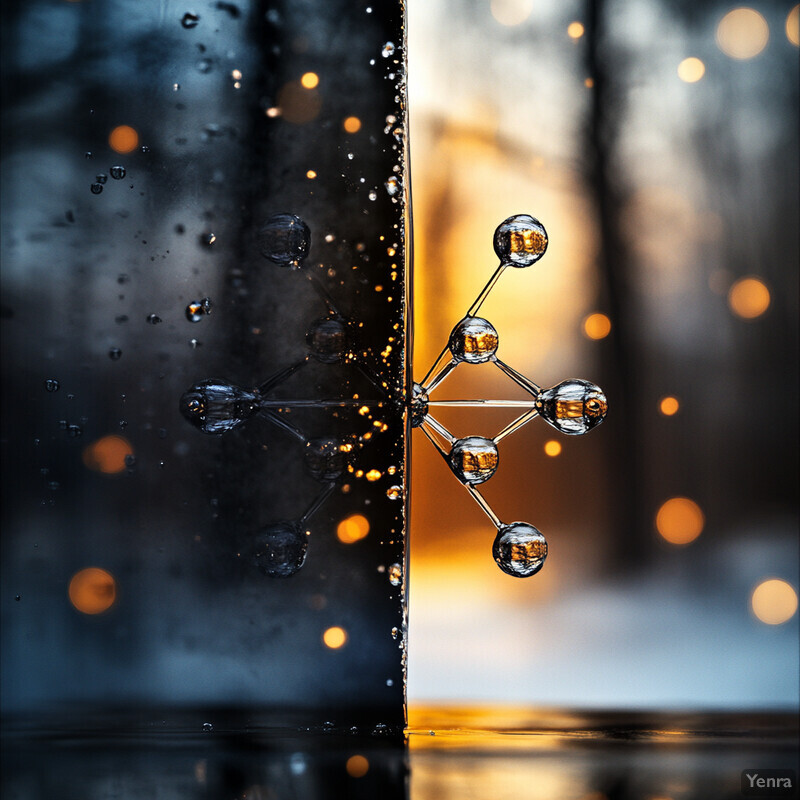
Adverse event data, such as those collected in pharmacovigilance databases, often contain hidden signals that a drug might influence disease pathways unrelated to its primary indication. AI can detect patterns where a side effect in one population might imply a beneficial effect in another. For example, if a heart medication consistently improves a certain biomarker in patients with a different, unrelated condition, this could hint at a repurposing opportunity. By turning adverse event analyses into discovery tools, AI helps transform what was once purely a safety concern into valuable lead-generation data.
11. Phenotypic Screening Analysis
AI-driven image analysis can interpret phenotypic assay data, linking observed cellular changes to potential therapeutic benefits of existing drugs in new disease contexts.
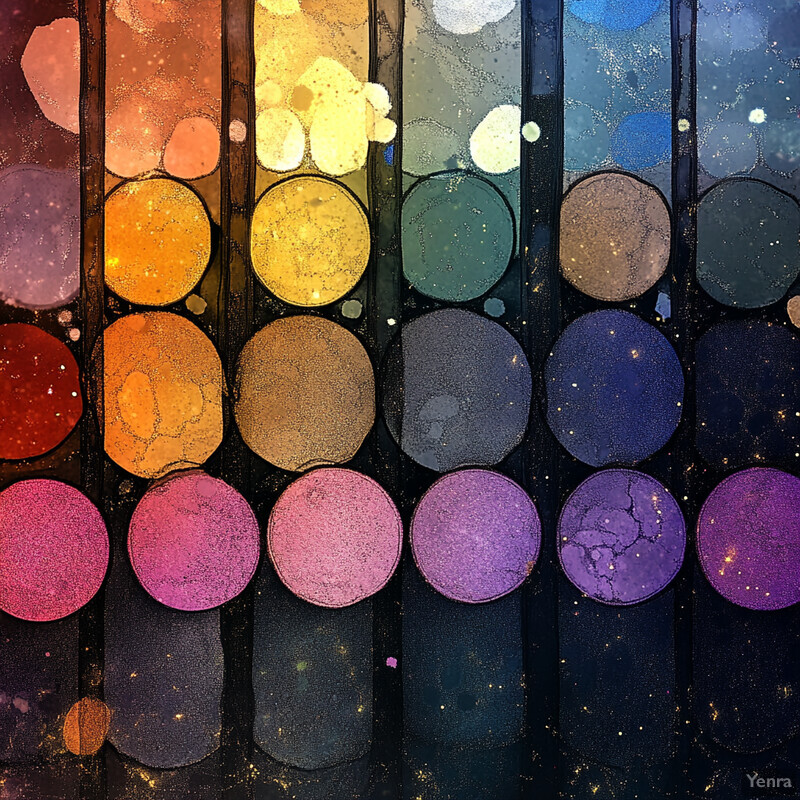
Phenotypic screens involve observing measurable changes in cell or tissue models when exposed to certain drugs. AI-driven image recognition and machine learning algorithms can interpret these complex phenotypic changes far more efficiently and objectively than human evaluators. They can correlate morphological or behavioral alterations with particular molecular pathways or disease states. This refined interpretation can reveal unexpected drug activities, pointing to new therapeutic uses. In this way, AI makes phenotypic screening a more powerful component of the drug repurposing toolkit.
