Video: Drone Threat Detection
Song: Drone Threat Detection (Lyrics)
1. Enhanced Object Recognition Through Deep Learning
AI-driven deep learning models improve the accuracy and speed of recognizing unauthorized drones.
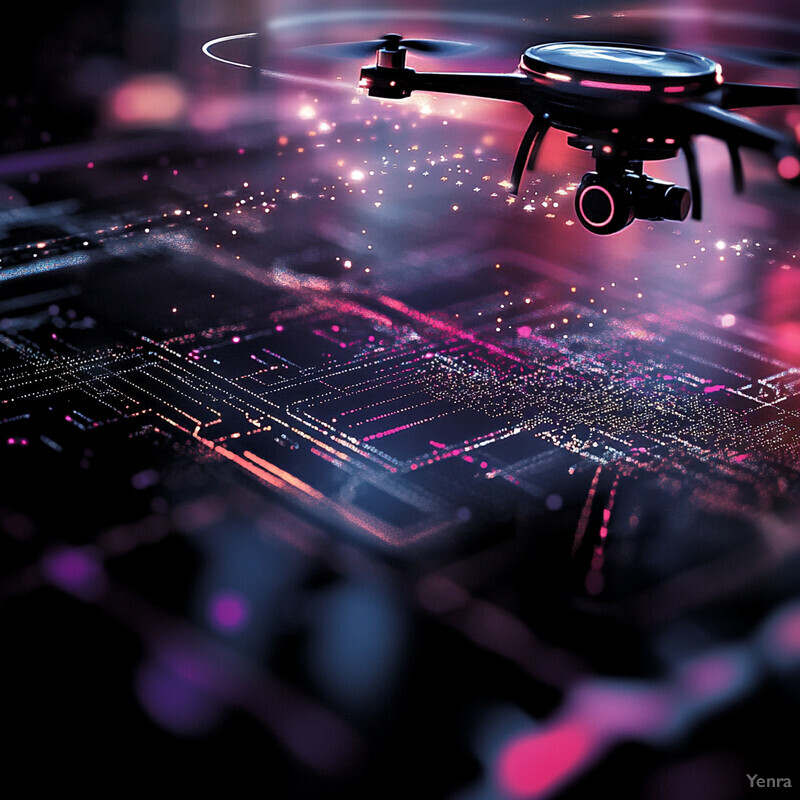
By leveraging deep learning architectures such as convolutional neural networks, modern threat detection systems can distinguish between various aerial vehicles with remarkable precision. This improvement stems from training models on massive datasets that include diverse drone shapes, sizes, and configurations, ensuring that subtle variations do not escape notice. As a result, security teams can rapidly identify and classify potential threats, minimizing false alarms and strengthening overall aerial perimeter defenses.
2. Real-time Drone Identification and Classification
AI systems now classify and identify drones almost instantaneously, streamlining threat assessment in live scenarios.
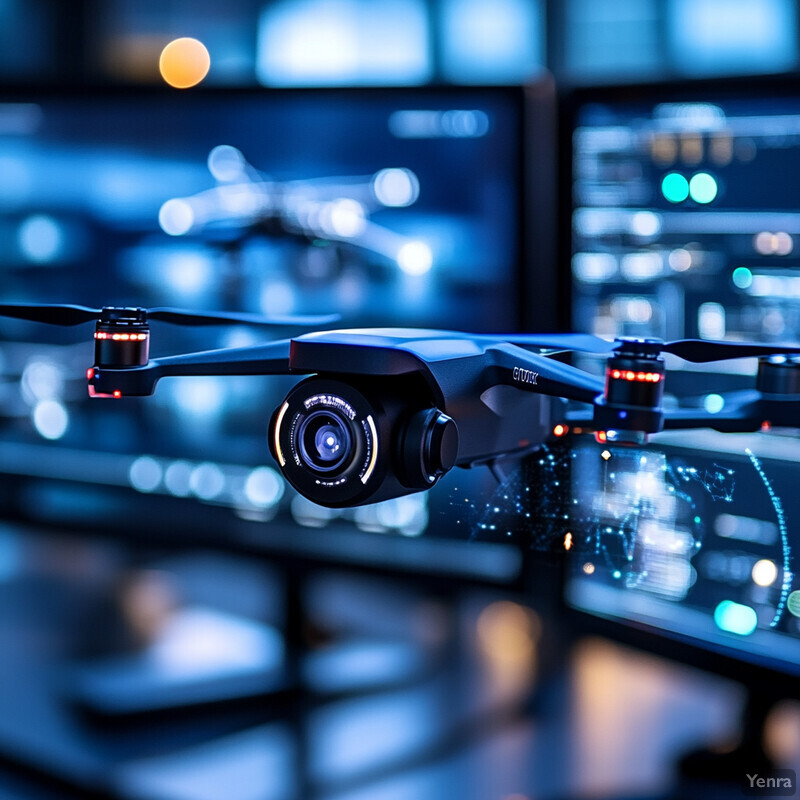
Integrating AI into real-time detection platforms allows security personnel to swiftly evaluate which drones pose a hazard. By quickly analyzing signatures like rotor sound frequencies, flight patterns, and silhouette outlines, the system can classify approaching UAVs within seconds. This rapid response provides operators with actionable intelligence, guiding decisions to intercept or neutralize harmful drones before they compromise sensitive areas.
3. Predictive Analytics for Drone Flight Paths
AI predictive models forecast drone trajectories, enabling proactive interception and countermeasures.
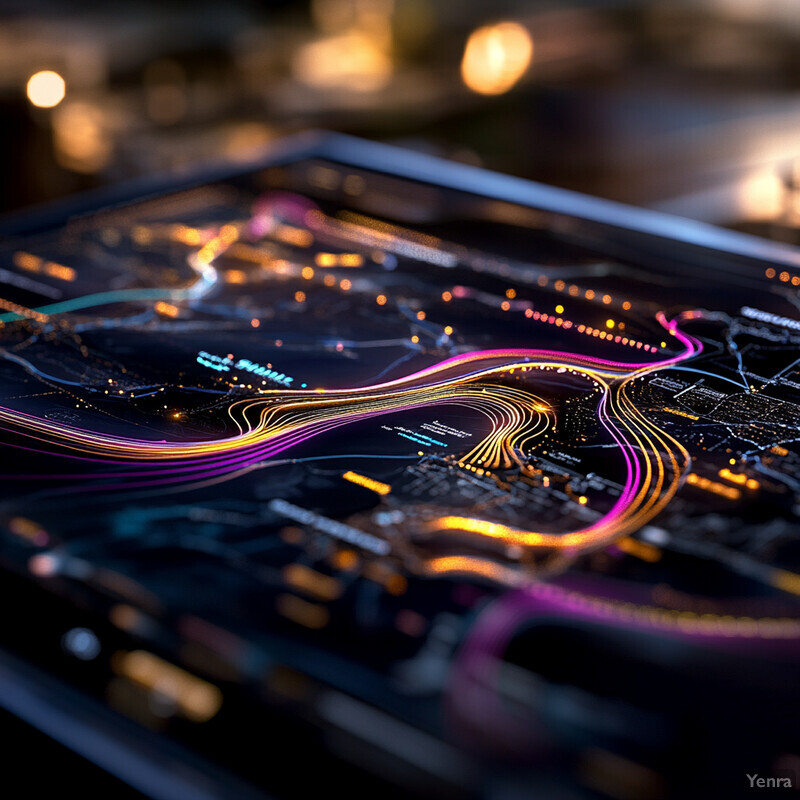
Through advanced machine learning techniques, AI systems can anticipate a drone’s most probable route based on historical flight patterns, wind conditions, known waypoints, and real-time telemetry. This foresight empowers defense teams to station countermeasures optimally and prepare responses well in advance. By predicting drone trajectories, security personnel can act decisively, securing high-value targets against potential attacks, smuggling attempts, or reconnaissance missions.
