1. Automated Metadata Tagging
AI-driven image and video recognition technologies can automatically generate descriptive tags, freeing teams from manual keywording and improving content discoverability.
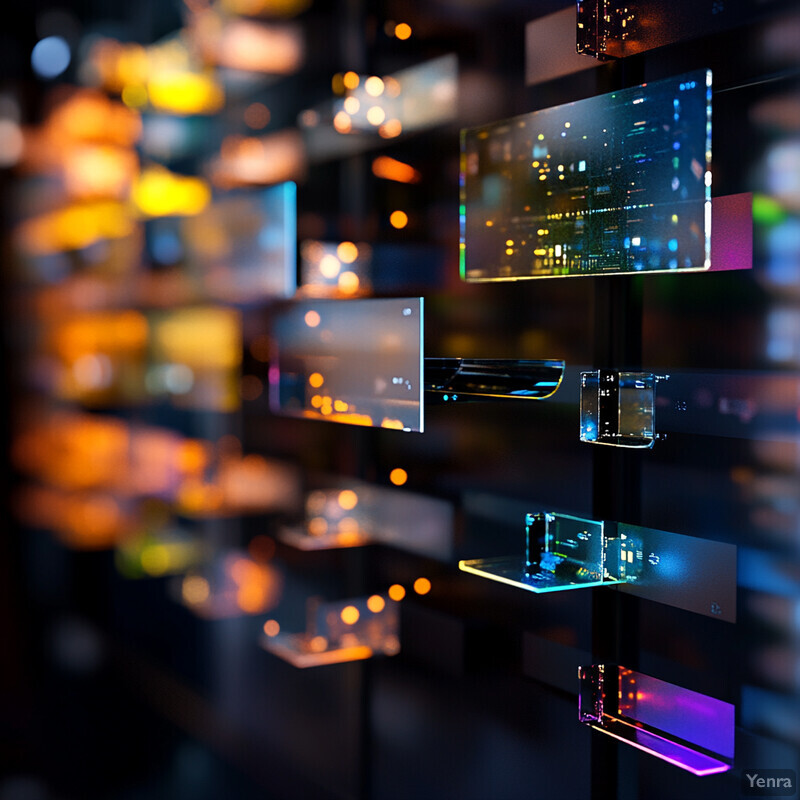
Traditionally, teams spent countless hours manually tagging images, videos, and documents with relevant metadata to facilitate future discovery. AI-powered image and video recognition has transformed this tedious process by automatically identifying objects, scenes, faces, and text within assets. These automated tags save time, reduce human error, and create more comprehensive, granular metadata than a human could easily provide. As a result, the DAM repository becomes more structured, improving search accuracy and enabling faster, more intuitive browsing of large asset collections.
2. Intelligent Search and Retrieval
Natural language processing (NLP) and semantic search capabilities enable users to search for assets using full sentences or concepts, rather than relying on exact keyword matches.
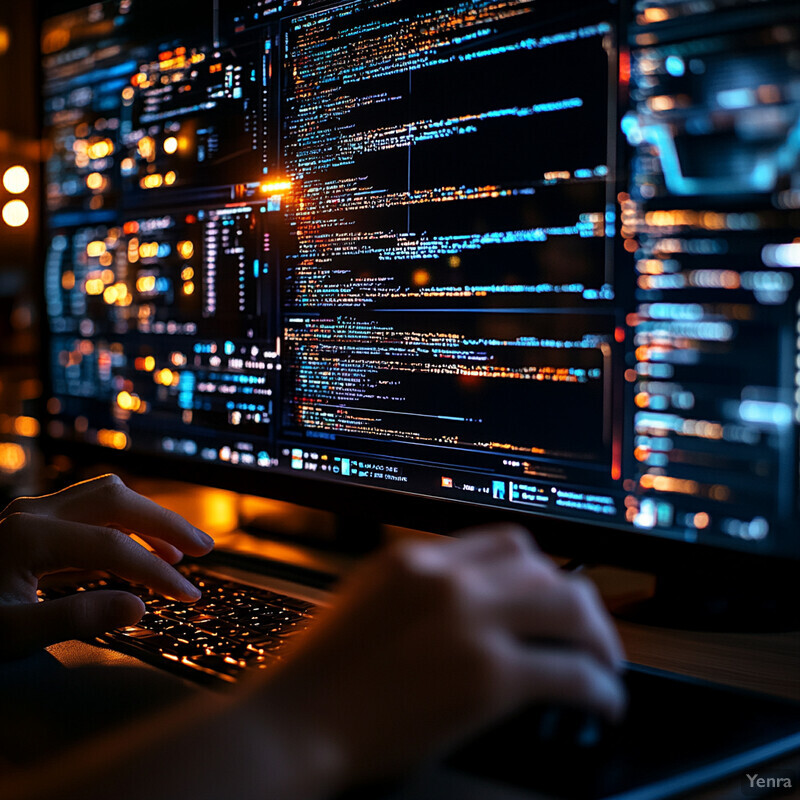
As Digital Asset Management systems grow in complexity, finding the right asset at the right time is increasingly challenging. AI’s natural language processing (NLP) and semantic understanding capabilities make searches more intuitive. Instead of relying solely on exact keywords, users can search with phrases, questions, or conceptual descriptions—such as “images of happy families enjoying nature”—and receive relevant results. This intelligence dramatically shortens the path from the initial search query to the final retrieved asset, improving productivity and user satisfaction.
3. Facial and Object Recognition
Computer vision models identify people, logos, objects, and locations in images and videos, enabling more granular indexing and easier retrieval.
