1. Automated Data Integration
AI can automatically aggregate and unify large volumes of customer data from multiple channels—website analytics, CRM systems, social media, call centers—providing a more holistic view of the customer journey.
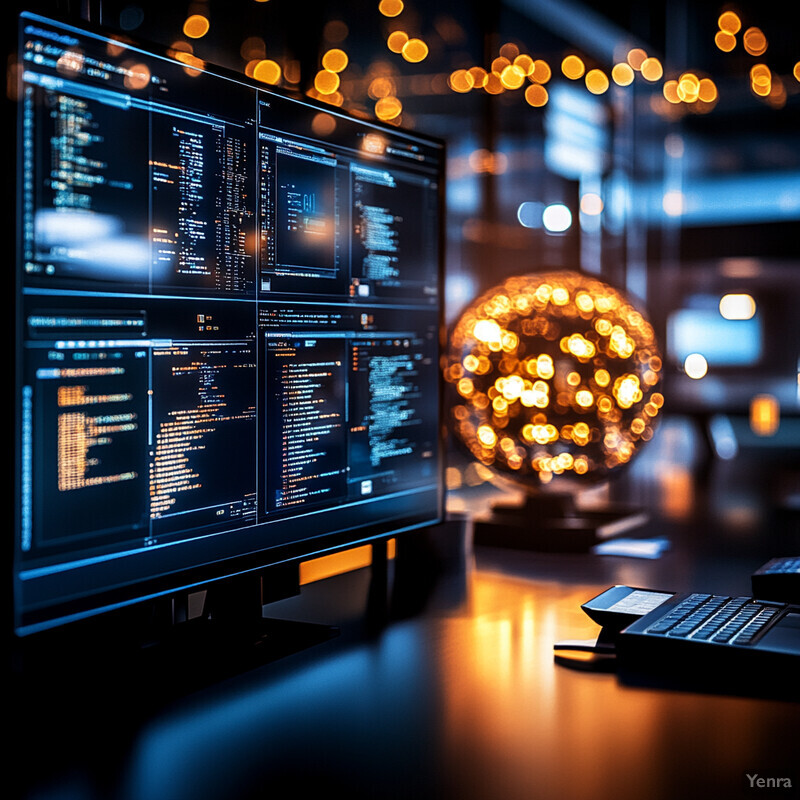
Traditional customer journey mapping often involves pulling data from multiple sources—website analytics, CRM platforms, social media sentiment, and more—then manually cleaning, organizing, and synthesizing this information. AI streamlines this process through automated data integration, using machine learning algorithms to reliably extract and reconcile disparate data points in real-time. By doing so, AI reduces the complexities and inefficiencies of manual data wrangling. As a result, organizations gain a unified and holistic view of their customers’ behaviors, preferences, and interactions across various channels. This consolidated perspective ensures that decision-makers can focus more on strategic insights rather than on administrative tasks, making the entire journey mapping process both more accurate and actionable.
2. Real-Time Behavioral Insights
Machine learning algorithms can detect shifts in customer behavior as they happen, enabling businesses to promptly adjust messaging, product recommendations, or service approaches.
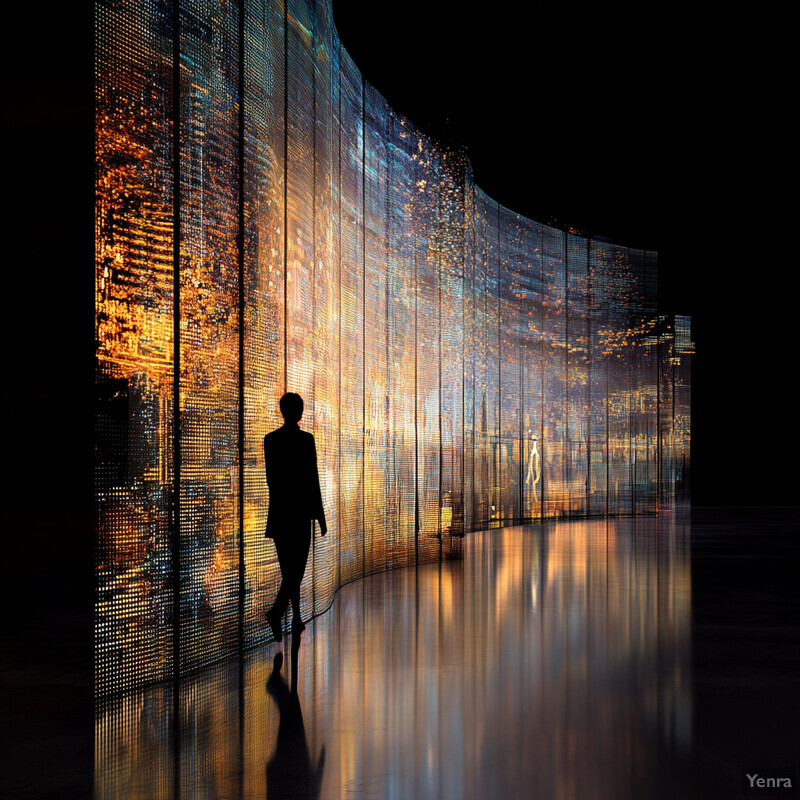
In the dynamic digital marketplace, customer preferences and behaviors shift quickly. AI-powered analytics tools continuously monitor these changes, capturing subtle variations in user clicks, browsing patterns, or purchase behaviors the instant they occur. These real-time insights allow businesses to adapt their messaging, product features, or support interventions on the fly. For example, if customers suddenly gravitate towards a new product feature, AI can highlight that trend immediately, prompting marketing teams to create relevant campaigns or customer support to refine their guidance. This high-resolution, moment-to-moment intelligence ensures that journey maps evolve fluidly in response to emerging patterns, keeping customer experiences relevant and engaging.
3. Customer Segmentation at Scale
AI-driven clustering methods can identify micro-segments or personas within the broader customer base, allowing for more precise journey mapping and tailored experiences.