4. Signal Intelligence and Radio Frequency Analysis
AI-driven RF analysis tools filter and interpret complex signals to detect and track illicit drones.
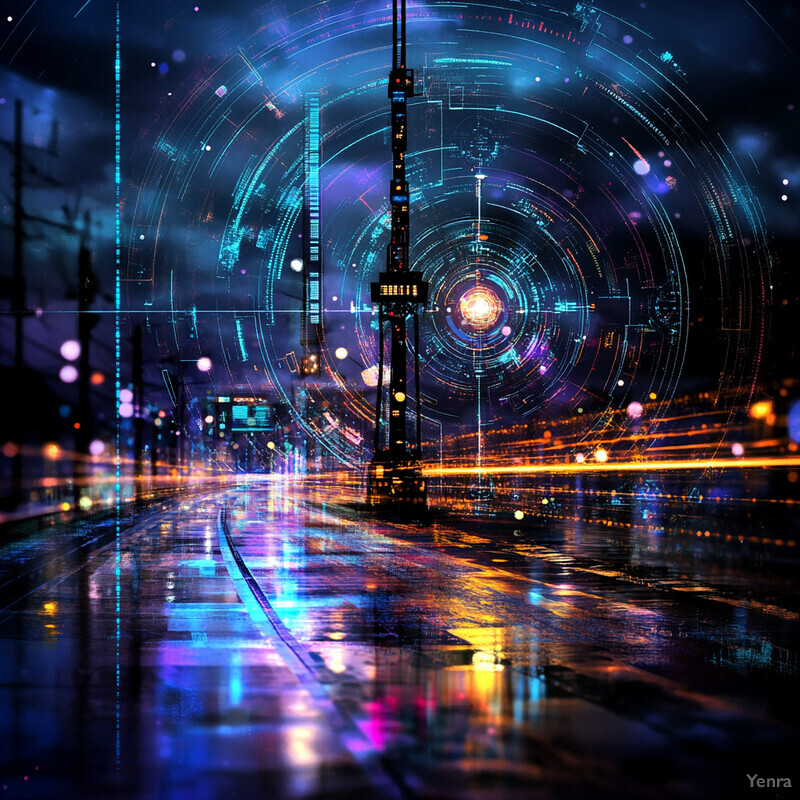
Modern threat detection solutions employ AI to sift through crowded electromagnetic spectra and pinpoint the unique radio frequencies used by drone controllers. By recognizing these signals and discerning subtle patterns even in noisy environments, the system isolates suspicious drones from benign radio traffic. This enables efficient identification of unauthorized UAVs attempting to remain stealthy, ultimately enhancing situational awareness and security posture.
5. Multi-Sensor Data Fusion
Combining data from multiple sensors with AI leads to more robust and reliable drone threat detection.
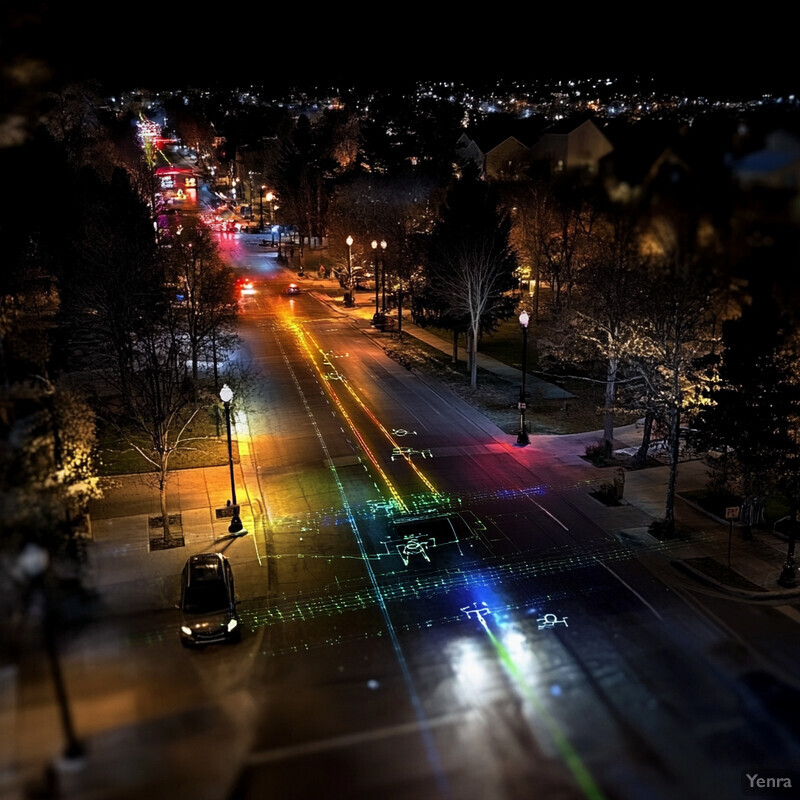
By uniting information from radar, LIDAR, infrared, acoustic, and visual sensors, AI-driven data fusion platforms present a comprehensive operational picture. AI algorithms correlate and compare sensor inputs, filtering out noise and reducing false positives. This integrated perspective allows security teams to make confident decisions based on cross-verified intelligence, ensuring they can respond quickly and effectively to any UAV threat, regardless of the conditions.
6. Autonomous Interception Protocols
AI-guided drones and systems can autonomously intercept and neutralize threat drones with minimal human intervention.