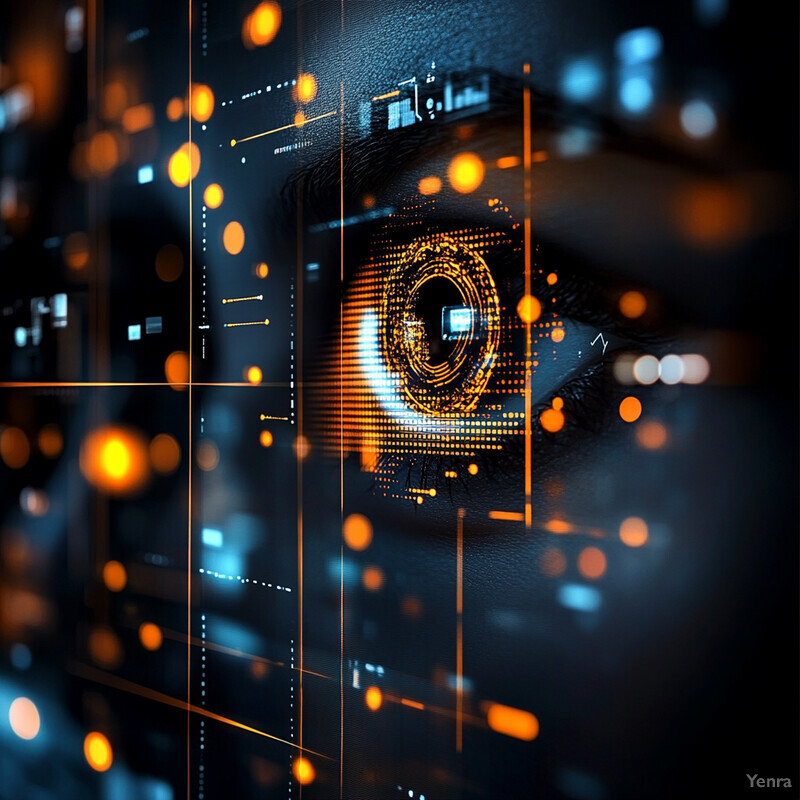
In large asset libraries, pinpointing a specific individual, logo, product, or location can be like finding a needle in a haystack. Advanced computer vision models allow the DAM to identify faces, objects, and brand elements within images and videos. Once recognized and tagged, these features are searchable, enabling brand managers, content creators, and marketers to instantly locate assets containing a particular spokesperson, product model, or environment. This detailed visual metadata turns previously opaque image libraries into well-organized, easily navigable collections.
4. Voice and Speech Transcription
AI can automatically transcribe and timestamp speech in audio and video files, turning them into searchable, text-based assets.
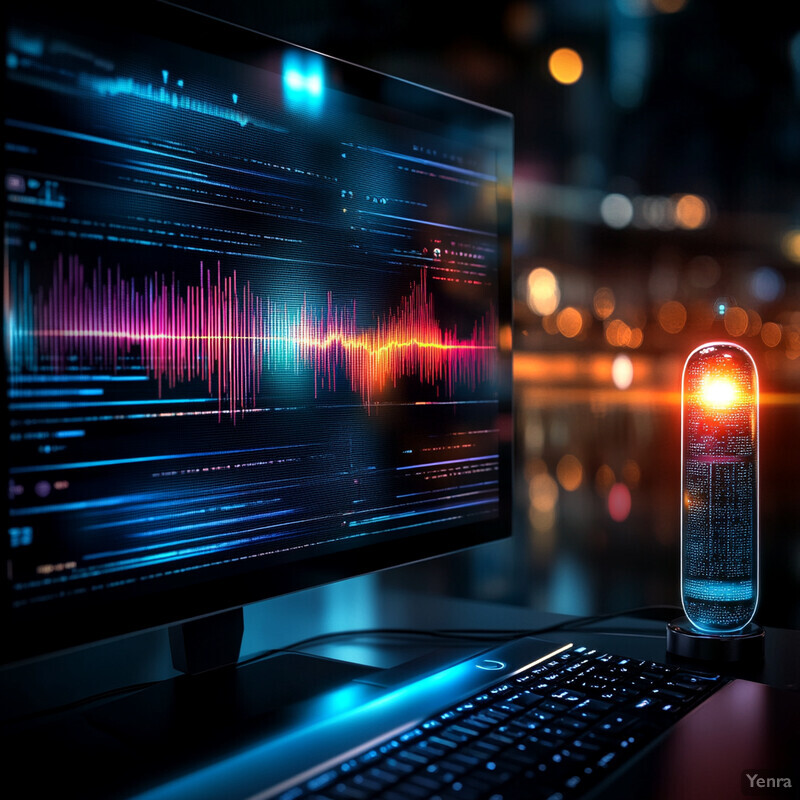
Audio and video assets often contain valuable dialogues, interviews, or presentations that remain hidden without a transcript. AI-driven speech-to-text services convert spoken words into searchable text, enriching audio and video files with descriptive metadata. These transcripts can be tagged, indexed, and integrated into the DAM, making it possible to rapidly locate a single spoken line, identify relevant sound bites, or align content with written materials. This enhancement unlocks the latent value of multimedia assets and streamlines editing, repurposing, and compliance processes.
5. Content Personalization
Machine learning algorithms analyze user preferences and past usage patterns to recommend the most relevant digital assets for each specific audience segment.
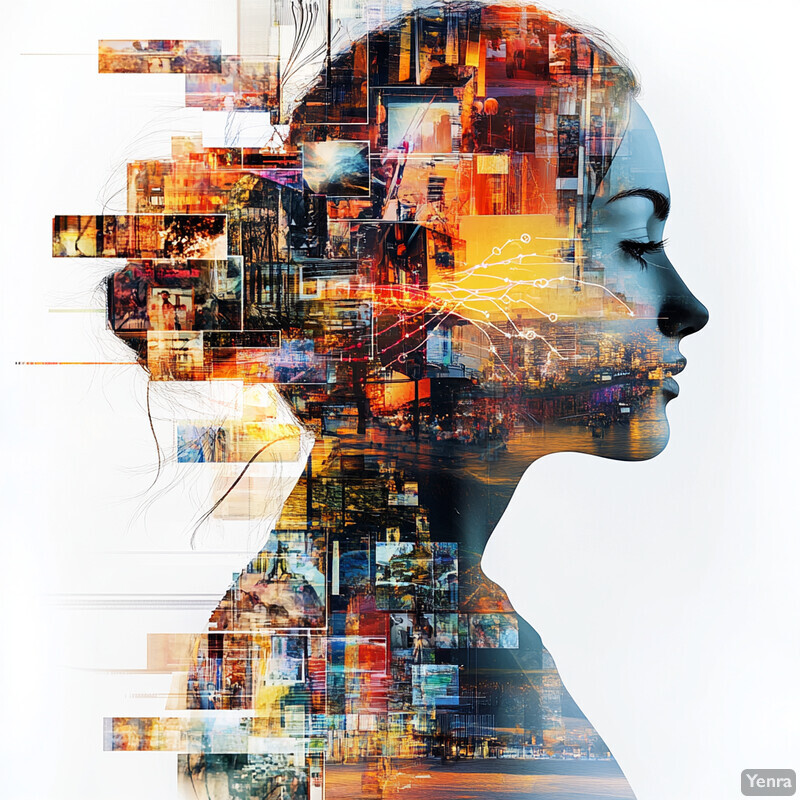
Different audiences demand different types of content. AI can analyze historical user behaviors, preferences, and past asset usage patterns to predict which materials will best meet their needs. By recommending the most relevant assets, AI helps marketers tailor campaigns for specific segments, sales teams access the most applicable collateral for certain industries, and creatives quickly find references that match their stylistic direction. This personalization leads to more efficient workflows and improved audience engagement.