12. Drug Combination Synergy Prediction
Deep learning models evaluate combinations of existing therapies to predict synergistic effects, hinting at how a repurposed drug might enhance efficacy when paired with another agent.
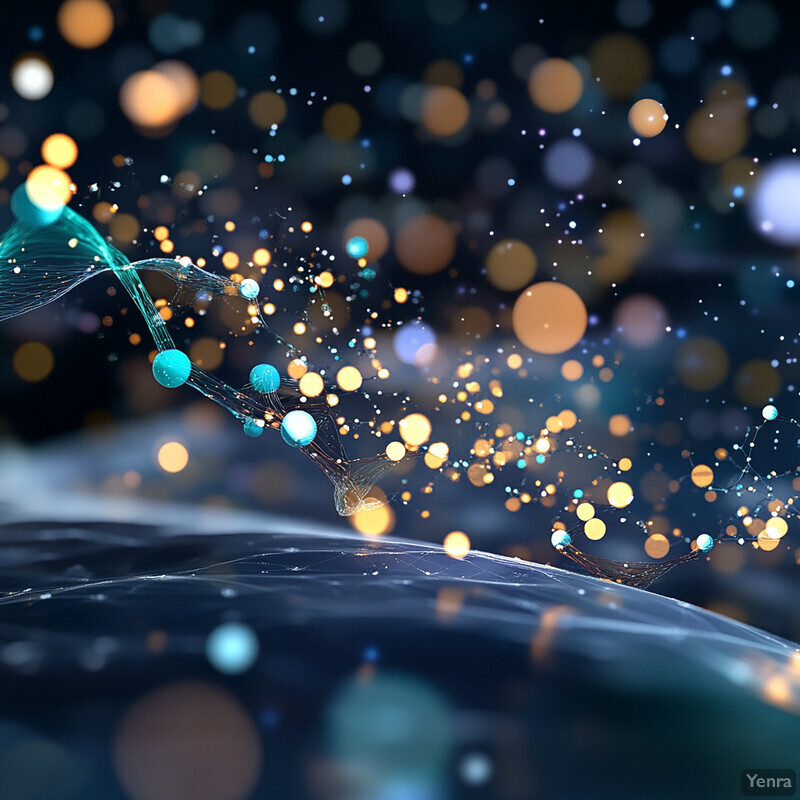
Combining drugs is often an effective strategy to improve treatment outcomes or reduce resistance. AI models can predict which pairs or sets of existing drugs might produce synergistic effects, helping to identify repurposing candidates that work best when administered alongside another agent. By analyzing molecular profiles, known drug interactions, and patient response data, machine learning algorithms highlight combinations that enhance efficacy or reduce side effects. This approach can transform monotherapy repurposing leads into effective combination therapies, broadening the impact of existing drug libraries.
13. Network Pharmacology Approaches
AI analyzes complex biological networks to understand how multiple targets and pathways may be modulated by a single drug, uncovering hidden repurposing opportunities within intricate disease networks.