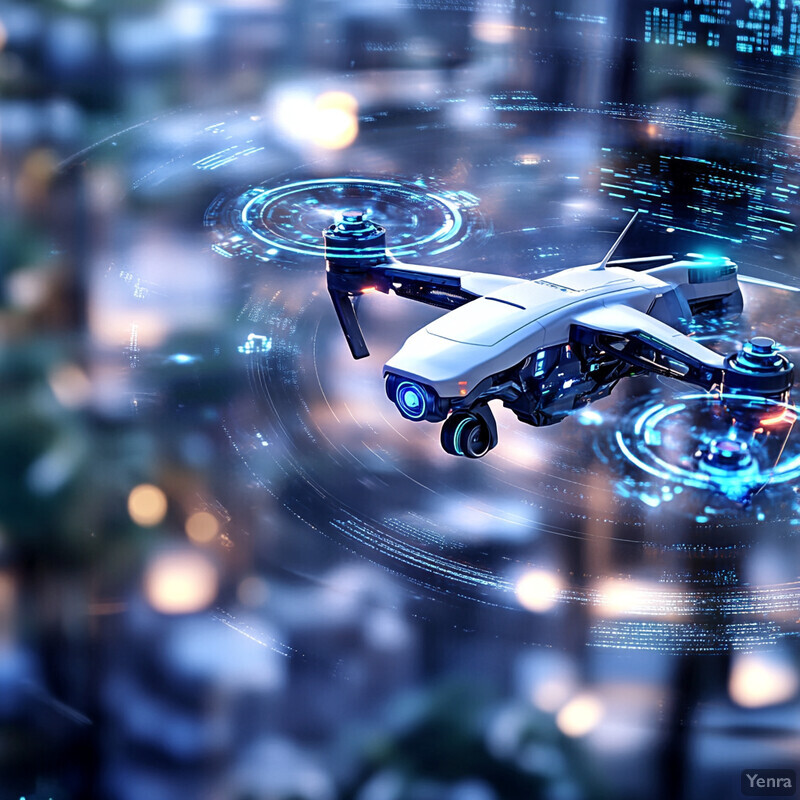
Artificial intelligence has enabled defensive UAVs to engage and outmaneuver hostile drones, employing tactics that are adaptive and efficient. These counter-drone systems leverage predictive modeling, swarm intelligence, and onboard vision to chase, jam, or disable intruders. As a result, human operators can focus on strategic oversight while AI-driven interception units handle tactical execution, reducing response times and improving overall defensive effectiveness.
7. Behavioral Pattern Analysis of UAS
Machine learning identifies suspicious drone behavior patterns, flagging unusual maneuvers and intent.
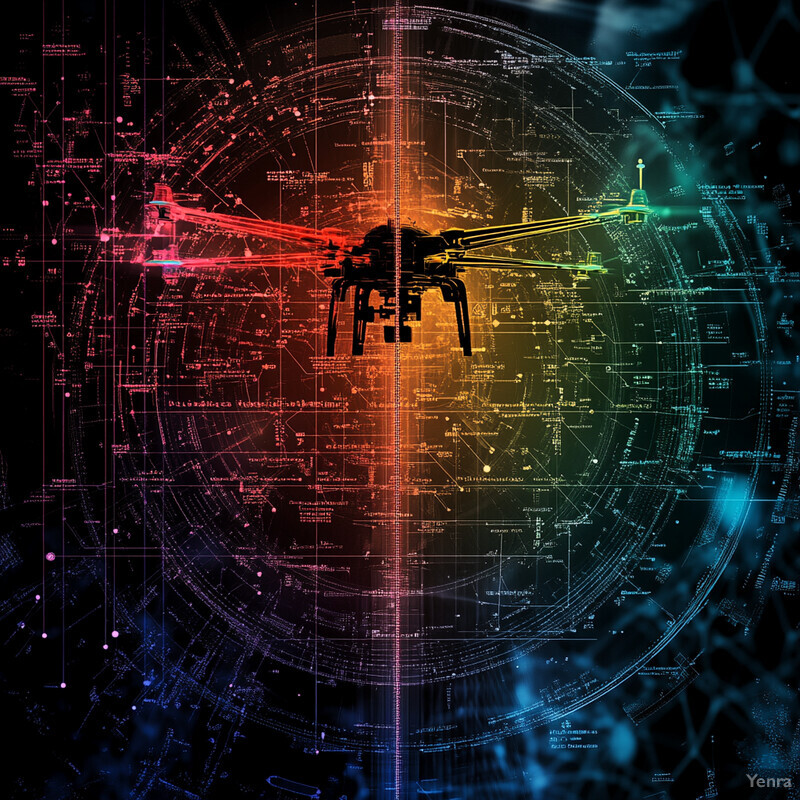
Just as cybersecurity systems detect abnormal network activity, AI-based drone detection tools can spot erratic flight behavior or suspicious hovering patterns. By building behavioral profiles from historical data, the system learns what constitutes normal UAV operations. Any deviation, such as lingering near sensitive zones, sudden altitude changes, or unpredictable flight paths, triggers alerts and focuses security resources where they are needed most.
8. Thermal Imaging Integration
AI uses thermal imaging data to detect drones that are obscured by darkness or camouflage.
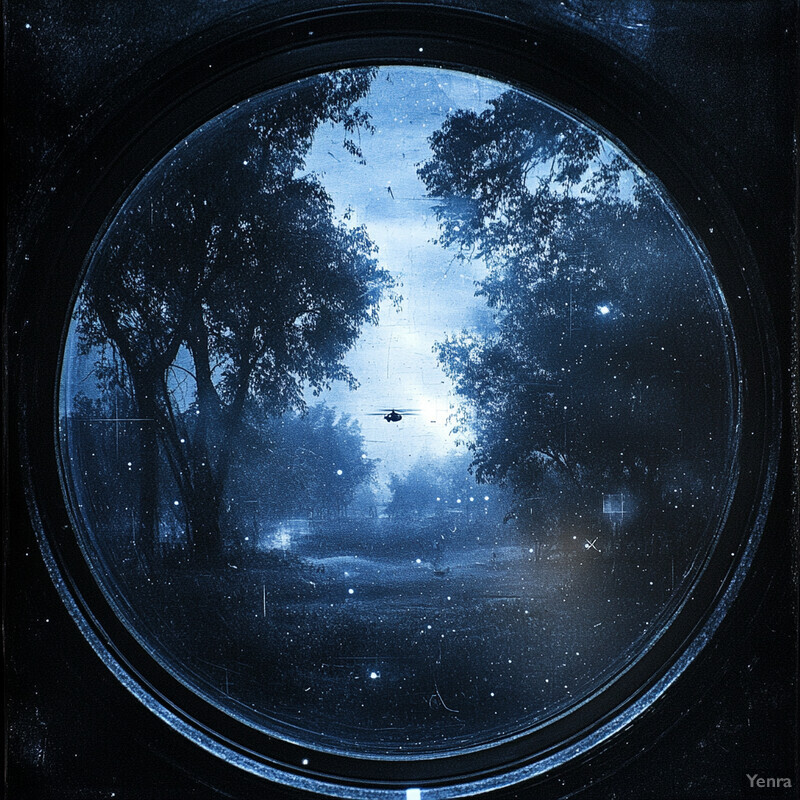
Thermal cameras can reveal heat signatures even when drones attempt to remain visually concealed. By applying AI algorithms to interpret these heat signatures, threat detection systems can identify UAVs in challenging environments—at night, in smoke-filled areas, or against a complex background. This capability ensures persistent surveillance and robust security, making it harder for malicious actors to hide their aerial platforms.