6. Automated Classification and Organization
Clustering algorithms group related assets together, helping users quickly navigate large repositories and intuitively browse the collection.
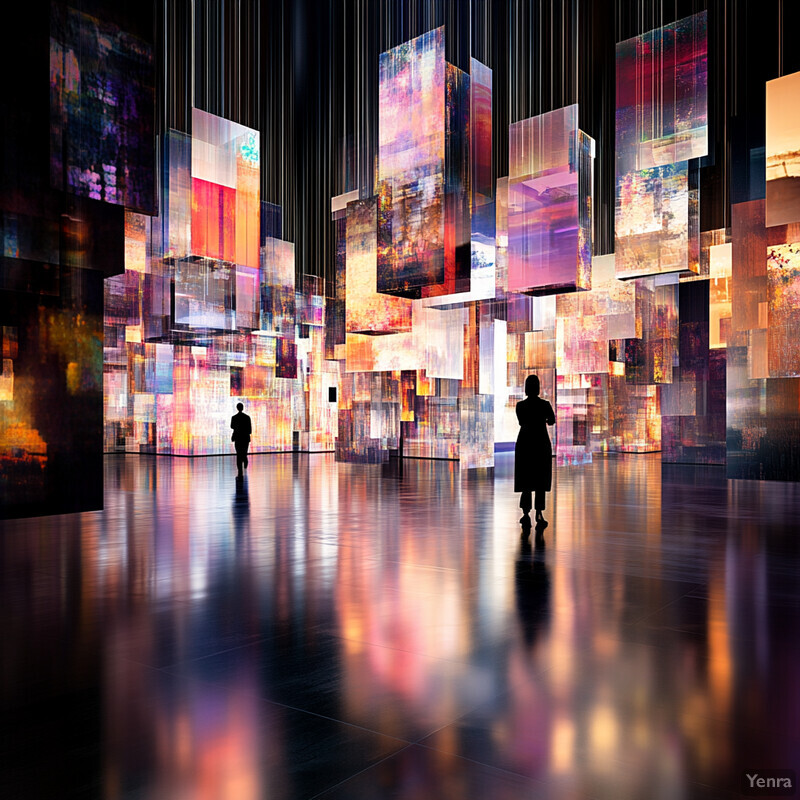
When dealing with thousands or even millions of assets, manual categorization becomes impractical. AI-driven clustering algorithms group similar assets based on their visual or textual content, enabling more intuitive navigation of the DAM. Instead of sifting through flat folder structures, users can browse dynamic groupings—such as images containing a particular product line, or graphics related to a marketing campaign—making it simpler to discover related content and reduce the time spent managing complex directories.
7. Version Control and Asset Lineage Tracking
AI can detect differences between asset versions and maintain a detailed history of edits, ensuring teams always work with the most current iteration.
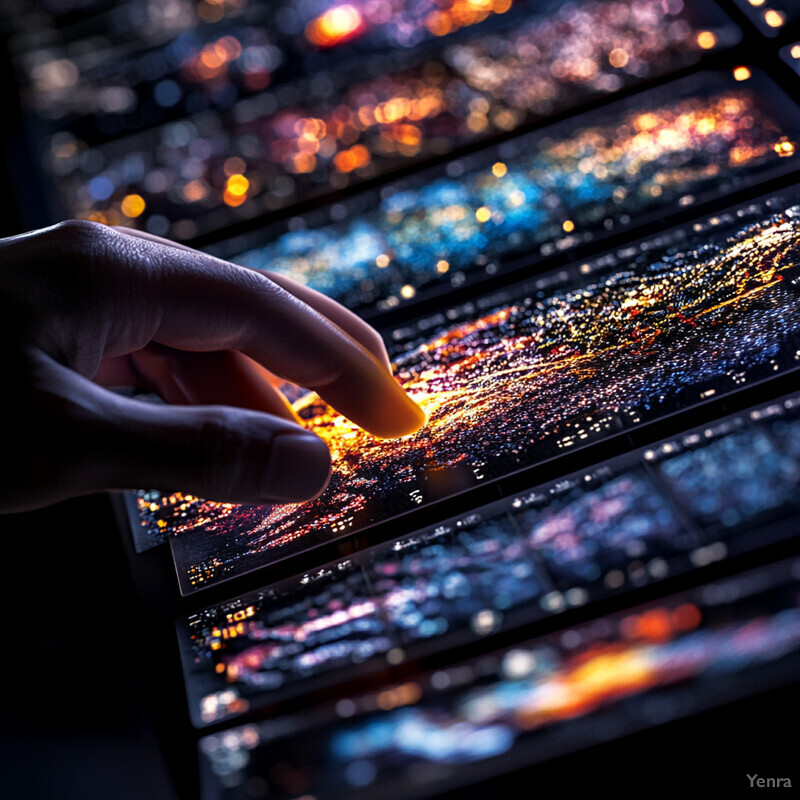
Creative workflows often involve multiple iterations of a single asset. AI can help identify differences between versions, track revisions over time, and document the lineage of changes. By understanding the relationships between versions—such as which video clip was derived from a master file or how an image was edited—teams can ensure that everyone is working with the most current, approved version. This type of asset intelligence prevents errors, maintains brand consistency, and supports compliance with editorial standards.
8. Predictive Analytics for Asset Utilization
By analyzing historical usage data, AI can forecast demand for particular assets, informing storage optimization and content strategy.
