1. Image Recognition for Artifacts
Deep learning-based image classification algorithms can quickly identify artifacts in photographs, even from partial or damaged images, helping scholars confirm authenticity and origin.
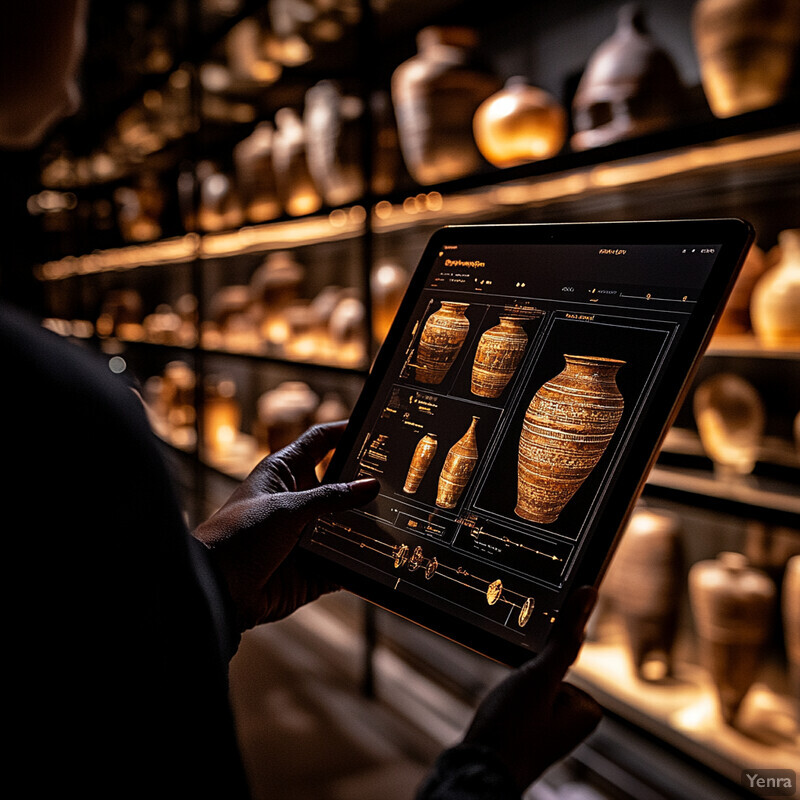
Advanced deep learning algorithms allow for the rapid and accurate identification of cultural artifacts from images, even when these objects appear partially damaged or are photographed at challenging angles. By training on vast datasets of known artifacts, these systems learn to recognize distinctive shapes, patterns, and surface details unique to particular cultures or historical periods. This technology enables researchers and curators to quickly sort through large image libraries or on-site excavation photographs, efficiently filtering out objects of interest and streamlining the identification process. Moreover, these AI-driven image recognition tools facilitate immediate classification, which traditionally depended heavily on the expertise and availability of a limited pool of specialists.
2. Style and Motif Analysis
By learning patterns of artistic styles, symbols, and motifs common to certain regions or eras, AI can assist in attributing unknown artifacts to particular cultural traditions or workshops.
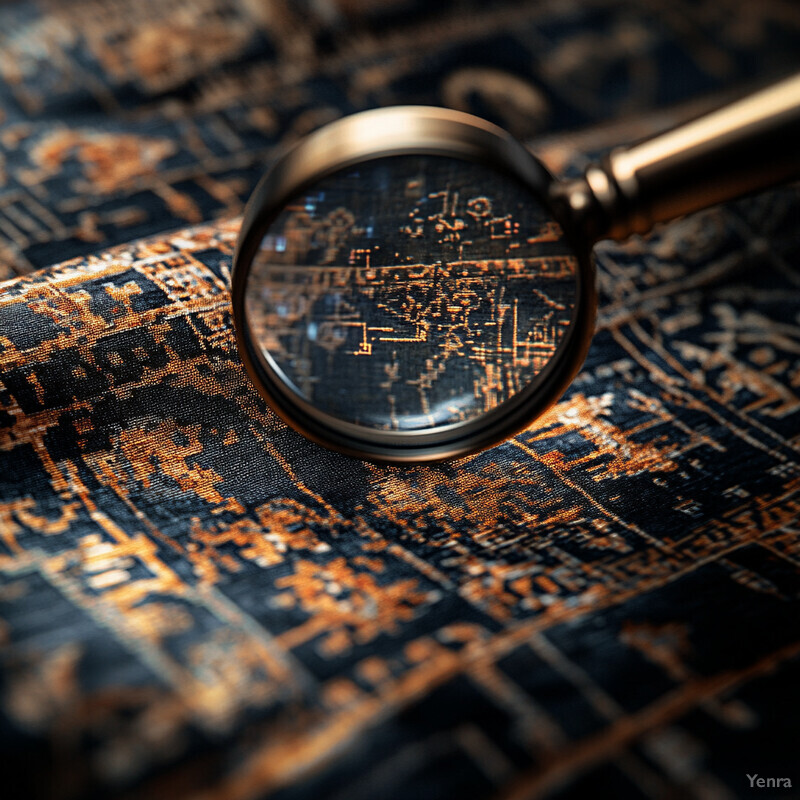
Cultural artifacts often carry signature motifs and stylistic elements that tie them to specific regions, artisan workshops, or historical eras. AI models, trained on extensive examples, can detect and categorize subtle stylistic nuances that human observers might overlook. By identifying these recurring patterns—whether it’s a particular ornamental swirl on pottery, a common geometric arrangement in textiles, or the brushwork technique in a painting—AI can help scholars narrow down an artifact’s cultural origins and timeline. This approach helps unify insights from multiple disciplines, allowing museums, historians, and archaeologists to attribute uncertain objects more confidently and accurately.