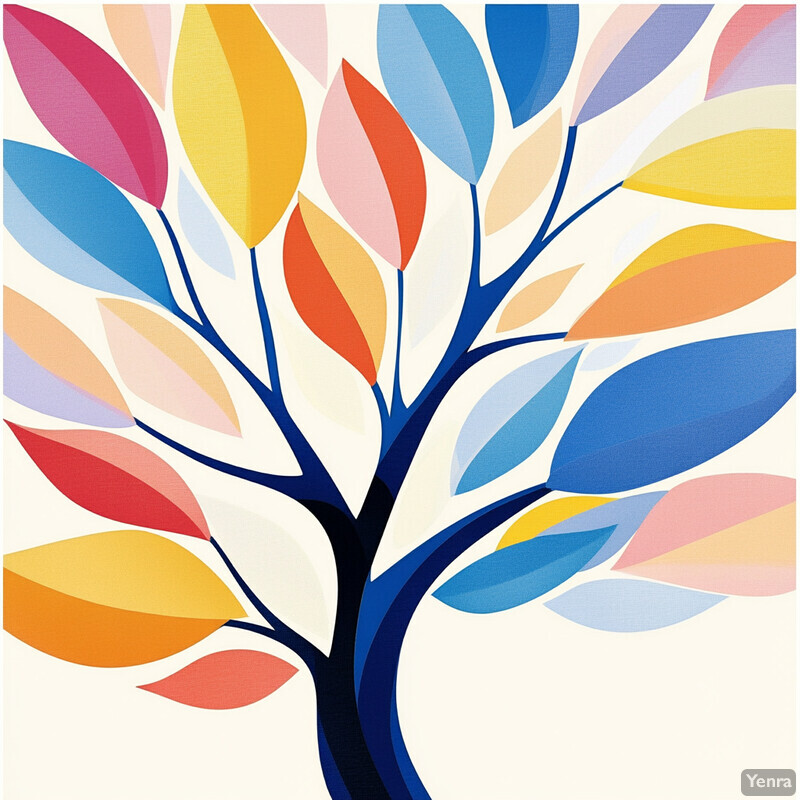
While traditional segmentation might break customers into broad groups based on demographics or general behaviors, AI enables far more nuanced and scalable segmentation. By leveraging clustering algorithms and unsupervised learning techniques, AI can sift through massive customer data sets and identify new micro-segments defined by shared interests, purchasing patterns, support needs, or even engagement frequencies. This deeper understanding helps businesses craft bespoke experiences and messaging strategies unique to each subset’s preferences. Ultimately, these precise micro-segments make it possible to deliver highly personalized journeys that resonate more strongly with individuals, improving conversion rates, retention, and overall satisfaction.
4. Predictive Journey Mapping
By analyzing historical data and ongoing customer interactions, AI can predict future touchpoints and customer paths, informing proactive strategies to improve the journey.
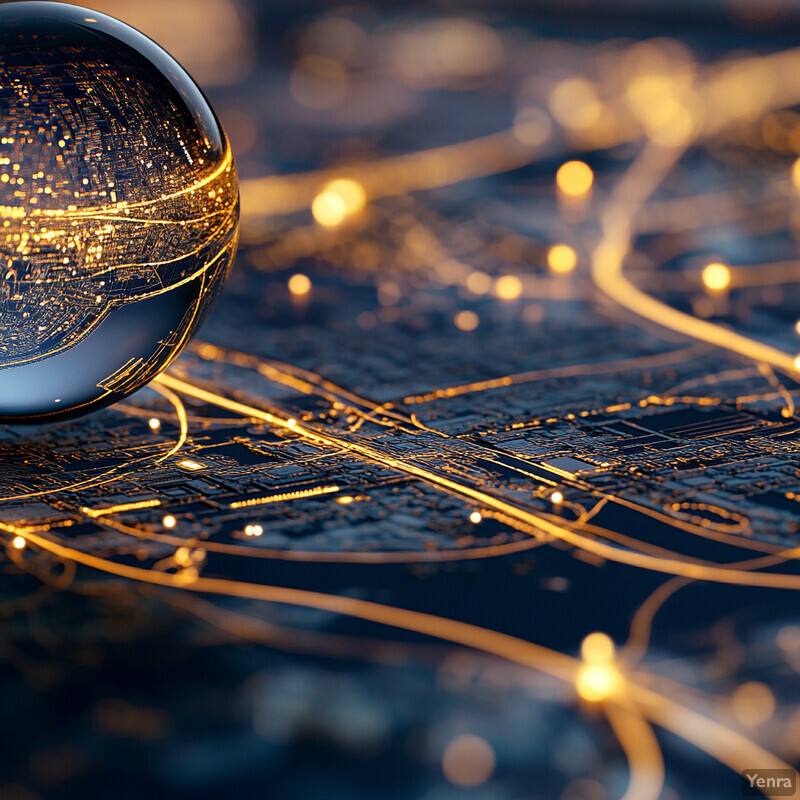
Beyond examining what customers have done, AI can anticipate what they are likely to do next. Predictive journey mapping uses machine learning models trained on historical user behavior and patterns to forecast future steps, interactions, and conversion events. This forward-looking capability helps teams proactively shape the journey. For instance, if historical data suggests a certain customer segment often abandons the process before a final purchase step, AI might predict this scenario and propose interventions—like tailored discounts or streamlined checkout options—before the customer reaches that point. By doing so, organizations can smooth friction points and guide customers more seamlessly from awareness to loyalty.
5. Sentiment Analysis
Natural language processing (NLP) can extract emotions and opinions from textual feedback (reviews, social media comments, support tickets) to identify frustration points and moments of delight within the journey.
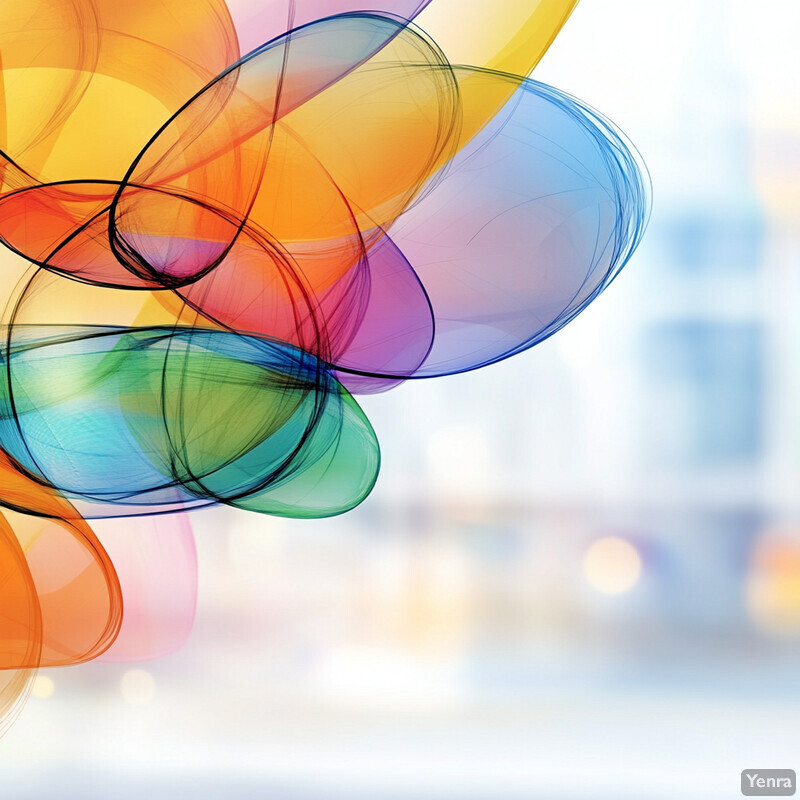
Understanding customer emotions is a crucial factor in effective journey mapping, and AI’s natural language processing (NLP) capabilities make this task scalable and precise. By parsing reviews, social media posts, survey responses, and support transcripts, AI-powered sentiment analysis tools can detect positivity, negativity, and neutrality, along with more nuanced emotional states such as frustration, anxiety, or delight. This insight reveals critical moments in the customer journey where emotions spike—be it confusion during onboarding or excitement upon receiving a product. Businesses can then refine these touchpoints, either by clarifying instructional content where confusion is high or amplifying aspects of the experience that evoke positive emotions.
