1. Real-time Predictive Analytics
AI-driven digital twins can continuously ingest sensor data and operational metrics to predict machine behavior and product quality trends, allowing for proactive decision-making.
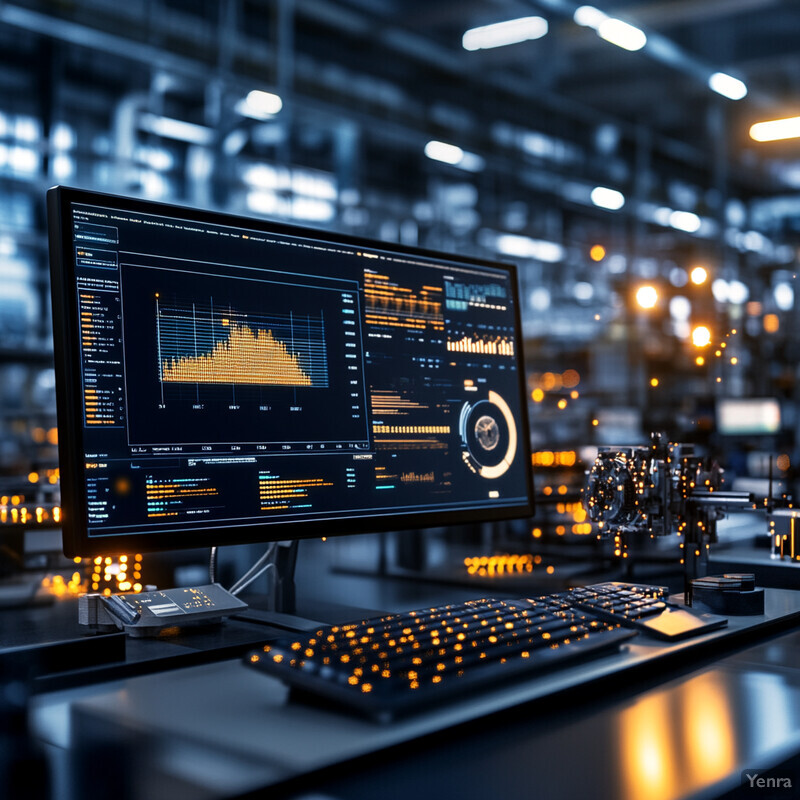
By incorporating AI-driven algorithms into digital twins, manufacturers gain the ability to continuously monitor live operational data feeds—such as temperature, pressure, vibration, and throughput—across their entire production ecosystem. This real-time data input, coupled with predictive modeling techniques like machine learning and neural networks, allows for early detection of performance deviations and emerging trends. Operators can foresee potential quality issues, identify when certain parameters might drift out of spec, and proactively adjust process variables before detrimental conditions occur. Ultimately, real-time predictive analytics transforms the digital twin from a static snapshot into a dynamic decision-support tool that consistently anticipates problems and optimizes manufacturing outcomes.
2. Advanced Anomaly Detection
Machine learning algorithms can identify subtle deviations from normal operation within the twin’s data streams, alerting engineers to emerging issues before they escalate.
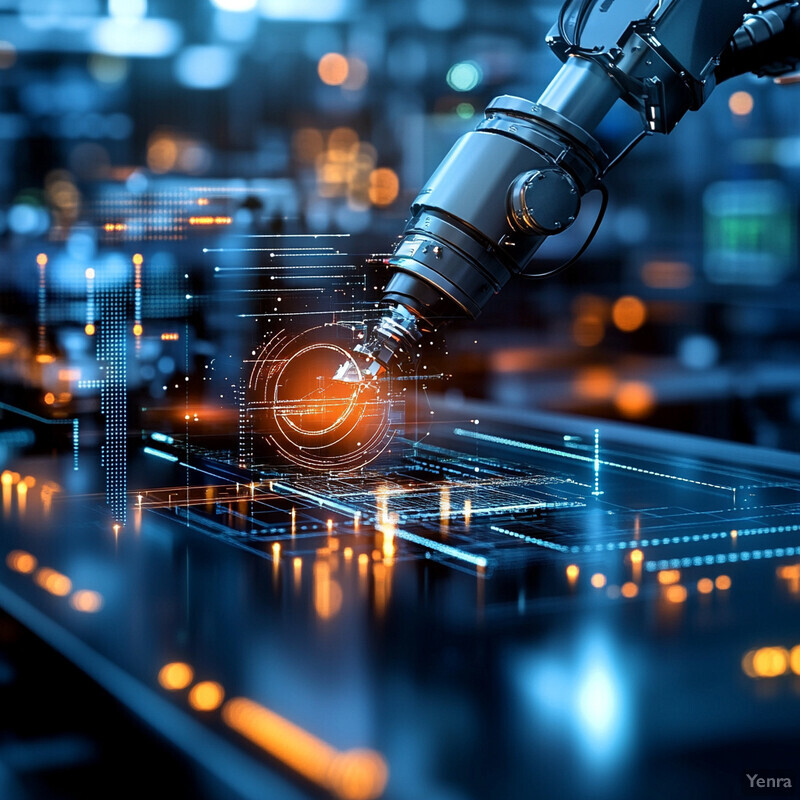
AI-enhanced digital twins excel at recognizing unusual patterns and subtle deviations in complex production processes. By learning the normal operating signatures and performance baselines of machinery, the AI models can highlight anomalies that may be too subtle for human operators to notice. This might include a slight increase in vibration amplitude in a milling machine or a minor drop in material hardness. As soon as an anomaly arises, the digital twin alerts the engineering team, enabling them to investigate and intervene early. By catching these irregularities sooner, manufacturers can prevent small issues from escalating into significant defects, unplanned downtime, or safety hazards.
3. Dynamic Process Optimization
AI can iteratively refine operational parameters—such as temperature, pressure, or flow rates—enabling the digital twin to recommend optimal adjustments to maximize efficiency and minimize waste.
