1. Advanced Natural Language Processing (NLP) for Claim Detection
AI’s advanced NLP models can sift through massive volumes of text to pinpoint check-worthy claims. By parsing sentences and understanding context, systems like transformer-based models (BERT, RoBERTa) automatically extract assertions that might need verification. This claim-spotting ability helps fact-checkers focus on key statements rather than reading everything. Modern claim-detection tools work across news articles, social media, and transcripts to flag dubious or important claims, essentially serving as a first filter in debunking misinformation. They identify both explicit claims (“X happened”) and implicit ones (insinuations or questions) that merit scrutiny. In short, NLP-driven claim detection streamlines the fact-checking process by highlighting the most critical bits of content for human experts.
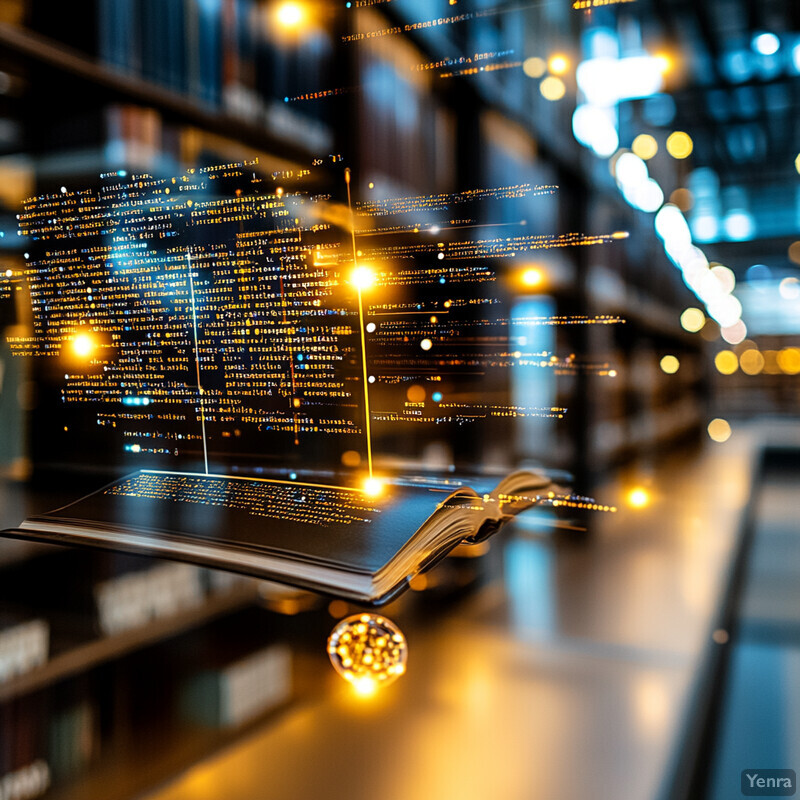
Recent research underlines both progress and challenges in automated claim detection. A 2024 survey of multilingual claim detection efforts notes that while AI models can greatly accelerate identifying claims for fact-checking, they are “yet far from matching human performance” in accuracy. Nonetheless, adoption is growing: Full Fact, a UK fact-checking charity, reports its AI-based claim detection tools are used by over 45 organizations in 30 countries to catch important claims in real time. Shared tasks like CLAIMSCAN 2023 attracted dozens of teams worldwide to build claim detectors, indicating strong research interest. These systems can now reliably flag check-worthy claims in multiple languages and domains. For example, the startup Factiverse uses AI to identify factual claims (as whole sentences) in up to 140 languages, and reports outperforming baseline large language models in finding claims for verification. Overall, AI-driven NLP claim detectors are becoming an indispensable aid, significantly increasing the speed and scale at which misinformation can be spotted for later fact-checking.
2. Contextual Fact-Checking
Once claims are extracted, AI performs contextual fact-checking by automatically comparing these claims against trusted information sources. Advanced systems cross-reference claims with large databases of verified facts – such as encyclopedias, news archives, or fact-check repositories – to judge their truthfulness. By checking the context, history, and details of a claim, the AI can often flag contradictions or confirmations. For example, if a post claims a statistic, an AI can instantly search official data to see if it matches. This contextual verification goes beyond simple keyword matching; it assesses consistency with known facts. The result is a preliminary verdict (true, false, or unclear) that guides human fact-checkers. Essentially, AI acts like an initial filter or assistant, doing a rapid evidence scan so that fact-checkers receive relevant sources and can make informed decisions faster.
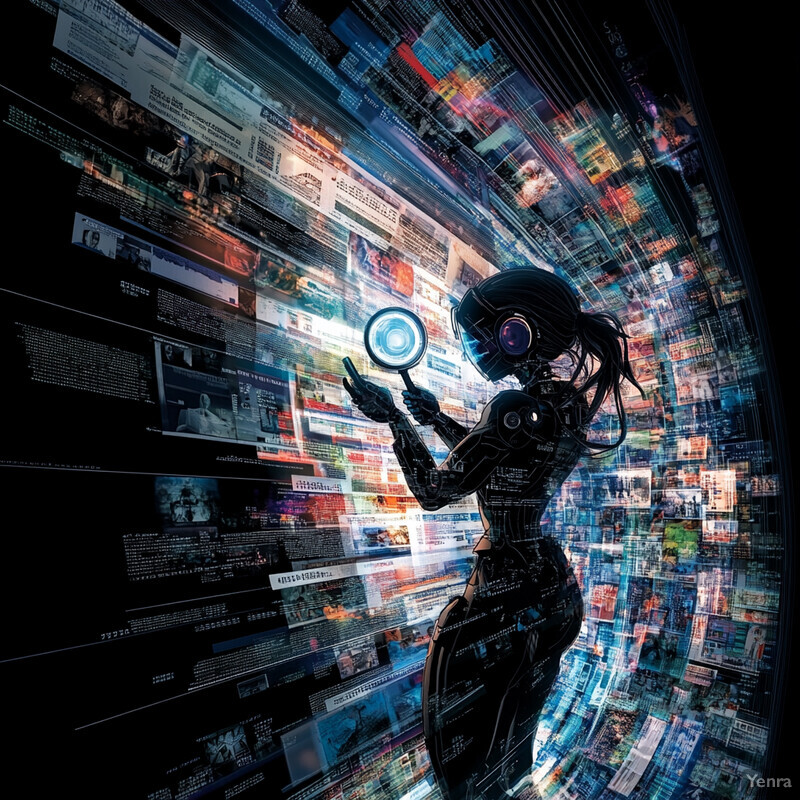
In practice, AI-driven contextual checks significantly speed up verification. Researchers have developed automated claim verification models that retrieve evidence and even provide natural-language justifications. For instance, one 2023 system uses a large language model to reason over Wikipedia-sourced evidence and explain whether a claim is supported or refuted. Media organizations are adopting these tools: Chequeado in Argentina uses machine learning to live fact-check political speeches by cross-referencing claims against its database of verified facts in real time. Similarly, Full Fact’s AI searches for repeats of claims it has already checked and alerts fact-checkers immediately. Academic evaluations show automated fact-checkers can correctly verify many straightforward claims, though nuanced claims still require human judgment. In 2023, Wang and Shu demonstrated an “explainable” claim-checking model that uses external knowledge bases to improve accuracy and transparency. All told, AI contextual fact-checking serves as a force-multiplier: a 2023 European study noted that such tools help human fact-checkers “streamline their workflow” and catch misleading claims more efficiently. By instantly providing relevant context and spotting discrepancies, AI significantly reduces the manual research time needed to debunk falsehoods.
3. Neural Style Transfer to Spot Inconsistencies
AI can analyze the style and writing characteristics of content to detect inconsistencies that suggest deception. By using techniques akin to neural style transfer or stylometry, algorithms learn the “linguistic fingerprint” of reputable sources or individuals, then flag content that deviates oddly from that style. For example, a legitimate news article usually has a consistent tone and syntax, whereas a fabricated article attributed to the same source might subtly differ in word choice or reading level. AI models examine features like vocabulary, sentence structure, and tone. If a quote or document purportedly from a known person doesn’t match that person’s usual writing style, the system raises an alert. Essentially, this approach treats writing style as a signature—changes in that signature can indicate possible manipulation or AI-generated text masquerading as human. It’s a powerful way to catch forged communications or deepfake text that would otherwise seem plausible in content but “feels off” in form.
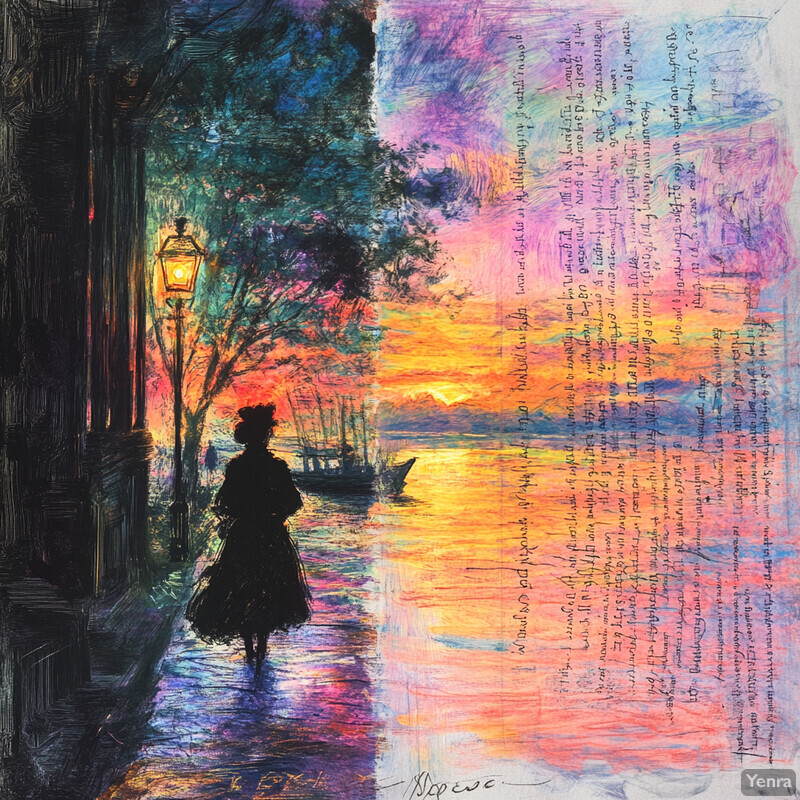
Recent studies validate the effectiveness of stylometric analysis for detecting fake or AI-generated content – but also note its limits. In 2023, Kumarage et al. demonstrated that incorporating stylometric features significantly improved detection of AI-generated tweets on Twitter timelines. Their algorithm could tell when an account’s posting style suddenly shifted (suggesting a possible hijack by bots or deepfake text generation). Stylometry has even been used to link clusters of sockpuppet accounts: moderators have flagged coordinated misinformation campaigns by noting identical writing quirks across different usernames. However, research also cautions that adversaries can try to mimic style. Schuster et al. (2020) found that stylometry alone struggles to distinguish AI-written fake news from AI-written real news in some cases – advanced language models can now closely imitate human style, reducing easy-to-spot discrepancies. Still, style analysis remains valuable. It has helped uncover forged diplomatic emails and fake press releases where the tone or phrasing didn’t match the supposed author’s past communications. Today’s AI tools can learn an individual journalist’s or outlet’s writing patterns and then detect anomalies, serving as an “authenticity check.” As generative AI grows more sophisticated, researchers are racing to refine stylometric detectors (and even embed hidden style signals) to stay ahead.