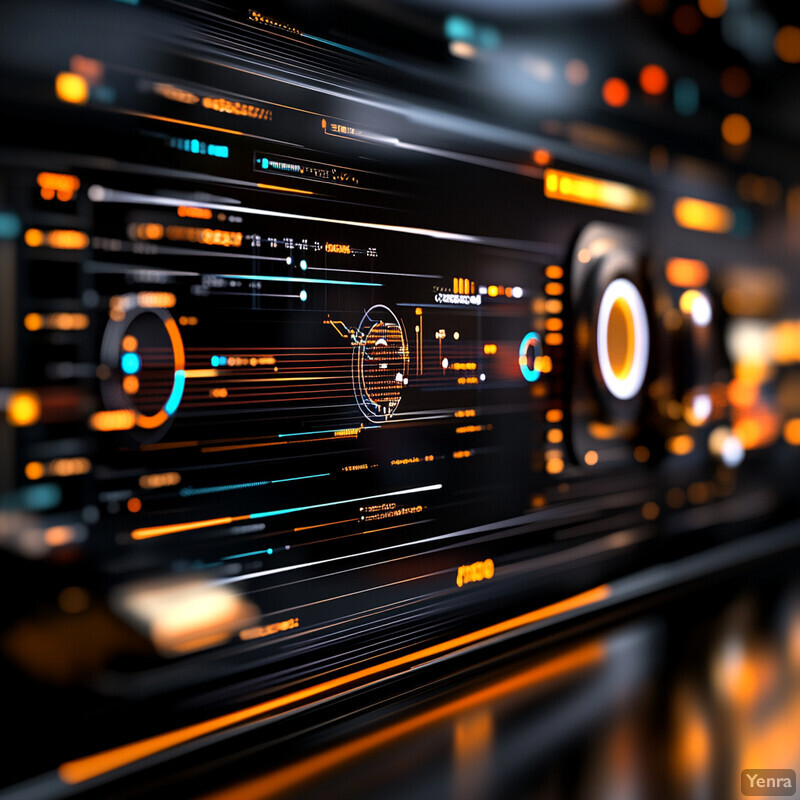
AI models can analyze historical data about how and when certain assets have been used to predict future demand. For example, by identifying seasonal trends, marketing initiatives, or usage patterns, the DAM can suggest which assets might be in high demand during an upcoming campaign. These insights help teams anticipate storage and retrieval needs, optimize their content strategies, and ensure that frequently accessed assets are easily available—leading to cost savings and more effective resource allocation.
9. Duplicate and Near-Duplicate Detection
Machine learning models identify visually or textually similar files, reducing redundant storage and decluttering the DAM environment.
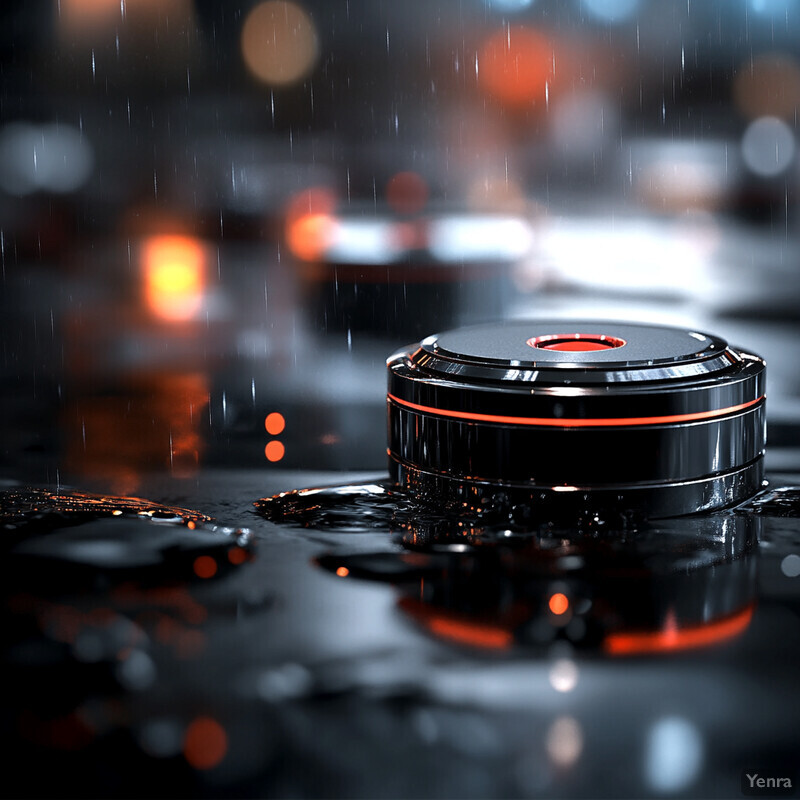
As DAM systems grow, it’s common for identical or highly similar versions of the same file to accumulate. AI-driven similarity detection algorithms can spot these duplicates, alert users, and assist in de-duplication efforts. By removing or consolidating redundant assets, organizations free up storage space, improve retrieval speed, and reduce confusion about which asset is the 'right' one to use. This leads to cleaner libraries, lower storage costs, and a more streamlined creative process.
10. Image and Video Enhancement
AI-driven image processing tools can improve quality, adjust color automatically, and even perform intelligent cropping or resizing.
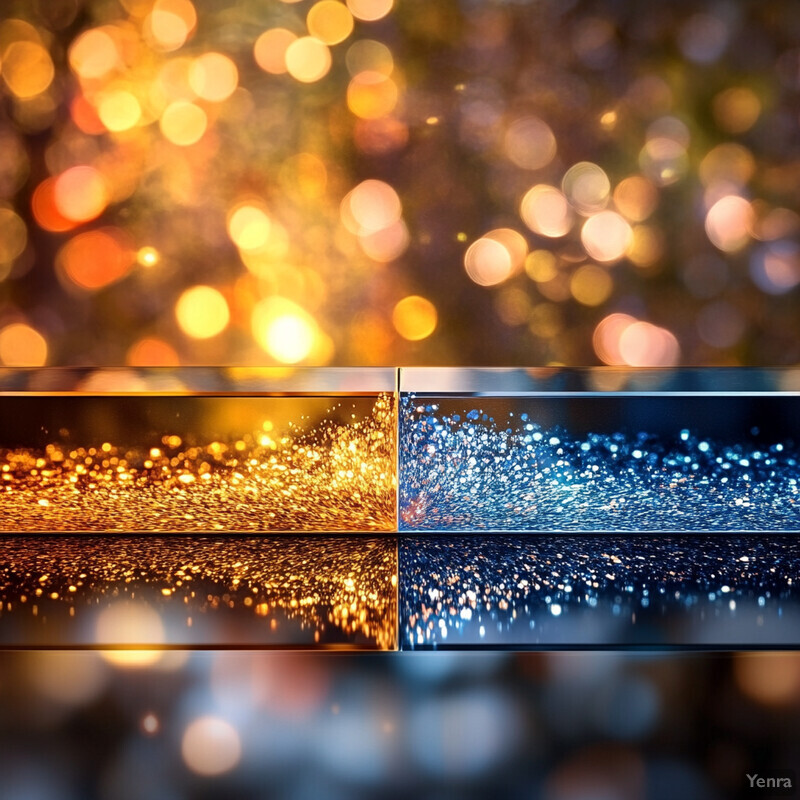
AI-powered image processing tools can optimize and enhance digital assets by adjusting parameters like brightness, contrast, and color balance automatically. They can also perform intelligent cropping, resizing, or background removal with minimal manual intervention. As a result, DAM systems can store higher-quality, ready-to-use visuals. This capability not only reduces the time spent in post-production editing but also ensures that end-users have immediate access to polished, professional-looking assets.