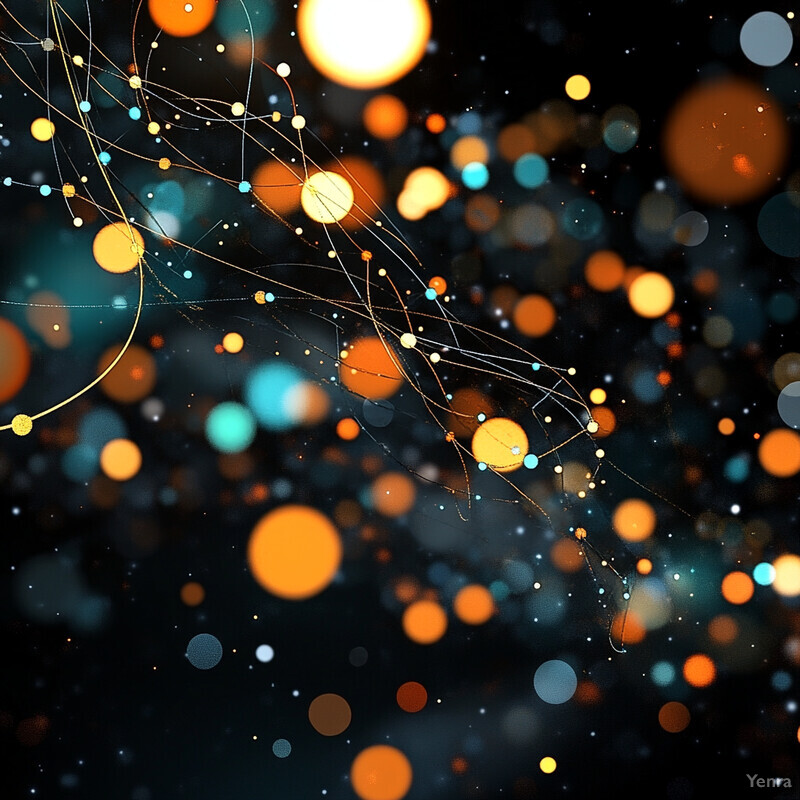
Diseases are complex networks of interacting molecules and pathways rather than just single protein targets. AI-driven network pharmacology can map these intricate webs, identifying where a drug might exert its influence at multiple nodes. By understanding how a drug affects entire biological systems, researchers can match it to diseases that share similar network imbalances. This systems-level perspective often reveals hidden connections missed by traditional one-target-at-a-time methods. As a result, network-based analysis boosts the success rate of identifying meaningful repurposing targets.
14. Reinforcement Learning for Optimization
Advanced reinforcement learning methods help iteratively test and refine repurposing hypotheses, improving the selection of candidates with each computational 'round' of learning.
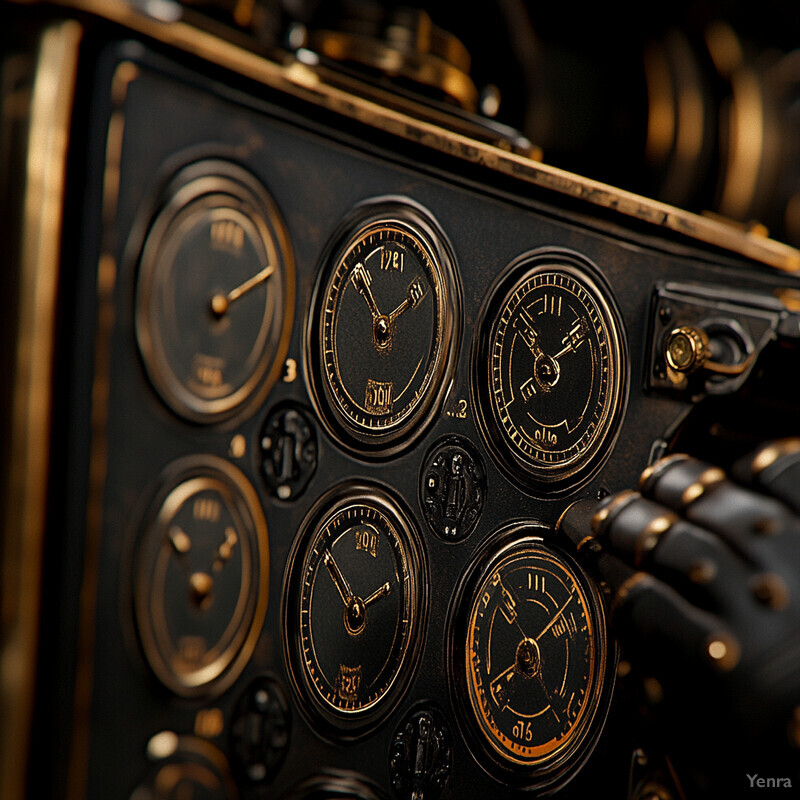
Reinforcement learning (RL) methods allow AI systems to learn through trial and error, adjusting their strategies as they gain feedback from virtual experiments. In drug repurposing, RL can iteratively refine candidate lists, focus on promising hypotheses, and discard unfruitful leads. By continuously improving its decision-making algorithms, RL approaches can prioritize the best paths forward and reduce wasted effort. This helps expedite the repurposing pipeline, ensuring that computational resources and research funds are invested in the most promising opportunities.