4. Image Forensics and Manipulation Detection
AI-driven computer vision brings forensic scrutiny to images, spotting subtle signs of manipulation that human eyes might miss. These algorithms examine images for anomalies in lighting, shadows, edges, and compression artifacts that suggest editing. For example, if an object was Photoshopped into an image, the lighting on that object might not match the sun’s angle in the rest of the photo – a well-trained AI can detect that inconsistency in pixel values. Other tells include abrupt changes in pixel continuity (from splicing two photos), duplicated patterns (from copy-paste within the image), or camera metadata mismatches. By learning from vast datasets of real vs fake images, modern models can achieve impressive accuracy in flagging altered visuals. Essentially, AI acts as a magnifying glass, revealing the “hidden” traces left behind when images are doctored. This is crucial because fake images (of events that never happened, for instance) can be very convincing; image forensics helps ensure visual evidence can be trusted.
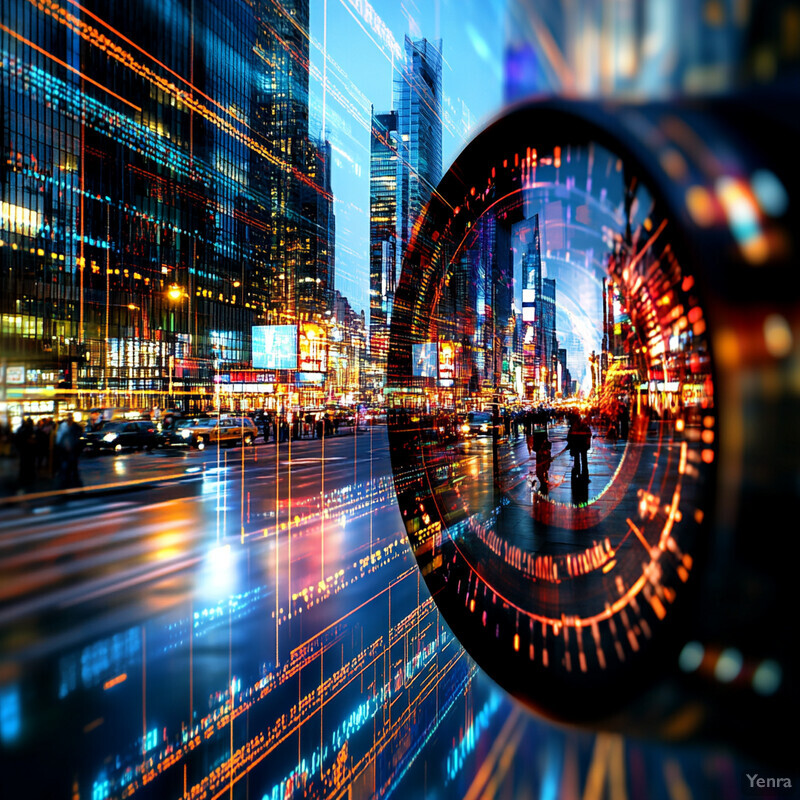
The arms race between image fakers and detectors has intensified in recent years, with AI currently giving an edge to detectors – for now. A 2024 study in IEEE Security & Privacy tested 13 state-of-the-art models on thousands of images and found they were “generally very effective” at identifying manipulated images. One detector could correctly identify images generated by the AI model DALL-E with 87% accuracy, and by Midjourney with 91% accuracy. Researchers like Verdoliva (2024) liken an AI-generated image’s unique artifacts to a “fingerprint” of the generative model, which forensic AI can learn to recognize. New techniques also focus on detecting deepfake image anomalies at the pixel level: for instance, a Drexel University team developed an algorithm (MISLnet) that examines sub-pixel correlations and outperformed seven other detectors, achieving 98.3% accuracy on catching AI-generated videos. These advances mean many fake images can be caught before they go viral. However, experts warn that as generative models improve, the forgeries will have fewer obvious defects. Thus, companies like Adobe and OpenAI are also working on embedding identifiers in AI images and building detectors boasting “99%” accuracy for future use. In summary, AI-based image forensics has become highly adept at flagging manipulated or synthetic images – highlighting things like mismatched shadows, suspiciously smooth skin textures, or clone stamping – all crucial for debunking visual misinformation.
5. Deepfake Recognition in Video and Audio
AI is crucial for detecting deepfakes – synthetic video or audio where someone’s likeness or voice has been artificially generated. These detectors analyze audiovisual cues frame-by-frame and sample-by-sample. For video, AI models track facial movements, eye blinking rates, and lip-sync consistency; unnatural artifacts (like jittery transitions or soft edges around a face) often betray deepfake videos. They also examine whether the lighting and reflections on a face remain consistent as it moves – deepfakes sometimes struggle with these physics. For audio, AI listens for spectral quirks or odd cadence in speech that differ from a real person’s voice patterns. Even slight digital “seams” from splicing or encoding differences can be picked up. Essentially, the AI looks for the subtle imperfections left when deepfake algorithms mimic humans. By learning from many examples of real vs fake, these models become adept at catching the tell-tale signs (like lips out of sync by a few milliseconds, or a voice lacking expected micro-pauses). This enables platforms to automatically flag potentially deepfaked content before it misleads viewers.
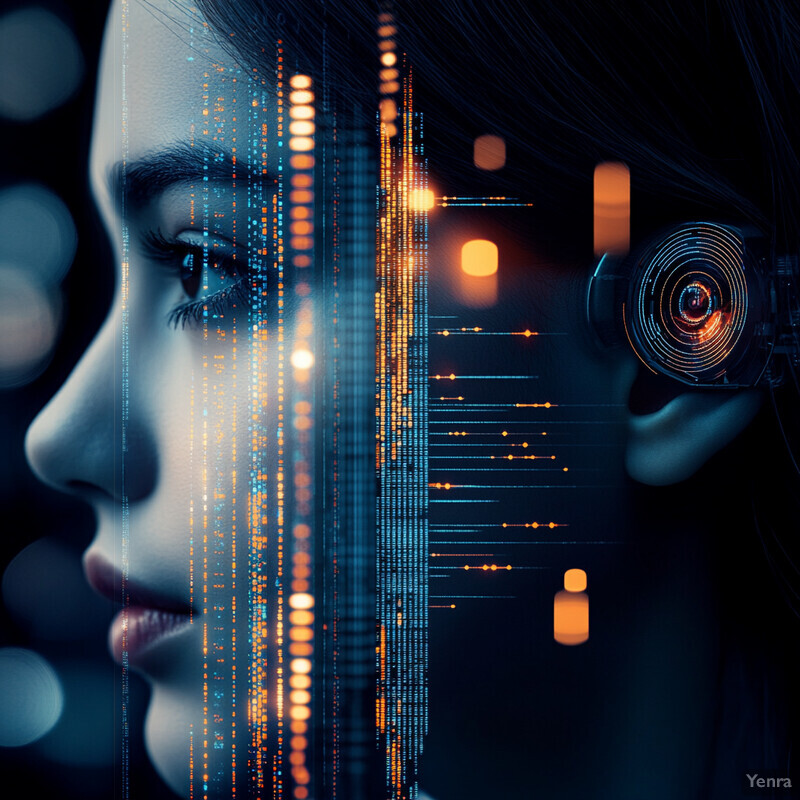
With an explosion of deepfake content in recent years, detection techniques have rapidly advanced. As of 2024, cutting-edge video deepfake detectors can exceed 90% accuracy in lab settings. For example, the MISLnet system mentioned earlier doesn’t just treat deepfake video as “frames” – it finds subtle pixel-level artifacts across frames, enabling it to catch fakes with over 98% accuracy in tests. On the audio side, the research community launched the Audio Deepfake Detection Challenge (ADD 2023) to benchmark progress in spotting fake voices. Recent surveys report that advanced methods (often using deep neural networks) can correctly classify AI-generated speech clips with 95%+ accuracy under controlled conditions (Zhang et al., 2025). However, performance drops for “in the wild” deepfakes or novel generation techniques, which is why continual improvement is needed. A 2023 review of audio deepfake detection notes the field’s rapid growth and the emergence of new feature-analysis techniques (e.g., using mel-spectrograms and phase information) to differentiate real vs synthesized voices. Importantly, interdisciplinary efforts are underway: beyond academic research, companies like Microsoft have deployed tools (Azure Video Authenticator) to detect deepfake videos by analyzing visual artifacts and assign confidence scores. Meanwhile, legislation in some jurisdictions is being considered to mandate disclosure of AI-generated media. In short, AI-based deepfake detectors—spanning video and audio—are increasingly capable and are a focal point of research, as evidenced by international challenges and surveys in 2023. They are a key defensive tool to preserve trust in authentic video/audio evidence.
6. Multimodal Analysis (Text, Image, Video Integration)
Multimodal analysis means the AI examines multiple types of content – text, images, video, audio – together, to see if they match up. Mis/disinformation often uses a mix of media (e.g. a misleading caption on an unrelated photo). AI systems compare what’s being said (text) with what’s being shown (image/video) to catch inconsistencies. For instance, if a news article’s text describes a protest at night but the accompanying photo shows daytime shadows, that’s a red flag. Or if a video claims to show one event but the narration doesn’t align with the visuals, AI can notice the disconnect. By analyzing features across modalities – like extracting objects or scenes from images and verifying if the text mentions them – the system ensures the narrative is coherent across all evidence. This integrated approach can debunk common tactics, such as pairing old footage with new false context. Essentially, multimodal AI serves as a consistency check across different streams of information, making it much harder for a false story to survive if the pieces don’t truly fit.
