1. Automated Population Estimation from Satellite Imagery
AI-driven computer vision techniques can extract population densities by analyzing high-resolution satellite images of buildings, vehicle counts, and nighttime lights. This allows urban planners to estimate population distributions at a much finer spatial scale than traditional surveys.
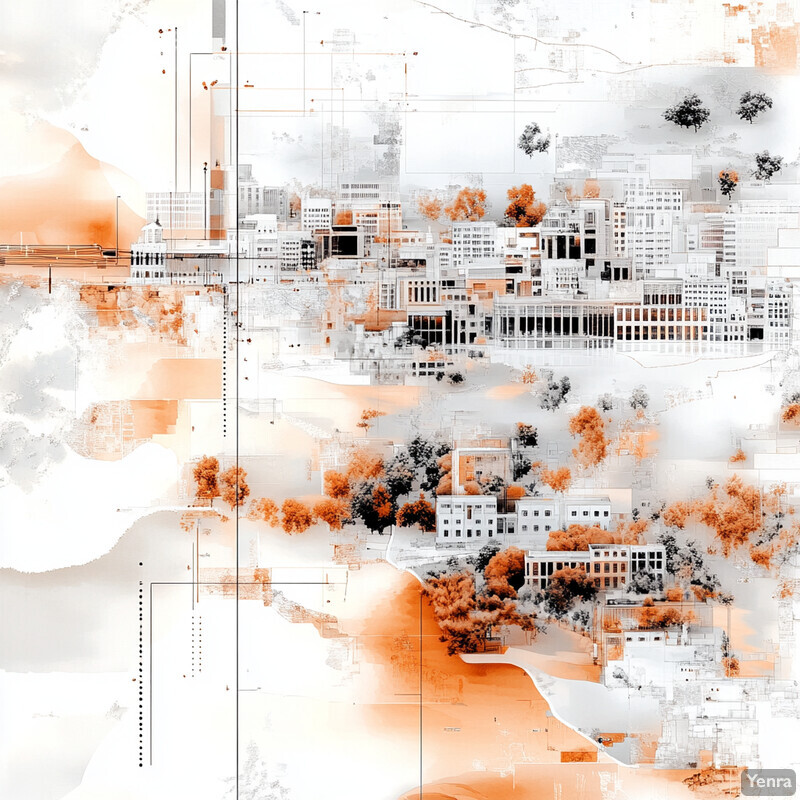
AI-driven computer vision techniques can analyze high-resolution satellite and aerial imagery to estimate population densities with extraordinary precision. Traditional methods rely heavily on census data collected every several years, often at coarse geographic scales. In contrast, AI models can identify and count buildings, approximate household sizes, detect patterns of illumination at night, and even assess vehicular activity. These insights enable planners to estimate population distribution down to the neighborhood—or even block—level. The result is a dynamic, near-real-time map of where people live, which is especially valuable in rapidly changing urban areas or regions where official data collection is limited.
2. Dynamic Forecasting of Demographic Shifts
Predictive models using machine learning can integrate historical census data, migration patterns, birth/death rates, and economic indicators to forecast demographic changes. These forecasts inform long-term urban infrastructure planning, ensuring that future housing, schools, and healthcare facilities match population needs.
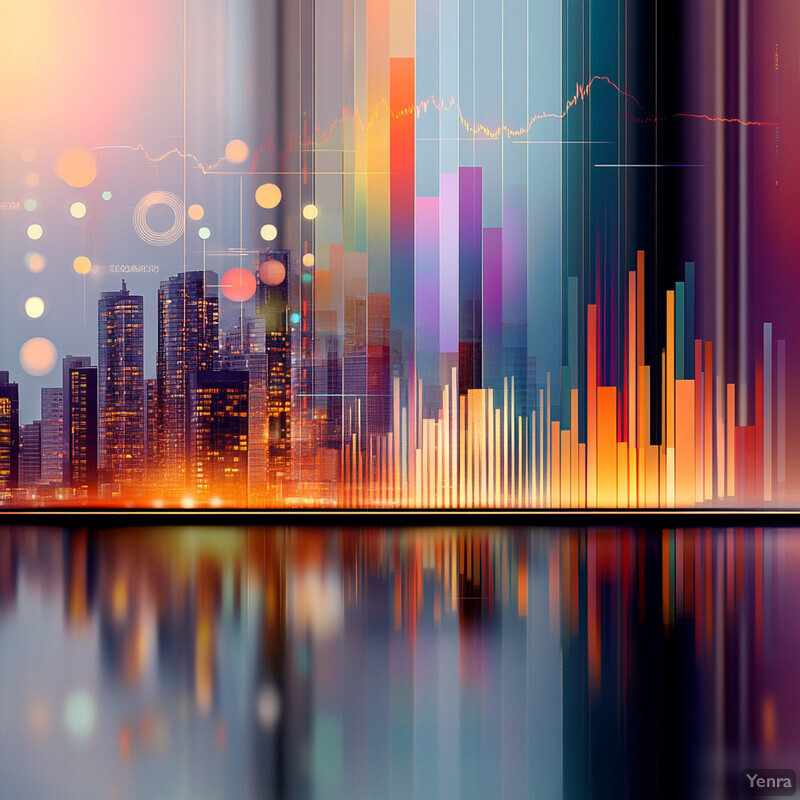
Urban populations do not remain static; they evolve due to birth rates, mortality trends, migration flows, and economic opportunities. AI-based predictive models aggregate historical census figures, labor market indicators, housing trends, and health data to model how populations might change over time. Planners can use these predictions to anticipate demand for schools, health facilities, housing projects, and transportation infrastructure well into the future. This foresight enables proactive strategies rather than reactive responses, facilitating sustainable urban development that matches anticipated demographic profiles.
3. Granular Socioeconomic Profiling
By correlating data from social media, local business registries, and public utility usage, AI can approximate income levels, educational attainment, and employment status for specific neighborhoods. This granular understanding helps direct economic development efforts to areas most in need.