15. Clustering and Similarity-Based Discovery
AI can group diseases based on molecular profiles and cluster drugs by chemical structure or mode of action, revealing unexpected overlaps that suggest a drug’s relevance to a new indication.
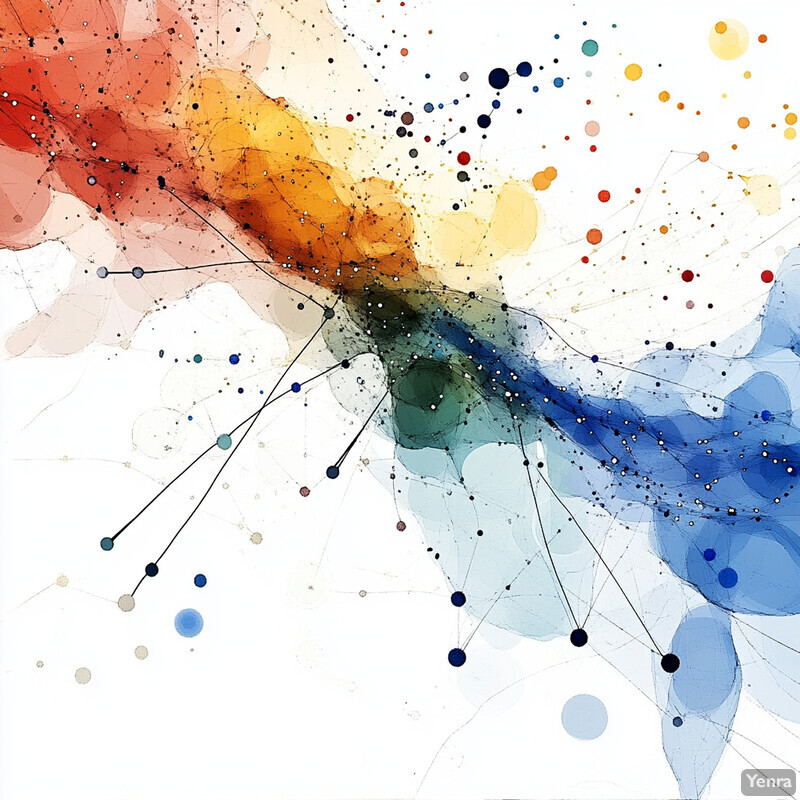
AI techniques can cluster drugs based on their chemical, structural, or pharmacodynamic similarities and similarly group diseases by their molecular characteristics. By examining these clusters, researchers can identify overlaps where a drug in one cluster could address a disease in another, thanks to shared underlying pathways. Such similarity-based approaches can highlight unconventional pairings that would not emerge from a straightforward target-based search. This pattern recognition step enriches the pool of repurposing candidates with options inspired by structural or functional analogies.
16. Federated Learning for Privacy-Preserving Insights
AI models trained on decentralized datasets—across multiple institutions—can identify repurposing candidates without sharing sensitive patient data, enhancing the breadth and diversity of the search.