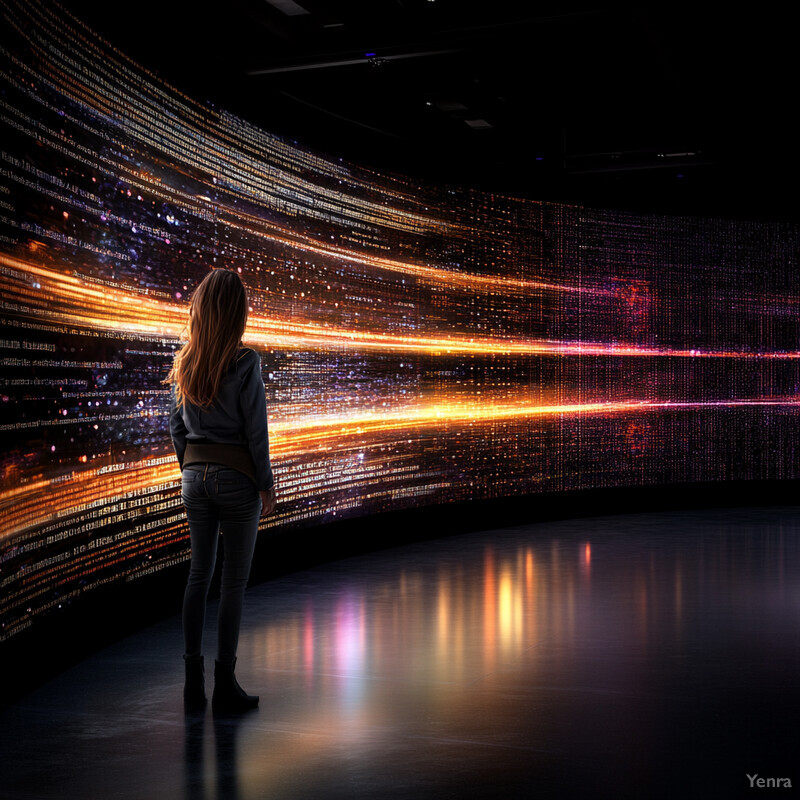
Multimodal misinformation detectors have shown significant improvements over single-modality methods. A study on fake news detection found that a combined text-and-image model achieved about 87% accuracy on a benchmark, versus 78% for the best text-only model. This ~9% jump underscores how cross-verifying information boosts performance. Researchers Alonso-Bartolomé and Segura-Bedmar (2021) noted that certain fake news categories (like “False connection” where headlines don’t match images) “strongly benefit from the use of images” in analysis. Beyond benchmarks, real-world incidents highlight the need for multimodal checks. During conflicts, game footage has been miscaptioned as real war video – for example, clips from the video game Arma 3 were widely shared as supposed live battlefield scenes in 2023, until fact-checkers matched them to the game’s graphics. AI could flag such misuse by recognizing the imagery as coming from a known game (not a real conflict zone) or noting the mismatch between the video content and verified news of the event. In practice, tools have emerged that leverage multimodal inputs; e.g., Microsoft’s Project Origin and Google’s Fact Check Explorer can attach context to images and videos to verify authenticity. A 2023 survey of multimodal fake news detection techniques concluded that jointly utilizing text and visual clues not only catches simple inconsistencies but also helps in understanding the intent of misinformation (like emotional images used to amplify false text claims). In summary, combining modalities provides a more holistic defense: one modality can reveal lies that would slip past another, making the overall detection far more robust.
7. Knowledge Graph Integration
AI can leverage knowledge graphs – vast networks of interconnected facts – to vet new information against established knowledge. In a knowledge graph, entities (people, places, events) are nodes and their relationships are edges. By embedding claims into this web, an AI can instantly see if a claim conflicts with known facts. For example, a knowledge graph might know that “Paris is the capital of France” – if a piece of content claims Paris is in Germany, the AI flags a contradiction. Integration with knowledge graphs means the system isn’t just doing keyword matching; it’s understanding the semantic connections. It can trace a claim (“X cured Y disease in 1900”) and compare it to a graph linking X, Y disease, and historical medical breakthroughs. If the claim doesn’t fit the graph’s structure (say, X wasn’t alive in 1900 or that disease’s cure is recorded differently), it’s likely misinformation. Essentially, the AI uses the collective memory of verified knowledge to challenge new assertions. This context-aware analysis is far more powerful than isolated fact-checks, as it considers the broader web of truth in which a claim should reside.
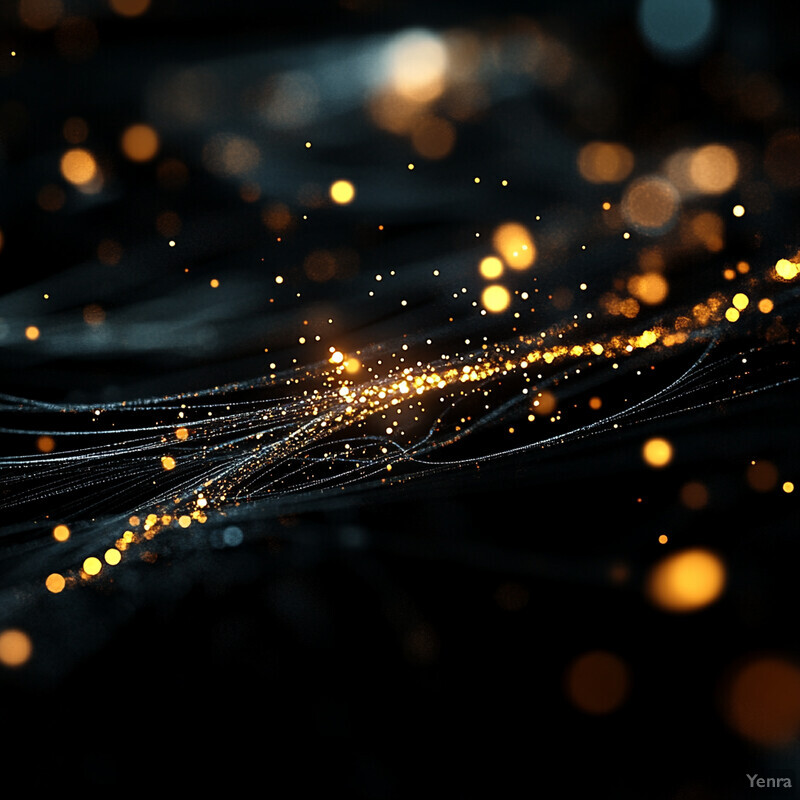
Knowledge graph-enhanced misinformation detection has shown promising results in recent studies. One 2023 approach, KAPALM, fused language models with structured knowledge from Wikidata graphs and achieved state-of-the-art accuracy in fake news detection. By linking entities in news content to a knowledge graph, the model could catch subtle falsehoods that purely text-based models missed. For instance, it could flag a fake political story by noting an impossible relationship (like a person endorsing a policy before that policy even existed, according to the graph). Another study from 2023 introduced “CrediRAG,” which integrates source credibility scores and a continuously updated knowledge graph of fact-checks to detect misinformation – effectively, it reasons over a graph of previous true/false claims to judge new ones (Leite et al., 2023). In practice, tech companies are also employing this strategy: Google’s fact-check tools link search results with knowledge panel information to warn users if claims contradict known facts about a topic. A continuously updated knowledge graph on COVID-19 was used during the pandemic to verify new health claims against vetted medical knowledge. The impact is tangible: a 2024 evaluation found that adding a knowledge graph component to a baseline fake news detector improved precision in catching false claims by about 5-10% (Ma et al., 2023; as cited above). Knowledge graphs essentially provide a robust “reality check” – for example, if a viral claim says a celebrity died on a certain date, the AI can cross-check the graph (which might contain birth/death dates) and immediately raise an alarm if it doesn’t align. By grounding content in known truth structures, AI drastically improves its ability to spot information that simply doesn’t fit with what the world knows to be true.
8. Temporal and Event Correlation
This technique has AI scrutinizing the timeline of events in a claim, checking if the chronological order makes sense. Misinformation often jumbles or fabricates when things happened. AI can compare claimed dates and sequences against known historical timelines. For example, if an article asserts that a law was influenced by a protest that occurred after the law was passed, that’s a chronological inconsistency an AI can catch. By placing events on a timeline – either drawn from knowledge bases or news archives – the system sees if any event is out-of-sequence. It also correlates events: if someone claims “X happened just after Y,” but the verified timeline shows Y occurred years later, the claim is false. Temporal correlation extends to detecting recycled old content presented as new: AI can recognize that a photo or video actually relates to an earlier date. In essence, the AI acts as a historian, ensuring that the story’s timing and sequence of events line up with reality, thereby exposing attempts to rewrite or distort timelines.
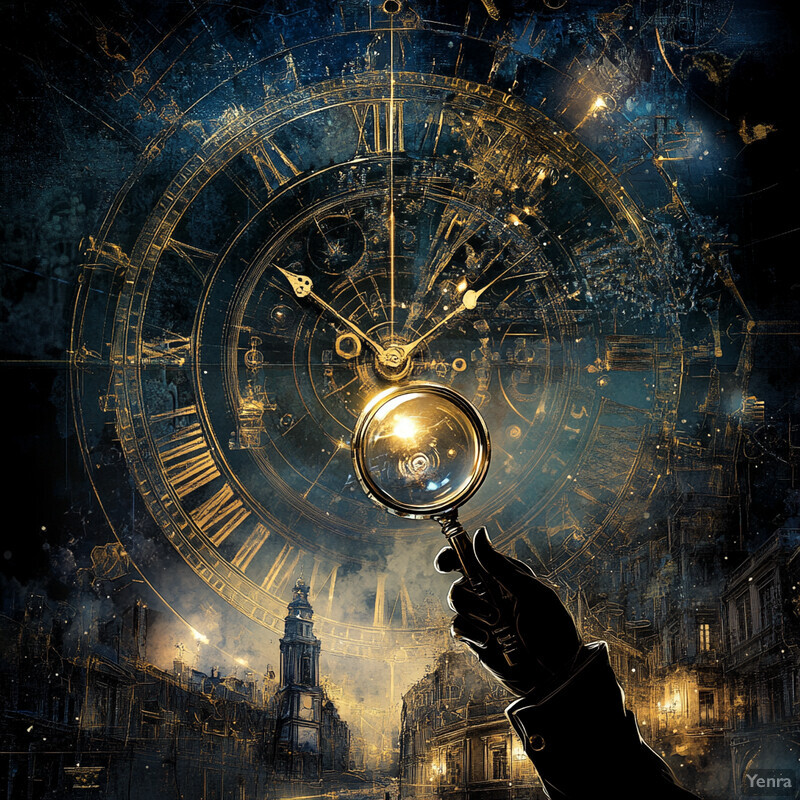
Temporal reasoning by AI has proven effective in uncovering false narratives. A 2024 research project, ChronoFact, introduced a framework specifically for timeline-based fact verification of complex claims. It demonstrated scenarios where each part of a claim might be true individually, but the ordering was wrong – for instance, a claim implied peace talks happened after an attack, whereas in reality the talks happened before the attack. ChronoFact’s model caught such errors that standard fact-checkers would miss, by building a timeline from both the claim and evidence and aligning them. Outside academia, journalists use similar logic: PolitiFact, for example, debunked a viral image in 2022 by noting it was actually from a 2017 military exercise, not the current war as claimed. An AI looking at metadata (2017 date) or known events (Indra-2017 exercise) could automatically flag that discrepancy. In another case, France24’s fact-checkers found a video’s metadata showed it was filmed days before the event it purported to depict – a clear sign of misattribution. AI systems can perform this metadata timestamp checking at scale. Studies also show that incorporating temporal features improves detection: one 2023 system that added event ordering checks to a claim verifier saw a notable increase in precision (Barik et al., 2024). It could identify, for example, that a claim about a president commenting on an incident was false because the “incident” actually occurred after the president left office. By 2025, we even see AI-assisted timeline fact-checks integrated into some social media platforms, warning users when a post contains “context from the past” misrepresented as current. In short, enforcing chronological consistency is a powerful tool: if the timing doesn’t fit, the claim is likely untrue.