3. Material Composition Analysis Using Spectral Data
Machine learning models can interpret data from multispectral or hyperspectral imaging to determine the composition of materials—such as pigment types in paintings or mineral content in ceramics—leading to more accurate cultural and chronological classification.
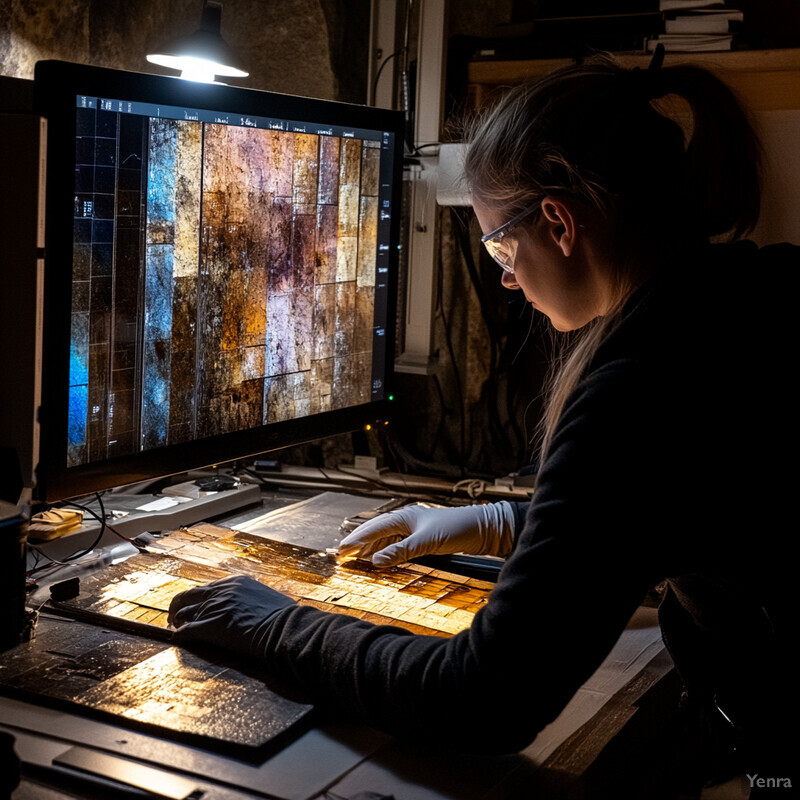
Many archaeological and historical investigations rely on understanding the materials used to create an artifact. By interpreting data from multispectral or hyperspectral imaging, AI models can “see” beyond the visible spectrum, detecting pigments, mineral compositions, and chemical residues embedded in an object’s surface. Such comprehensive analysis allows for more precise cultural identification since particular materials were often regionally or period-specific. In this way, AI-powered spectral analysis supports restorers, archaeologists, and historians in uncovering trade patterns, production techniques, and resource availability that shaped cultural expression.
4. Automated Dating and Chronology Estimates
AI can correlate stylistic and material attributes with known historical timelines, offering estimates for when a piece was created. Over time, this reduces reliance on manual, time-consuming methods.