6. Anomaly Detection
AI can spot unusual customer behavior, such as sudden drop-offs or unexpected surges in activity at certain touchpoints, helping companies quickly address problems or capitalize on opportunities.
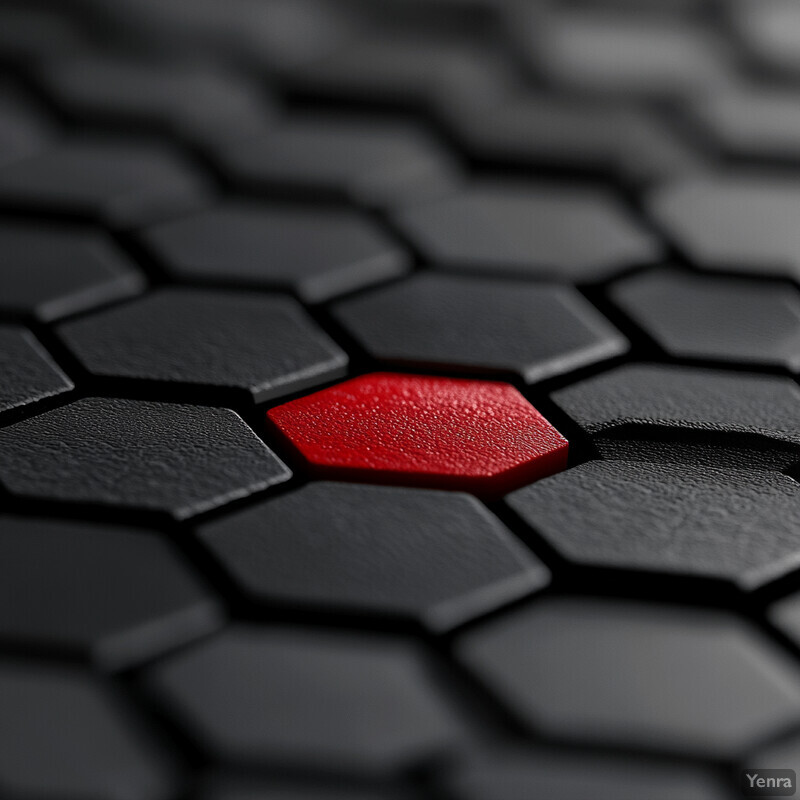
Customer journeys seldom unfold perfectly, and unexpected roadblocks or unusual user behaviors can derail progress. AI excels at identifying anomalies—sudden drops in engagement, surges in abandoned carts, spikes in customer support tickets, or any deviation from established behavioral norms. Through anomaly detection, organizations can quickly pinpoint where and why problems occur. Maybe a recent website update triggered a bug causing users to get stuck, or a new payment method introduced unexpected friction. By catching these anomalies early, businesses can rapidly deploy fixes or improvements, ensuring that customer journeys remain smooth and uninterrupted, ultimately preserving trust and satisfaction.
7. Personalized Recommendations
Recommender systems powered by AI can tailor product suggestions, content, and offers to individual customers, making each step of the journey more relevant and engaging.
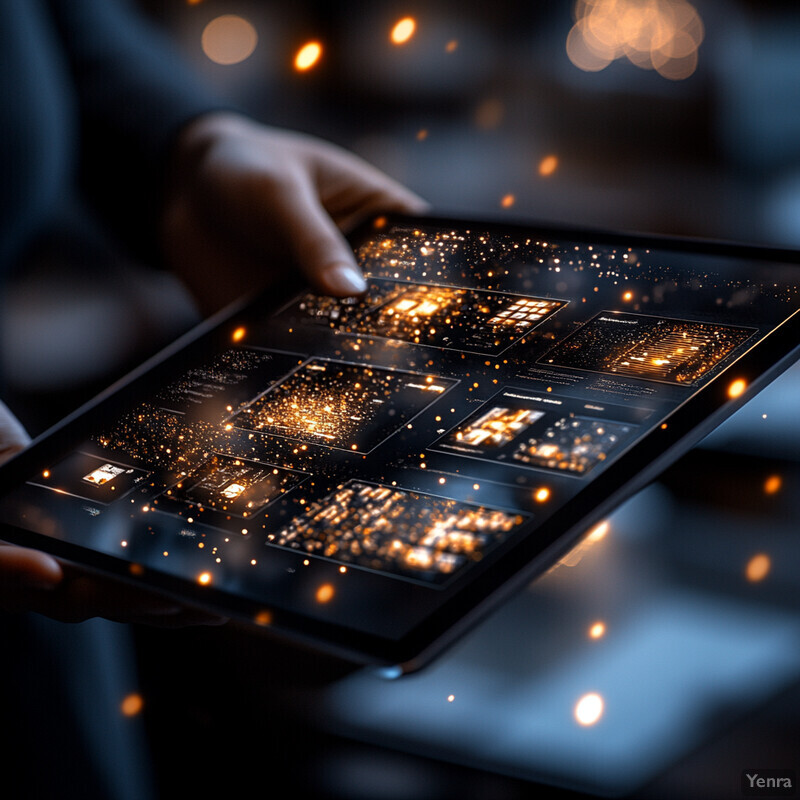
Personalization is a cornerstone of modern customer experiences. AI-driven recommender systems analyze historical purchase data, browsing behaviors, and even contextual information like location or device type to serve up precisely tailored suggestions. If a customer frequently buys from a certain product category, the AI may highlight relevant upsells, complementary items, or related articles to enrich their experience. These highly relevant recommendations deepen engagement, shorten decision-making processes, and foster a sense of being understood and valued. Over time, personalization not only encourages repeat purchases but also strengthens brand loyalty, making each step of the journey feel individually crafted.
8. Dynamic Customer Journeys
Instead of fixed, linear journeys, AI can dynamically adapt the sequence of touchpoints based on real-time context, preferences, and evolving customer needs.