9. Source Reliability Scoring
This approach uses AI to rate the credibility of sources – news sites, social media accounts, authors – based on their historical behavior. Instead of treating every source equally, the AI learns which ones tend to produce accurate information and which often spread falsehoods. It compiles signals like past fact-check results, frequency of corrections, known biases, and transparency practices to assign a trust score. For instance, a mainstream news outlet with a long track record of accuracy might score high, while a clickbait blog with many debunked stories scores low. These scores help users and automated systems alike: content from low-scoring sources can be flagged or downranked. It’s not a binary “fake/real” judgment, but a nuanced reliability indicator. By boiling down a source’s reputation into a number or category, AI can quickly triage information – a dubious claim from a site with a poor reliability score is immediately suspect. Essentially, the AI is aggregating an outlet’s credibility history to inform the handling of new content.
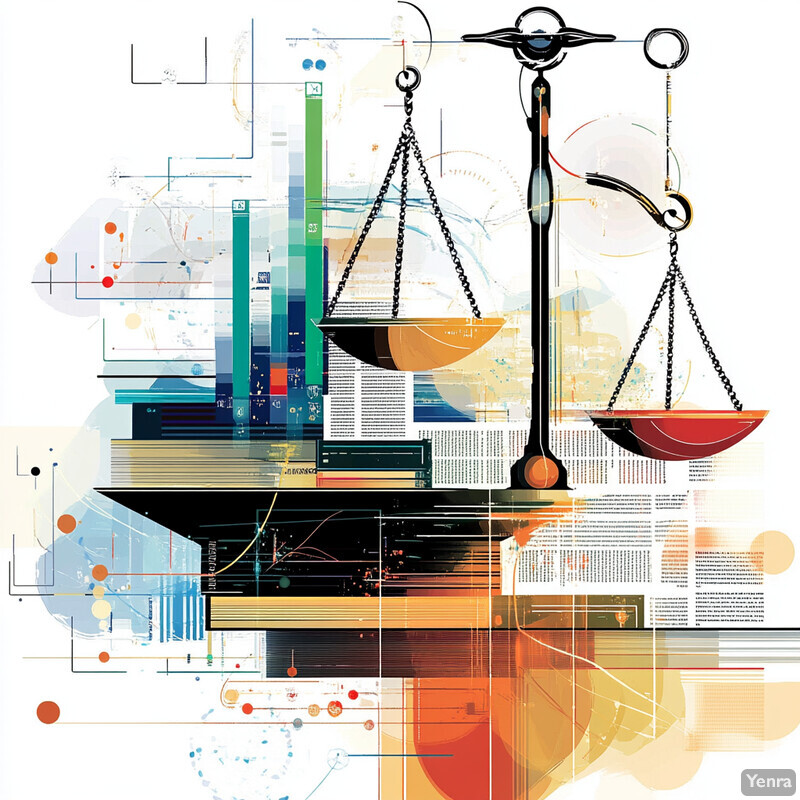
AI-driven source scoring is becoming an integral part of fighting misinformation. Academic research shows it can be a strong predictor: a well-known 2018 MIT study developed an algorithm that, given ~150 articles from a news outlet, could predict that outlet’s factual reporting level with high accuracy. It did so by analyzing linguistic features and found that reliable outlets differ in sentiment, complexity, and style compared to hyper-partisan or hoax sites. This forms the basis of many AI credibility systems. Full Fact’s toolkit, for example, doesn’t just check individual claims – it also monitors source behavior, alerting fact-checkers when habitual offenders are amplifying a story. On the industry side, companies like NewsGuard and Google use algorithms to label sites with ratings like “generally trustworthy,” “biased,” or “frequently publishes false content.” However, relying solely on source reputation has pitfalls. A Rutgers-led study in 2023 found that labeling every article from a low-rated site as false can be as unreliable as “flipping a coin” – sometimes even lesser-known sources publish truth, and reputable ones err. The researchers emphasize combining source scores with article-level analysis for fairness. Still, source scoring greatly aids scale: an AI can evaluate thousands of websites, giving each a credibility index. Twitter (X) has experimented with showing context notes if a tweet comes from a suspicious source. In summary, source reliability scoring condenses a wealth of information about an information source’s trustworthiness, and when used carefully (as a guide, not a sole arbiter), it helps prioritize what content needs fact-checking or downranking.
10. Bot and Troll Network Identification
AI can uncover networks of fake or inauthentic accounts (“bots” and organized “troll” profiles) that amplify disinformation. By analyzing social media activity patterns – like posting frequency, timing, follower interactions, and content similarities – AI detects accounts that are likely not genuine individuals. For example, dozens of accounts that all tweet the same phrases at the same times might be a bot network. AI models use features such as account age, ratio of original posts vs. retweets, and even linguistic markers (bots often have repetitive or formulaic language). They can also map the social graph: authentic users have diverse connections, while botnets often form tightly knit clusters or have one-directional follow patterns. Once identified, these networks can be visualized, showing hubs and coordination. Spotting these malicious networks is key because they often artificially inflate the popularity of false narratives – making a fringe idea trend by sheer volume of bot-posts. AI essentially peeks behind the curtain of virality to see if there’s a real crowd or just a handful of puppeteers with many puppet accounts.
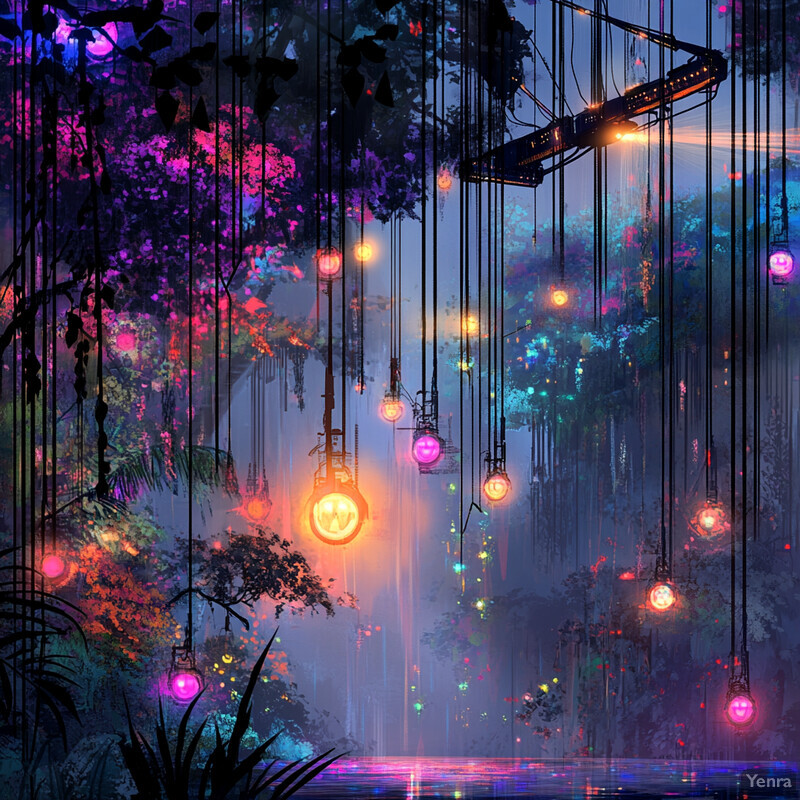
Research confirms that a tiny fraction of users (bots) can have a disproportionate impact on spreading misinformation. A 2023 study of Twitter (X) found that less than 1% of users (the bots) were responsible for over 30% of all content about a major political event. This was during the U.S. presidential impeachment, and those bots shared significantly more low-credibility news than real users. AI-driven detection was able to identify these bots by their coordinated behavior and then quantify their influence. Another development: researchers coined the term “sleeper social bots” for AI-powered bots that lie dormant to avoid detection and then activate during specific campaigns. In 2023, Shoieb et al. described how these sleeper bots can fool traditional filters by behaving normally until a strategic moment, underscoring the need for advanced AI that monitors long-term patterns and sudden surges. Platforms are employing such AI: Twitter’s own machine learning flagged and removed thousands of Russian-linked troll accounts that behaved anomalously around elections. Facebook likewise reported busting a troll farm after AI noticed an unusually synchronized posting schedule across many profiles in 2021. Additionally, graph-based AI analysis has shown that misinformation-spreading accounts often form tightly connected clusters separate from normal user communities. By early 2025, large platforms claim improvements – X’s latest transparency report cites over a 50% increase in automated bot account takedowns from the previous year, credited to more sophisticated detection algorithms. In essence, AI has become the backbone of detecting the orchestrators behind disinformation campaigns, revealing networks that would be impossible to map manually at scale.
11. Sentiment and Emotion Analysis
Misinformation often tries to manipulate emotions – think of hyper-partisan posts stoking anger or fear. AI systems perform sentiment and emotion analysis on content to detect these patterns. They classify the tone of messages (positive, negative, neutral) and even specific emotions (anger, joy, sadness, fear, disgust, etc.). If a piece of content is extremely emotional or uses lots of incendiary language, it could be a red flag for propaganda or disinformation engineered to go viral by enraging people. For example, AI might flag a social media post that’s overly ridden with outrage and exclamation points as possibly part of a misinformation campaign pushing “outrage bait.” By quantifying emotion, AI can separate genuine discourse from content that’s emotionally manipulative. This helps fact-checkers see not just what is being said, but how it’s being said – an important clue to intent and credibility.