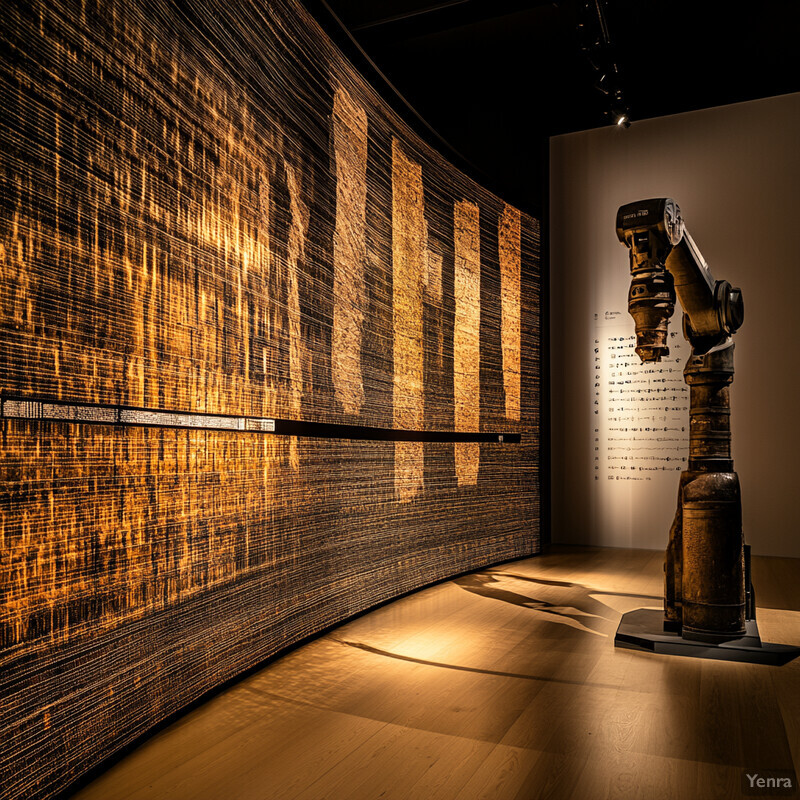
Determining the age of an artifact is a cornerstone of cultural identification. AI-driven techniques can leverage patterns found in stylistic attributes, manufacturing methods, and material compositions to estimate the time period during which an object was created. Over time, as these systems learn from countless dated examples, they become increasingly proficient at correlating certain features with distinct historical epochs. This automated approach greatly assists in building comprehensive timelines without the prolonged manual effort that typified traditional methods. Consequently, researchers can accelerate their work in assembling historical narratives and understanding cultural evolution.
5. Translating Epigraphic Inscriptions
Natural language processing (NLP) models trained on ancient scripts and languages can help decipher inscriptions on artifacts, plaques, and tablets, making the identification and understanding of cultural context more accessible.
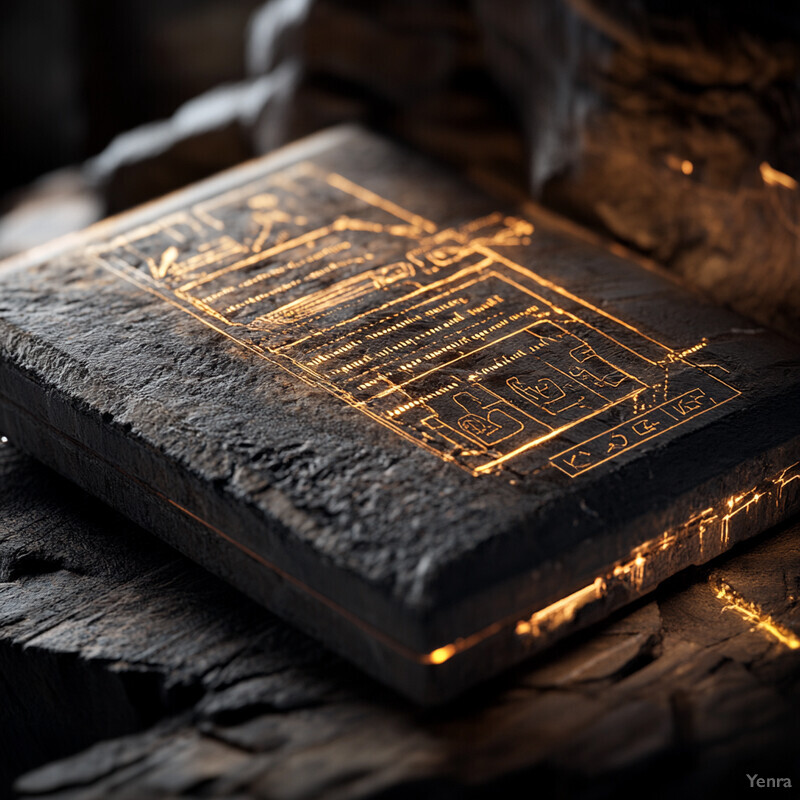
Inscriptions found on ancient artifacts—whether they are carved reliefs, engraved plaques, or handwritten manuscripts—hold a wealth of cultural, religious, and historical information. AI-powered natural language processing models, trained on large corpuses of ancient scripts, can help decode these messages even when the languages involved have limited known lexicons. By analyzing character patterns, linguistic structures, and contextual clues, these systems can produce meaningful translations or partial interpretations that guide experts towards deeper understanding. As these models improve, they become invaluable tools in unlocking stories hidden in objects, bridging gaps in historical records, and enriching our appreciation of cultural heritage.