9. Anomaly Detection in Airspace
AI-driven anomaly detection highlights unexpected airspace incursions or unfamiliar drone types.
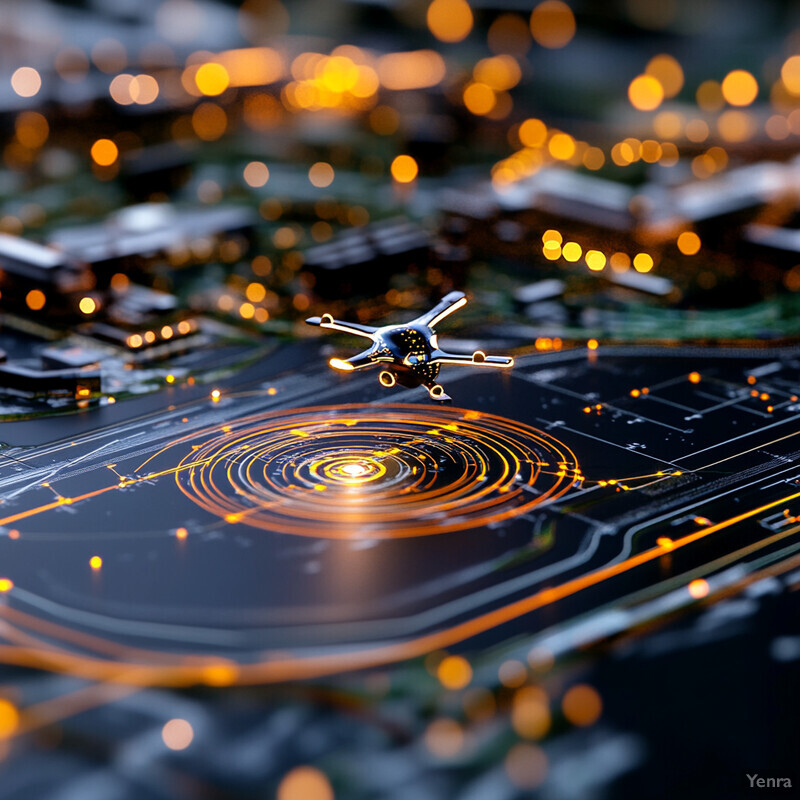
Modern air traffic is complex, with numerous legitimate drones and aircraft coexisting. By establishing a baseline of normal aerial activities, AI systems can pinpoint anomalies—be it an unfamiliar drone design, unusual altitude pattern, or abrupt velocity changes. This proactive approach ensures security operators are alerted immediately to potential threats, enabling swift intervention and reducing the risk of harmful outcomes.
10. Improved Edge Processing Capabilities
AI at the edge processes drone detection tasks locally for faster and more reliable threat assessment.
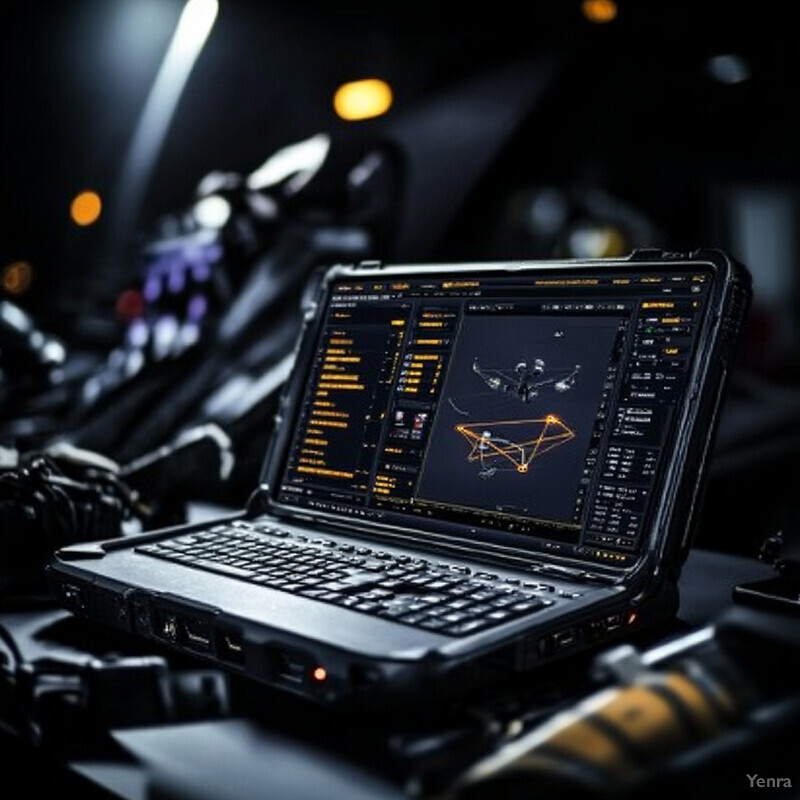
Deploying AI models directly on edge devices, such as local servers or smart cameras, minimizes the latency associated with sending data to distant cloud centers. This reduction in processing time ensures real-time detection and response. Additionally, edge computing enhances system resilience—if connectivity is lost, frontline units can still identify and respond to drone threats using pre-trained models, maintaining security even under challenging conditions.
11. Neural Network-Based Acoustic Signature Recognition
Advanced AI models analyze acoustic signatures to detect stealthy drones by their unique sound patterns.