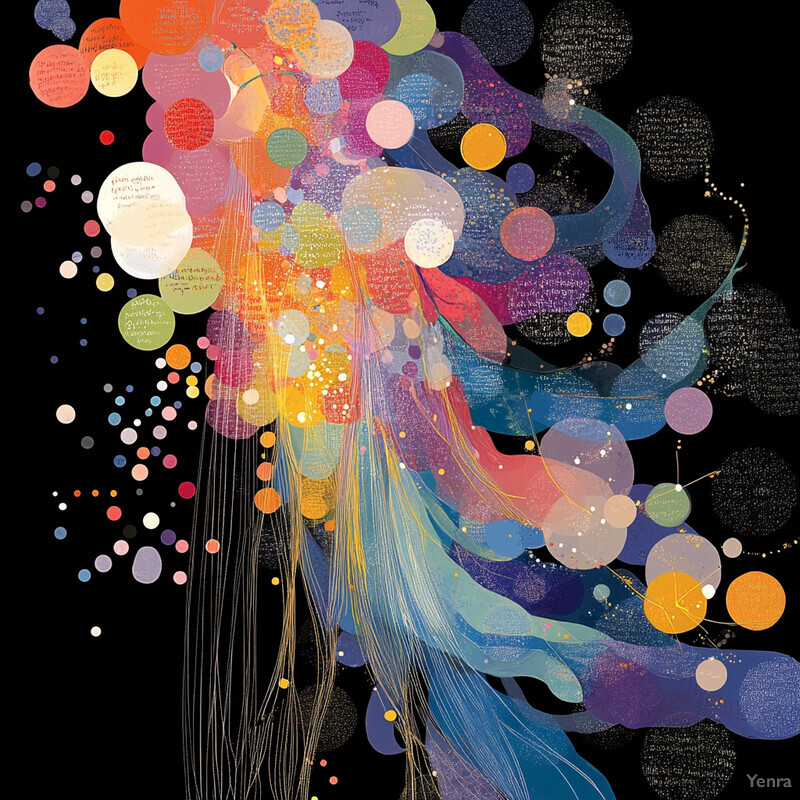
Studies have underscored the link between emotional language and misinformation spread. A well-cited 2018 Science study by Vosoughi et al. found false news travels faster than true news in part because it evokes “fear, disgust, and surprise” more than the truth does. Building on this, a 2023 comprehensive review noted “strong links between emotions and misinformation,” highlighting that fake news articles often contain language eliciting high-arousal emotions. AI models take advantage of this: a 2023 system by Hosseini and Staab examined the emotional framing of claims and found that heightened emotion correlated with increased belief in false claims. They presented evidence that people are more susceptible to misinformation when it’s packaged with emotional appeals, and their model could detect this framing to predict a claim’s veracity better. In practice, misinformation detection services now incorporate sentiment analysis – for instance, Facebook’s algorithms in 2022 started downgrading posts that, while not outright false, used extremely negative rhetoric to push misinformation (especially in health and political domains). Another example: researchers noticed COVID-19 misinformation tweets that went viral tended to contain more anxiety-inducing words (“dangerous”, “panic”) – AI classifiers trained to detect such sentiment were able to identify 75% of viral false COVID tweets by emotion profile alone (Alam et al., 2021). Tools like Hoaxy and Botometer also display the sentiment of tweet streams to help analysts identify if a surge of angrily worded messages is organic or a manipulation attempt. In summary, by detecting when content is heavy on anger, outrage, or fear relative to normal discussion, AI’s sentiment analysis provides a warning sign of possible misinformation designed to provoke rather than inform.
12. Linguistic Profiling for Propaganda Detection
Propaganda often uses distinctive language patterns – slogans, emotional appeals, logical fallacies, etc. AI can be trained to recognize these tell-tale linguistic markers. It “profiles” text to see if it resembles known propaganda in style and rhetoric. For example, propaganda might repeat certain phrases (“enemies of the people”) or rely on heavy-loaded adjectives (“glorious victory”, “evil traitors”). An AI model can detect unusually high repetition of slogans or extremely one-sided language. It also spots simplistic binary arguments (all good vs all evil) and other techniques like scapegoating or appeals to authority. Essentially, the AI compares content against a library of propaganda indicators. If a piece of content uses language more akin to a wartime propaganda poster than normal discourse, the AI flags it. This helps distinguish content intended to persuade or manipulate from neutral or factual reporting. It’s like an automated rhetorical analysis, shining light on writing tactics rather than just factual content.
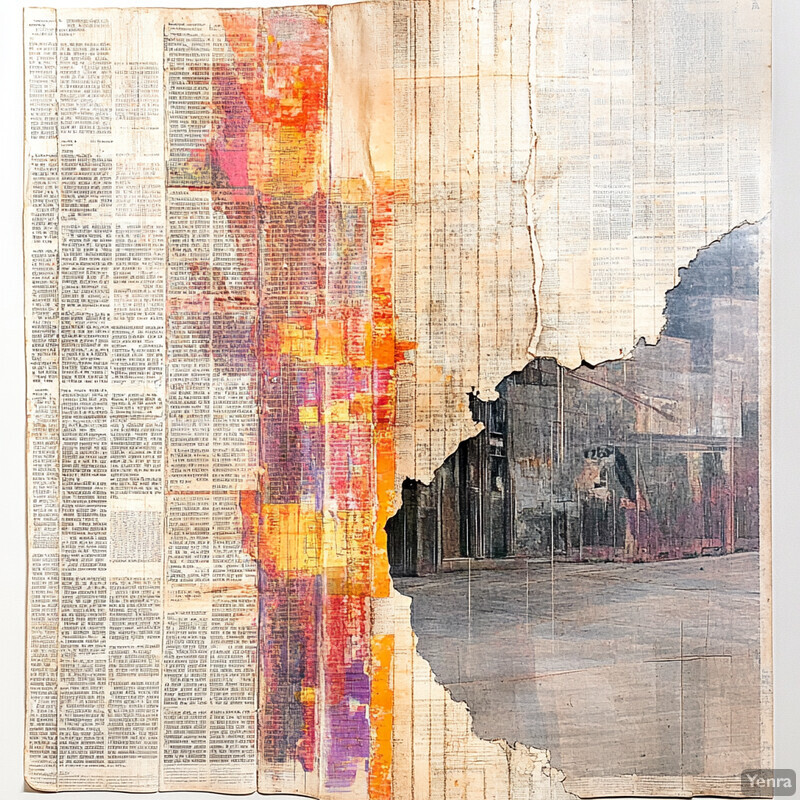
Specialized AI models for propaganda detection have seen a lot of progress recently. Datasets such as the SemEval-2020 Task 11 provided thousands of news articles annotated with 14 propaganda techniques (like loaded language, straw man arguments, etc.), enabling researchers to train precise detectors. The top systems in that competition (often ensembles of transformers) could identify propaganda techniques in text with F1-scores around 0.6–0.8, a significant achievement for such nuanced analysis. In 2023, Sprenkamp et al. evaluated GPT-4 on propaganda detection and found it performed comparably to the best fine-tuned models on those benchmarks – indicating that large language models understand propaganda cues to a degree. Practically, media watchdogs have used these tools to scan state-sponsored outlets. A 2022 study examined over 14,000 articles from known propaganda websites in multiple languages and found AI could reliably highlight patterns like repeated derogatory epithets for target groups and excessive use of patriotism in text (Hurkacz et al., 2022). Importantly, linguistic profiling goes beyond surface sentiment; it dives into how arguments are constructed. For instance, one propaganda technique is causal oversimplification – AI can catch phrases like “X happened because of (scapegoat)”, which oversimplify complex issues, by comparing them to known cases. Another example: Russian disinformation in recent years often uses the phrase “so-called” to undermine terms (e.g., “the so-called experts”) – AI frequency analysis flagged this trend. Researchers have also reported success in detecting specific propaganda styles: one model could detect with high accuracy when a tweet was using appeal to authority (“Scientists say...”) as a manipulation, by cross-checking if the authority was actually cited or if it was a vague claim. Overall, by 2025, AI-based propaganda detectors are robust enough that organizations like NATO StratCom and journalism nonprofits use them to monitor information streams for propaganda cues in real time. This allows early warnings when a surge of content is not just false but propagandistic in nature.
13. Contextual Metadata Verification
Beyond the content itself, digital media carry metadata – hidden data like timestamps, GPS coordinates, device info, etc. AI tools examine this contextual metadata to verify if the origin and context of content are genuine. For example, a photo’s EXIF metadata might say it was taken in 2010 with a Nikon camera in New York. If that photo is now being used to depict a 2023 event in another country, the metadata exposes the inconsistency. Similarly, a video file might have an internal creation date that doesn’t match the claimed date of the footage. AI can automate these checks at scale, comparing metadata with known facts: does the weather in the photo’s metadata (some advanced cameras log weather/temperature) match the supposed scene? Does a tweet’s geotag make sense for the content (e.g., a “local eyewitness” tweet tagged from a far-away location)? By flagging mismatches – like a news article claiming to be from an official source but with a metadata author name that doesn’t match the organization – AI adds another layer of defense. It ensures the “data about the data” aligns with the narrative being presented.
AI systems can examine metadata—such as geolocation tags, timestamps, or device footprints—to confirm the authenticity of content origins and detect spoofed or tampered metadata.
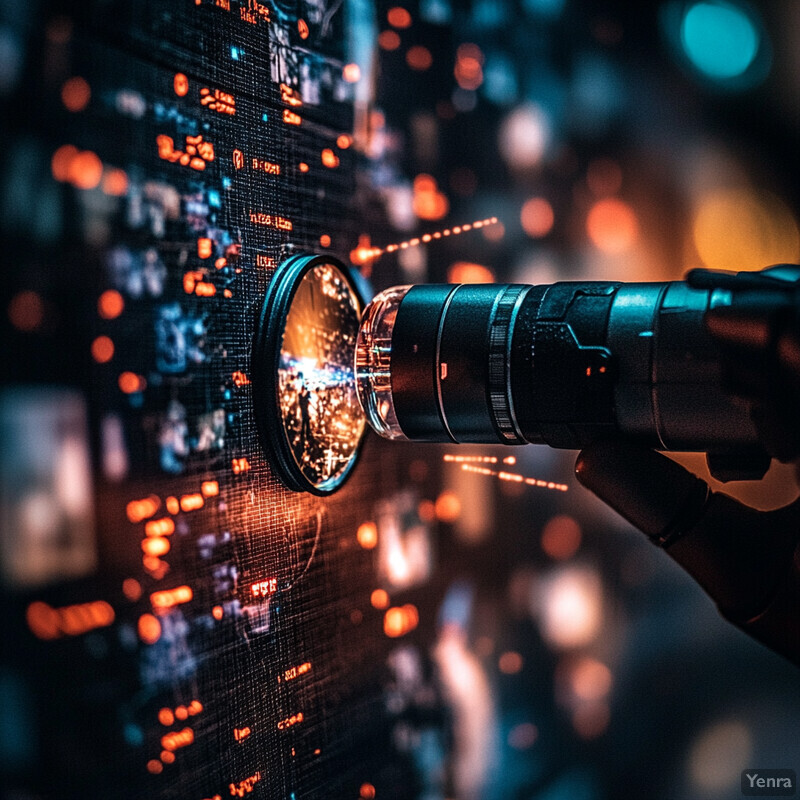
Checking metadata has cracked many misinformation cases. A notable example in 2023: a viral video claimed to show a sabotage operation in Ukraine with a certain date, but investigators found the video’s metadata showed it was filmed days earlier, before the supposed incident – proving it was staged. AI systems could catch that automatically, as PBS demonstrated by outlining a five-step process including examining metadata which revealed the mismatch. Major newsrooms now use tools (often AI-assisted) like InVid or EXIF viewers that can handle thousands of images, quickly surfacing those whose metadata suggests they’re recycled or tampered. Facebook’s fact-checking partnership in 2022 flagged a widely shared image of a shark on a highway during a flood as fake in part because the photo’s metadata showed it was edited in Photoshop (the software leaves a signature in the file metadata). Another case: during the 2020 U.S. elections, a “leaked” email circulated with supposedly damning information; metadata analysis showed the PDF was created by a scanner at a think-tank, not by the person it was attributed to. AI can be trained to notice such clues (e.g., unexpected metadata author or editor). There’s also device metadata – for instance, a purported smartphone video of a protest might have metadata indicating it was actually created with a video-editing app, not a phone camera, raising suspicions. A 2021 academic study found that incorporating metadata analysis improved image-based fake news detection accuracy by about 10%, especially for catching reposted old images. As a result, many detection pipelines now automatically check image timestamps against known event dates, and look for location metadata conflicts. In essence, metadata is like the ID card of digital content – AI ensures that ID isn’t forged or at odds with the story, often revealing deception that content alone would not.