6. Mapping Geographic Origins
By comparing features of artifacts with known cultural distributions, AI can help map an object’s likely place of origin, offering insights into trade routes, cultural exchanges, and migration patterns.
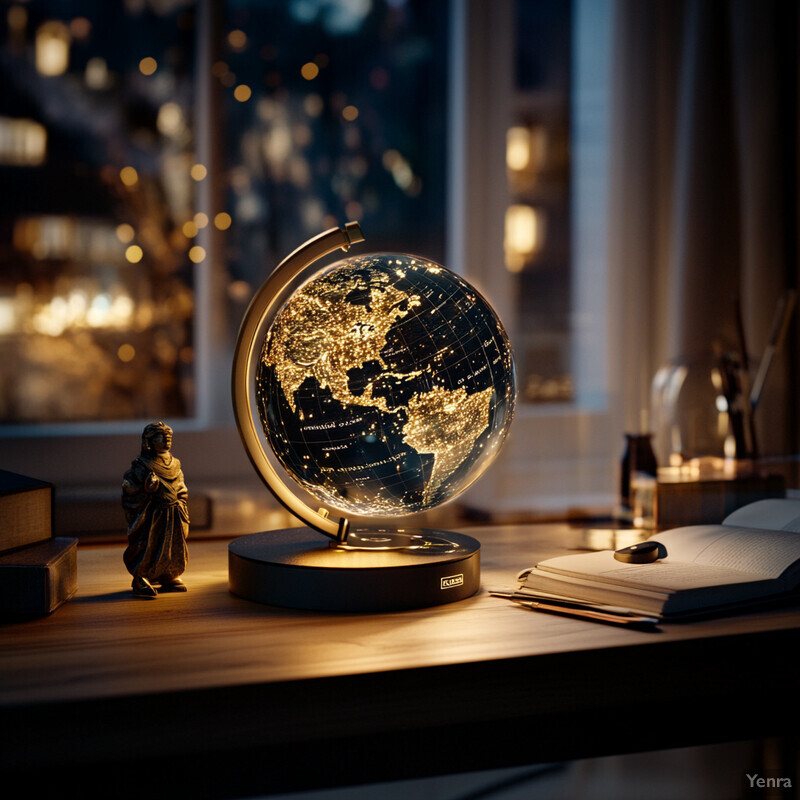
Determining where an artifact originated is crucial for understanding cultural exchanges, trade routes, and historical migration patterns. AI techniques can match an object’s stylistic, material, and iconographic features to similar known artifacts from particular regions. By compiling and analyzing extensive data sets of materials, designs, and production techniques, these models help researchers pinpoint likely cultural and geographical origins. Over time, the continuous enrichment of these datasets allows for more precise clustering of artifacts into their cultural landscapes, enabling a fuller picture of how civilizations interacted and influenced one another.
7. Clustering and Classification of Large Collections
Museums and institutions often struggle with enormous, unlabeled collections. AI-driven classification can cluster similar objects, making large-scale cataloging more efficient and revealing previously unnoticed patterns.