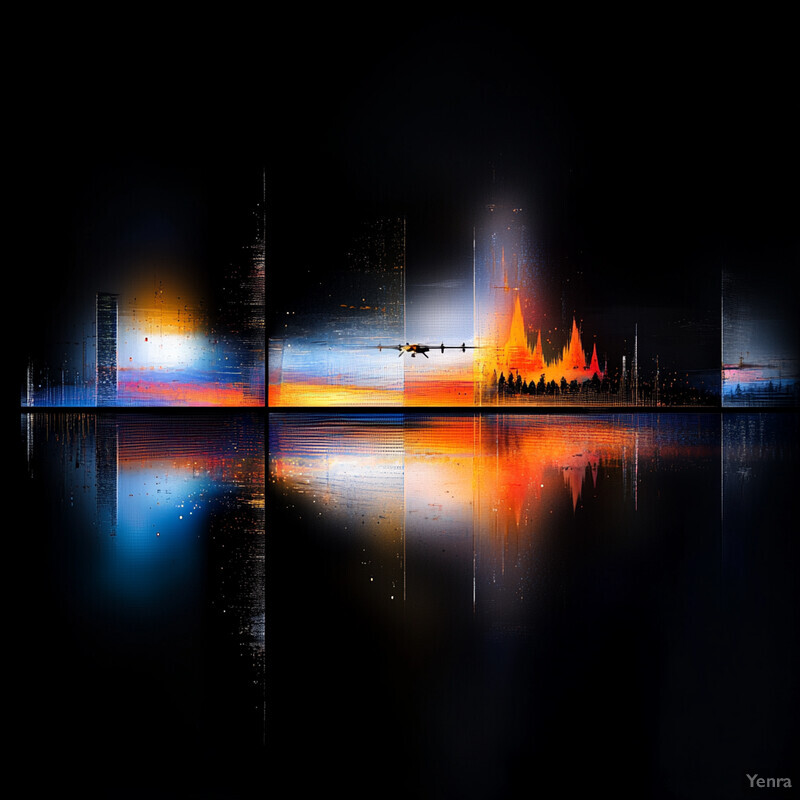
Even quiet drones produce subtle acoustic footprints. By training neural networks on a wide range of recorded drone sounds, AI can identify these patterns in noisy, real-world environments. Such acoustic analysis allows security teams to detect UAVs that may not be visible to cameras or radar, ensuring comprehensive surveillance coverage and strengthening the overall defense against aerial incursions.
12. Adaptive Learning for Evolving Threats
AI models continuously learn from new data, adapting to novel drone designs and tactics.
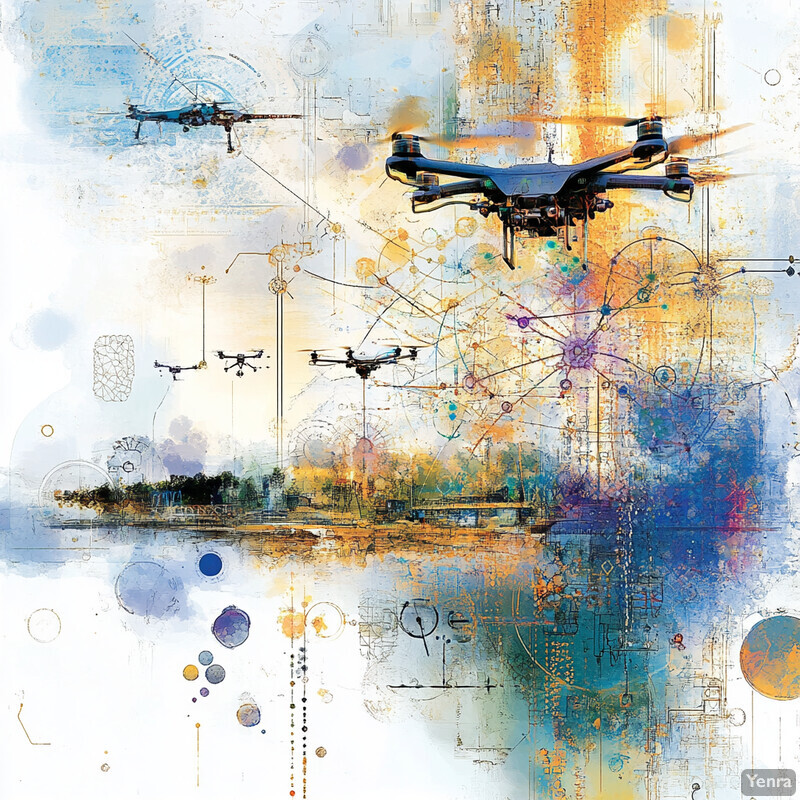
The drone threat landscape evolves rapidly, with malicious actors testing new configurations, flight behaviors, and stealth methods. Adaptive AI models update their detection parameters as they encounter fresh data. This continuous learning process ensures the system remains effective against emerging UAV technologies, never stagnating and maintaining a robust defense posture even as threats become more sophisticated.
13. Computer Vision for Camouflaged Drones
AI-powered computer vision algorithms help detect drones hidden behind foliage or disguised against complex backdrops.
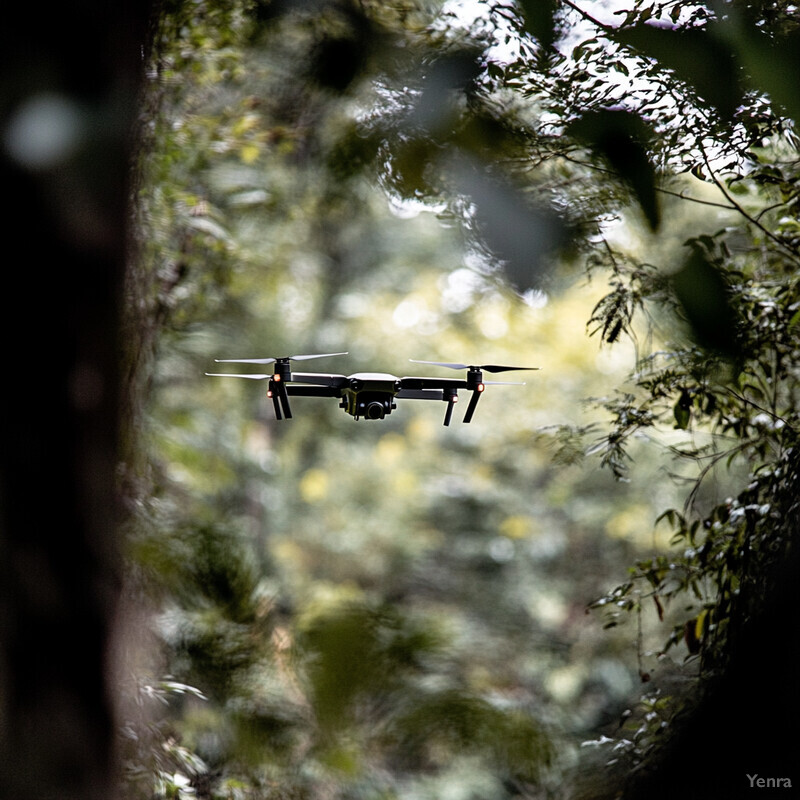
Through advanced object recognition and segmentation techniques, AI can detect drones that visually blend into their surroundings. Whether hidden in tree canopies or painted to match a building’s exterior, these UAVs can still be spotted by algorithms trained to find subtle discrepancies. This high-level vision capability ensures drones cannot exploit visual camouflage to evade detection, protecting critical infrastructure from covert surveillance or attacks.