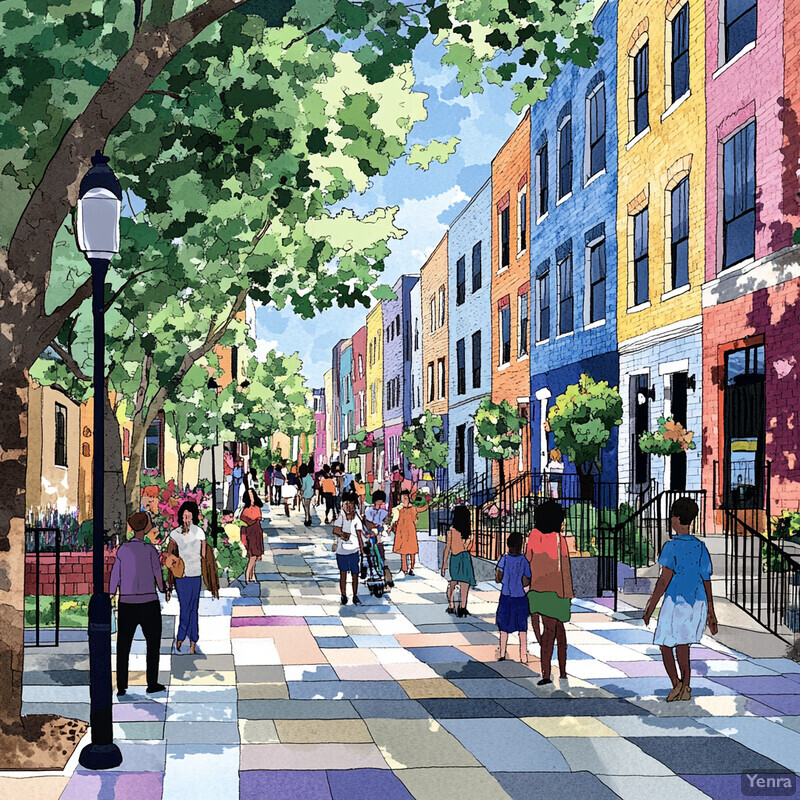
Traditionally, socioeconomic data about urban populations is gathered through periodic surveys and census counts, which can quickly become outdated or lack detail at the micro-level. AI augments these methods by integrating data streams from diverse sources—such as social media activity, business registrations, employment listings, utility usage patterns, and property transaction records. These advanced models provide a much finer-grained understanding of neighborhood-level income distribution, educational attainment, employment stability, and consumer behavior. As a result, planners gain a nuanced socioeconomic landscape that helps them prioritize interventions, subsidies, and community programs for the groups that need them most.
4. Real-Time Analysis of Human Mobility
With AI processing large streams of anonymized GPS and smartphone data, planners can understand where and when people move within a city. Such insights help optimize public transportation routes, street design, and pedestrian infrastructures to accommodate shifting travel patterns.
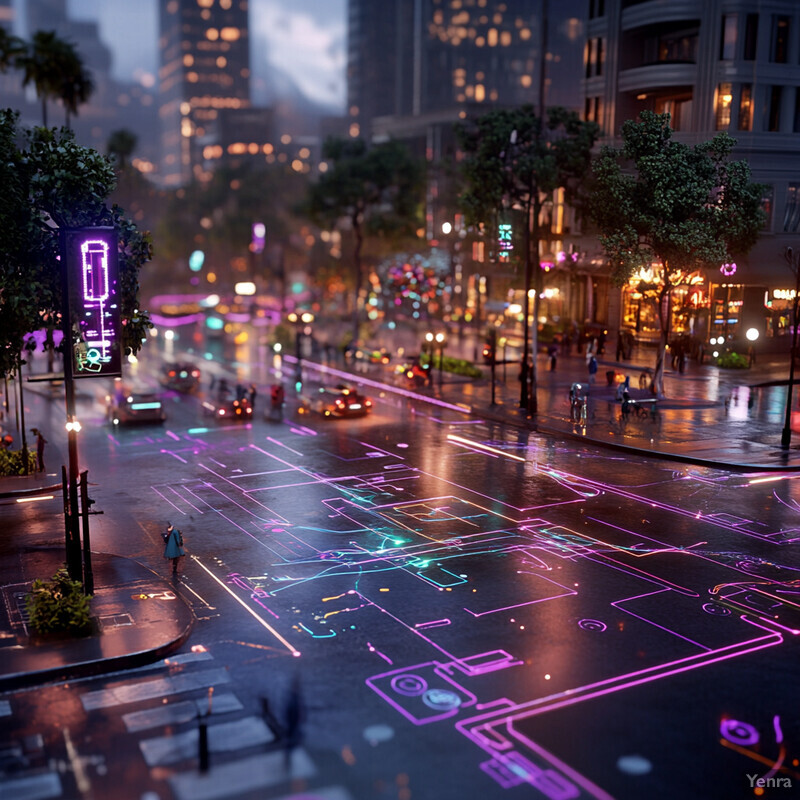
Cities are in constant flux as people commute for work, leisure, education, and errands. AI systems can digest vast amounts of anonymized mobile phone GPS data, ride-sharing trip logs, and public transit usage records to track how people move through urban spaces. By modeling these mobility patterns, planners can identify areas with congestion, pedestrian hotspots, underserved transit corridors, and shifting consumer hubs. Over time, this knowledge guides the optimization of transportation networks, the placement of bike lanes, the scheduling of bus and metro services, and even the distribution of commercial districts to align with the actual travel behaviors of the population.
5. Identification of Vulnerable Populations
Machine learning models can detect spatial clusters of elderly residents, low-income households, or individuals with limited English proficiency. By identifying these vulnerable groups, policymakers can design targeted interventions—such as accessible community centers or multilingual public services.
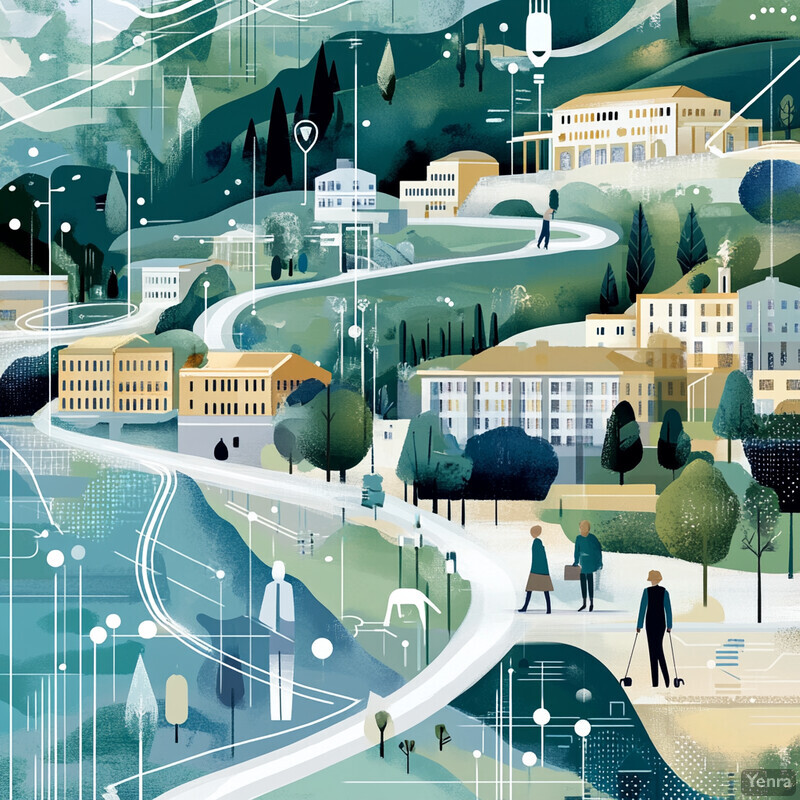
Understanding the distribution of at-risk populations—such as the elderly, low-income groups, people with disabilities, or non-native language speakers—is crucial for equitable urban planning. AI can sift through various datasets including healthcare access records, property values, demographics surveys, language usage patterns, and educational achievement indicators to pinpoint neighborhoods with higher vulnerability indices. By identifying these clusters, planners can develop targeted policies like accessible public facilities, free language classes, home health visits, affordable housing measures, and job training programs. This ensures that interventions are not uniformly distributed but strategically aimed where they have the greatest potential impact.