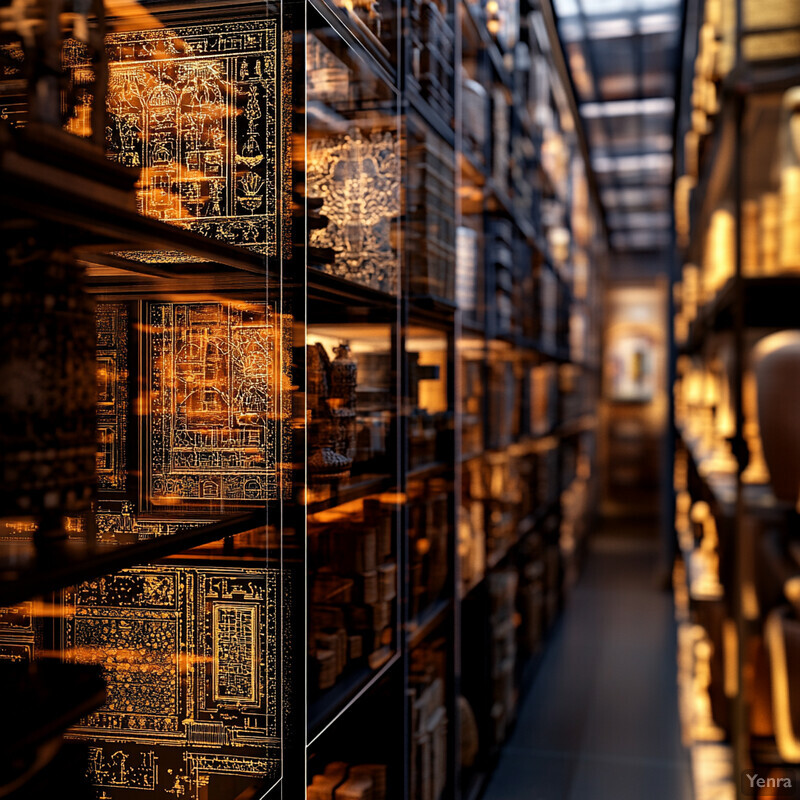
Museums, archives, and archaeological institutions often hold enormous collections, many of which remain uncategorized. AI-driven clustering algorithms can group objects based on visual similarity, materials, or stylistic features, making it significantly easier to navigate and understand large assemblages. Rather than individually cataloging each piece, which is slow and resource-intensive, these advanced techniques quickly highlight patterns and associations. The resulting classifications can lead to new insights into cultural practices, production methods, and historical developments, and also simplify the work of curators who must often make sense of vast, heterogeneous inventories.
8. Provenance Research Assistance
Algorithms can sift through large datasets—archives, auction records, and museum catalogues—to find matches or references to a given object, helping researchers trace provenance and avoid illicitly trafficked cultural materials.
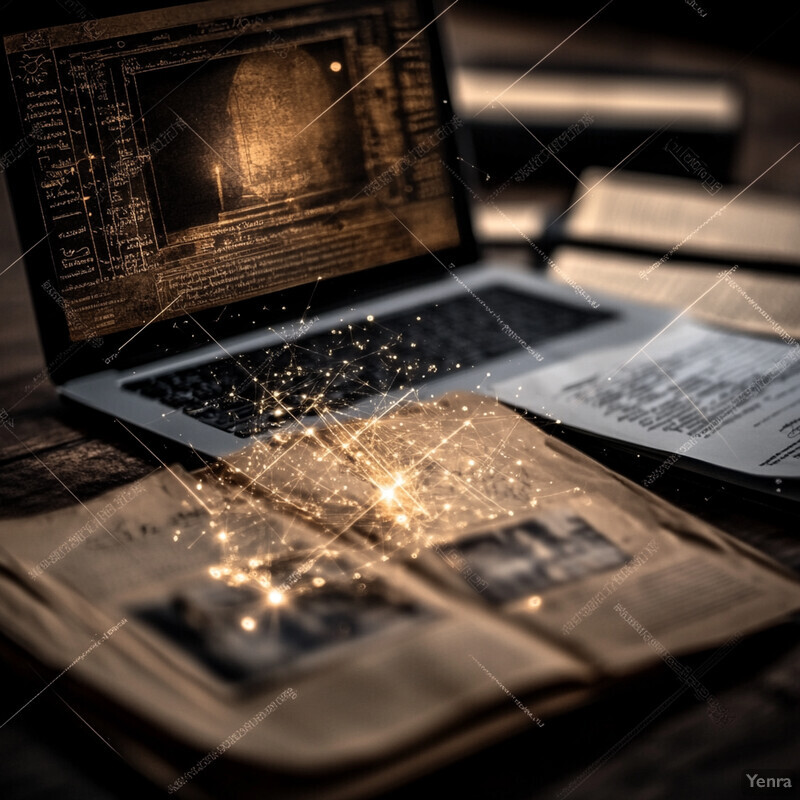
Provenance—the history of ownership and origin of an artifact—is a key factor in understanding cultural context and ethical considerations. AI tools can help trace an object’s past by searching through digital databases, auction records, academic publications, and museum catalogues. By using pattern recognition and textual analysis, these models can locate references to similar pieces, identify sales patterns, or connect an artifact to a particular known collection. This capability helps museums and collectors confirm authenticity, return stolen objects to their rightful owners, and ensure that cultural heritage is preserved and appreciated responsibly.
9. Damage and Restoration Analysis
AI can identify wear patterns, micro-cracks, and missing pieces in artifacts. By comparing these markers to known standards, it aids conservators in understanding how to best preserve and restore fragile objects.