14. AI-driven Drone Swarm Detection
AI techniques detect and track coordinated drone swarms, recognizing collective patterns of movement.
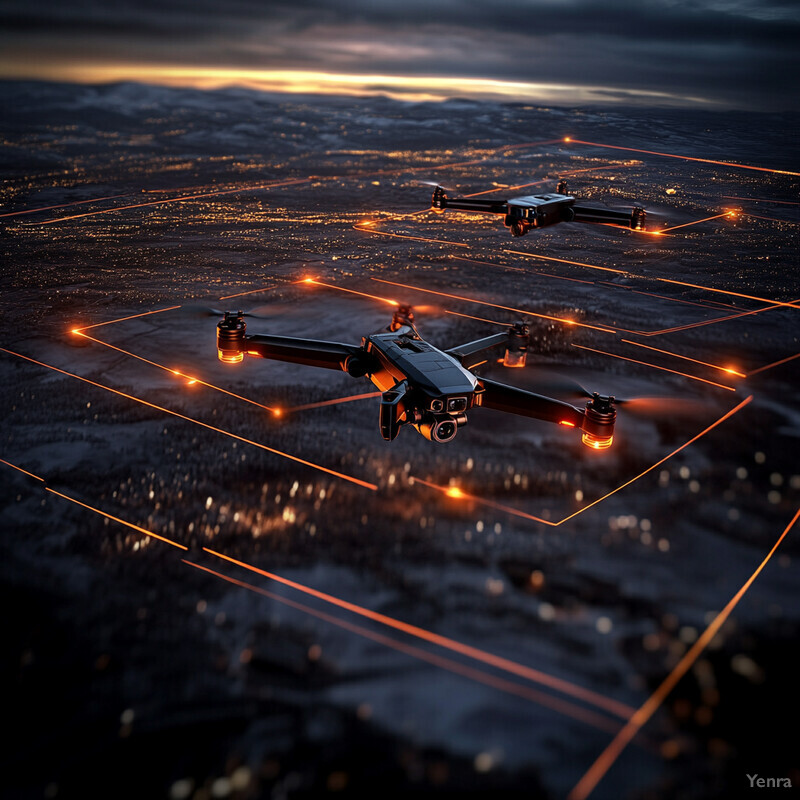
When multiple drones operate together, they can pose a formidable threat. AI-driven detection systems leverage cluster analysis and pattern recognition to identify swarms, understanding their formation, direction, and collective behavior. This intelligent grouping capability allows security forces to anticipate the swarm’s objectives and take timely, strategic countermeasures against a coordinated UAV assault.
15. Contextual Analysis and Threat Prioritization
AI contextually evaluates drones based on location, time, and intent to prioritize real threats over harmless aircraft.
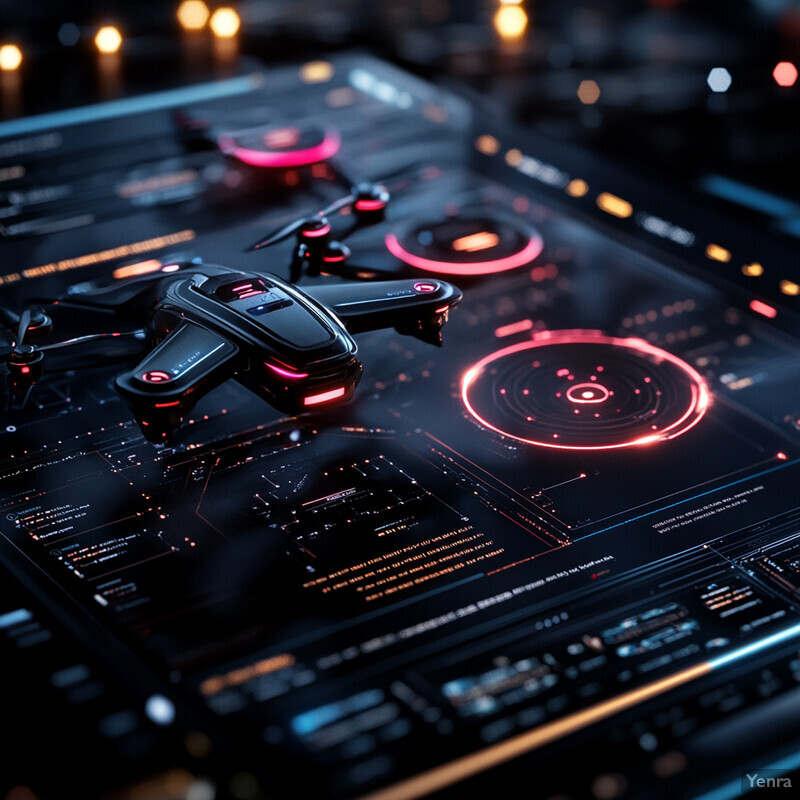
By factoring in the environment—such as proximity to critical assets, restricted zones, and events—AI-driven systems determine which drones demand immediate attention. They consider the time of day, flight authorization levels, and historical flight records to differentiate hobbyists or delivery drones from hostile actors. This contextual intelligence ensures that finite security resources are allocated where they matter most, enhancing both efficiency and safety.
16. Long-Range Optical Tracking with AI
Powerful AI-assisted optics track drones at extended distances, maintaining surveillance far beyond conventional ranges.