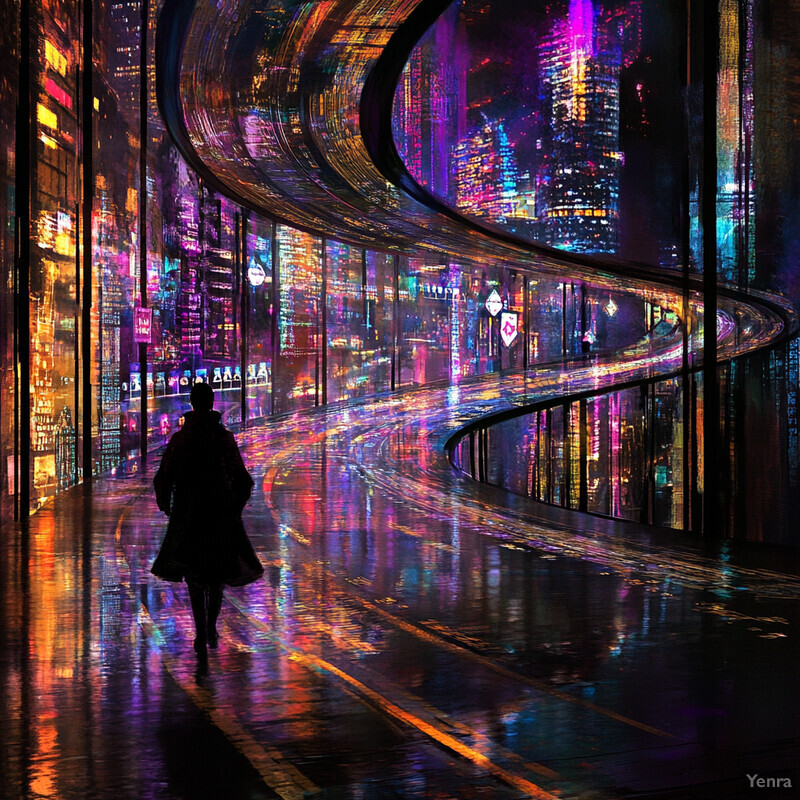
Traditional journey maps are often static outlines that assume most customers follow a similar path. AI challenges this assumption by dynamically adjusting journeys in real-time based on evolving conditions. For example, if a customer appears hesitant about completing a purchase, AI might introduce a live chat assistance option at the crucial moment. If another user seems rushed and often skips instructional content, the journey can be streamlined to save them time. This dynamic orchestration turns the linear journey into a fluid, adaptive experience where customers are guided along routes that best fit their current context, needs, and pace, significantly improving conversion and satisfaction rates.
9. Voice of Customer Analysis
AI can analyze open-ended survey responses, chat transcripts, and call recordings to identify recurring themes, concerns, and desires that inform improvements to the overall journey.
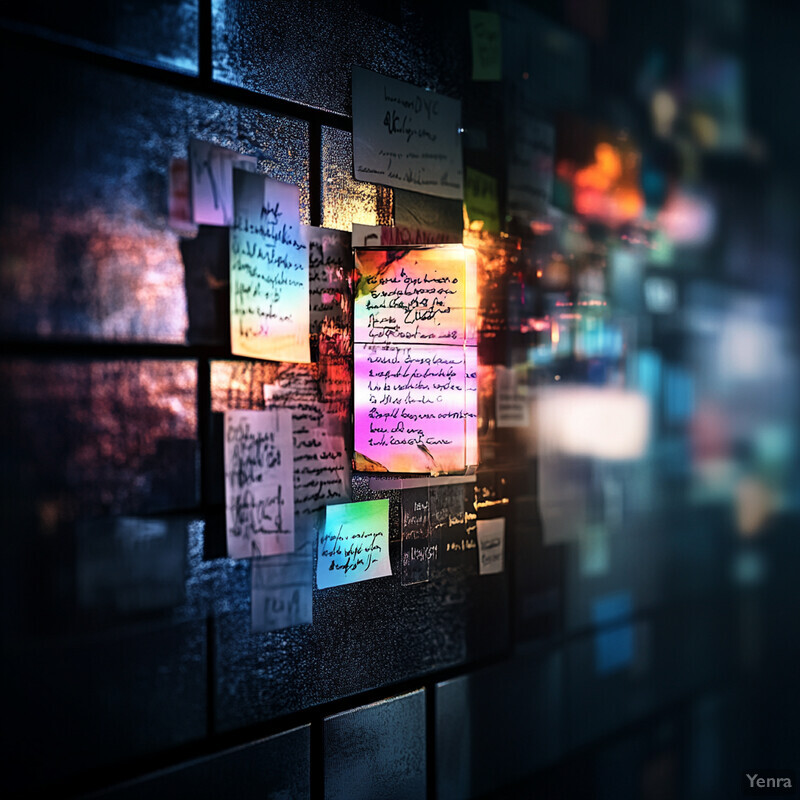
Feedback comes in many forms—from Net Promoter Score (NPS) surveys and focus groups to social media comments and live chat transcripts. With AI, businesses can efficiently aggregate, interpret, and surface themes within this unstructured data. For instance, NLP models can detect recurring complaints about complicated checkouts, or highlight praise for new product features. By identifying these patterns, organizations gain a richer understanding of what customers truly value or struggle with. Rather than relying on anecdotal evidence or cumbersome manual reviews, AI-driven Voice of Customer analysis ensures that improvements to the journey are based on comprehensive, data-driven insights.
10. Churn Prediction and Prevention
By using machine learning to predict which customers are likely to abandon a product or service, businesses can intervene earlier in the journey and implement targeted retention strategies.
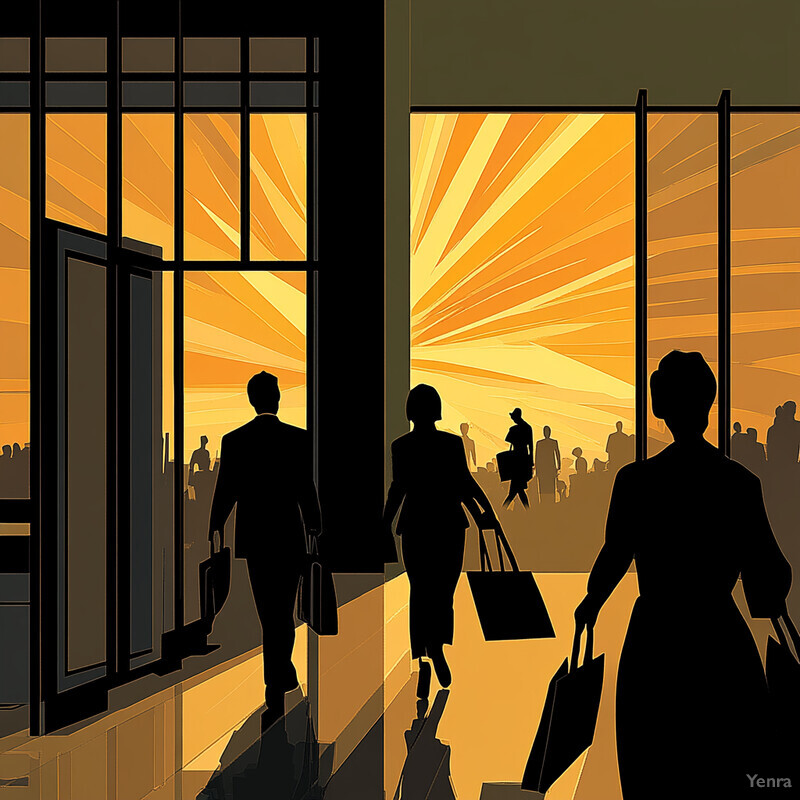
Identifying customers on the verge of defecting is crucial for many organizations. AI models can predict churn by recognizing behavioral patterns—like reduced engagement, a dip in purchase frequency, or negative sentiment in communications—that often precede abandonment. With these early signals, companies can intervene preemptively, offering special discounts, enhanced support, or loyalty rewards to re-engage these at-risk customers. By actively heading off churn before it happens, companies preserve long-term revenue and improve customer lifetime value. This proactive approach transforms the journey from a potential dead-end into a path for renewal and sustained connection.