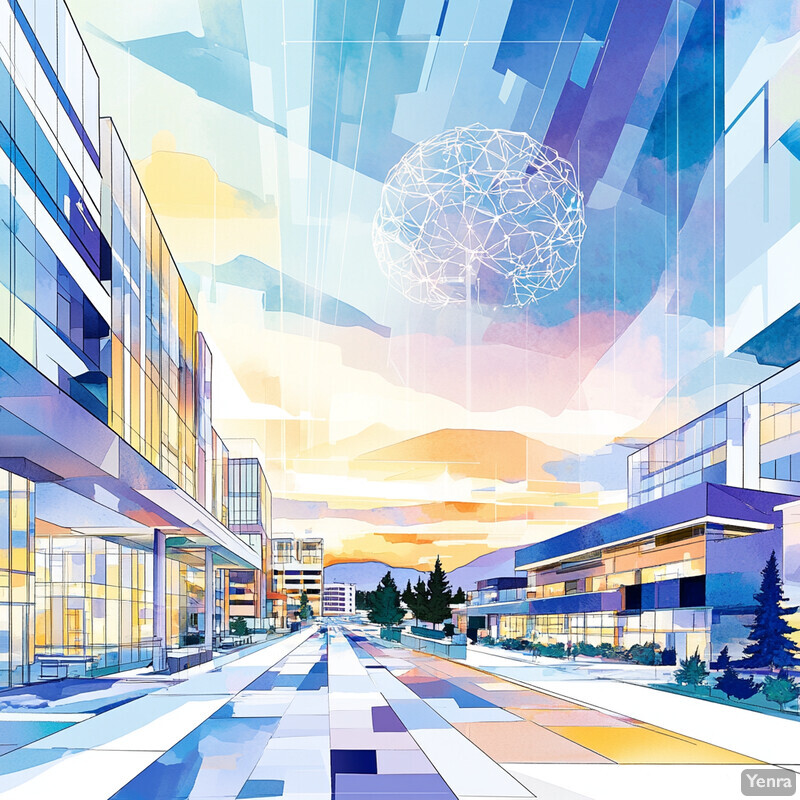
Federated learning allows AI models to be trained on decentralized datasets without pooling patient-level data in a single location. In a field where patient confidentiality and data security are paramount, this approach lets researchers glean insights from multiple hospitals or institutions while safeguarding privacy. By accessing a broader range of data, these models can identify repurposing candidates that are robust across diverse populations. This not only strengthens the evidence base for potential repurposed drugs but also ensures that recommendations are inclusive and generalizable.
17. Patient Stratification and Precision Repurposing
By using AI to subtype patients genetically or clinically, researchers can predict how certain existing drugs might be particularly effective in specific patient subsets, refining repurposing strategies.
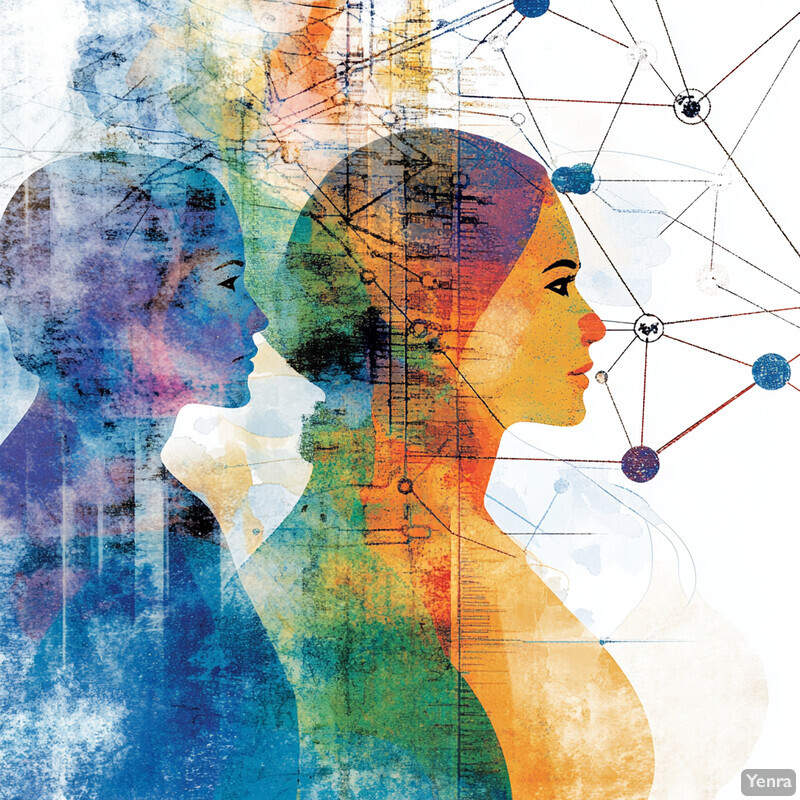
AI can segment patient populations into subgroups based on genetic makeup, disease stage, or biomarker profiles. By precisely defining patient subtypes, researchers can pinpoint which repurposed drugs are most likely to work for which group. This precision approach ensures that the right patients receive the right therapy, increasing efficacy and reducing unnecessary treatments. Tailoring repurposed drugs to patient subpopulations elevates the quality of care and maximizes the clinical impact.