11. Brand Compliance Checks
AI can verify brand consistency by recognizing logos, color schemes, and brand elements, ensuring that all distributed materials adhere to brand guidelines.
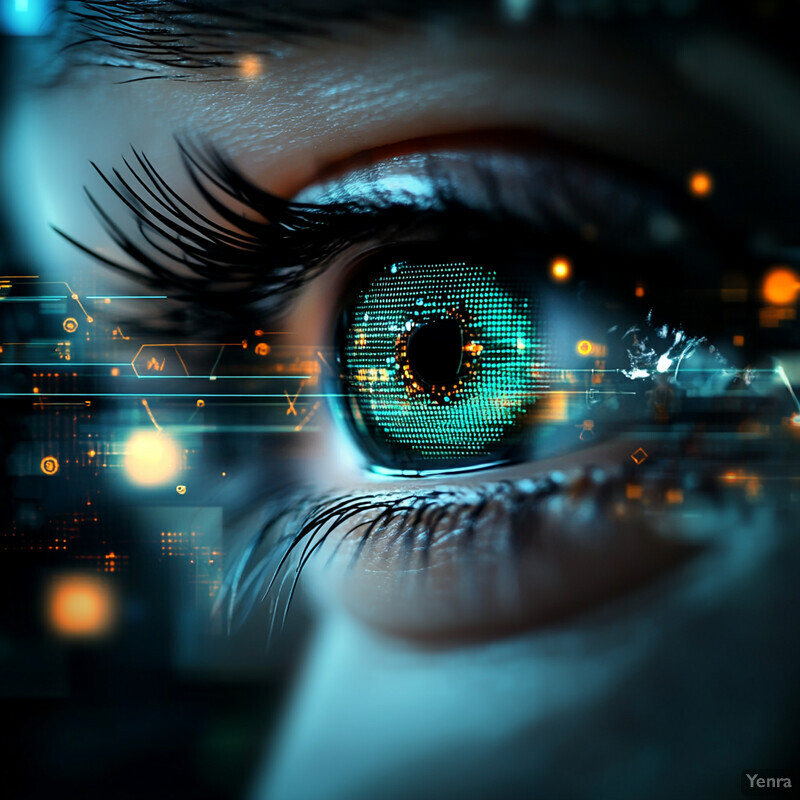
Maintaining brand consistency across all external and internal materials is a key challenge. AI can help by recognizing logos, color palettes, fonts, and design elements within stored assets. If an asset deviates from brand guidelines—perhaps using an outdated logo or non-approved font—the DAM can flag it automatically. This proactive approach helps brand managers and marketing teams maintain a cohesive visual identity and prevents off-brand content from circulating, bolstering both trust and professionalism.
12. License and Rights Management
Intelligent systems can detect copyrighted material or track licensing restrictions, alerting users when assets are used beyond allowed terms.
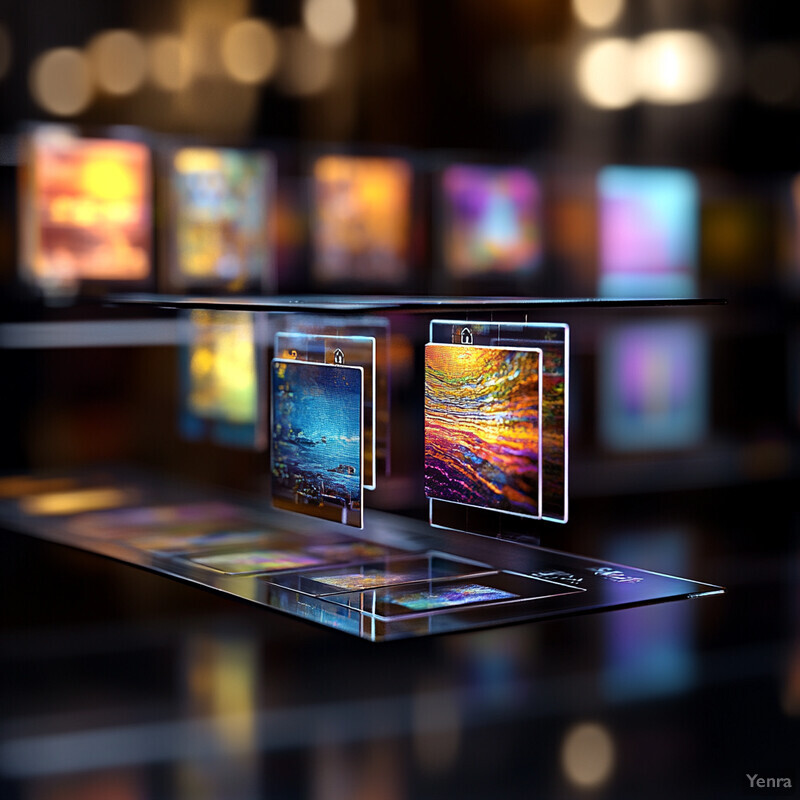
Organizations often license images, videos, or music for specific uses and time frames. AI can assist by reading embedded metadata, identifying copyright markers, and tracking usage conditions. With automated alerts, the DAM can warn users if an asset’s license is about to expire or if it’s being used in an unauthorized manner. This leads to more responsible asset usage, fewer legal risks, and cost savings by preventing misuse of licensed materials.
13. Automated Content Summarization
AI can create short summaries or highlight reels from long-form content, making it easier to preview and repurpose assets.