11. Journey Time Optimization
AI can determine the ideal intervals between touchpoints, identifying when and where customers are most receptive, thus streamlining the pace and cadence of the journey.
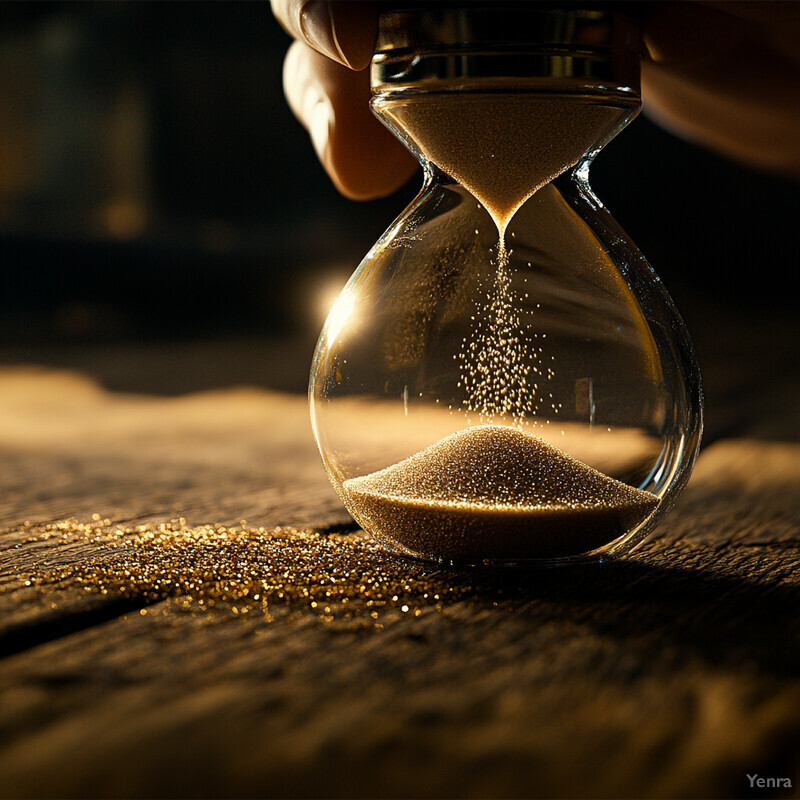
Timing is everything when it comes to customer engagement. AI can analyze when customers are most responsive and how long each journey stage should ideally last. If data shows users respond best to follow-up emails two days after a purchase, or that re-engagement campaigns are most successful after a week of product usage, AI will provide these insights. The result is carefully timed touchpoints that reach customers when their interest peaks or when they are most likely to need assistance. This refined cadence reduces customer fatigue, improves conversion rates, and ensures that every interaction is delivered at an opportune moment.
12. Enhanced A/B and Multivariate Testing
AI-optimized experimentation can dynamically allocate traffic to test variants that are performing well, reducing the time to identify the best journey improvements.
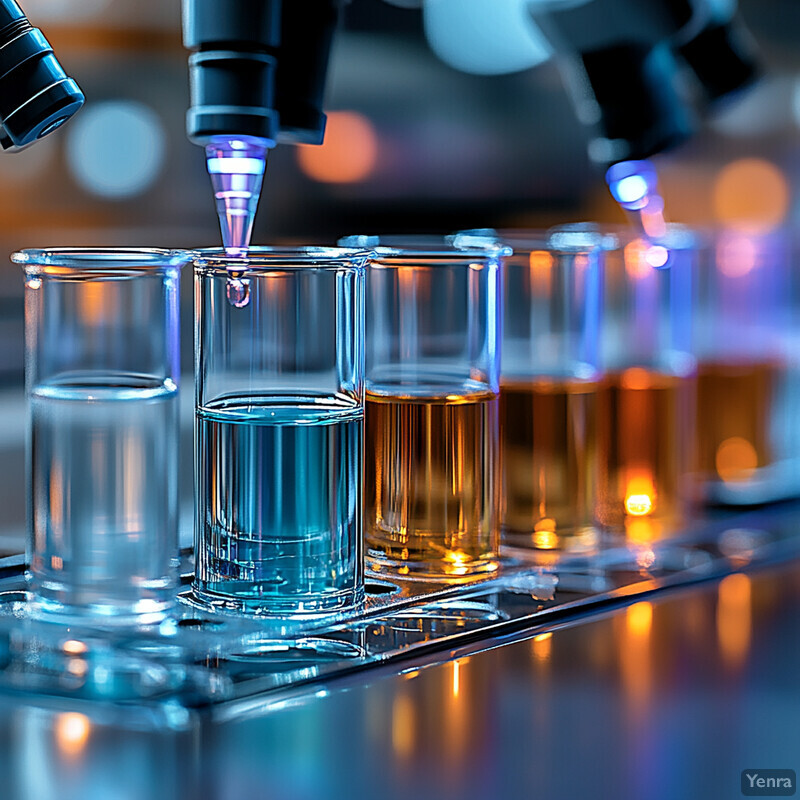
Experimentation is key to continual improvement, yet traditional A/B testing may take weeks or months to yield clear results. AI accelerates this process by automatically monitoring multiple test variants in real-time, dynamically adjusting traffic to emphasize better-performing options. This adaptive experimentation approach shortens the testing cycle and ensures that the winning variations are identified quickly and reliably. Over time, enhanced A/B and multivariate testing leads to more refined journeys, as businesses iterate and hone their approaches based on robust, data-driven evidence.
13. Proactive Issue Resolution
AI-powered chatbots and virtual assistants can anticipate common customer queries at different journey stages, offering immediate solutions and guiding customers forward.