18. Predictive Toxicology and Safety Profiling
Machine learning models can forecast how repurposed drugs would behave from a safety standpoint in different patient populations, reducing the risk and cost of clinical testing.
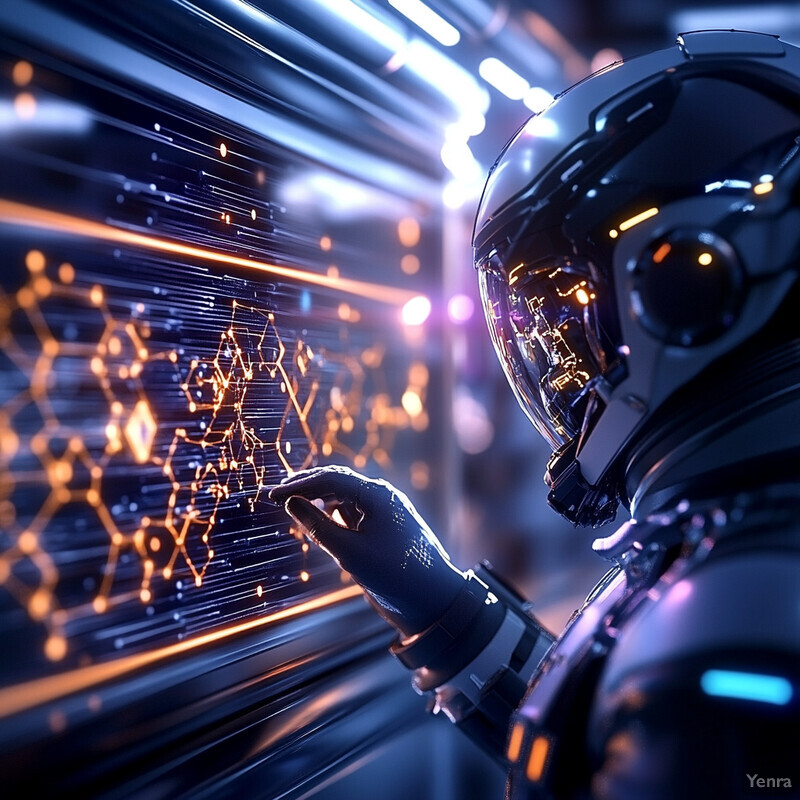
Before repurposed drugs can be used in a new indication, their safety must be confirmed. AI models help predict potential toxicity or adverse reactions by analyzing vast datasets of known drug-toxicity relationships and molecular properties. Machine learning can forecast whether a repurposed drug will pose risks in new patient populations or at higher doses. This predictive profiling ensures that only candidates with acceptable safety margins proceed to clinical validation, saving time and money while protecting patient well-being.
19. Clinical Trial Candidate Selection
AI tools can prioritize which repurposed candidates have the highest likelihood of success in clinical trials, streamlining the process and reducing wasted resources on low-probability attempts.