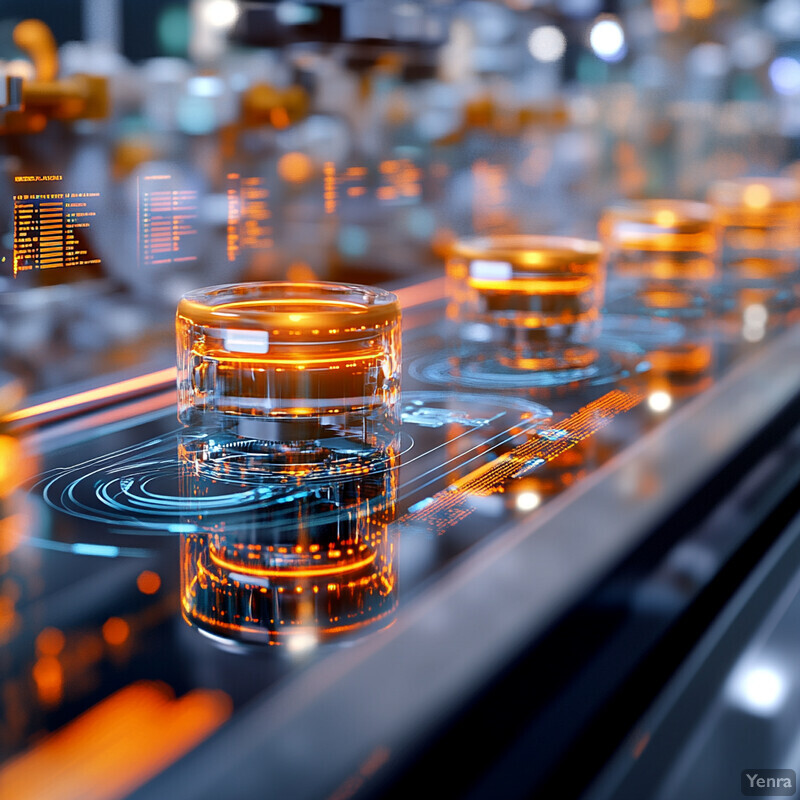
AI-driven optimization within digital twins enables a constant quest for peak efficiency. Instead of relying on periodic human-led audits or guesswork, the AI agents systematically analyze the interplay of variables—such as feed rates, spindle speeds, curing times, and material blends—to find the ideal operational setpoints. Over time, the system refines these parameters based on continuously updated data, adjusting them as conditions evolve. This responsive optimization not only drives greater productivity and cost-effectiveness but also reduces the environmental footprint by minimizing energy use and waste. Ultimately, the digital twin can self-tune manufacturing processes, maintaining a consistently high level of performance with minimal human intervention.
4. Automated Root-Cause Analysis
By leveraging advanced analytics, digital twins can trace complex fault conditions back to their fundamental causes, reducing troubleshooting time and improving system reliability.
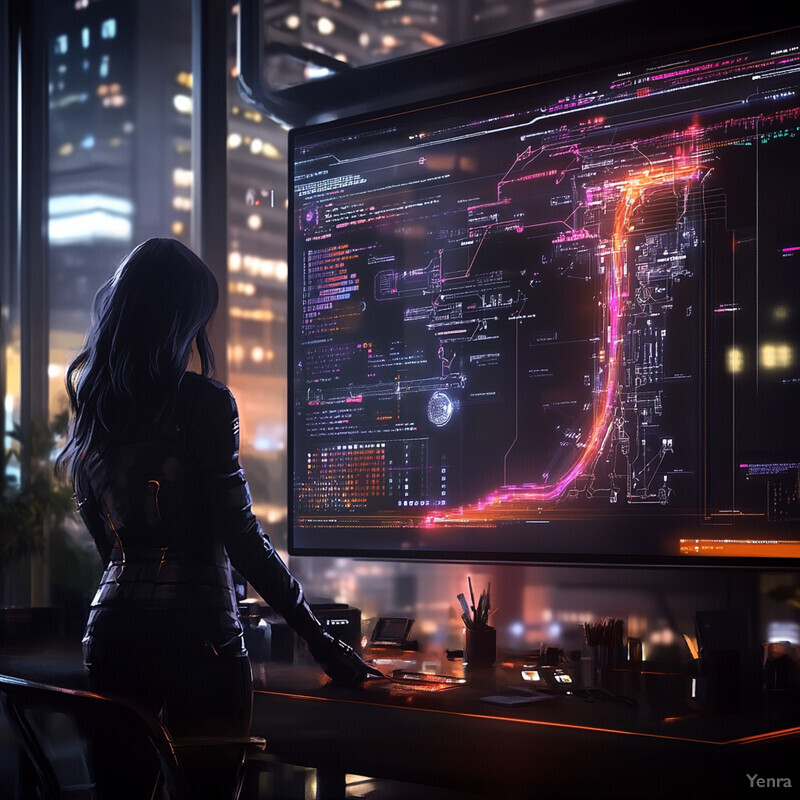
When a defect or unexpected outcome emerges, identifying its root cause can be like finding a needle in a haystack, especially within highly complex, multi-step production lines. AI-powered digital twins streamline this process by mining historical and real-time data, correlating events, and applying statistical and machine learning techniques. These algorithms sift through production logs, sensor readings, operator inputs, and final product inspections to pinpoint underlying issues, such as a miscalibrated sensor or improper timing in a multi-stage assembly. By rapidly narrowing down probable causes, digital twins accelerate corrective actions, improve problem-resolution efficiency, and enhance overall system reliability.
5. Predictive Maintenance Scheduling
AI-enhanced digital twins can forecast when components will fail or need service, ensuring maintenance is conducted just-in-time, thereby reducing downtime and maintenance costs.
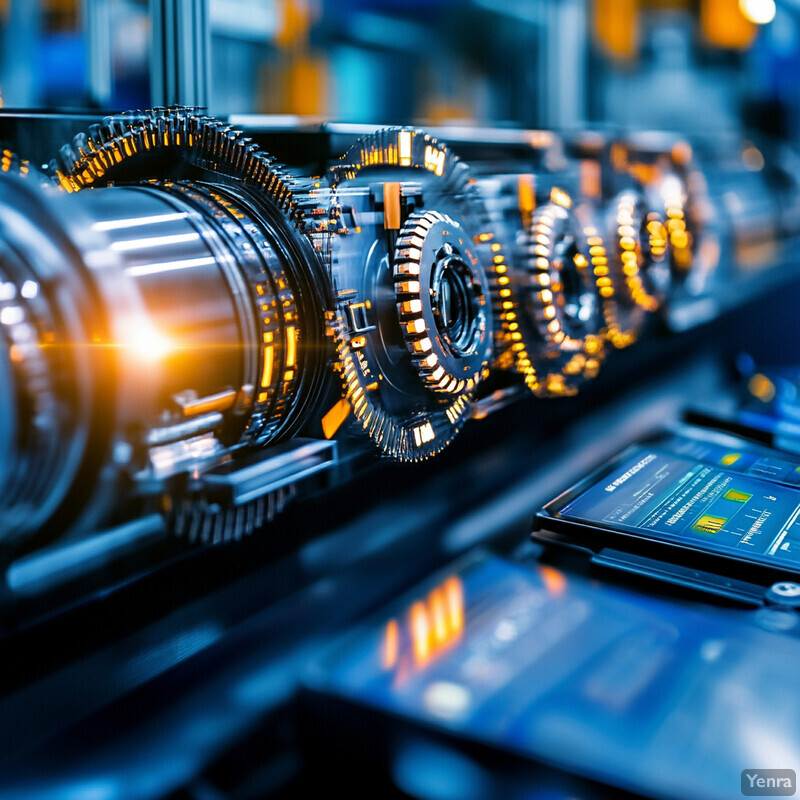
Traditional maintenance schedules often rely on fixed intervals or reactive responses after a machine fails. In contrast, AI-driven digital twins leverage predictive models to forecast when individual components will degrade based on operating conditions, usage patterns, and environmental factors. The twin continuously updates its assessments as new data arrives, identifying the perfect window for planned maintenance—neither too early to waste resources nor too late to risk a breakdown. This proactive approach reduces downtime, extends equipment life, and optimizes parts inventory management, effectively turning maintenance into a strategic, data-driven activity that keeps the plant running smoothly.