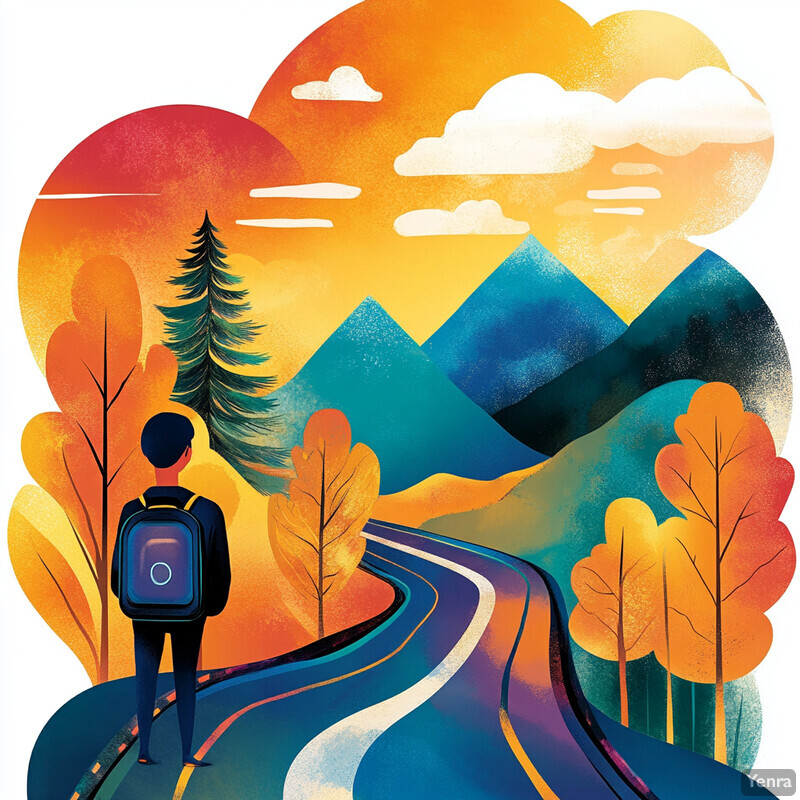
Customers often encounter predictable stumbling blocks at certain stages—such as difficulty understanding a product’s features or navigating a new interface. AI-powered chatbots and virtual assistants can preempt these common issues by offering guidance or clarifications at just the right time. For example, if a user hesitates during the onboarding process, the chatbot might proactively present a quick tutorial. This reduces frustration and support ticket volume, while simultaneously improving the customer’s confidence and satisfaction. As a result, proactive issue resolution turns challenging journey steps into opportunities for delightful, supportive interactions.
14. Channel-Orchestration
AI can determine the most effective communication channel for each touchpoint—email, SMS, push notification, or in-app message—based on individual customer preferences and behaviors.
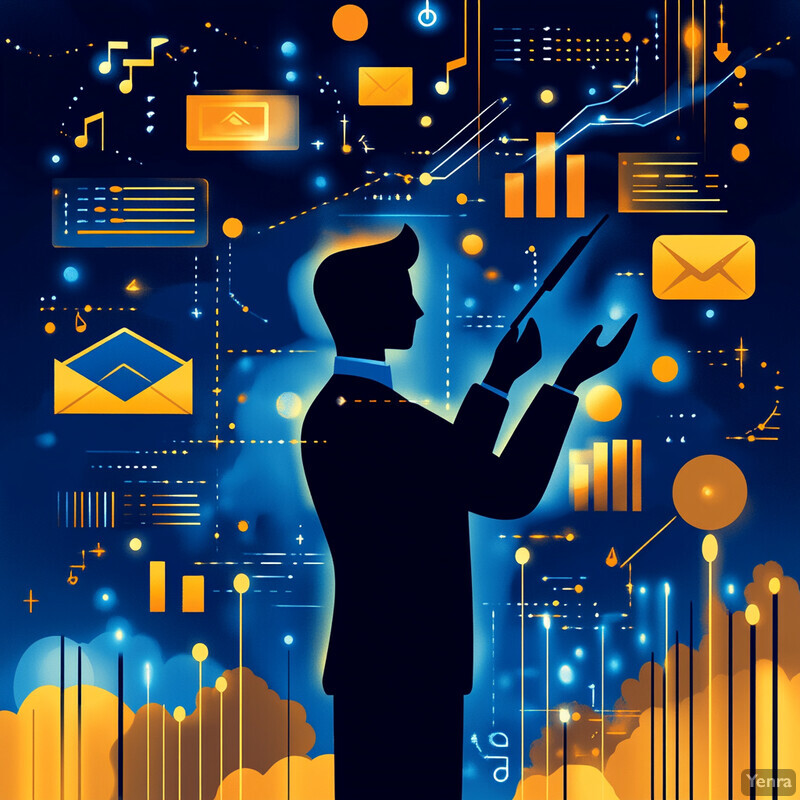
Not all customers prefer the same channels of communication. Some respond best to personalized email offers, while others engage more readily with SMS notifications or mobile push alerts. AI can assess each customer’s past engagement patterns, predicting which channels will be most effective at any given stage. By orchestrating the right message through the right medium—be it voice, chat, email, or social—AI ensures that brands communicate with customers in a manner that feels natural and convenient. This channel-optimized approach leads to higher response rates, deeper engagement, and a more harmonious overall journey.
15. Attribution Modeling
Advanced machine learning models can help businesses understand which journey touchpoints are most influential in driving conversions, allowing for more informed resource allocation.
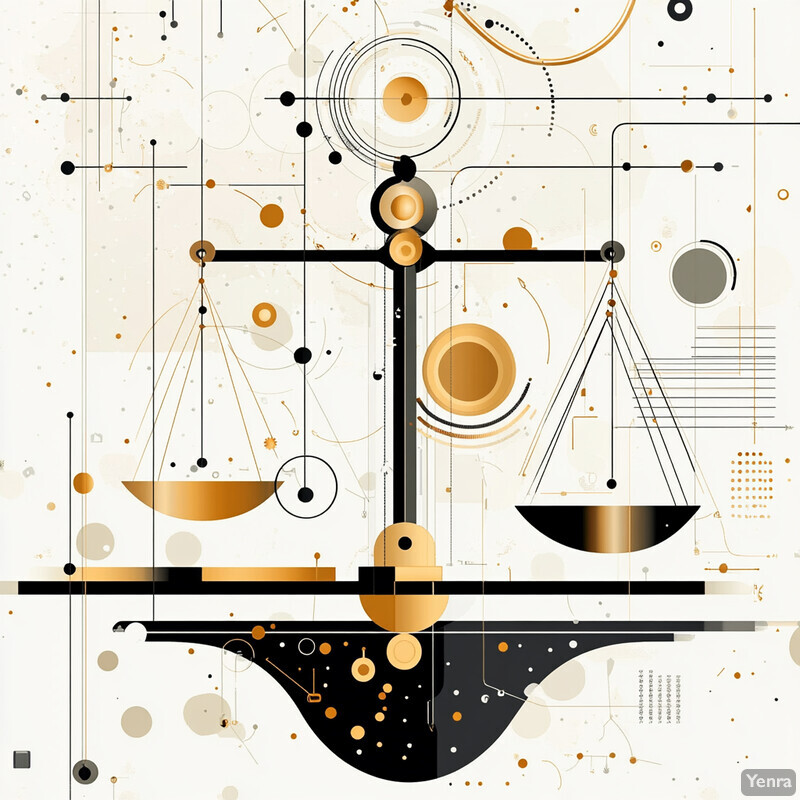
Understanding the relative impact of various marketing and engagement efforts is challenging. AI-powered attribution modeling can decode the complexity by assigning value to each touchpoint—from the initial ad click to the final checkout button—based on how it contributes to the ultimate conversion or outcome. By revealing which steps in the journey are most influential, AI allows teams to allocate resources more effectively, double down on what works, and refine or remove low-performing interactions. This evidence-based approach ensures that every component of the journey meaningfully contributes to the desired results.