6. Refined Household Composition Estimates
Instead of relying solely on census data, AI models can integrate rental records, property tax data, and school enrollment figures to infer household sizes and compositions. This refined household-level understanding informs decisions regarding family services, local zoning, and community policing resources.
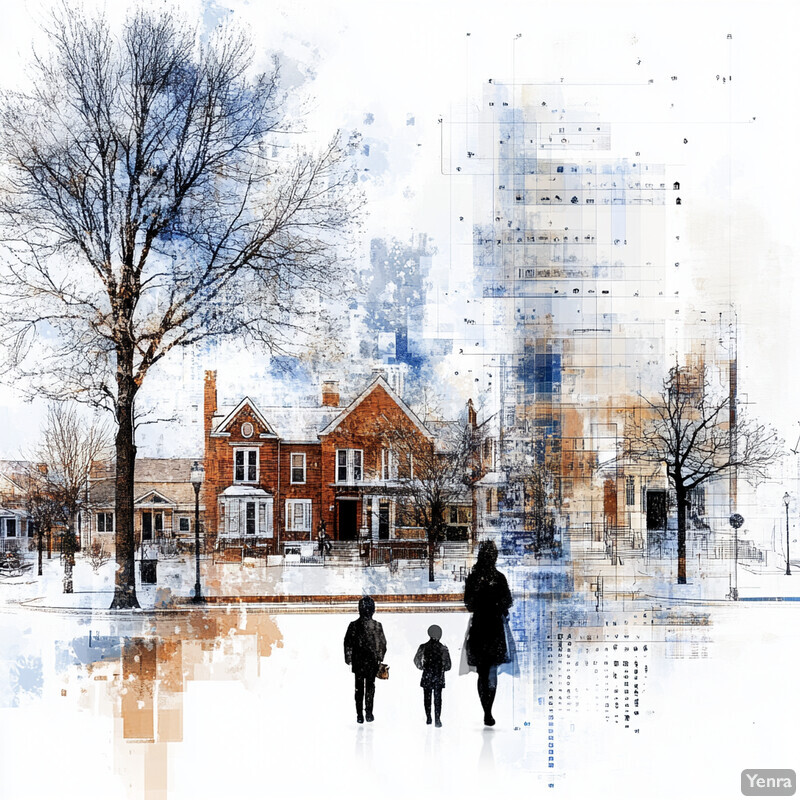
While censuses provide baseline data on household sizes and types, they may not fully capture rapid demographic changes, particularly in urban areas with fluid rental markets and diverse family arrangements. AI techniques can integrate property rental databases, property tax records, school enrollment data, and utility consumption patterns to infer more precise household characteristics. This refined picture helps urban planners design services that better fit the needs of various household types. For example, neighborhoods with many young families might require more daycare centers and playgrounds, while those with larger extended families might need housing units with more bedrooms and communal spaces.
7. Predictive Modeling of Gentrification and Displacement
Leveraging housing price trends, business turnover, and resident relocation data, AI can forecast where gentrification might occur. Planners can use these predictions to create policies that mitigate displacement, preserve affordable housing, and maintain community cohesion.
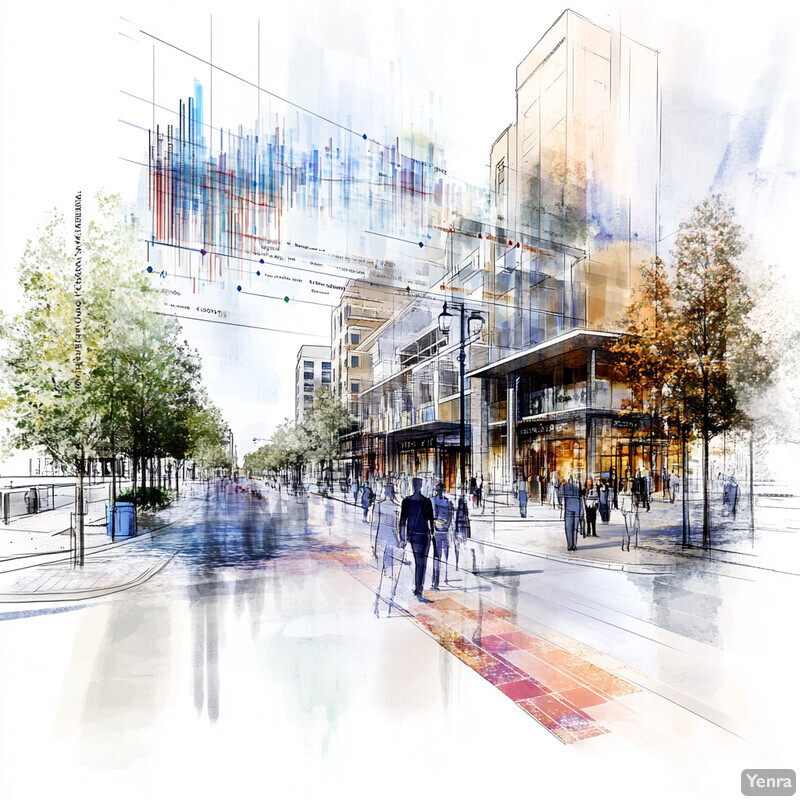
Gentrification often unfolds incrementally, leaving planners reacting to changes rather than anticipating them. AI can identify early signals—such as rising rental prices, increasing property sales, shifts in commercial activities, and changes in resident demographics—to forecast areas likely to experience gentrification. Armed with this knowledge, cities can implement policies that preserve affordable housing stock, support long-standing local businesses, and introduce safeguards against involuntary displacement. This approach moves beyond after-the-fact mitigation, empowering planners and policymakers to guide urban transformations in ways that retain social and cultural diversity.
8. Data Integration from Multiple Sources
AI pipelines can merge satellite imagery, census surveys, administrative records, and IoT sensor data—previously siloed sets—into unified demographic datasets. This holistic view reduces blind spots and improves the accuracy of population models and planning decisions.