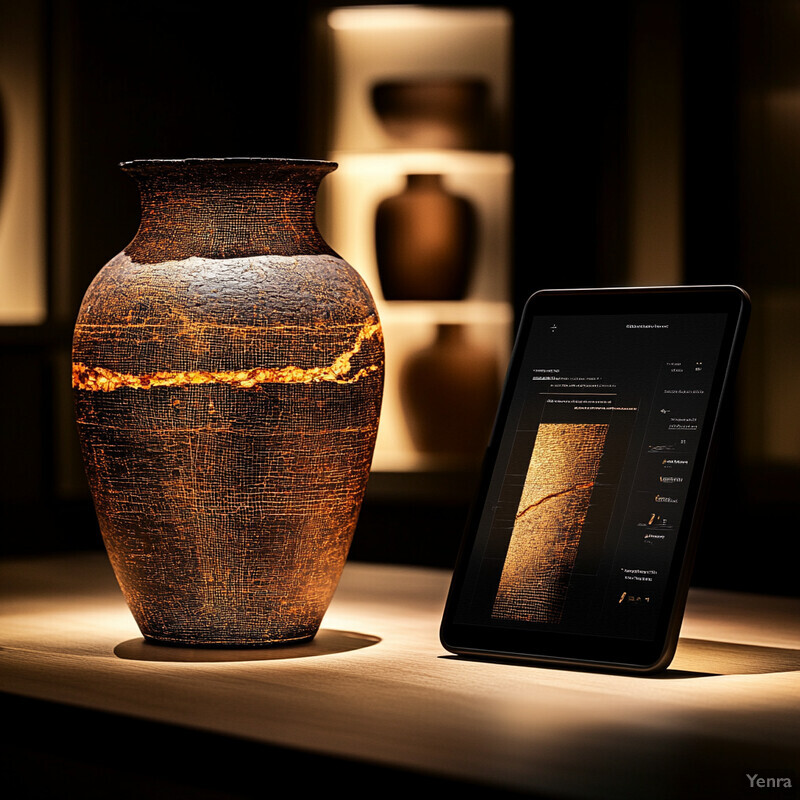
Over time, artifacts suffer wear and tear, and restorations are sometimes needed to preserve them for future generations. AI models trained on large image sets of undamaged objects can identify discrepancies in texture, color, and structure, pinpointing where deterioration or past repairs have occurred. By comparing an artifact’s current condition with known reference standards, these systems guide conservators on how best to restore it. Such data-driven insights optimize the choice of restoration materials, techniques, and timing, ultimately helping maintain the artifact’s cultural integrity and extending its lifespan.
10. Predictive Modeling of Lost Artifacts
Through pattern recognition, AI can generate hypotheses about the existence of currently unlocated artifacts. For example, if certain types of pottery are known to exist in one region, the model might predict that similar items should be found elsewhere due to historical trade routes.
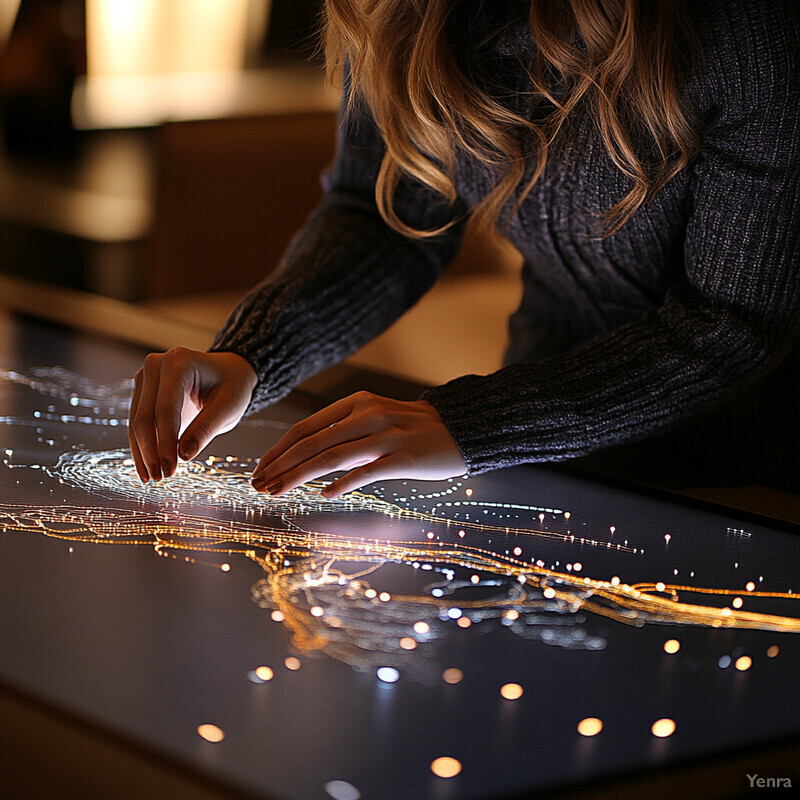
Historical records and cultural mapping often hint at the existence of significant artifacts that have yet to be found. Using patterns observed in the distribution of discovered objects—such as common trade routes or recurring manufacturing centers—AI can predict where similar items might be unearthed in the future. Archaeologists can then focus excavation efforts more strategically, reducing the costs and time associated with exploratory digs. By acting as a digital compass, predictive modeling helps fill gaps in cultural narratives and ensures that the story of past civilizations becomes more complete over time.