16. Early Warning Indicators
AI can raise alerts when certain KPIs (like NPS drops or click-through rates fall below expected thresholds) deviate from norms, enabling quick intervention.
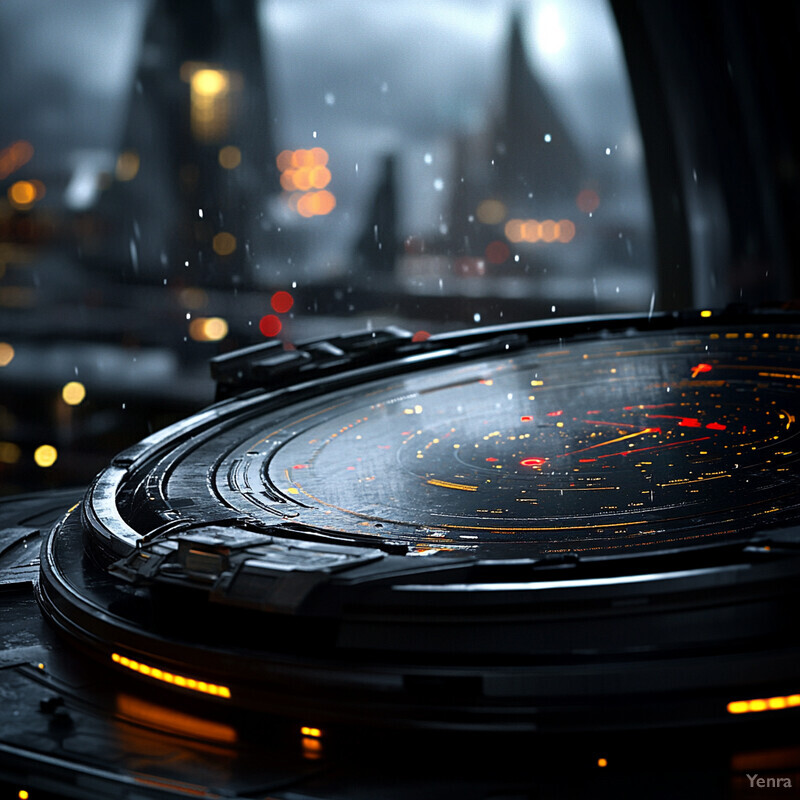
Business performance metrics often shift gradually, and small deviations can be easy to overlook until they become pressing problems. AI excels at identifying subtle trends and early warning indicators—for example, a slight uptick in support tickets related to a particular feature or a minor decrease in a key engagement metric. By raising alerts early, these indicators give companies the opportunity to investigate and respond before minor issues escalate into significant barriers. Through continuous monitoring and alerting, AI keeps organizations vigilant, nimble, and ready to course-correct at a moment’s notice.
17. Predictive Personalization of Content
Content engines powered by AI can anticipate what types of information, visuals, or stories will resonate most at various stages, increasing engagement and satisfaction.
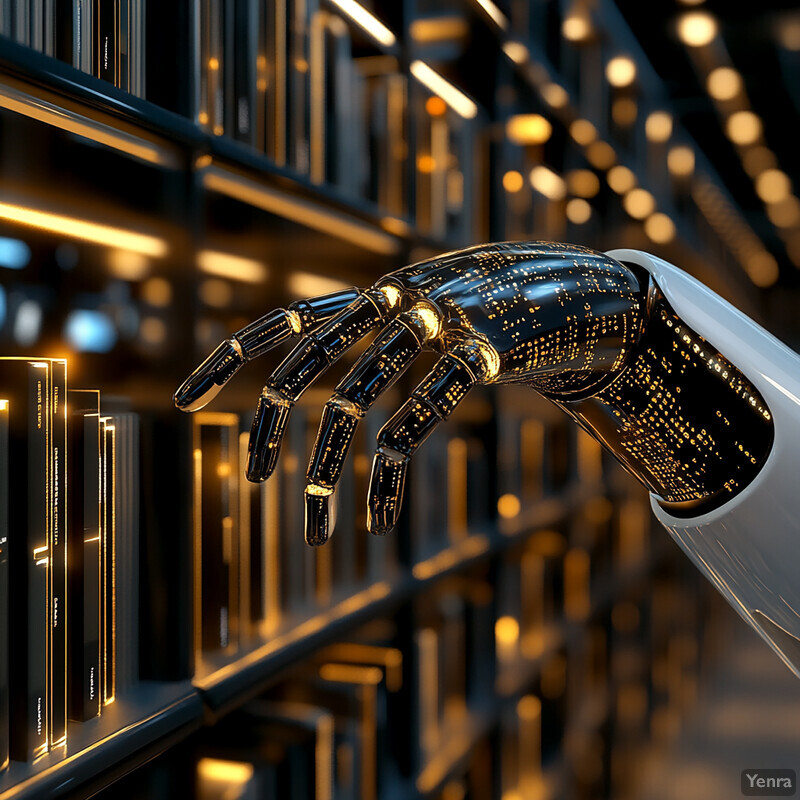
Content is a key driver of engagement, but not all customers respond the same way to identical messages, images, or offers. With AI, businesses can predict what types of content are likely to resonate with individual segments or even specific users. These models consider factors like browsing history, known interests, or past content engagements to serve more appealing articles, videos, or promotional materials. Over time, this predictive personalization fosters deeper loyalty, as customers find that every piece of content feels curated just for them, guiding them more naturally and enjoyably through their journey.
18. Enhanced Empathy Modeling
AI can simulate how customers feel at each stage by analyzing sentiment, historical behaviors, and context, guiding businesses to deliver more empathetic, human-like interactions.