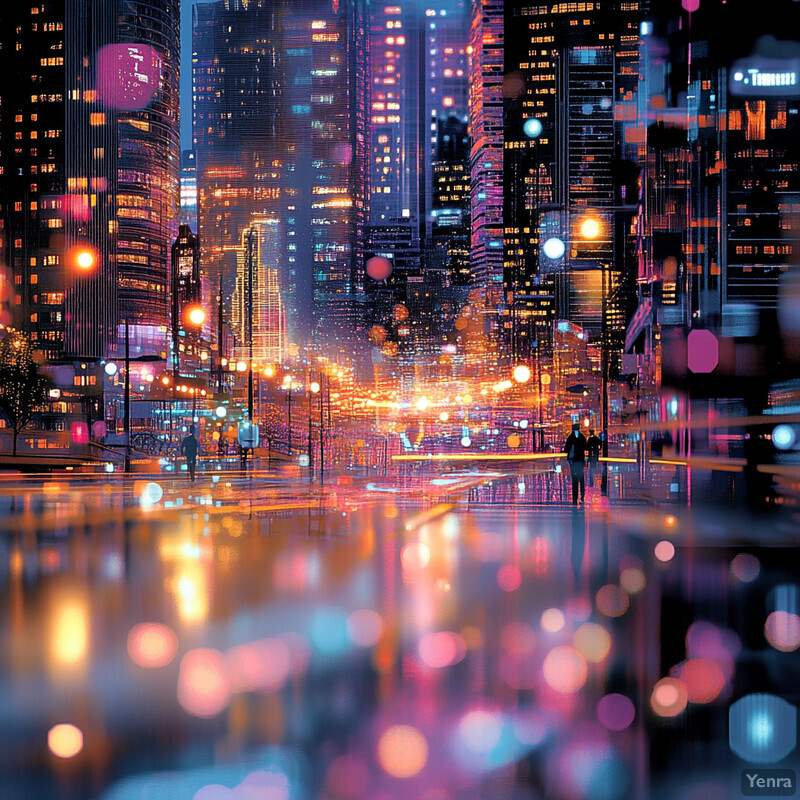
Information about urban populations often resides in separate silos—census bureaus, administrative records, satellite imagery, utility companies, and transportation authorities. AI-driven data integration pipelines bring these disparate sources together into a unified framework. By aligning and cross-referencing different datasets, AI helps planners overcome blind spots and achieve a more holistic understanding of demographic conditions. This integrated approach leads to improved accuracy in population models and enables more cohesive urban strategies that take into account all facets of city life, from energy consumption patterns to public health outcomes.
9. Spatial Clustering for Community Identification
Unsupervised machine learning methods can cluster neighborhoods based on demographic, socioeconomic, and infrastructural attributes. Identifying naturally forming “community clusters” helps planners understand local dynamics and tailor services to each area’s unique needs.
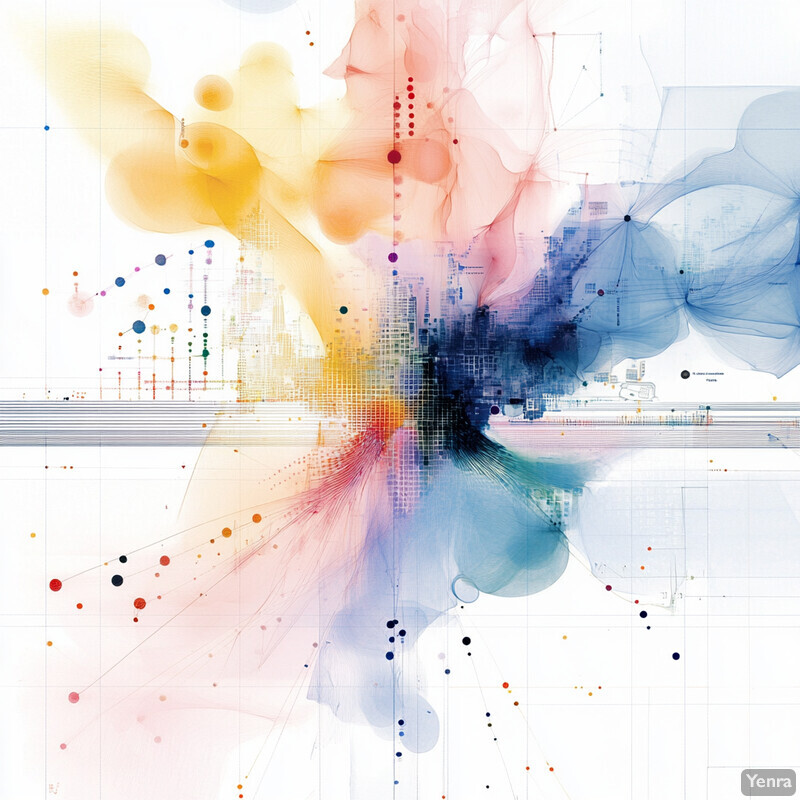
Not all communities align neatly with administrative boundaries. Unsupervised machine learning techniques can detect natural groupings or “clusters” within a city based on multiple indicators—such as age distribution, educational attainment, ethnic composition, land use, and access to amenities. This reveals the organic formation of neighborhoods and communities as they truly exist. Recognizing these functional clusters guides planners in tailoring interventions, preserving cultural districts, allocating resources more precisely, and fostering stronger community identities. It helps ensure that policies resonate with the actual social and cultural fabric of the city, rather than arbitrary political jurisdictions.
10. Enhanced Equity and Inclusion Assessments
AI can systematically evaluate how resources—like parks, hospitals, public transit stops—are distributed across demographic groups. This enables planners to detect inequities and guide policy decisions toward more equitable resource allocation.
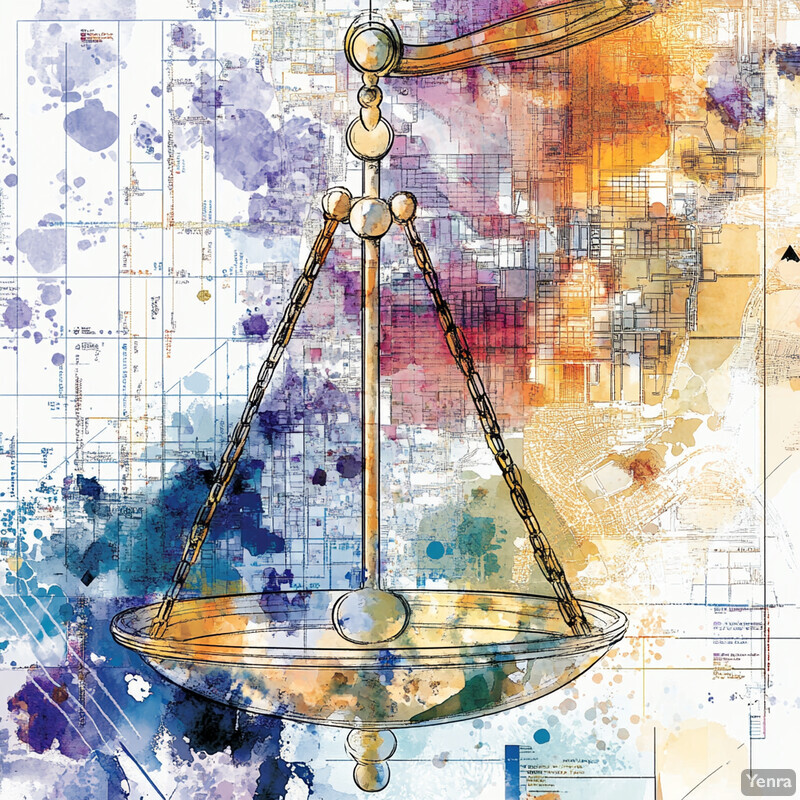
An equitable city ensures that all residents, regardless of their background, have comparable access to essential services and resources. AI can systematically evaluate how infrastructure, public amenities, healthcare facilities, schools, parks, and transit options are distributed relative to demographic groups. By overlaying resource maps with socioeconomic and demographic data, planners quickly identify which communities are underserved. This enables more informed policy decisions, such as positioning new clinics in low-access areas or adding green spaces in neighborhoods lacking recreational facilities, thereby improving quality of life for historically marginalized communities.