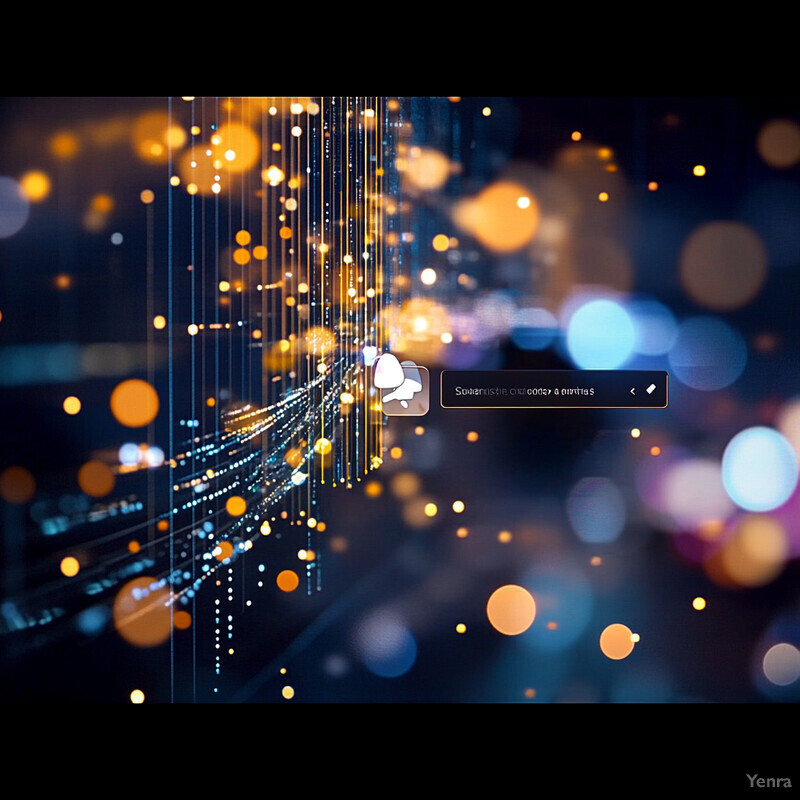
Large volumes of long-form content—such as video footage, lectures, or webinars—are cumbersome to review in their entirety. AI-driven summarization tools can generate concise overviews, highlight key moments, or produce highlight reels. Incorporating these summaries into the DAM makes it easier for users to quickly assess the relevance of content and find what they need. This capability also supports faster decision-making, content curation, and the discovery of previously underutilized materials.
14. Contextual Recommendations
Understanding the semantic context of user queries, AI can suggest supplementary assets—like related images, data sheets, or case studies.
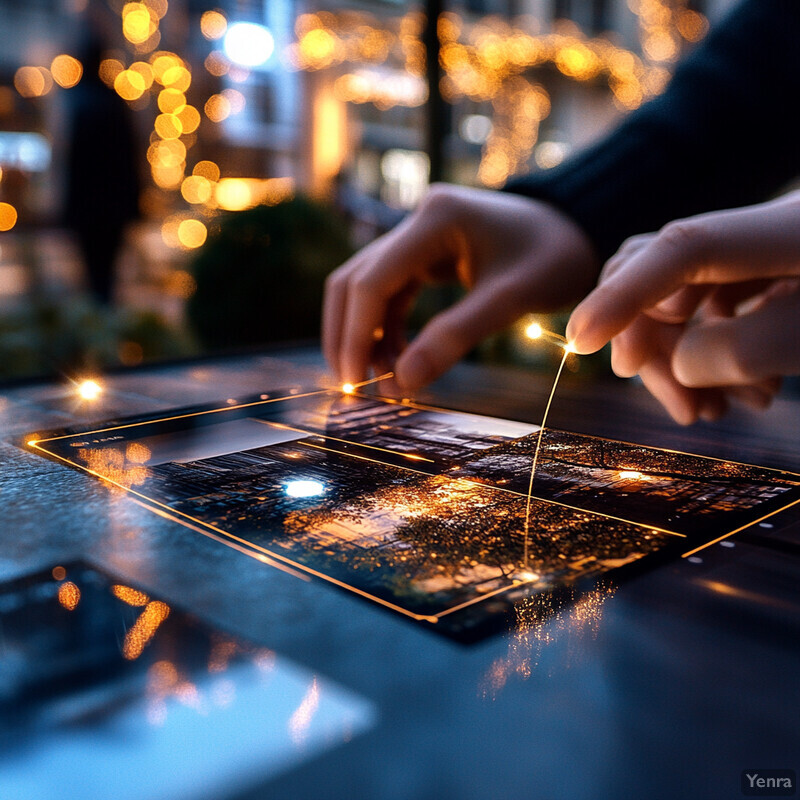
Beyond simple keyword matches, AI can use semantic context to suggest supplementary or related assets. For example, if a user is viewing a product launch video, the DAM might recommend related product images, data sheets, or case studies that help present a complete marketing package. This contextual understanding fosters more holistic and strategic use of assets, guiding users toward comprehensive sets of materials that work in synergy.
15. Multilingual Support and Translation
NLP models can translate metadata, tags, and transcripts, facilitating global collaboration and asset distribution.
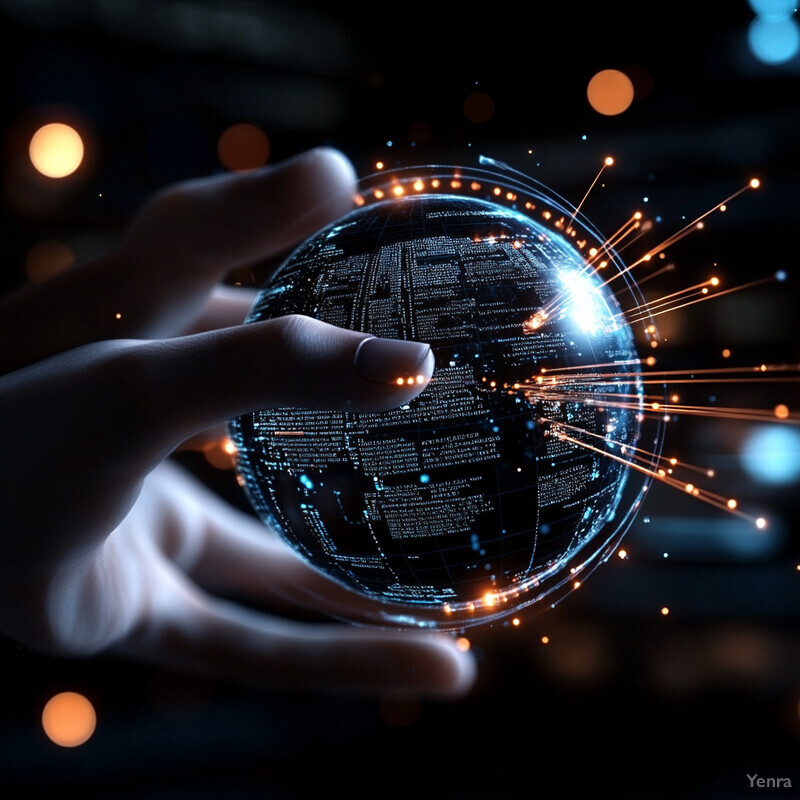
In today’s global marketplace, teams often need to collaborate across languages. AI-driven natural language processing can translate metadata, transcripts, and even image text, making it easier to search and manage assets in multiple languages. This functionality enables organizations to store, access, and distribute content worldwide without language barriers, ensuring that everyone has equal access to vital assets.