6. Enhanced Simulation Accuracy
AI algorithms can continuously learn from physical system performance and refine the digital twin’s simulation models, making them more accurate representations of real-world behavior.
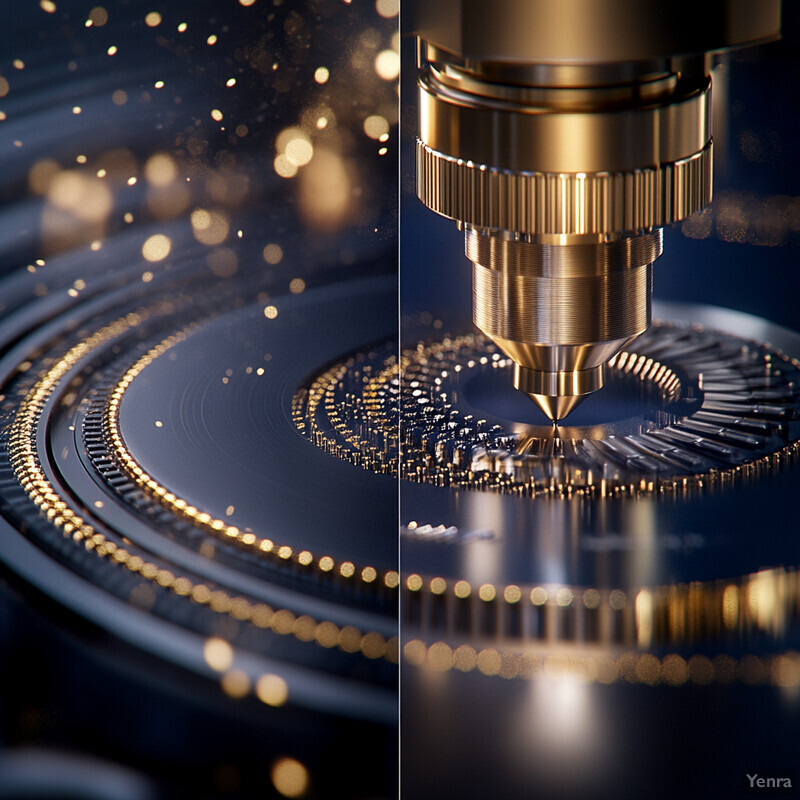
Digital twins represent virtual counterparts of physical equipment and processes, but their value depends on how faithfully they mirror reality. AI algorithms improve this fidelity by learning from feedback loops: they compare simulated outcomes with real-world results and adjust underlying models accordingly. For instance, if a simulated machining step consistently underestimates cutting tool wear, the model refines its parameters until predictions align closely with observed behavior. This iterative learning elevates the accuracy and reliability of simulations, enabling engineers to trust the twin’s insights for design validation, process tuning, and performance forecasting with greater confidence.
7. Scenario Testing and What-If Analysis
By integrating reinforcement learning, the digital twin can rapidly evaluate different manufacturing strategies, allowing engineers to test potential changes without interrupting production.
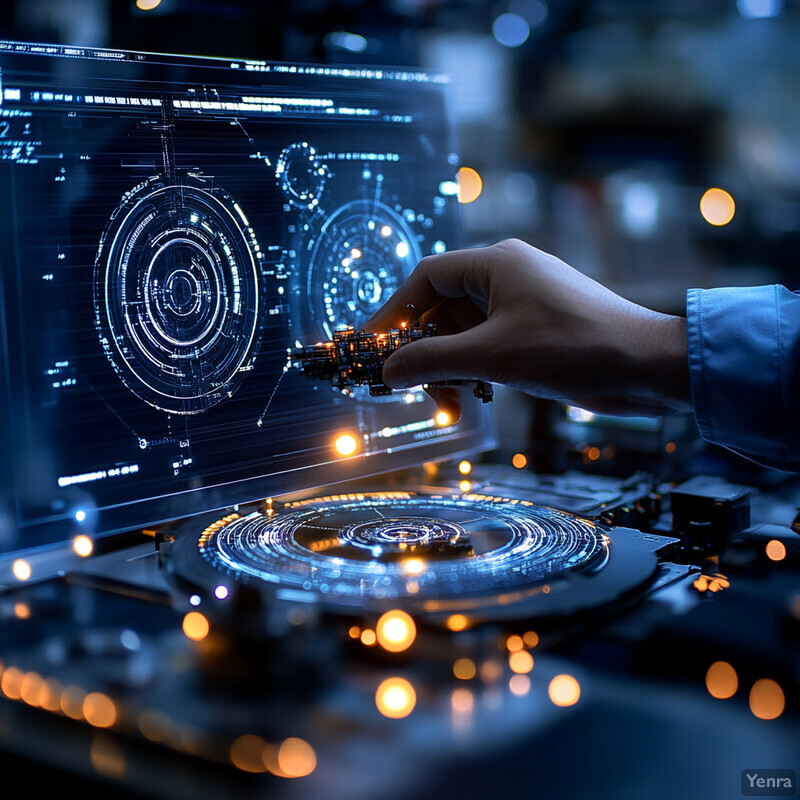
Before implementing new strategies, such as changing raw material suppliers or adjusting production speeds, manufacturers can use AI-driven digital twins to simulate a wide range of hypothetical scenarios. Employing reinforcement learning and other AI techniques, the twin rapidly evaluates “what-if” conditions, testing how changes might affect throughput, yield, quality, and cost. This virtual experimentation saves significant time and resources, as it avoids costly trial-and-error in the actual plant. Decision-makers gain actionable insights from these scenario analyses, empowering them to make data-backed changes that improve production processes without risking interruptions or quality slips.
8. Process Autonomous Control
AI can enable partial or fully autonomous control loops, where the digital twin not only suggests changes but can also execute adjustments to maintain optimal operation.