11. Temporal Analysis for Seasonally Varying Populations
Cities with seasonal workers, students, or tourism influxes can leverage AI to track and predict these periodic demographic fluctuations. In doing so, they can scale services or adjust transportation routes during peak seasons.
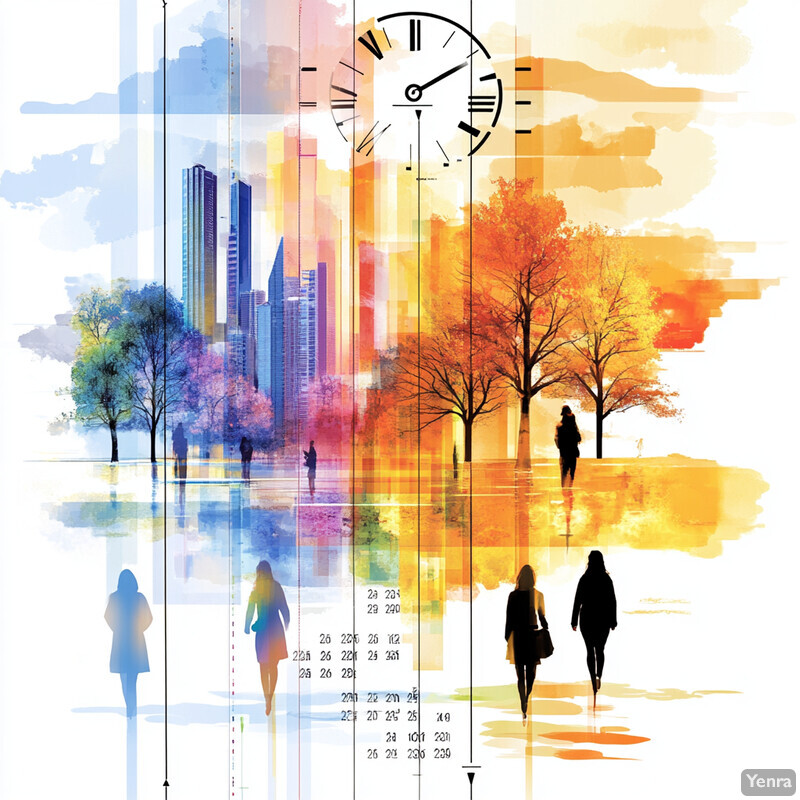
Some urban centers experience significant seasonal fluctuations in their population—tourists, temporary workers, or students. AI models can integrate hotel occupancy data, university enrollment cycles, and event calendars with transportation and retail indicators to map these periodic changes. Recognizing when and where populations swell or recede helps city planners adjust public services accordingly. They might increase public transit frequency during peak tourism season, allocate more security staff during large events, or plan temporary housing solutions for seasonal workers. This temporal lens ensures that urban infrastructure remains flexible and responsive year-round.
12. AI-Driven Scenario Testing
By feeding various “what-if” conditions into AI-based demographic models, planners can evaluate potential outcomes before implementing policies. Such scenario testing reduces risk and improves decision-making robustness.
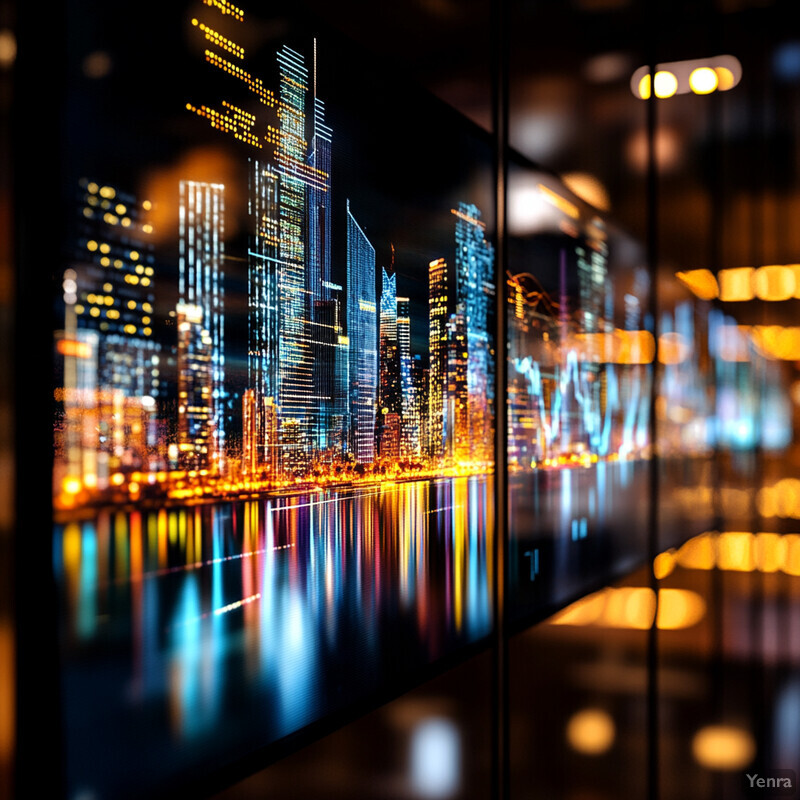
Urban planning involves making decisions under uncertainty. AI models can simulate different demographic scenarios by tweaking certain variables—such as increasing immigration, changing fertility rates, or altering economic conditions—and projecting their effects on population distribution and resource demands. This scenario testing allows planners to explore multiple futures and develop contingency plans. Such foresight informs more resilient urban strategies, minimizing the risk of costly missteps. For example, if models predict a surge in elderly residents, the city can proactively expand healthcare infrastructure or accessible public transportation before the change fully materializes.
13. Improved Accuracy in Informal Settlement Surveys
In areas where traditional census methods fall short, AI can interpret aerial and street-level imagery to estimate populations living in informal settlements. Identifying these overlooked communities ensures they receive the infrastructure and services they need.