11. Virtual Reconstruction of Fragmented Artifacts
Computer vision algorithms can virtually “piece together” broken or partial artifacts by recognizing shapes, textures, and curvature. This can help identify the original form and cultural classification of fragmented objects.
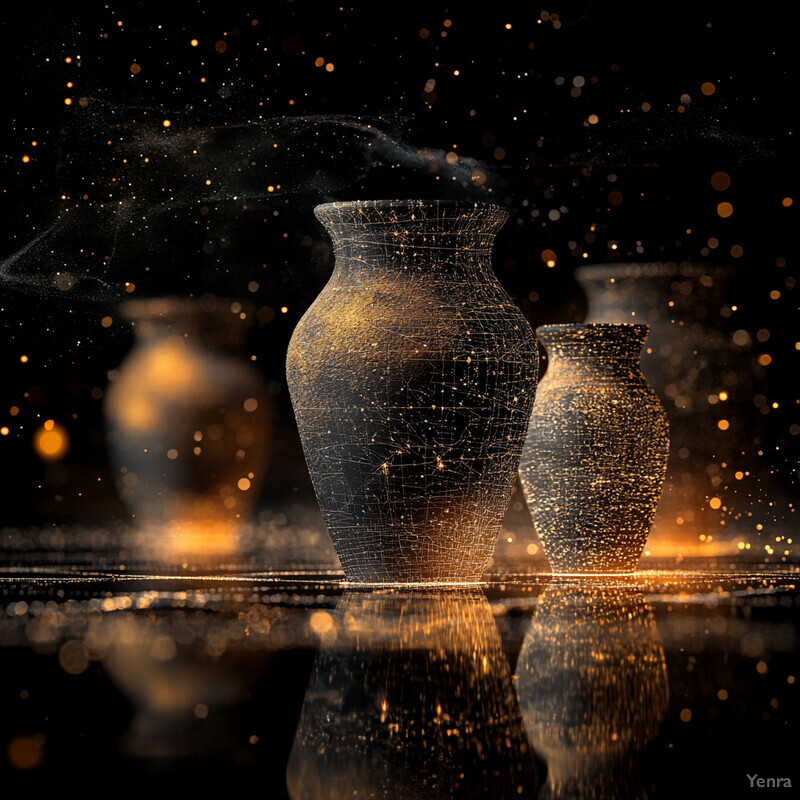
Archaeological excavations frequently yield fragmented pieces of pottery, statues, and other cultural objects. AI-driven computer vision and 3D modeling technologies can virtually piece together these fragments by analyzing their shapes, textures, break patterns, and surfaces. The reconstructed virtual models enable scholars to visualize how the original artifact may have looked and better understand its function and cultural significance. Beyond restoration insights, this technology aids in identifying artifact types that might otherwise remain unrecognized, shining new light on lost or incomplete pieces of cultural heritage.
12. Semantic Search in Databases
With AI-driven semantic search, researchers can query vast databases of cultural artifacts by describing features, contexts, or functions rather than relying solely on keyword-based searches. This makes comparisons and identifications more intuitive.