16. Sentiment Analysis and Tone Detection
Identifying the emotional or thematic tone within images, videos, or written content helps tailor asset selection to specific campaign goals.
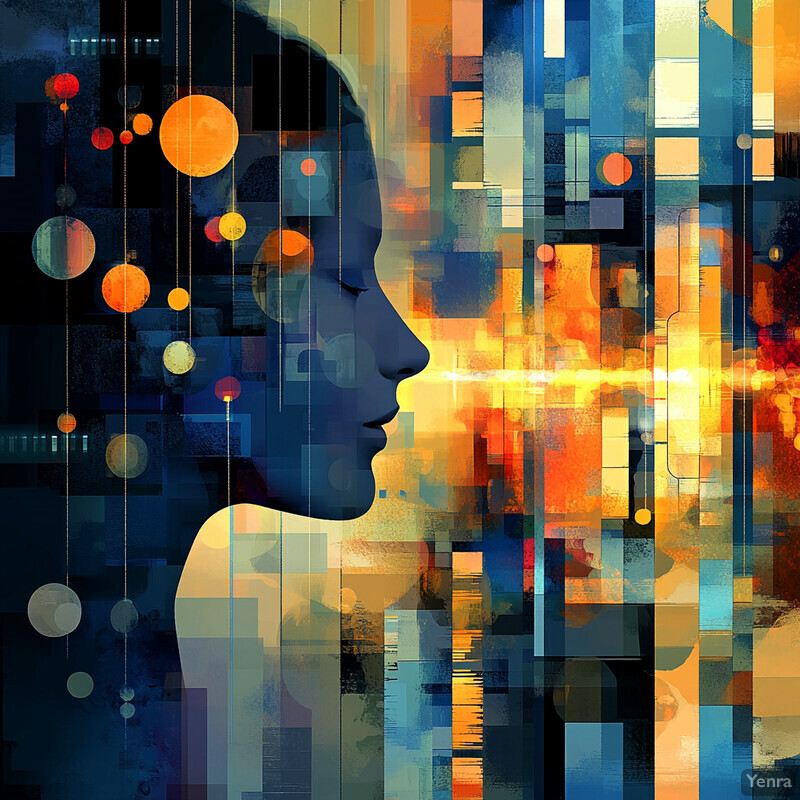
Understanding the emotional tone of visual or audio content can be essential for marketers and content strategists. AI models trained in sentiment analysis can detect whether images or videos convey joy, nostalgia, tension, or excitement. By categorizing assets by mood or sentiment, users can more strategically choose visuals for campaigns, ensuring that the emotional impact aligns with brand messaging and audience expectations.
17. Smart Archiving and Lifecycle Management
Predictive models determine when assets become outdated or rarely used, prompting systematic archiving and conserving storage.
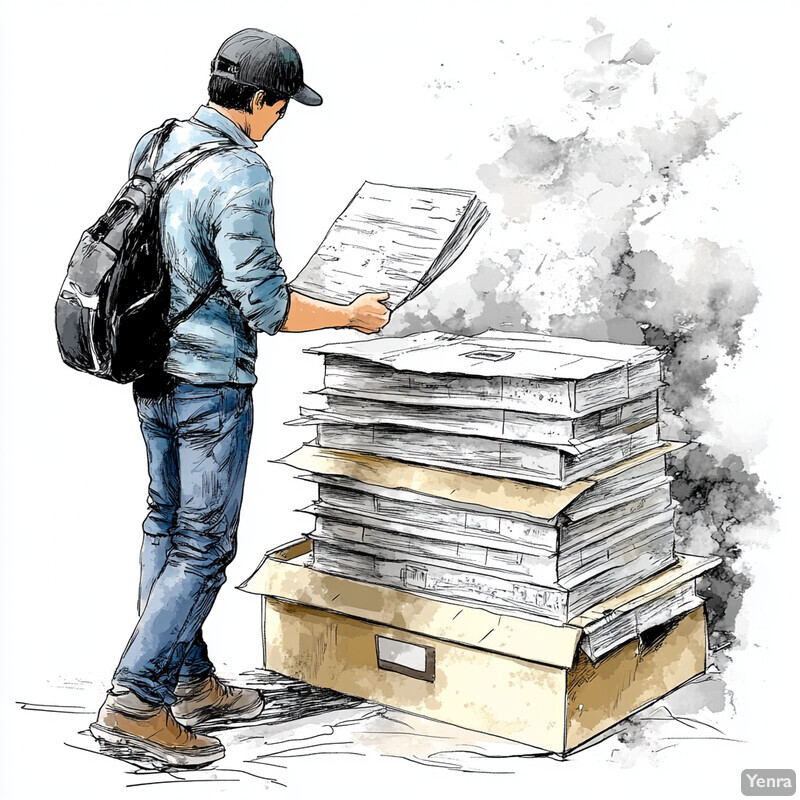
Digital assets have lifecycles—some will be frequently used for a period and then become obsolete. AI can predict when assets are likely to see declining usage, prompting archival or suggesting when it’s safe to retire them. By automating lifecycle management, organizations keep their DAM lean, ensuring that actively used assets are readily available while obsolete or infrequently accessed files move to cost-effective storage tiers or are removed altogether.
18. Automatic Content Categorization by Vertical
AI can classify assets according to industry, campaign, product line, or department, simplifying targeted asset retrieval.