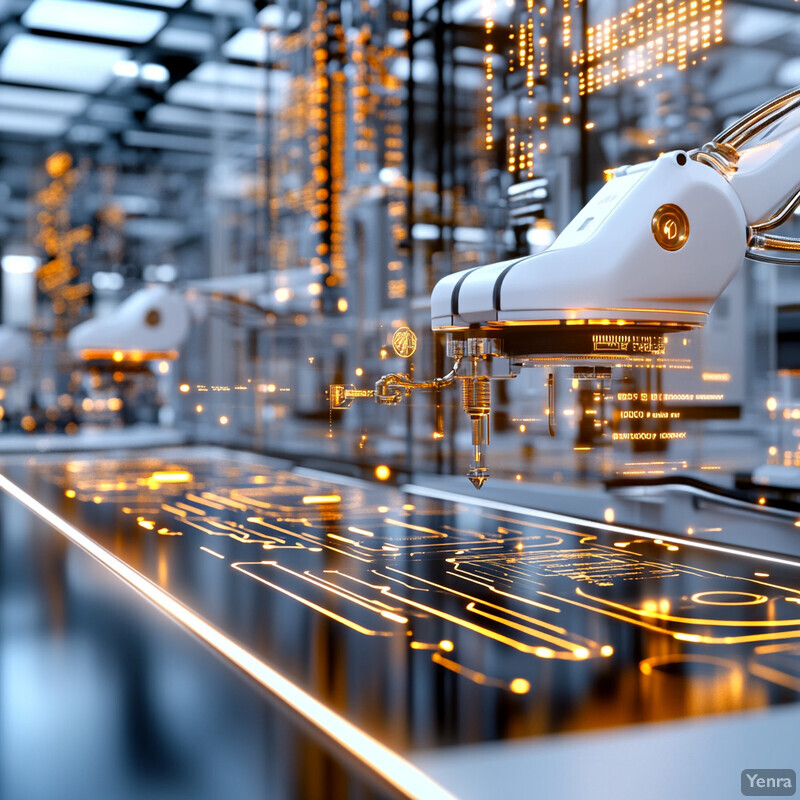
Beyond offering recommendations, some AI-augmented digital twins can move toward closed-loop process control. By continuously comparing actual outcomes against desired targets, the digital twin’s AI engines adjust machine settings and process parameters in real-time. In doing so, the system autonomously maintains optimal operating conditions without waiting for human intervention. This capability reduces reaction times, eliminates human error, and ensures consistent output quality. While human experts still oversee the systems to enforce safety and compliance, autonomous control elements enable manufacturing environments to become more agile, stable, and cost-effective.
9. Virtual Commissioning and System Validation
Before deploying new machinery, AI-driven digital twins can virtually commission equipment, identifying integration issues and validating controls in a risk-free environment.
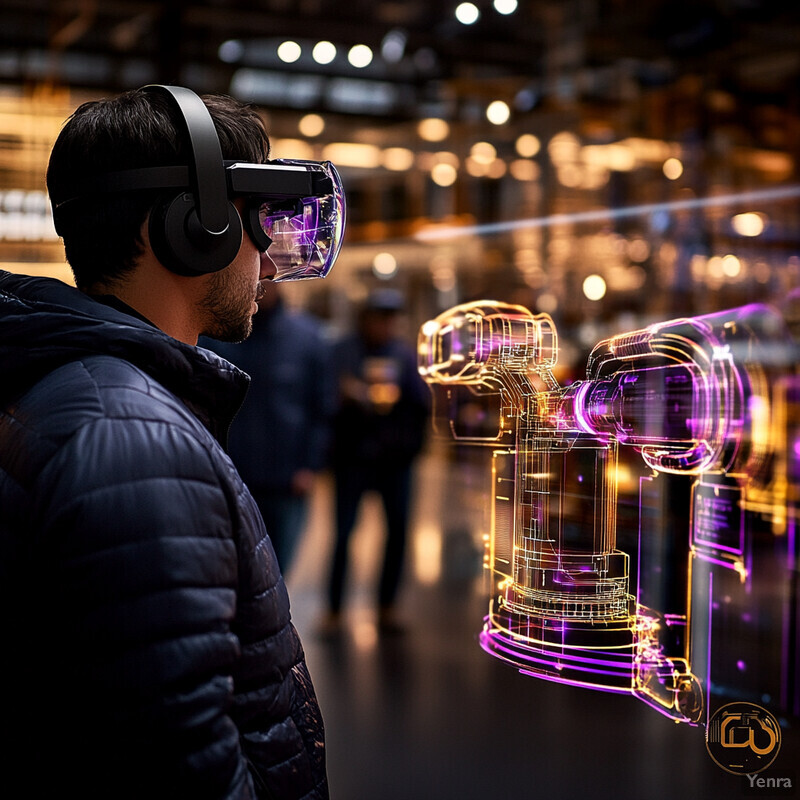
In complex manufacturing projects, integrating new machinery or systems can be risky, time-consuming, and expensive if done purely in the physical world. AI-enhanced digital twins allow engineers to virtually commission equipment long before it’s physically installed. By simulating machine behavior under various load conditions, control strategies, and workflow sequences, the twin reveals potential misalignments, communication gaps, or software bugs. Correcting these issues virtually prevents costly rework, reduces startup delays, and ensures that when the actual machines go online, they operate smoothly and efficiently from day one.
10. Generative Design for Manufacturing
Coupling AI with digital twins allows exploration of new product designs and process configurations, generating innovative solutions that improve performance and reduce material usage.
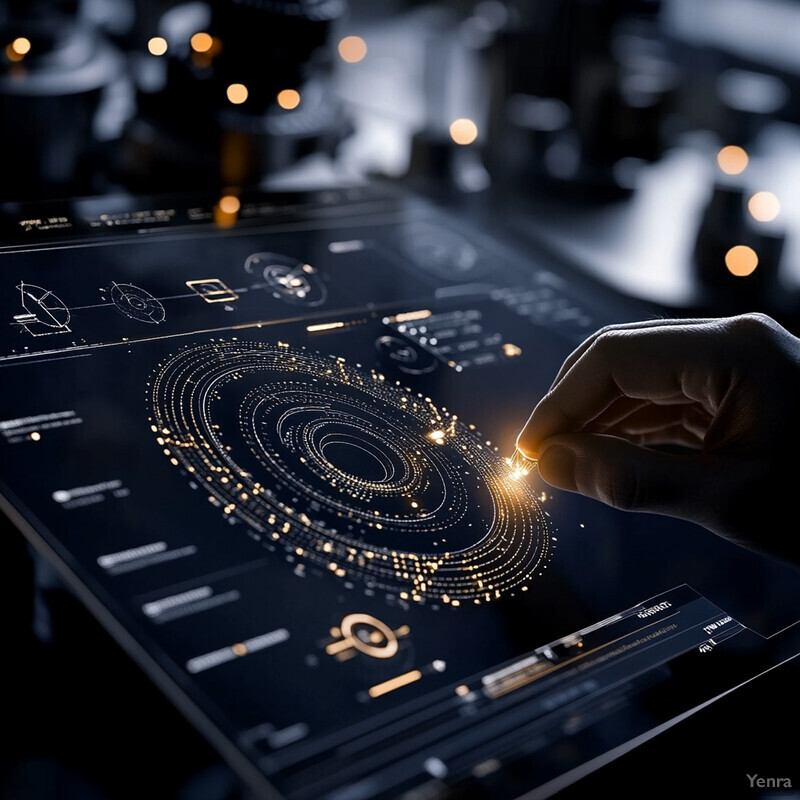
Generative design leverages AI algorithms to propose innovative solutions to engineering challenges by iterating through countless design variations. When combined with digital twins, generative design can also consider real-world constraints and historical performance data to ensure concepts are practical and manufacturable. The twin evaluates each proposed design’s feasibility, cost, and efficiency, guiding AI-driven adjustments until the optimal solution emerges. This synergy accelerates the innovation cycle, leading to lighter, stronger, and more cost-effective products that fully leverage the capabilities of the manufacturing process.