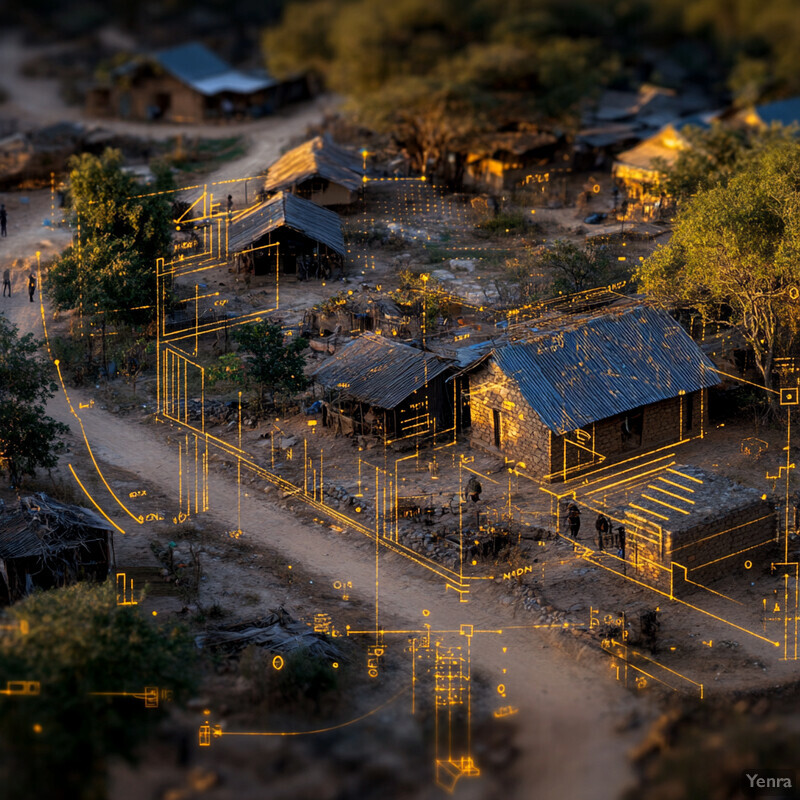
Many growing cities host informal settlements that are poorly captured by conventional surveys or censuses. AI can interpret high-resolution aerial images, crowd-sourced data, and other unconventional indicators to approximate the number of residents and their living conditions in these areas. By recognizing the unique patterns of building density, makeshift structures, and environmental cues, AI generates more reliable estimates than traditional mapping methods. These insights guide interventions such as upgrading local infrastructure, providing sanitation services, or offering legal pathways to secure land tenure, ensuring these populations are not overlooked in the city’s development plans.
14. Detecting and Correcting Census Undercounts
AI can highlight discrepancies between reported census numbers and signals from utilities, school enrollments, or mobile phone usage. Identifying underrepresented neighborhoods helps correct population estimates, influencing the fair distribution of public funds and political representation.
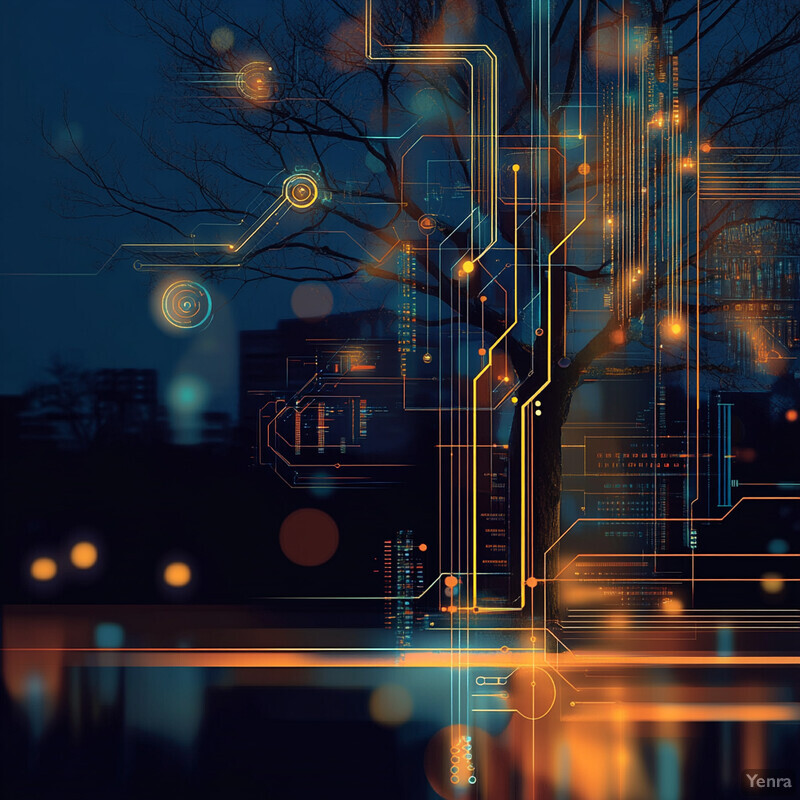
Censuses often miss certain groups or undercount populations in rapidly changing neighborhoods. AI can help cross-check official records against other data sources—like school enrollments, utility consumption, mobile phone usage, or healthcare registrations—to flag discrepancies. When patterns suggest that a particular district might be underrepresented, planners can investigate and adjust their estimates accordingly. More accurate population counts influence the fair allocation of public funds, guide political representation, and ensure that all communities receive appropriate services and infrastructure investments.
15. Rapid Crisis Response Planning
In the event of disasters or pandemics, AI models can swiftly integrate newly emerging data to estimate where the hardest-hit populations are. Timely insights enable efficient emergency resource deployment and recovery planning.
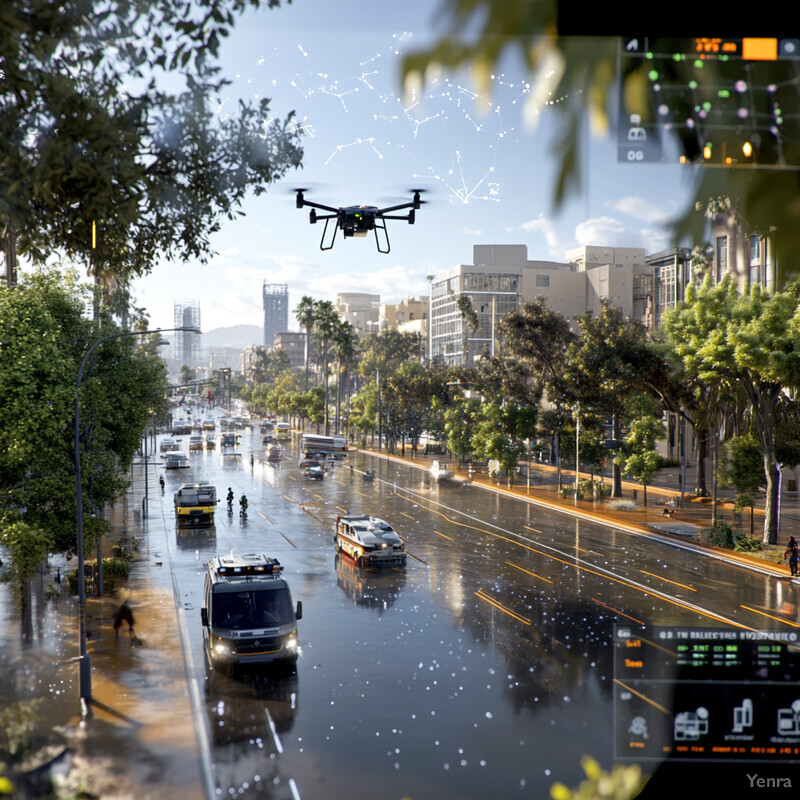
In times of crisis—be it a natural disaster, public health emergency, or sudden economic shock—understanding the spatial distribution and specific vulnerabilities of the population is essential for swift action. AI can rapidly integrate new data as it emerges, such as hospital admission rates, emergency service calls, road blockages, or social media pleas for help. This real-time demographic intelligence enables decision-makers to identify neighborhoods in urgent need, allocate rescue teams efficiently, open temporary shelters in the right locations, and deliver medical supplies where they are most required. The result is a more agile, data-driven response that can save lives and reduce suffering.