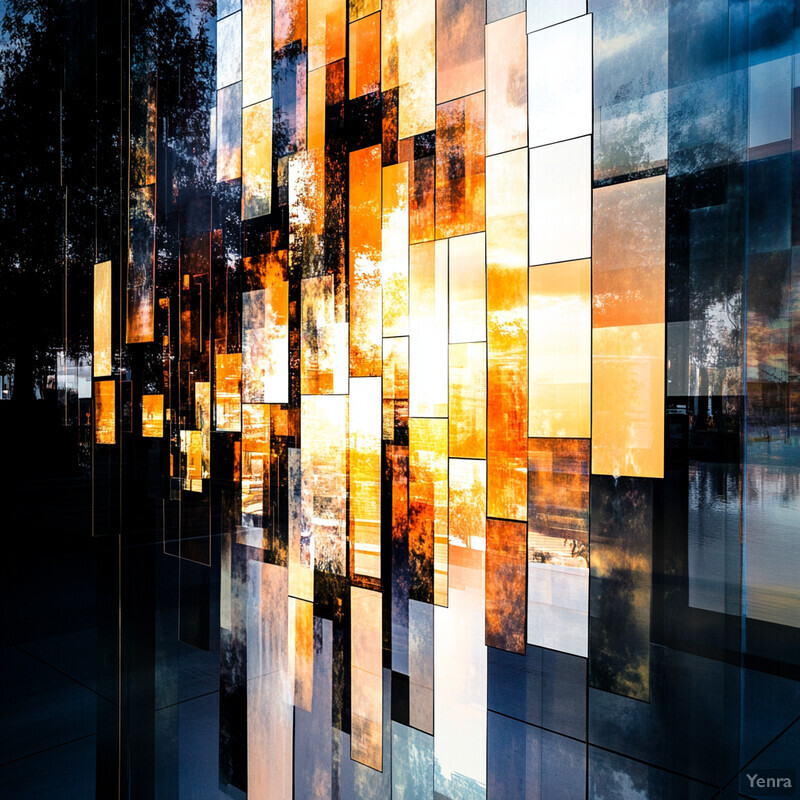
Different teams or departments may organize assets by product line, campaign, or industry vertical. AI can streamline this categorization by analyzing the content and context of assets, then grouping them accordingly. When assets are sorted by these meaningful categories, team members can quickly locate files relevant to their unique needs, increasing efficiency and removing friction in cross-departmental collaboration.
19. Generative Asset Modification
Generative AI tools can quickly produce variations of existing assets—such as alternative backgrounds or updated text overlays—streamlining creative workflows.
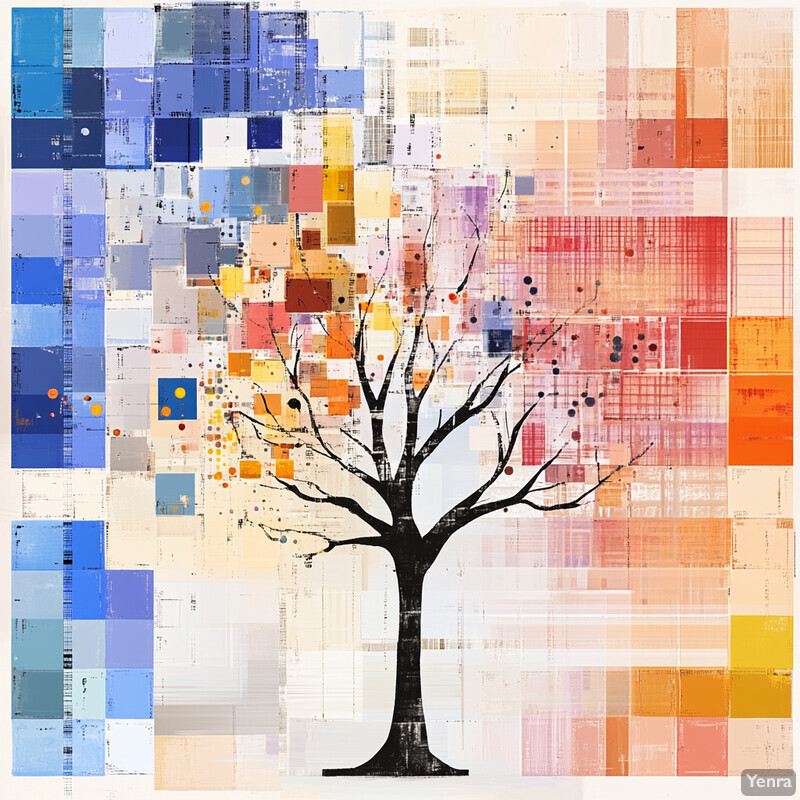
With the rise of generative AI models, DAM systems can offer on-the-fly content modifications, such as creating alternate backgrounds, resizing images to fit different formats, or generating variations of a graphic. This allows for rapid experimentation and adaptation of core assets without the need for intensive manual editing. It empowers teams to produce multiple tailored versions of content quickly, streamlining creative workflows and fostering greater agility in meeting audience demands.
20. Enhanced Reporting and Dashboards
By extracting insights on asset performance, AI-driven analytics provide actionable recommendations for content strategy, asset reusability, and overall ROI optimization.
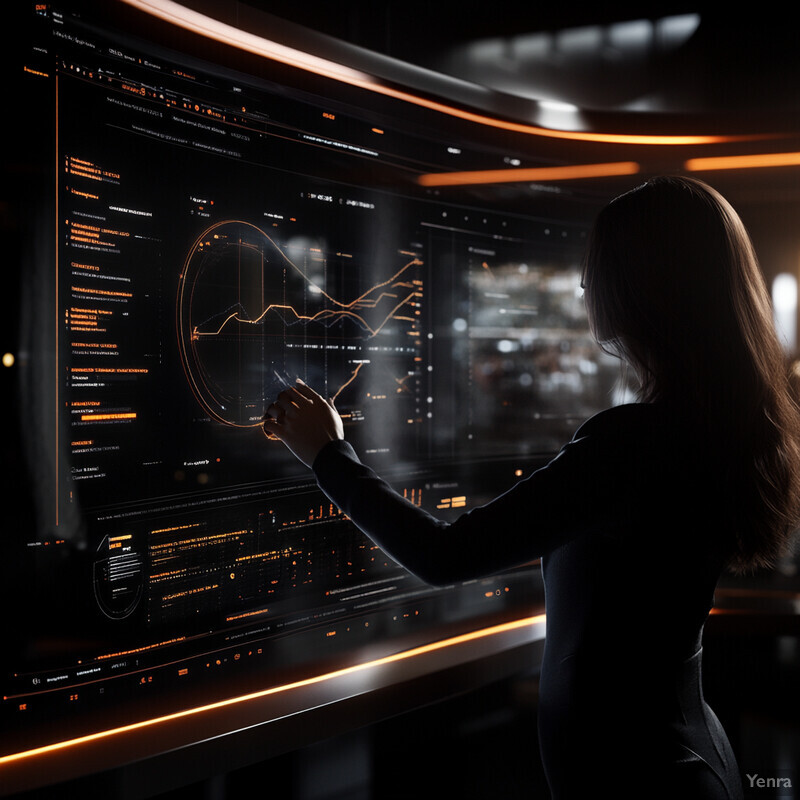
By analyzing usage patterns, engagement metrics, and overall asset performance, AI-powered analytics can produce insightful reports and visualizations. These dashboards guide content strategists, marketers, and product managers in making data-driven decisions about which assets to promote, update, or retire. With AI surfacing hidden trends and opportunities, organizations gain clearer visibility into their DAM’s effectiveness, driving continuous improvement and maximizing the return on their digital content investments.