11. Adaptive Quality Control
AI-powered digital twins can automatically adjust quality parameters on the fly, ensuring consistent product quality in the face of variable input materials or changing environmental conditions.
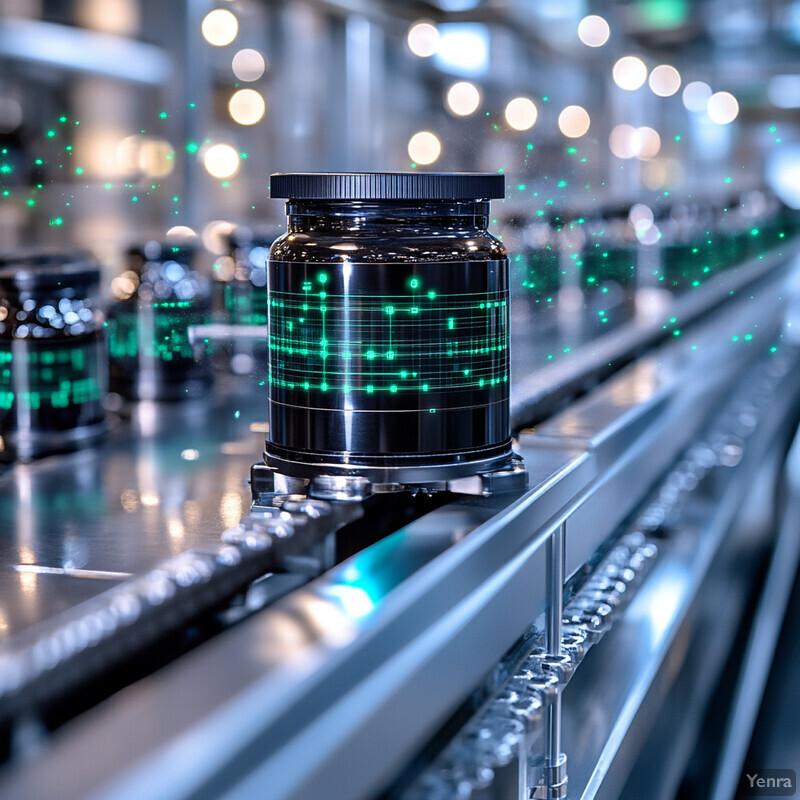
Quality control is often a reactive step—products are inspected at the end of the line and may require rework or scrapping if defects are found. AI-powered digital twins transform quality control into a proactive, continuous process. By monitoring each production stage, analyzing patterns, and correlating process inputs with output quality, the twin learns to anticipate when certain adjustments are needed. It can dynamically tweak machine settings, material feed rates, or curing times to maintain product specifications. As conditions shift—due to subtle environmental changes or material variations—the digital twin’s AI ensures the process stays within quality bounds, minimizing rejections and maximizing customer satisfaction.
12. Supply Chain and Logistics Integration
By modeling the entire value chain within a digital twin, AI can optimize inventory management, logistics, and lead times, improving overall operational efficiency.
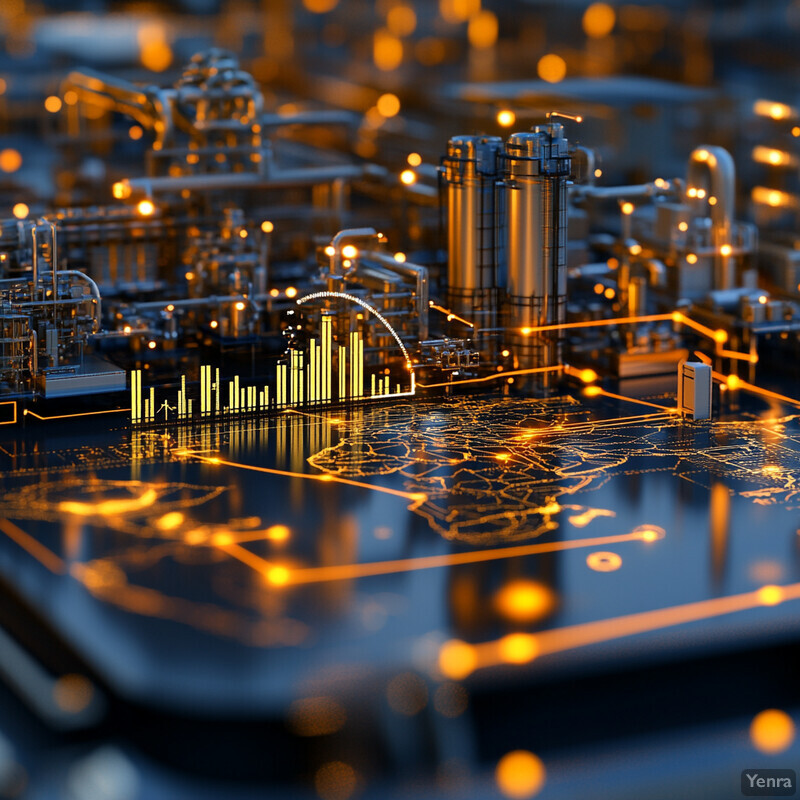
Modern manufacturing isn’t confined to a single factory floor; it stretches across global supply networks. AI-enhanced digital twins enable seamless integration of the production environment with supplier data, inventory levels, transportation timelines, and market demands. By modeling the entire value chain, the twin can forecast supply disruptions, optimize inventory replenishment strategies, and adjust production schedules accordingly. This real-time insight ensures efficient material flows, minimizes lead times, and aligns production output with actual demand. Over time, the entire supply chain becomes more resilient, agile, and cost-effective.
13. Optimized Production Scheduling
Advanced machine learning models within the digital twin can handle complex scheduling challenges, balancing throughput, workforce availability, and machine capacities in real-time.