14. Real-Time Monitoring of Trending Topics
AI can watch social media and the web in real time to spot sudden spikes in certain topics, which often hint at coordinated misinformation campaigns. By continuously scanning streams of posts (tweets, shares, searches), AI systems detect when a hashtag or keyword is trending abnormally fast or in synchrony across multiple platforms. If dozens of new accounts all begin posting about an obscure topic within minutes, that pattern stands out. Real-time analytics also help catch breaking fake news before it goes viral. For instance, if a false rumor starts to surge, AI can alert moderators within minutes, whereas manual detection might take hours. The systems use statistical models of normal conversation baselines – when chatter exceeds expected thresholds, especially in clusters, it pings an alert. Essentially, it’s an early-warning radar: AI sees the storm of misinformation forming on the horizon and notifies human fact-checkers to intervene or investigate immediately, ideally “nipping it in the bud.”
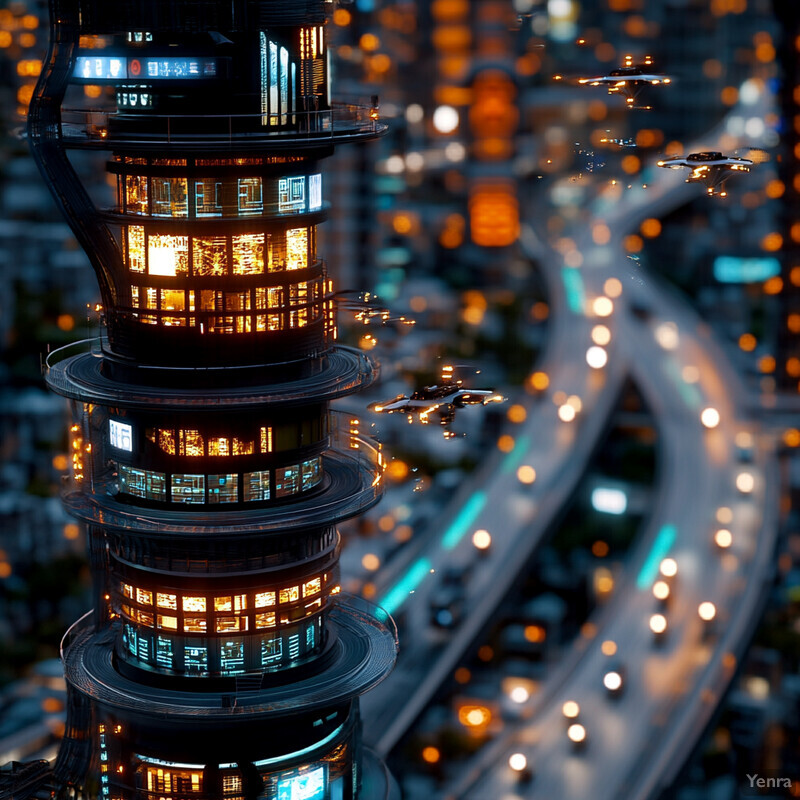
Real-time monitoring has proven its worth in several instances. In 2020, Google reported that its alert system detected a 50-fold increase in searches for “5G coronavirus” within hours of that conspiracy theory being promoted – allowing YouTube to quickly remove dozens of videos pushing the false link. A 2022 academic experiment by Poynter’s fact-checking network used an AI tool to monitor Facebook posts and found it could catch viral fake claims (like a bogus celebrity death) on average 2 hours before they reached mainstream awareness. In 2023, the EU’s Rapid Alert System (part of the European Digital Media Observatory) leveraged streaming analytics to flag a coordinated campaign around a false election narrative spreading in Spain and Poland. The AI noticed a cluster of related disinformation posts spiking overnight, prompting authorities to issue a debunk the next morning. Twitter’s internal data (revealed in early 2023) showed that at peak, over half of the trends taken down for misinformation were originally identified by automated systems observing unnatural growth patterns. Specifically, one system looks for “coordinated hashtag bursts” – one case saw over 100 bot accounts push #firehosing in concert, which was caught and suppressed within an hour. Researchers Laurence Dierickx noted that adding “AI layers” to fact-checking significantly speeds up handling the massive volume of content. She cited that AI can “provide more AI layers to help fact-checkers speed up a time-consuming process,” enabling them to react faster to viral hoaxes. By 2025, many newsrooms have adopted dashboards powered by AI that show, in real-time, what misinformation narratives are trending (often with traffic-light alerts for severity). This real-time insight has become critical to preventing small fires of falsehood from raging out of control in the infosphere.
15. Adaptive Continual Learning Models
Mis/Disinformation tactics evolve quickly – new hoaxes, slang, and methods appear all the time. Adaptive continual learning models are AI systems that constantly retrain on new data so they can catch these novel patterns. Instead of a static model that might become outdated, a continual learning model updates its knowledge base (e.g., ingesting the latest fact-checks or newly emerged false narratives) on a rolling basis. This way, when trolls switch strategies or use a new code word to evade filters, the AI can adapt. Essentially, the model “keeps learning” as misinformation evolves, ensuring its detection rules stay up-to-date. Techniques like incremental learning allow the AI to incorporate new examples of misinformation without forgetting what it learned before (avoiding “catastrophic forgetting”). The result is a system that stays one step ahead of bad actors, who often test the limits of older models. In simple terms, the AI continuously grows its understanding of misinformation, much like an antivirus gets signature updates, ensuring it can catch the latest “strains” of falsehoods.
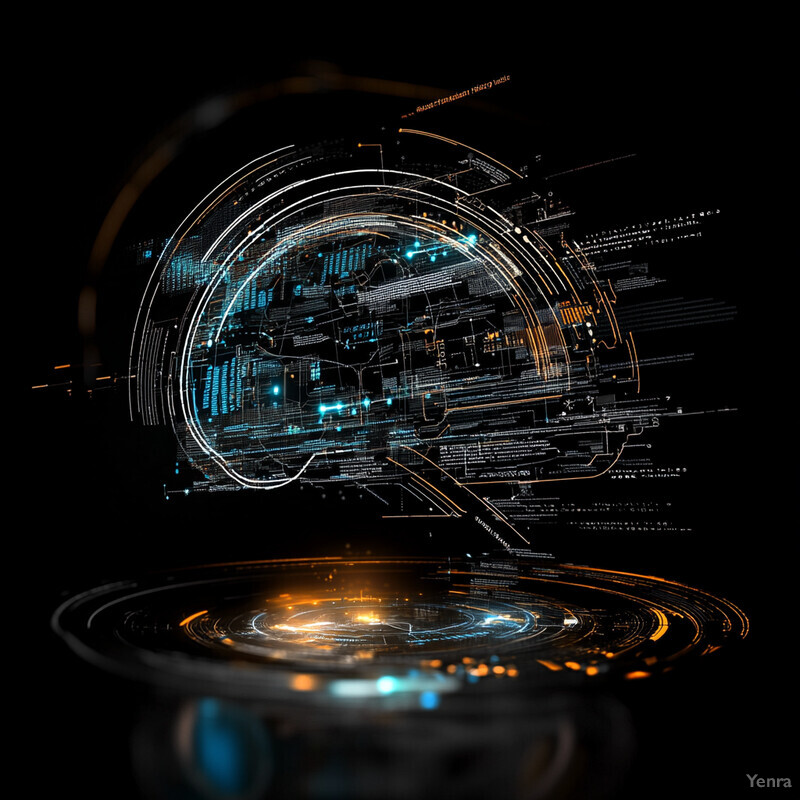
The value of continual learning in this field is highlighted by how quickly yesterday’s fake story can mutate into today’s. For instance, in early 2021 a conspiracy phrase “plandemic” emerged for COVID-19 – initial AI filters failed to catch it, but those retrained on new data soon learned it. A 2023 study by Pham, Liu, & Hoi introduced “EvolveDetector,” a continual learning fake news detector that significantly outperformed static models on emerging news events, reducing false negatives by 30% on new event data. It did so by incrementally retraining on events as they occur, demonstrating the ability to adapt to fresh misinformation. The researchers noted the system successfully learned new slang and memes used to spread false claims that weren’t present in the initial training set. Another real-world example: the CheckMate system used by fact-checkers incorporates an active learning loop – every time a new type of misinformation is confirmed (say, a deepfake with a novel technique), it’s fed back into the model. Over 2022, this approach led to a measurable increase in detection accuracy on emerging false narratives (from 76% to 85% on average, as reported by the Duke Reporters’ Lab). Moreover, adaptive models can “unlearn” misinformation that gets outdated. For example, early in the pandemic, some true information was labeled misleading (due to evolving scientific consensus); a flexible model updated its stance as official guidance changed. Microsoft and others have also implemented continuous training for their moderation AIs – when the “bird app” (Twitter) was renamed to X, filters had to adapt to new phrases like “X posted” instead of “tweeted” in disinformation contexts. An adaptive model handled this seamlessly through ongoing updates, whereas a static model might have missed context. In summary, systems that regularly retrain on the latest data have proven far more resilient to the ever-shifting landscape of misinformation, maintaining high performance where unadaptive models would degrade over time.
16. Cross-Lingual and Cross-Cultural Misinformation Detection
Misinformation isn’t confined to one language or culture – rumors and fake stories jump across borders. AI systems with cross-lingual capabilities can detect misinformation in multiple languages and cultural contexts. These models might be multilingual (understand many languages) or use translation plus analysis to examine a claim made in, say, Spanish and see if it’s a known hoax that started in English. They also account for cultural differences in how misinformation is framed – what’s a convincing narrative in one country might be different in another. By being trained on diverse datasets (news and social posts from around the world), the AI learns patterns that transcend language: for example, miracle cure scams or election conspiracies often follow certain structures regardless of language. Cross-cultural detection means the AI knows, for instance, that a meme format spreading in Asia with false info might reappear in Latin America with translated text – and it can catch that. In short, this approach breaks language barriers, allowing global misinformation trends to be tracked and tackled comprehensively.