16. Cultural and Language Community Mapping
By analyzing linguistic patterns in social media and public documents, AI can map cultural or language-based communities. Recognizing these groups supports better outreach efforts, linguistically appropriate services, and the preservation of cultural amenities.
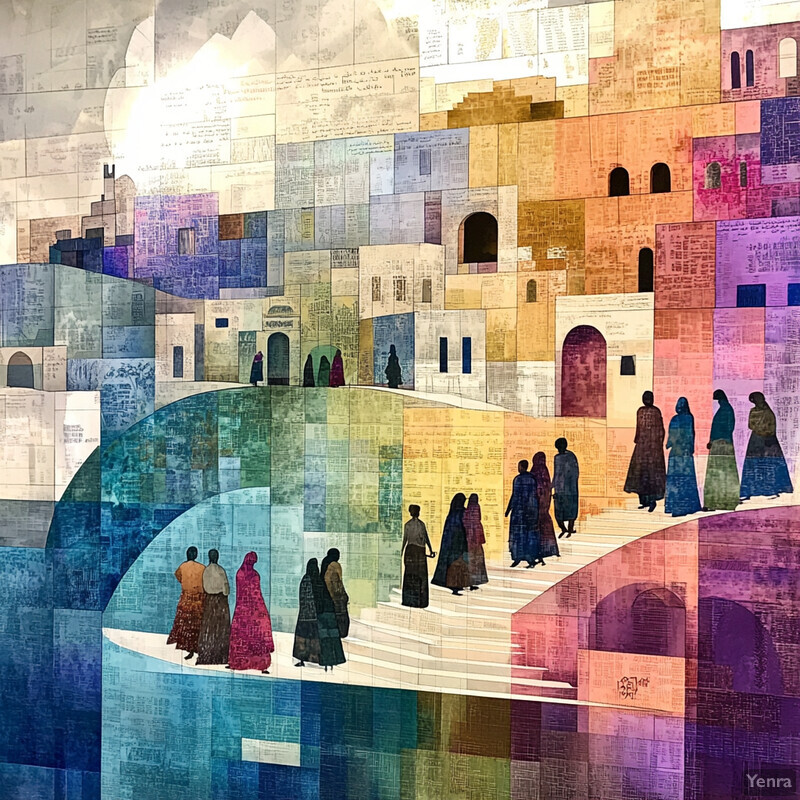
Cities are cultural mosaics where linguistic and cultural communities often reside side by side. AI can analyze language usage in social media posts, community newsletters, or public documents, identifying clusters of specific linguistic or cultural groups. This insight helps planners provide culturally and linguistically appropriate public services, educational materials, and community centers. Ensuring that signage, customer service lines, and public health messages are available in multiple languages, for instance, can greatly improve social cohesion and inclusion, strengthening the trust between city authorities and diverse populations.
17. Enhanced Transportation Demand Forecasting
AI-driven demographic analysis helps model the relationship between population characteristics and transportation usage. Planners can use these insights to anticipate future transit demands and optimize public transit routes.
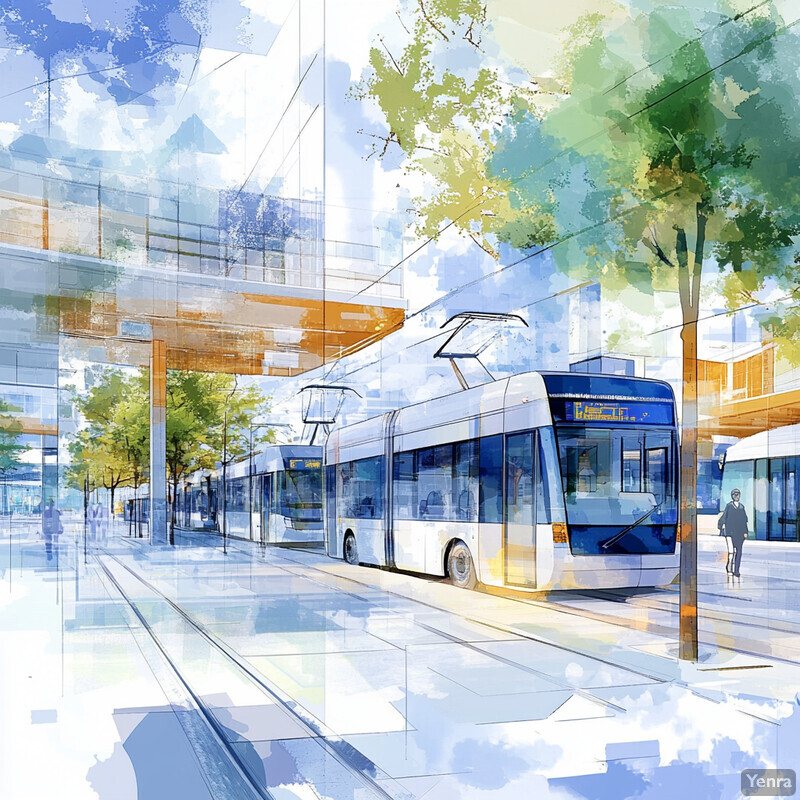
Reliable transportation planning demands understanding who needs to travel, where, when, and why. AI models integrate demographic profiles with factors like land use, employment centers, economic activity, and existing transit patterns to forecast transportation demand. By anticipating the future volume and direction of traffic, planners can design new transit routes, synchronize traffic lights, add bike-sharing stations, or build pedestrian overpasses where they will be most effective. Such data-driven planning helps reduce congestion, improve accessibility, and ensure that mobility investments are both cost-effective and aligned with community needs.
18. Informed Zoning and Land Use Policies
Population data refined by AI can guide zoning decisions to ensure that residential, commercial, and industrial allocations align with current and projected demographic distributions.