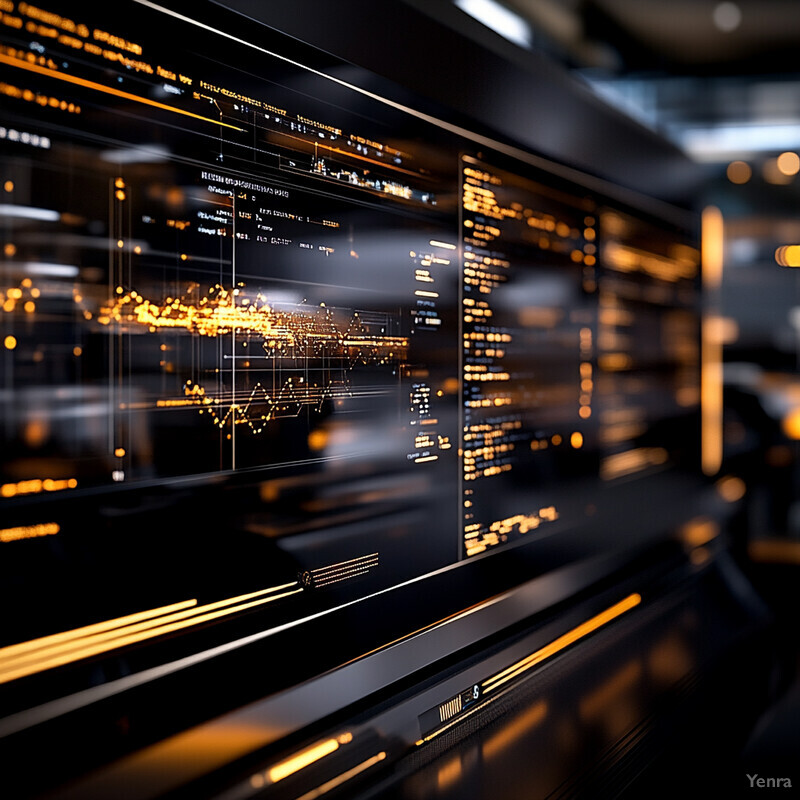
Production schedules are complex puzzles involving machines, workers, materials, and deadlines. AI-driven digital twins analyze this complexity through advanced optimization algorithms that balance competing objectives. They consider factors like machine availability, workforce shifts, maintenance windows, and order priorities to propose the best possible production plans. These schedules can be recalculated on the fly in response to unforeseen events like equipment failures or supplier delays. By continuously seeking the ideal balance, digital twins help manufacturers achieve higher utilization rates, minimize idle time, and improve on-time delivery performance.
14. Energy Usage Optimization
AI-driven twins can analyze energy consumption patterns, suggesting shifts in operation schedules or machine settings that reduce power usage and lower environmental impact.
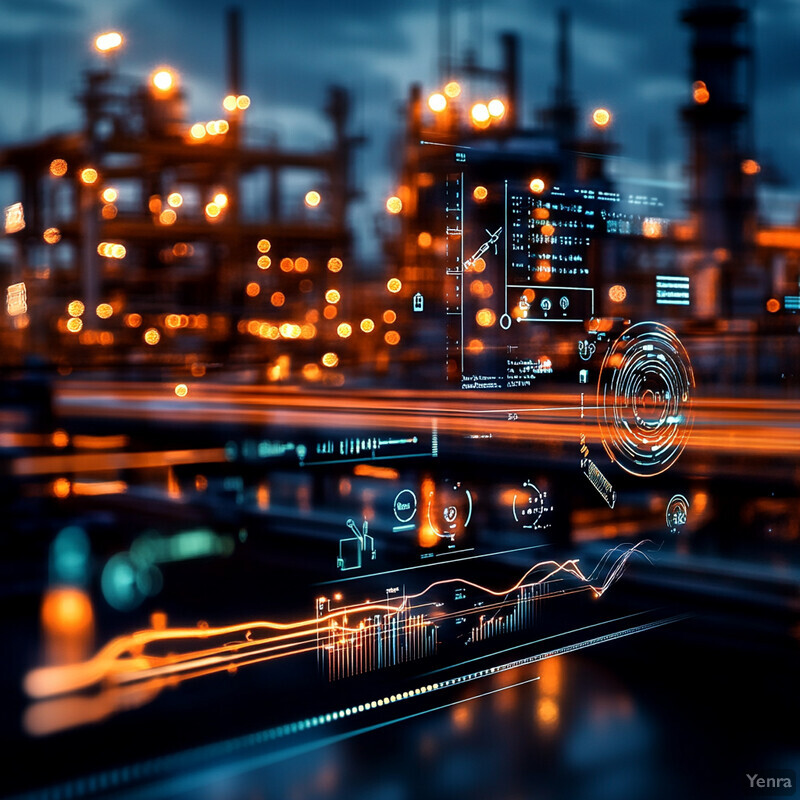
Energy consumption is a significant cost driver in manufacturing operations, and sustainability is an increasingly important concern. AI models within digital twins can analyze patterns of energy use, identifying when and where consumption peaks occur. They can then recommend energy-saving strategies, such as adjusting process timings to off-peak hours, fine-tuning machine operations, or incorporating renewable energy sources. By systematically reducing unnecessary energy usage and aligning production with more efficient resource management, digital twins help manufacturers lower costs, improve environmental footprints, and meet stringent sustainability goals.
15. Adaptive Control Algorithms
As conditions change—due to machine wear, material variability, or external factors—AI models in the digital twin refine control algorithms to maintain consistent performance.
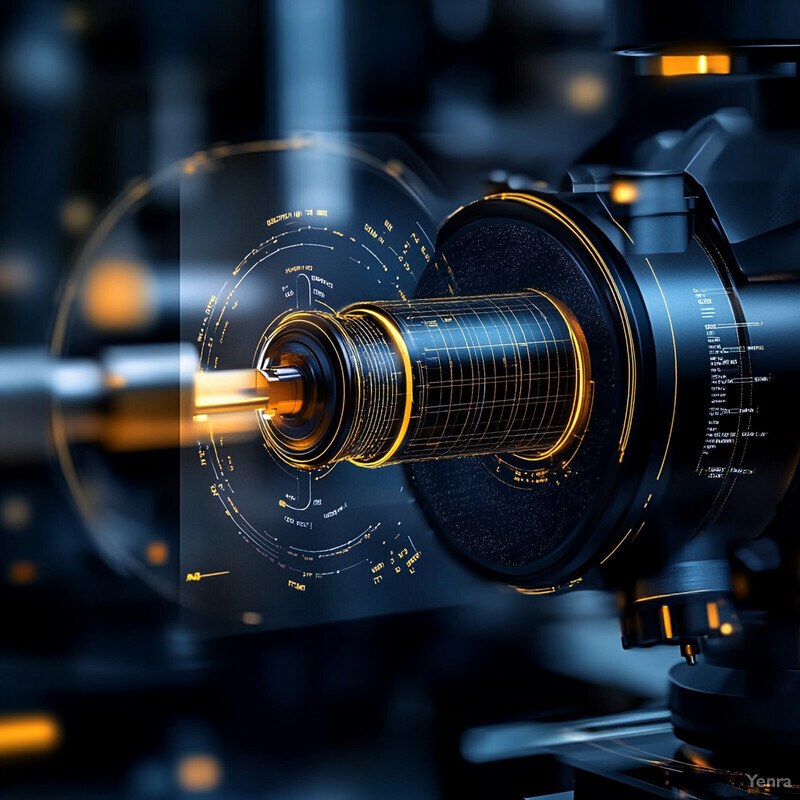
Manufacturing environments are never static; tools wear down, environmental conditions shift, and materials vary in quality. AI-empowered digital twins accommodate this volatility by constantly refining their control algorithms. Instead of relying on fixed control loops, the twin’s machine learning models update control parameters as inputs evolve. For instance, the twin might learn that a cutting tool starts to produce slightly less accurate parts after a certain number of cycles and can automatically adjust speeds or replace the tool at the right moment. This adaptability keeps the process in an ideal state, ensuring peak performance regardless of changing conditions.