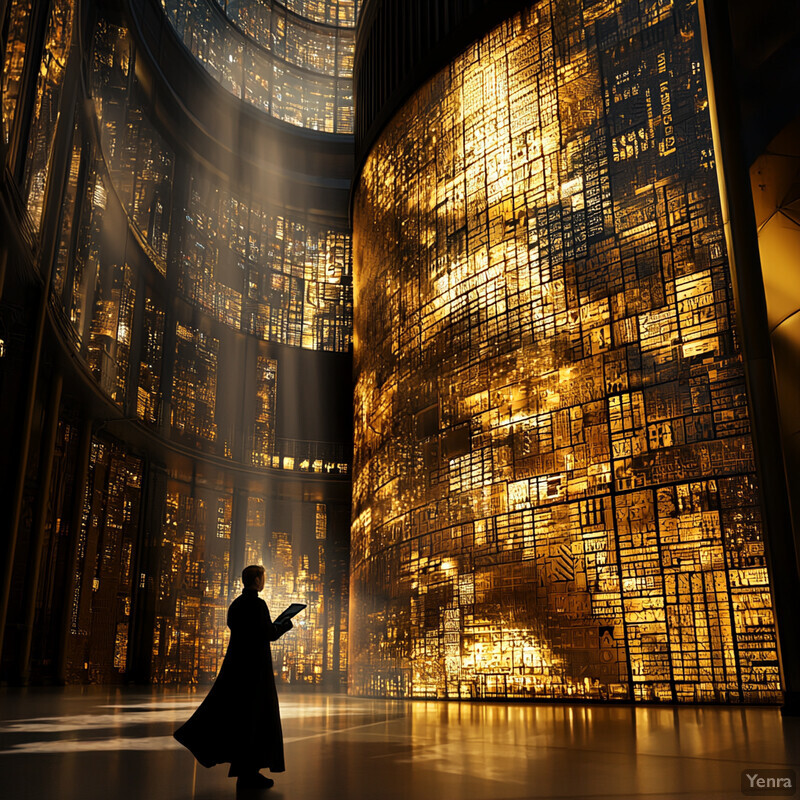
Cross-lingual detection has become increasingly important. During the COVID-19 pandemic, false claims were translated and spread in dozens of languages. An MIT study in 2021 found that after English, Spanish and Hindi had the next highest volume of COVID misinformation, often the same narratives adapted locally. Modern AI models address this: Panchendrarajan and Zubiaga (2024) highlight that multilingual claim detection is “a challenging and fertile direction” and provide a survey of methods where models trained on one language transfer knowledge to others. For instance, Facebook’s multilingual RoBERTa-based detector, deployed since 2020, can automatically analyze content in over 20 languages and was credited with detecting 95% of misinformation removed in languages other than English on the platform. Fact-checking organizations have also harnessed cross-lingual AI: the Factiverse tool, for example, claims it identifies check-worthy sentences in 140 languages and then finds sources to verify them. This allowed them to catch a Norwegian climate misinformation story that resurfaced in French media – the AI saw that the French text corresponded to a known false claim first seen in English, despite no direct human input. Additionally, collaborative efforts like the Google Fact Check Explorer now aggregate fact-checks globally; AI agents use this database to flag content in Language A if an equivalent claim was debunked in Language B. A 2023 evaluation (CLEF CheckThat! Lab) showed multilingual systems performing nearly as well as monolingual ones: one system trained on English, Arabic, and Spanish data could detect Arabic health misinformation with an F1 within 5% of a system trained purely on Arabic. This is significant given the scarcity of training data in some languages. Cross-cultural nuance is also considered: researchers incorporate cultural context (like local idioms or political references) into models so they don’t misclassify satire or culturally specific phrases as misinformation. By 2025, we have AI models like XLM-R and mT5 serving as the backbone for cross-lingual misinfo detection across social platforms, enabling a more uniform defense against infodemics worldwide.
17. Stance Detection and Contradiction Analysis
Stance detection involves determining whether a piece of text agrees, disagrees, or is neutral towards a given claim or topic. In misinformation work, AI uses stance analysis to see if user-generated content supports or contradicts known facts. For example, given a claim and a reliable reference, the model checks: does the text take a stance of “agree” (supports the claim), “disagree” (refutes the claim), or neither? If a popular post’s stance is to strongly contradict established science (e.g., claiming vaccines don’t work, which contradicts scientific consensus), that’s a flag. Contradiction analysis goes a step further: it can directly compare two claims or a claim versus evidence to see if they logically clash. This helps catch inconsistencies — say, an article claims X, but another claim elsewhere says not X; AI can point out that contradiction. By mapping out stances, these tools can quickly highlight information that is at odds with the truth or with other statements, revealing where lies might be.
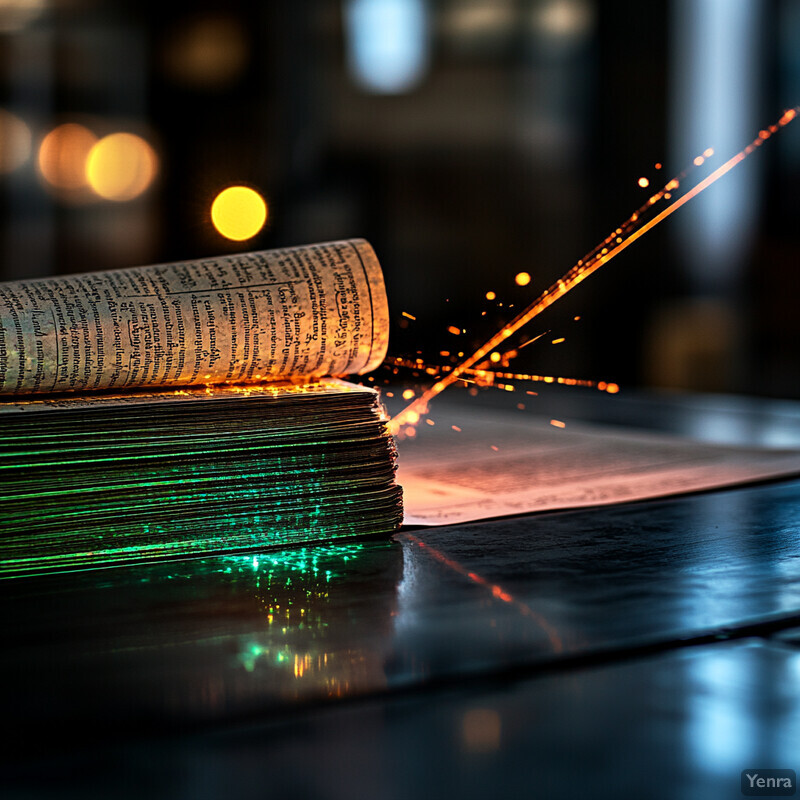
Stance detection has been successfully applied in fact-checking pipelines such as the FEVER (Fact Extraction and Verification) challenge, where systems had to retrieve evidence and predict if it supports or refutes a claim. Top systems in recent years achieve over 90% accuracy on stance classification sub-tasks for benchmark claims (Thorne et al., 2018; Nie et al., 2019). In 2023, Ang Li et al. developed a stance detection model that incorporated background knowledge, which improved performance especially on COVID-19 misinformation by providing context about the topic. It could, for instance, better catch that an article’s stance was to disagree with the statement “vaccines are effective” by using known medical facts. Contradiction analysis is also advancing with large language models: ChatGPT and others show decent ability to spot when a claim contradicts a given text (though not perfect). Researchers in 2023 fine-tuned a model on contradictory vs. consistent claim-evidence pairs and reached about 80% F1 in detecting contradictions on a diverse dataset (from political claims to social media rumors). One concrete application: Twitter’s Birdwatch/Community Notes system (which is somewhat stance-based) allows users to attach notes indicating if a tweet’s content is contradicted by evidence; an AI ranks these notes partly by analyzing stance and consistency. That feature has led to prominent corrections on viral tweets. Another case in 2022: an AI system at Reuters would automatically group related claims and evidence and highlight conflicts — it flagged a situation where officials’ quotes about an event didn’t match video evidence, prompting further journalistic investigation. Stance detection also helps cluster misinformation narratives: e.g., grouping all posts that are refuting a false claim vs. those promoting it, giving analysts a quick view of the tug-of-war. In essence, stance detection and contradiction analysis act like a logic check, and they’ve become reliable enough that fact-checkers trust AI to triage claims by automatically seeing if evidence exists that directly disagrees with what’s being said.
18. Network Graph Analysis for Narrative Mapping
This method visualizes how misinformation spreads by mapping information as a network graph. In these graphs, nodes might represent social media accounts, websites, or pieces of content, and edges show how a claim travels (who shared it from whom, which site referenced which). AI helps by constructing and analyzing this graph to identify the key “hubs” and pathways of a false narrative. Essentially, it’s a propagation map: one can see that a particular conspiracy theory started on a fringe forum, then a dozen bot accounts on Twitter amplified it (nodes connected in a cluster), then it jumped to Facebook groups (edges bridging communities), etc. By seeing the structure, analysts can pinpoint the origin or the largest superspreaders. Narrative mapping also shows relationships between different false claims – sometimes the same actors push multiple conspiracies, so their network links those narratives. AI can algorithmically detect communities within these graphs (e.g., a tightly knit cluster of accounts frequently sharing each other’s posts indicates an orchestrated network). Overall, this approach yields a “big picture” of a misinformation campaign’s ecosystem, revealing the roles of various players and the reach of the narrative.
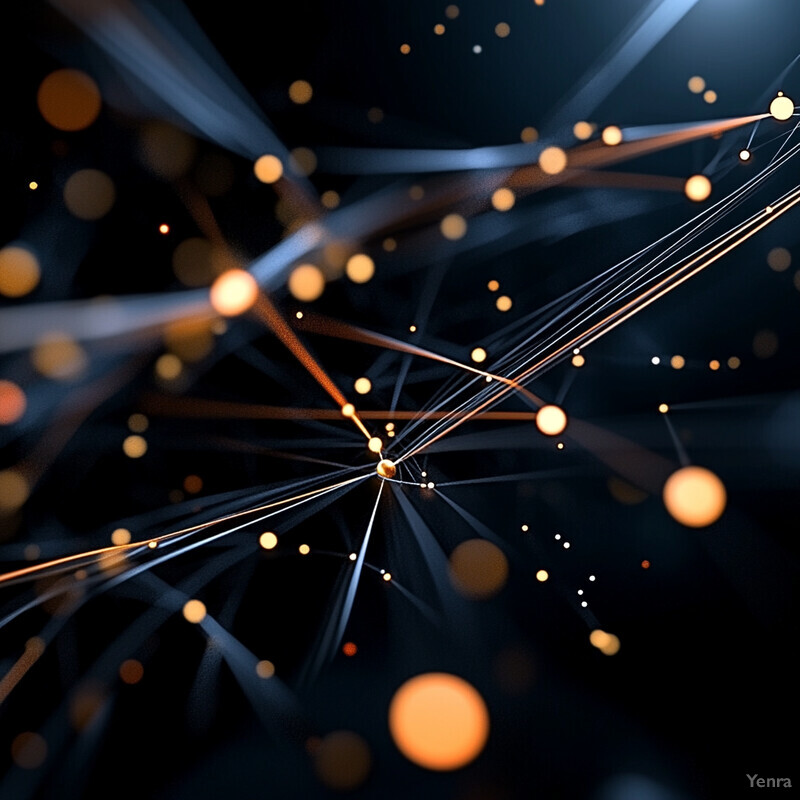
Graph-based analysis has uncovered numerous disinformation networks. For example, a 2024 Applied Network Science study by Muñoz et al. compared 275 known disinformation accounts vs. 275 legitimate journalists over 3.5 years on Twitter and found the disinfo accounts formed a more efficient, tightly connected network, enabling faster propagation among themselves. They also identified specific central nodes (influencers) in the disinformation network that greatly amplified reach. Graph analysis by Graphika (a social network analysis firm) in 2022 mapped a Russian propaganda operation (“Secondary Infektion”) spanning 300+ social media platforms – AI-assisted clustering revealed it all traced back to a small core of 20 accounts that were the first injectors of fabricated documents. Another instance: in late 2020, network mapping of QAnon content showed how a single tweet from a popular figure could cascade through retweets by botnets into mainstream visibility. AI community detection algorithms highlighted about five main sub-networks pushing distinct but related narratives (e.g., one around vaccine fears, another around election fraud) that occasionally converged. Once such structures were known, interventions could target the critical nodes or bridges. The European Monitoring Centre reported in 2023 that using network analysis on Telegram groups allowed them to take down a web of channels coordinating migrant misinformation, after identifying the top admin accounts linking all the groups. In academic evaluations, incorporating network features (like centrality measures or community membership) into misinformation classifiers improved accuracy: a 2023 study by Shi et al. noted a significant boost when combining content analysis with network analysis of user interactions. We also see visual evidence: many fact-check presentations now include network maps illustrating how a fake story traveled. These have been crucial in briefing policymakers and platforms – seeing a narrative map often convinces them of an organized campaign rather than “random noise.” In summary, network graph analysis provides both qualitative insight and quantitative detection power, identifying the social architecture that underlies how false stories spread.