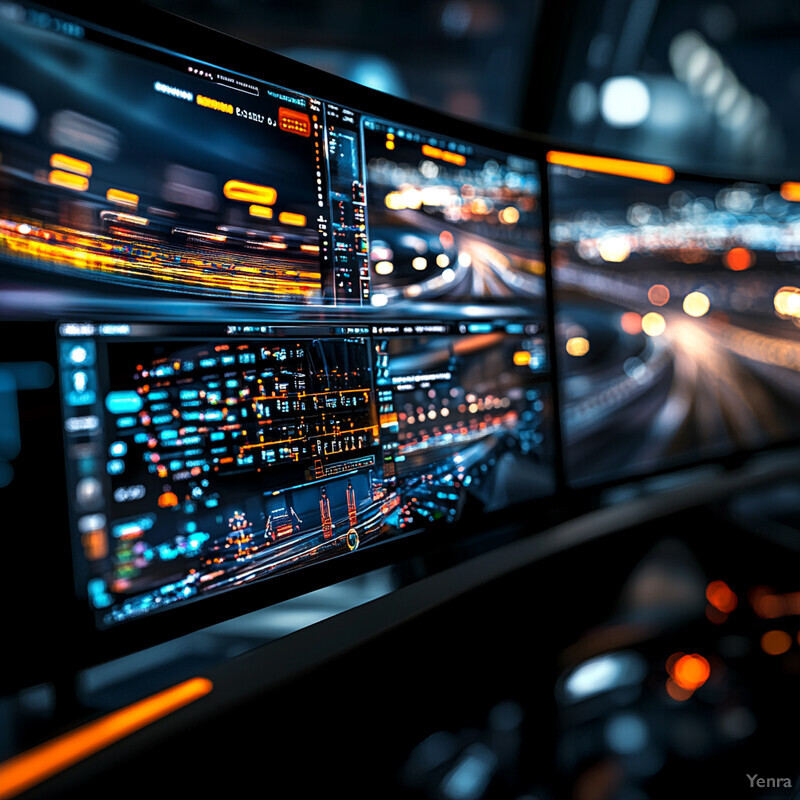
Running a clinical trial is expensive and time-consuming, so selecting the most promising repurposed drug candidates is crucial. AI tools can prioritize candidates based on a range of factors—mechanistic plausibility, preliminary efficacy signals, safety profiles, and patient population fit. By narrowing down the candidates to those with the greatest chance of success, AI reduces the likelihood of failed trials and speeds up the arrival of effective treatments to market. This strategic approach to candidate selection streamlines the entire drug development pipeline.
20. Continuous Learning Systems
AI systems that continuously update their knowledge from ongoing research, new clinical data, and patient feedback loops enhance the timeliness and accuracy of drug repurposing insights over time.
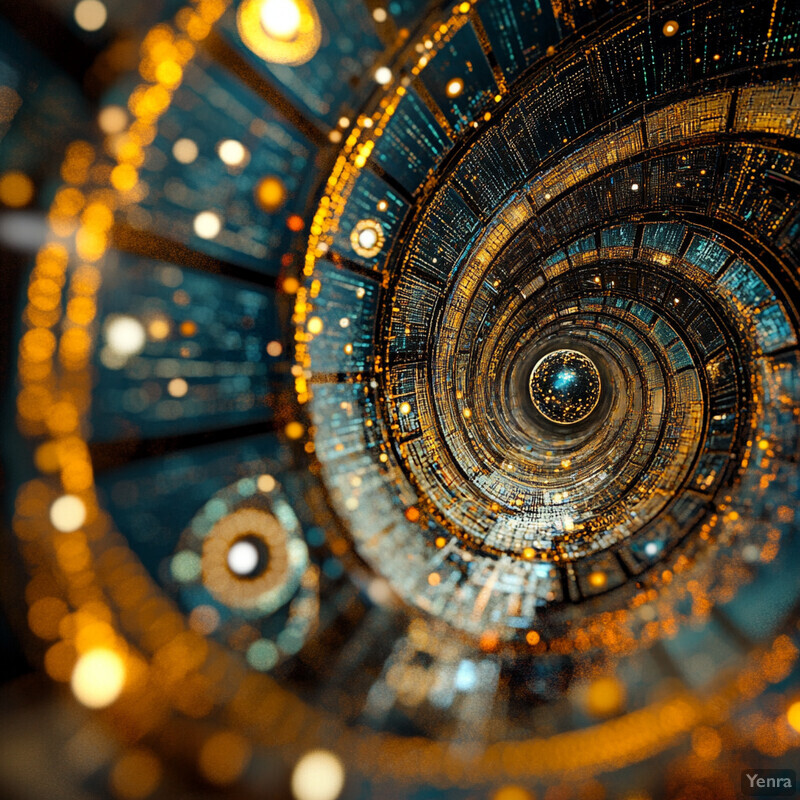
A key advantage of AI is its ability to continuously learn and adapt as new data becomes available. These systems can incorporate newly published research, update their models with recent clinical trial outcomes, and integrate fresh patient-level data. This iterative learning ensures that insights into drug repurposing remain current and reflective of the latest scientific understanding. Over time, this dynamic feedback loop enhances prediction accuracy, broadens the scope of discovery, and keeps the repurposing efforts cutting-edge and impactful.