16. Workforce Training Simulations
AI-enhanced digital twins can provide realistic training environments for operators, simulating machine responses and process variations to build operator skills safely and efficiently.
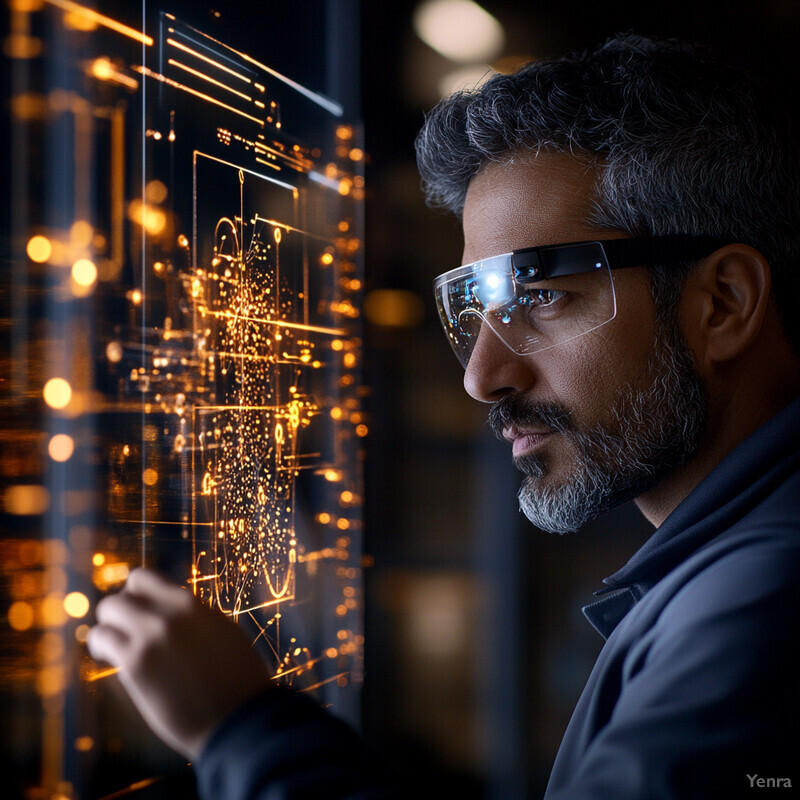
Digital twins are not only for machinery and processes; they can also model the human factor in manufacturing. AI-enhanced training environments simulate realistic production scenarios, allowing operators to practice complex tasks, troubleshoot equipment issues, and learn new procedures without risking production delays or equipment damage. By giving employees a safe virtual sandbox, the digital twin helps build their confidence, competence, and problem-solving skills. Over time, a better-trained workforce contributes to improved productivity, quality, and safety in the actual manufacturing environment.
17. Lifecycle Cost Analysis
Intelligent digital twins can assess long-term operational costs, from maintenance intervals to energy consumption, enabling strategic planning and cost savings over a product’s entire lifecycle.
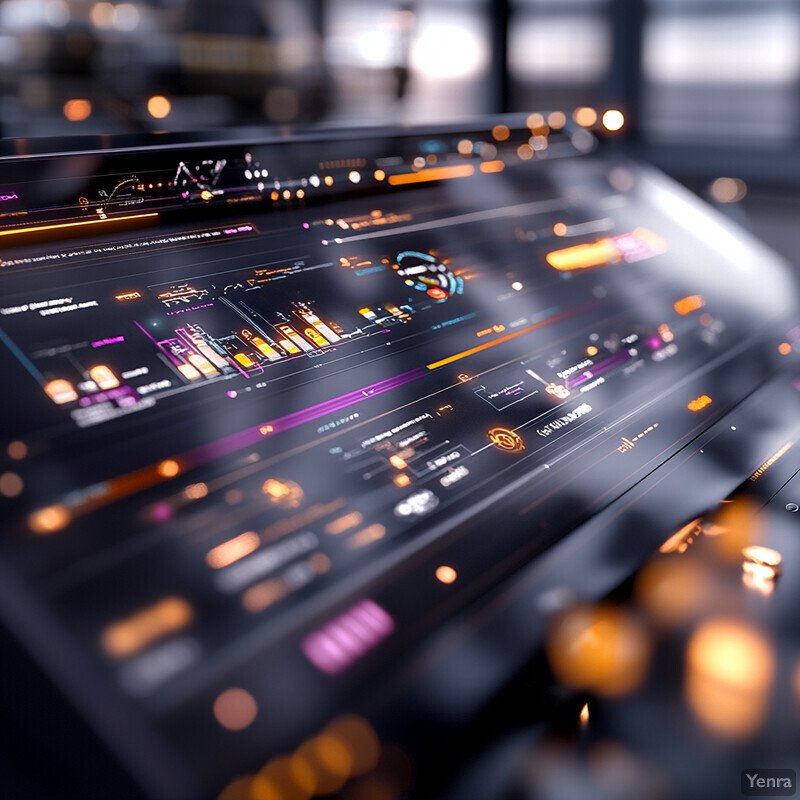
The economic impact of manufacturing decisions extends far beyond immediate production runs. AI-driven digital twins facilitate comprehensive lifecycle cost analysis by modeling not just the short-term variables—like raw material expenses and labor costs—but also long-term factors, such as equipment depreciation, maintenance frequencies, energy consumption, and product returns or warranty claims. This holistic view empowers decision-makers to optimize for total cost of ownership. Strategic investments and design changes can then be made with an eye toward long-term profitability, resource utilization, and overall business sustainability.
18. Material and Resource Optimization
AI models help determine the best mix of materials or manufacturing steps to achieve desired product properties while minimizing cost, environmental impact, and material waste.