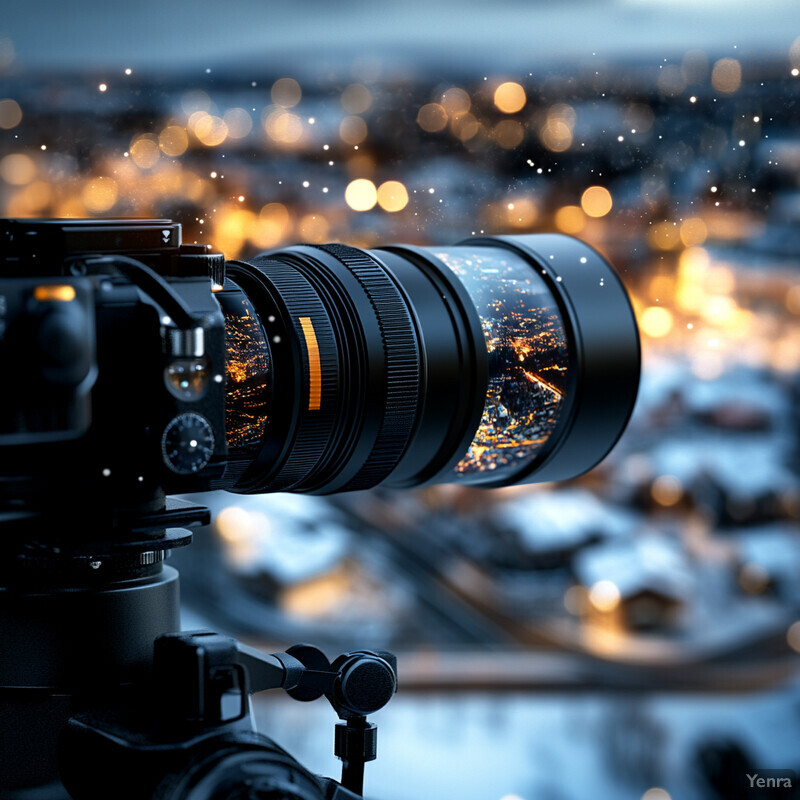
Modern lenses combined with AI image stabilization and enhancement techniques allow security teams to observe drones that would otherwise appear as indistinct specks. The AI refines blurry images, compensates for atmospheric distortions, and locks onto moving targets. This capability ensures early detection of distant threats, granting valuable time to orchestrate defenses, deploy countermeasures, or alert law enforcement authorities.
17. Deep Reinforcement Learning for Defense Strategies
Reinforcement learning techniques help AI refine defense strategies by simulating countless encounter scenarios.
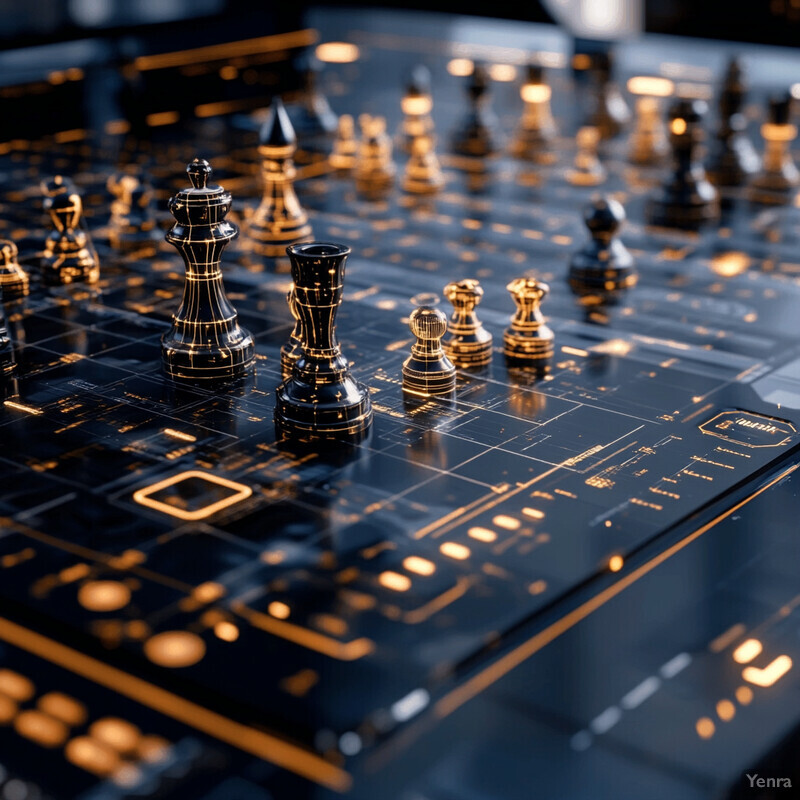
By running thousands of simulated interactions between friendly interceptors and hostile drones, reinforcement learning algorithms discover optimal tactics. These self-improving systems learn which maneuvers, interception angles, and electronic countermeasures yield the best outcomes. Over time, this iterative approach refines the defense strategy, ensuring faster and more decisive responses to evolving UAV threats.
18. Automated Incident Response Coordination
AI-based systems coordinate immediate, multifaceted responses to drone threats by triggering alarms, alerts, and countermeasures.
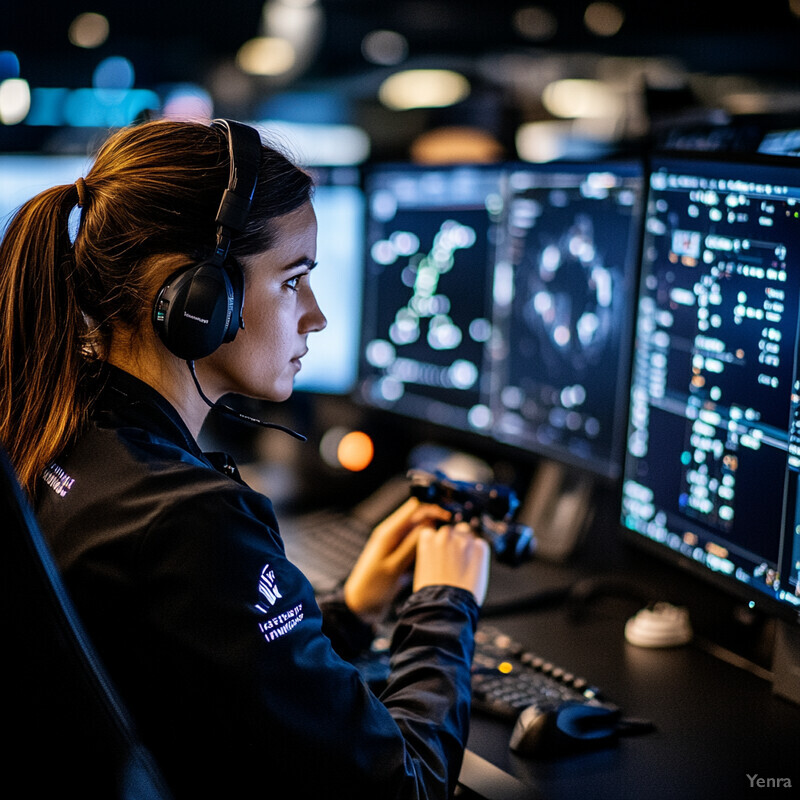
When a threat is detected, AI can orchestrate a swift and unified response. It may activate automated jammers, deploy interceptor drones, notify security personnel, and reroute patrols simultaneously. By harmonizing these actions, the AI reduces decision latency, ensuring a well-synchronized defense that prevents the attacker from exploiting any security gap during a critical moment.
19. Data-driven Vulnerability Assessment
AI evaluates past drone incursions to identify weak points and inform future security improvements.