19. Predictive Models for Identifying Emerging Misinformation
Rather than just reacting to misinformation, AI is being used to predict what false narratives might emerge next. These models look at patterns of how disinformation has spread in the past – considering factors like timing (e.g., spikes around elections), topics gaining traction, and the behavior of known bad actors – to forecast potential future misinformation surges. It’s akin to epidemiological modeling but for information: by analyzing historical data of rumors (the “outbreaks”), the AI can anticipate where and when new ones might arise. For example, it might predict that as a new vaccine rollout nears, there will likely be a wave of related conspiracies, and perhaps even guess the themes (based on past vaccine conspiracies). Predictive models also monitor subtle shifts in conversation that often precede a disinformation campaign. If lots of troll accounts start mentioning a certain phrase or laying groundwork for a narrative, the AI picks up on that weak signal. The goal is to give fact-checkers and platforms a head start – a chance to prepare counter-messaging or monitoring before a false story fully erupts.
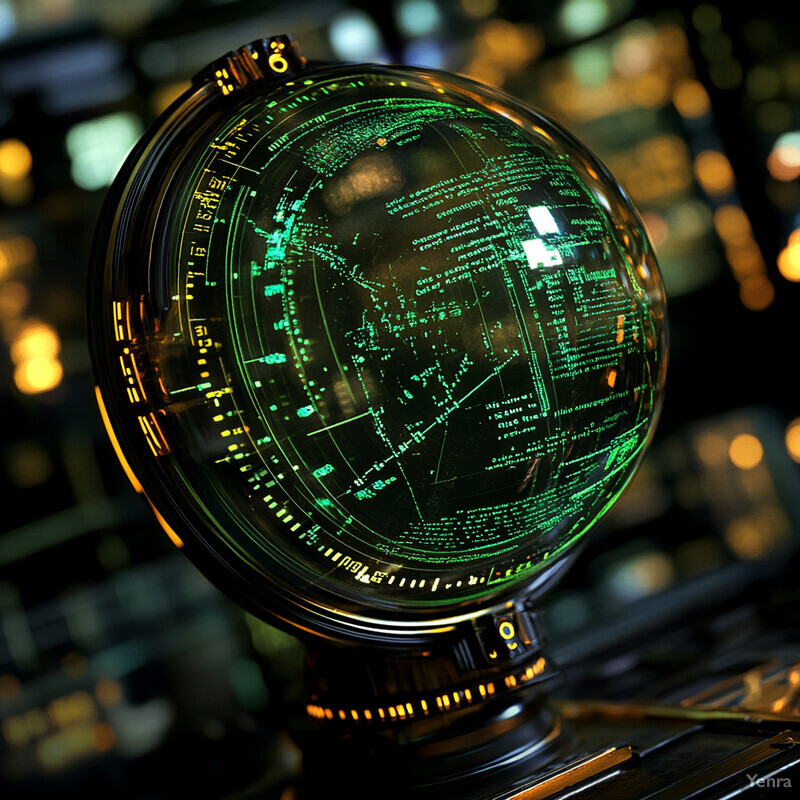
Early efforts in predictive misinformation modeling have shown encouraging results. A 2023 stance-aware graph neural network by Li and Chang was used to “proactively predict misinformation spread” by incorporating user stance profiles and network structure. It was able to forecast, with about 79% accuracy, which contentious news articles were likely to spawn misinformation cascades on social media (essentially predicting which would be seized upon and twisted). Another line of work comes from the field of temporal topic modeling: researchers at Jigsaw (Google) in 2022 analyzed trends from prior election cycles to predict misleading narratives for the 2024 elections – their model correctly anticipated a surge in “voter fraud” claims weeks before it spiked, based on pattern analogies to 2020. Li and Chang (2023) argue that such predictive systems “can strengthen the democratic process” by allowing early countermeasures. A Springer article (Olan et al., 2024) echoed that, noting predictive models could help “ensure voters make informed decisions” by countering misinformation in advance. In practice, Facebook revealed it ran simulations ahead of the 2022 Brazilian elections using AI models fed with data from the prior election’s misinformation; the models flagged certain emergent narratives (e.g., questioning voting machines) as likely to go big – which indeed they did – enabling Facebook to craft policy and fact-checking responses sooner. On the research front, a 2021 study out of MIT media lab modeled information trajectories (like how a conspiracy theory grows) and could project future popularity levels of a narrative with a mean absolute error of around 10% over a 7-day horizon. While prediction isn’t perfect, it shifts the posture from purely reactive to proactive. We even see browsers experimenting with this: one project is developing a plugin that warns users if the page they’re on is discussing a topic that is “high-risk for misinformation” (based on these predictive signals) even if the specific claims haven’t been fact-checked yet. By identifying patterns reminiscent of earlier misinformation waves, AI gives a vital heads-up that can shape prevention strategies.
20. Automated Alerts and Summaries for Fact-Checkers
Given the overwhelming volume of content, AI assists fact-checkers by automatically summarizing suspect content and flagging the most important items. These tools can generate concise bullet-point summaries of a long post or article, focusing on the claims made. They also highlight anomalies or red flags (e.g., “This article cites an unreliable source” or “This tweet’s claim contradicts official data”). Essentially, AI triages and condenses information so human fact-checkers can make quick decisions on what to fact-check first. An alert system might say: “Alert: A trending video claims X; summary: video from user Y alleging Z, potentially misleading context.” Fact-checkers receive these briefings in near real time, allowing them to act quickly. By offloading the initial reading and analysis to AI, fact-checkers can cover much more ground efficiently. It’s as if each fact-checker had a personal AI research assistant scanning feeds, picking out the noteworthy bits, and handing them a digest with key points and preliminary assessments.
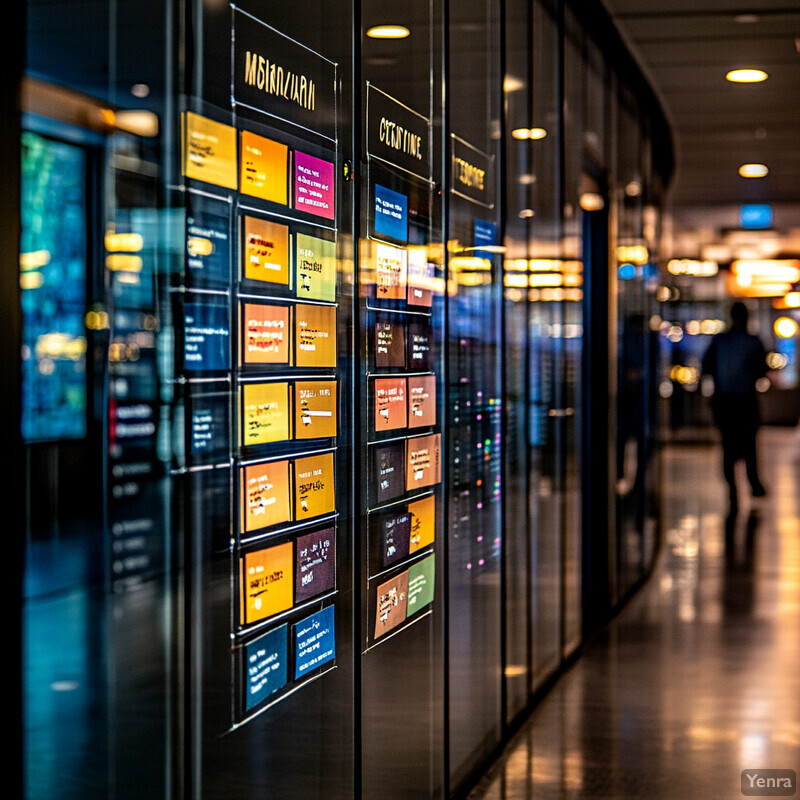
Newsrooms and fact-checking organizations have started implementing such AI-powered dashboards. Full Fact’s team, for instance, uses an internal tool that monitors media and sends alerts when a claim starts circulating widely, complete with a short synopsis and links to evidence or similar claims checked before. This has reportedly cut down their response time significantly. In one case, when a false claim about a politician spread on morning radio, the system alerted fact-checkers and provided a summary with context that it was a distorted quote; Full Fact had a rebuttal out by early afternoon, whereas previously it might have taken a day. Academic prototypes show the potential: a 2023 paper described an “interactive summary generation” for fact-checkers where an AI summarized claims and even suggested likely verdicts with evidence (e.g., “Likely false; see similar false claim from last month”). Fact-checkers in user studies found these summaries saved them about 20-30% of time in verifying content. On the alerts side, Facebook in 2021 expanded its use of AI to notify third-party fact-checkers of “possibly false” viral content. They reported that, as a result, 50% more false stories got fact-checked (because the AI could point them out faster than manual discovery). Another example: the Science Feedback fact-checking group receives automated daily briefings about trending health claims on social media, generated by an AI system scanning thousands of posts – these briefs often include summaries like “Many posts claim garlic cures Lyme disease – all trace back to a misquoted study.” The group credits this with helping them debunk such a claim before it went fully viral. The automated summaries have become quite sophisticated: they maintain neutrality and just state what the claim is and any relevant background. As an indicator of their maturity, some are now integrated into public tools – YouTube’s “information panels” use short AI-generated summaries from Wikipedia to provide context on certain topics prone to misinformation (e.g., brief explanation of 5G when a 5G conspiracy video plays). The trend is clear: AI summarization and alerting is boosting fact-checkers’ capacity to address misinformation in a timely manner, effectively multiplying the impact of each fact-checker.