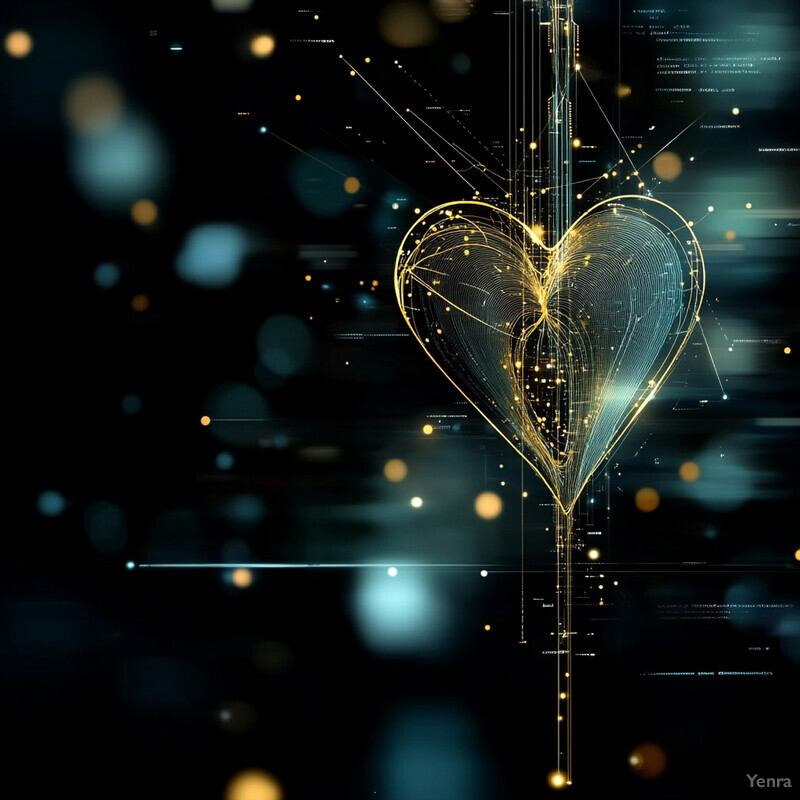
Great customer experiences aren’t just about efficiency—they’re about understanding how customers feel at each point. AI can approximate empathy by analyzing sentiment data, usage patterns, and contextual cues to infer what emotions customers might be experiencing. If the data suggests users become anxious at a certain form or frustrated during a particular process, the company can respond by simplifying interfaces, adding supportive messaging, or providing gentle encouragement. By refining touchpoints to reflect the customer’s emotional state, journey mapping becomes more human-centric, nurturing trust, comfort, and positive brand associations.
19. Cost and ROI Optimization
By identifying inefficiencies and waste in the customer journey, AI can recommend where to focus resources for maximum impact, optimizing budgets and boosting overall returns.
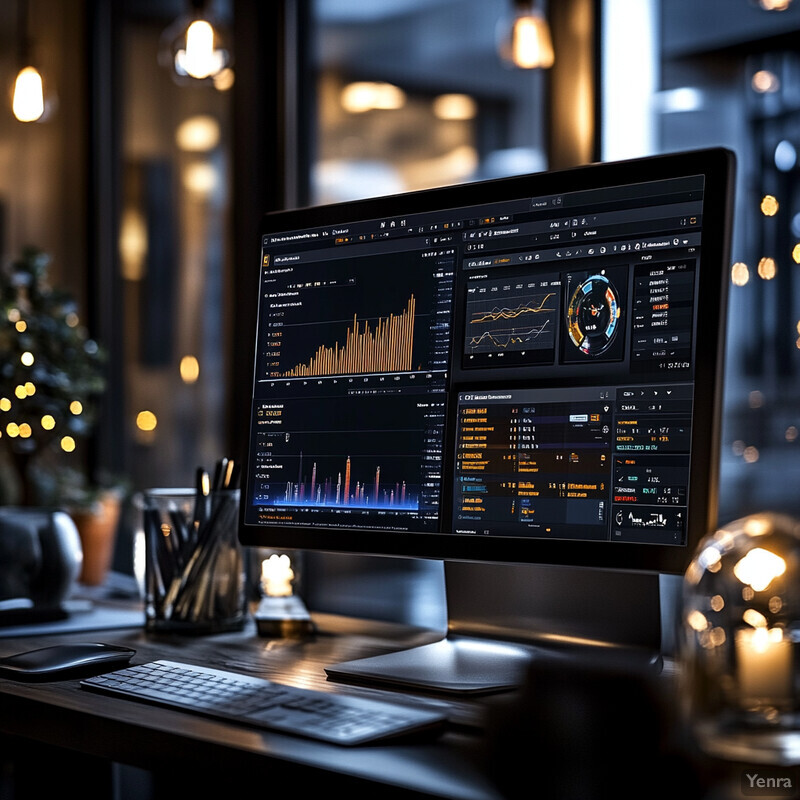
Maximizing return on investment is a priority for any business. AI can analyze a wealth of journey data—including costs associated with marketing campaigns, customer acquisition, and retention strategies—and correlate them to outcomes like conversion rates or average order value. By pinpointing which efforts yield the highest returns and which resources are underutilized, AI provides clear guidance for optimizing spending. This ensures that each step of the customer journey not only enhances the user experience but also contributes to the company’s financial health, enabling data-driven budget allocation and strategic resource planning.
20. Continuous Improvement Loops
With AI continuously learning from new data, companies can regularly update and refine their customer journey maps, ensuring they remain relevant, effective, and aligned with evolving customer needs.
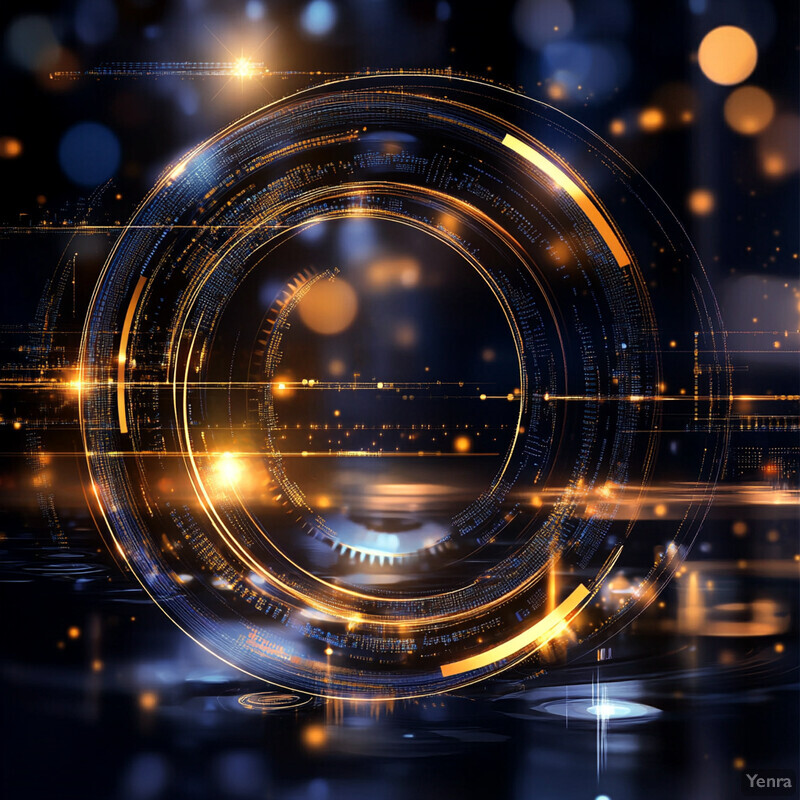
Customer behaviors and market conditions change continuously, and journey maps must evolve accordingly. AI’s self-learning algorithms thrive in this environment, constantly absorbing new data, recognizing new patterns, and updating predictive models. This creates a perpetual improvement cycle: customer journeys that are continually refined, tested, and optimized based on the latest evidence. Over time, the result is a living blueprint of the customer experience—one that adapts to shifting demographics, emerging technologies, and evolving customer expectations. Such continuous evolution helps companies stay ahead of the curve, delivering ever more streamlined, satisfying, and meaningful customer journeys.