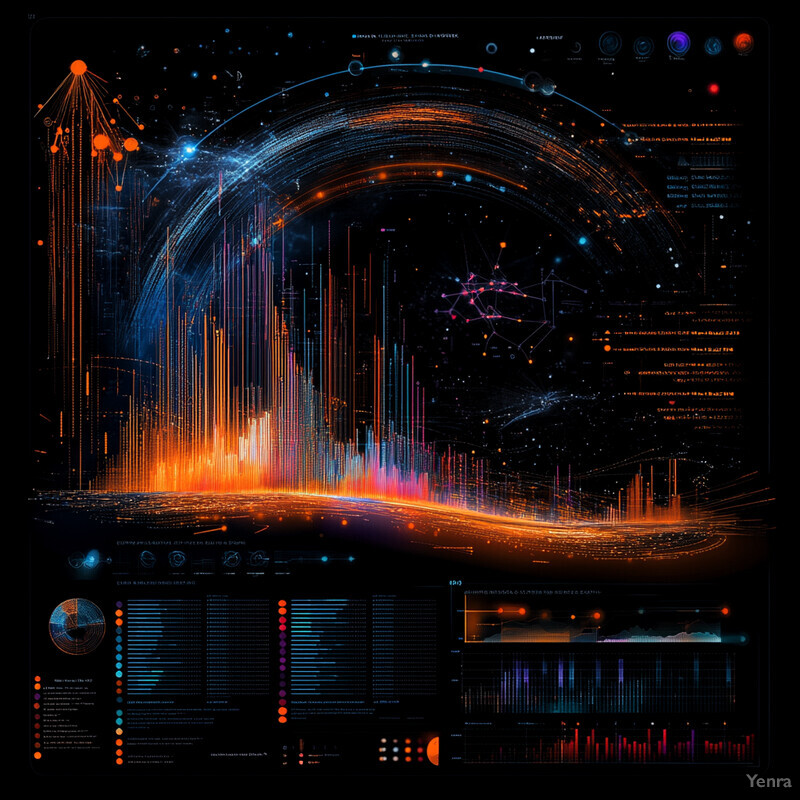
By analyzing historical data on drone sightings, intercepted threats, and false alarms, AI systems pinpoint patterns in security vulnerabilities. Perhaps certain blind spots or time windows are exploited more frequently. By illuminating these weaknesses, AI-guided assessments provide valuable recommendations—introducing new sensors, adjusting patrol schedules, or bolstering defenses at strategic perimeters—thus continuously strengthening the aerial security framework.
20. Enhanced Situational Awareness Through Augmented Reality
AR interfaces powered by AI overlay drone detection data onto real-world views, helping operators assess threats quickly.
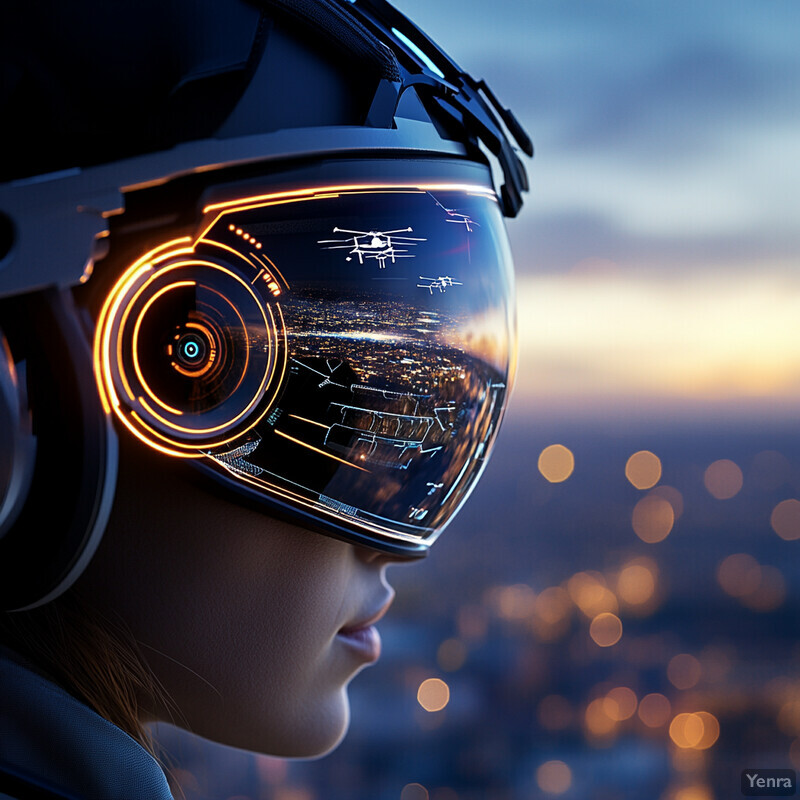
Augmented reality glasses or head-up displays, fed by AI detection systems, superimpose drone positions, flight paths, and threat levels onto the operator’s field of vision. This intuitive interface streamlines decision-making, allowing security personnel to visualize and respond to threats without toggling between screens. With crucial information readily available in their line of sight, operators are empowered to act decisively and efficiently.
Drone Threat Detection Song Lyrics
(Intro)
Scanning the horizon, AI’s got our back
Drones on the radar, we’re staying on track
Twenty ways we’re stepping up, no time to slack
In the digital skies, we’re ready to attack
(Verse 1)
Deep learning eyes see shapes in the dark
Object recognition hitting every mark
In real-time we classify the threat on the fly
Predictive paths traced, drones can’t deny
RF frequencies filtered, no static in the code
Data fusion layers give a full episode
Autonomous interception, we send a reply
With tactics defined by AI supply
(Pre-Chorus)
Behavioral analysis, patterns we decode
Thermal imaging cuts through every shadow road
Anomalies spotted where no eye can see
Edge computing steps in, setting data free
(Chorus)
We got twenty ways to guard these skies
With AI shining through augmented eyes
We’re securing the perimeter, no surprise
A future of safety, watch the drones realize
(Verse 2)
Acoustic signatures reveal stealthy wings
Adaptive models learn as the data sings
Computer vision peels back natural disguise
Swarms detected as formation applies
Contextual cues rank the danger ahead
Long-range optics keep the fleet in our thread
Deep reinforcement refines our game
Automated response aligns our aim
(Pre-Chorus)
Vulnerability mapped with data at the helm
Augmented reality shows the whole realm
From the code to the field, we’re standing tall
With AI as our shield, we answer the call
(Chorus)
We got twenty ways to guard these skies
With AI shining through augmented eyes
We’re securing the perimeter, no surprise
A future of safety, watch the drones realize
(Bridge)
No threat too distant, no craft too small
Our systems evolve, break down every wall
From new designs to cunning stealthy art
These twenty ways tear deception apart
(Outro)
As the world spins on, we raise the bar
AI in command, the next-gen star
Drones beware, we’ve changed the game
With twenty methods known by name.