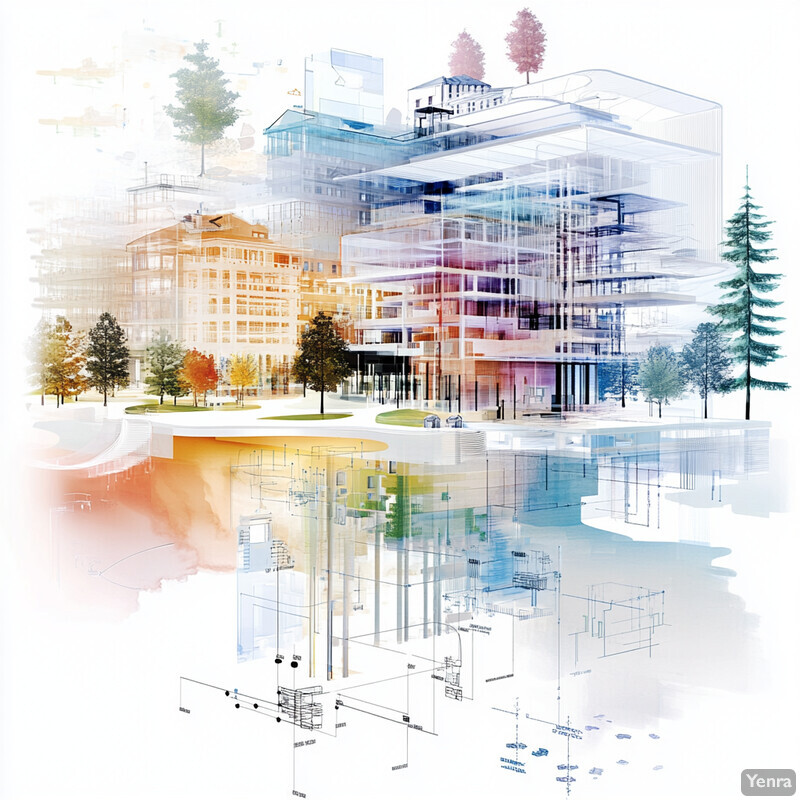
Zoning decisions shape the physical form of a city and its socioeconomic trajectory. AI-driven demographic analysis provides detailed insights into current and future population structures, land use patterns, and housing demands. Armed with this knowledge, urban planners and policymakers can adjust zoning regulations to better accommodate expected growth, prevent overcrowding, promote mixed-use development, or preserve green spaces where needed. These informed choices help prevent tensions between residential and industrial activities and ensure that the city’s physical layout meets the evolving demands of its inhabitants.
19. Sustainable Resource Allocation
Understanding the nuances of demographic patterns helps match infrastructure investments—water treatment plants, waste management facilities, energy grids—to the actual needs of particular neighborhoods.
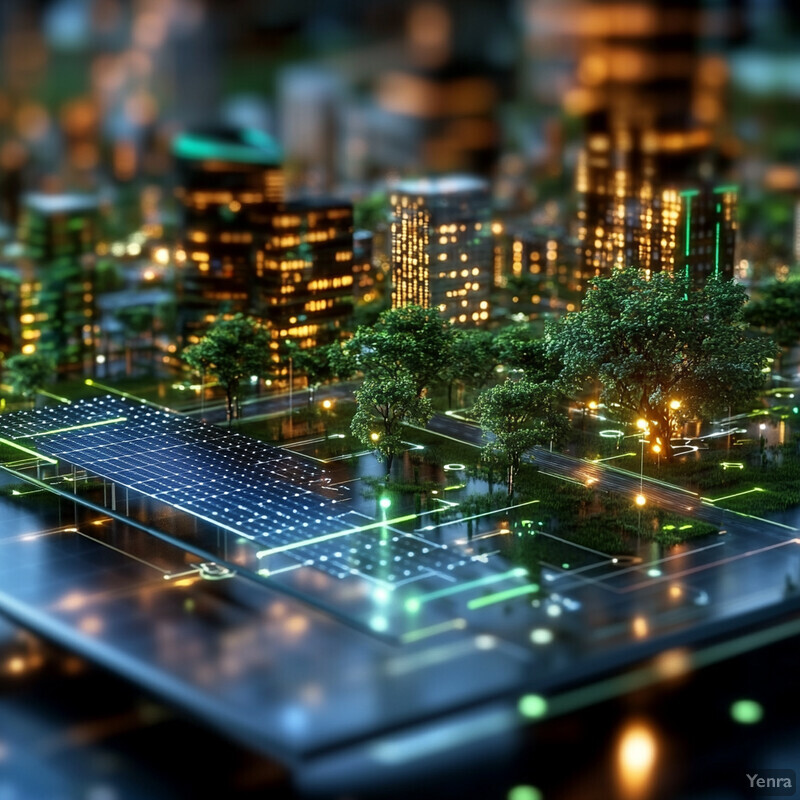
Providing adequate infrastructure and services—water, energy, waste management, healthcare, and education—depends on knowing where people live, work, and play. AI enables fine-grained demographic analysis that helps align resource distribution with actual needs. For instance, water treatment plant expansions can be planned in areas where population growth is forecasted to rise, while energy grids can be strengthened in regions anticipating industrial development. By optimizing where and when to invest in infrastructure, cities become more efficient, reduce environmental footprints, and enhance the overall quality of urban life.
20. Continuous Monitoring and Updating of Urban Plans
AI-powered tools can constantly process new data, providing urban planners with up-to-date demographic insights. This continuous monitoring enables them to adapt policies in near real-time, ensuring that urban plans remain dynamic and relevant.
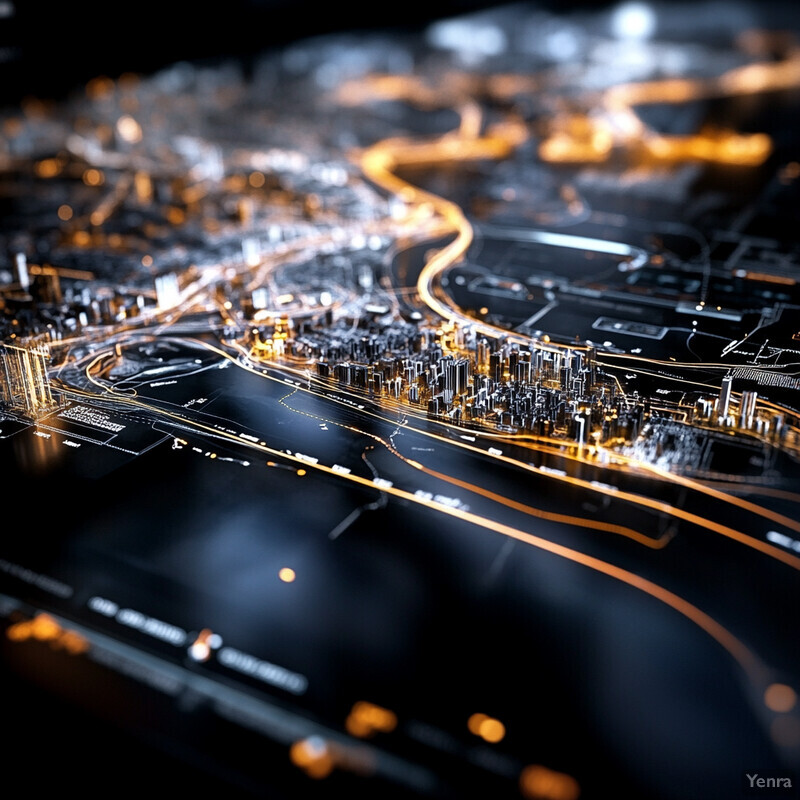
In the past, demographic analysis was often static and quickly outdated. AI tools allow for continuous processing of new data, ensuring that urban plans can be updated and refined in real time. As conditions shift—such as sudden migration inflows, emerging business hubs, or new cultural trends—planners can adjust their strategies promptly. Instead of waiting for the next census or multi-year study, decision-makers have near-instant access to demographic insights. This dynamic, iterative approach to planning fosters more resilient, adaptable, and responsive urban environments that keep pace with the evolving needs of the population.