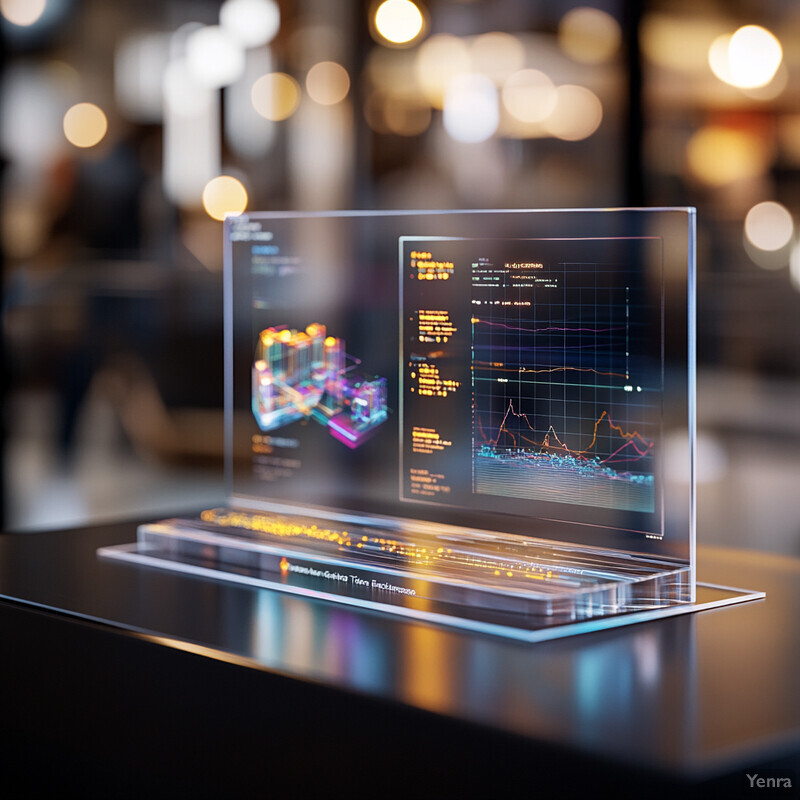
Selecting the right materials, suppliers, and production methods can mean the difference between profit and loss. AI-enhanced digital twins help manufacturers identify optimal combinations of materials and process settings to achieve desired product attributes while minimizing waste, cost, and environmental impact. By evaluating different material suppliers, recycling scenarios, and machining strategies, the twin ensures that each decision supports the company’s operational goals. As a result, manufacturers can produce higher-quality goods with fewer defects, less scrap, and lower energy inputs.
19. Continuous Improvement Insights
Over time, AI within digital twins can identify patterns and trends in production data, providing actionable insights to continually enhance process stability, yield, and throughput.
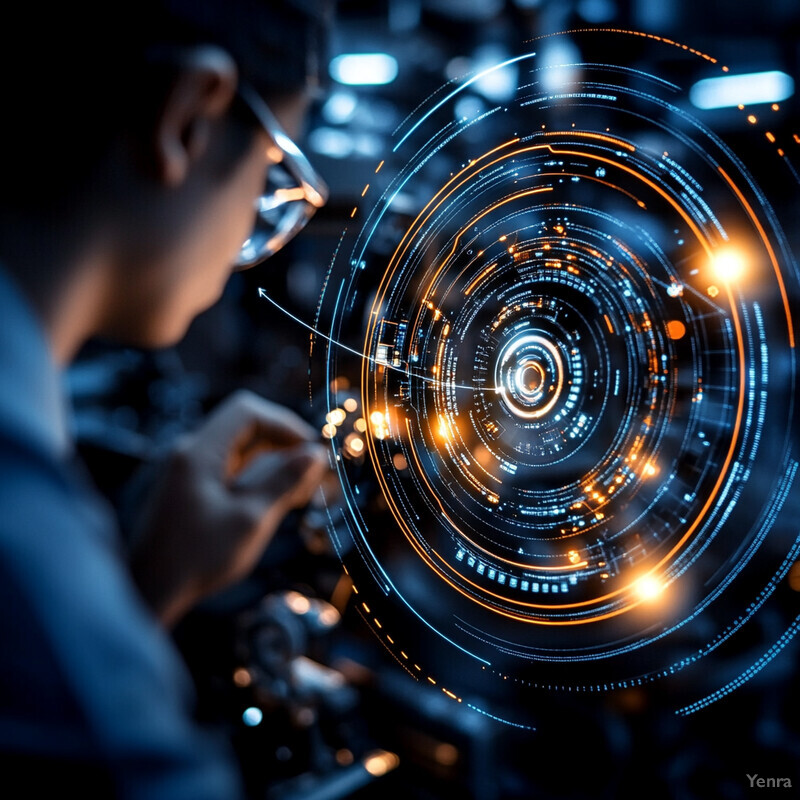
In complex manufacturing environments, continuous improvement efforts often require digging deep into historical data, evaluating new strategies, and systematically testing changes. With AI, digital twins become powerful engines of continuous improvement by automatically surfacing meaningful insights and patterns. The twin may highlight that a slight shift in temperature control consistently improves yield or that a certain supplier’s materials correlate with fewer defects. Armed with these evidence-based recommendations, engineers and managers can implement targeted improvements, measure their impact, and foster a culture of data-driven innovation that steadily boosts performance over time.
20. Faster Iteration and Innovation
With the predictive power and real-time feedback loops provided by AI, digital twins enable more rapid prototyping, testing, and refinement, accelerating time-to-market for new products.
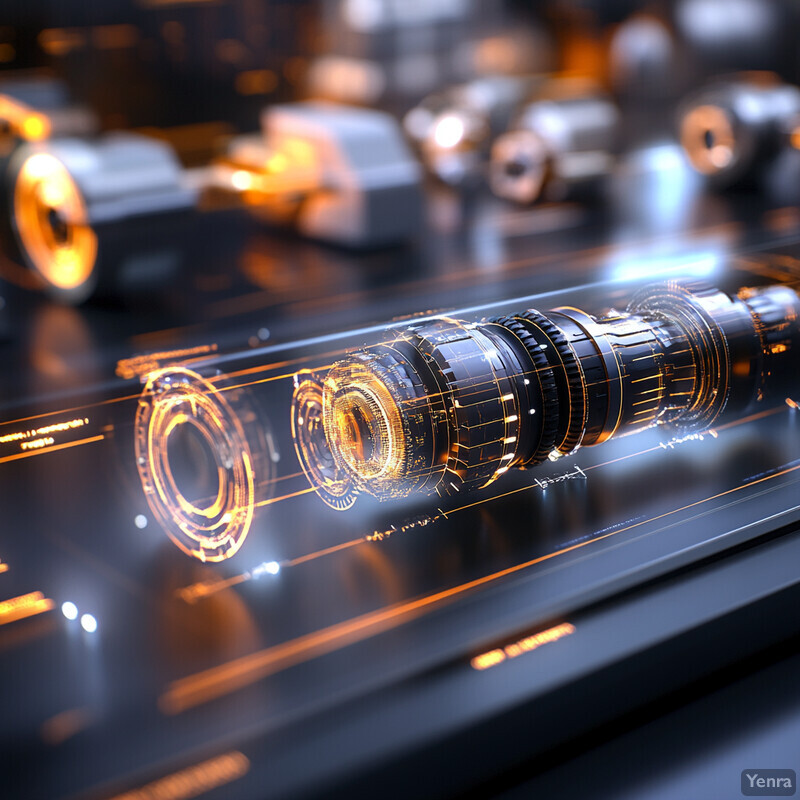
Rapid product development and process innovation are essential in competitive markets. AI-augmented digital twins accelerate this cycle by providing immediate feedback on design changes, production strategies, and process adjustments. Instead of waiting weeks or months to see the outcome of a modification in the real world, engineers can iterate virtually at lightning speed. The digital twin’s simulated feedback helps refine ideas quickly, guiding projects to market-readiness faster. This compressed innovation cycle ultimately leads to more resilient, efficient, and customer-focused products, giving manufacturers a critical edge in a constantly evolving landscape.