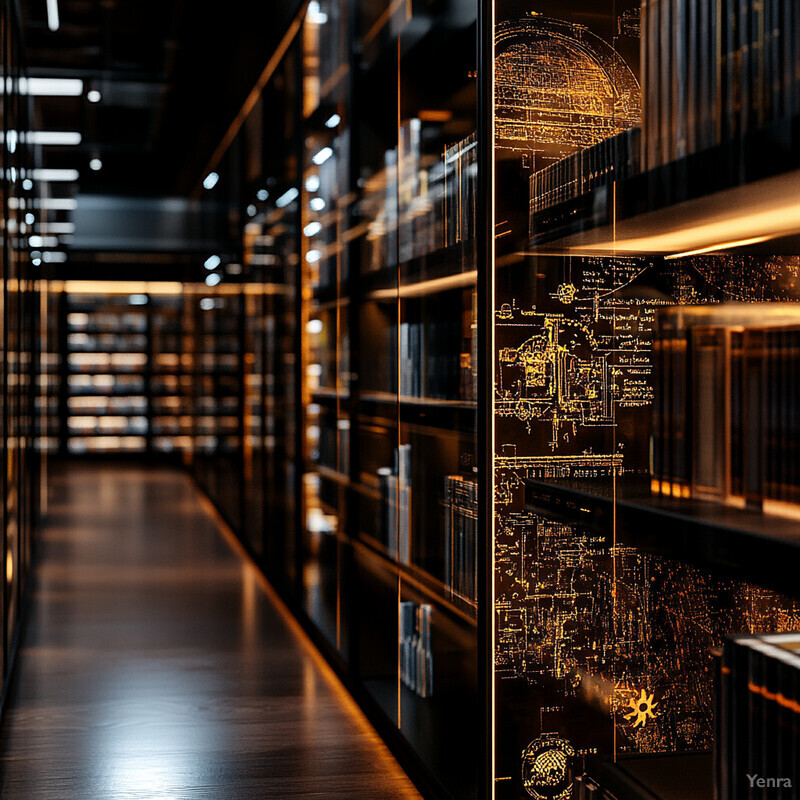
Traditional keyword-based searches in museum or archaeological databases can be limiting, as researchers may not know the precise terms to find what they need. AI-powered semantic search engines enable more natural querying, allowing scholars to describe attributes, cultural contexts, or functions of artifacts in everyday language. These advanced systems interpret the user’s intent, relating descriptive phrases to relevant objects and scholarly references. As a result, semantic search not only expedites research but also bridges linguistic and disciplinary gaps, fostering multidisciplinary discoveries and broadening our understanding of cultural artifacts.
13. Recommendation Systems for Curators
Just like online shopping recommendations, AI can suggest related artifacts for further study or exhibition based on stylistic or historical similarities, aiding curators in understanding cultural linkages.
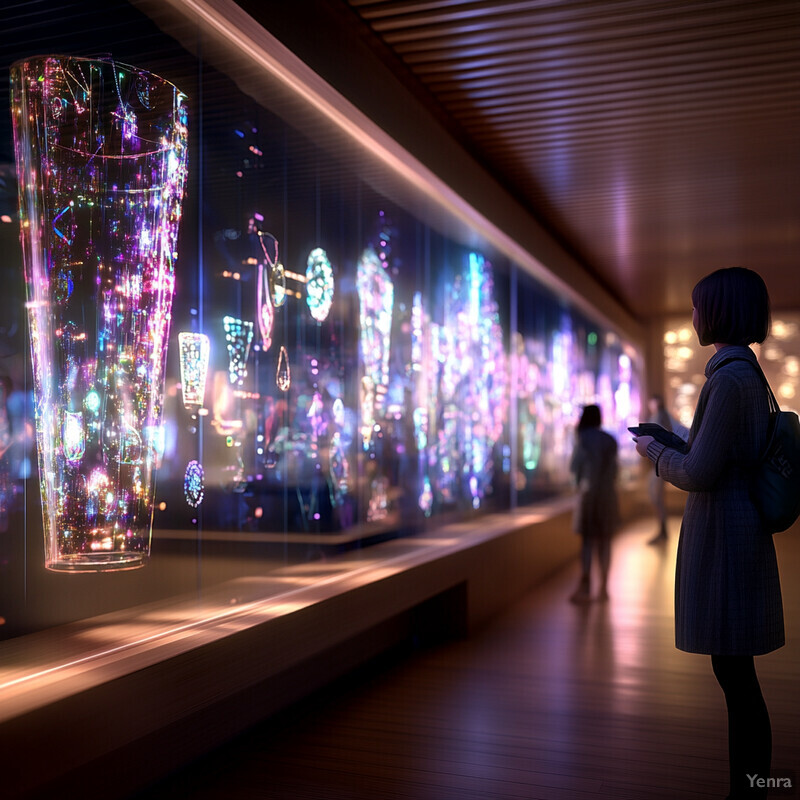
Cultural heritage experts often rely on their personal experience to find related artifacts for exhibitions, research, or educational materials. By applying recommendation algorithms—similar to those used by streaming services and online retailers—AI can suggest related objects that share stylistic, thematic, or temporal connections. This helps curators discover hidden links within their collections, plan more cohesive exhibitions, and provide richer narratives for visitors. Over time, these systems also adapt to curator feedback and evolving scholarly insights, ensuring that recommendations grow increasingly relevant and enlightening.
14. Pattern Recognition in Decorative Elements
Deep learning models can detect recurring decorative patterns or iconography across artifacts, linking them to specific cultural groups, religious traditions, or artisan guilds.
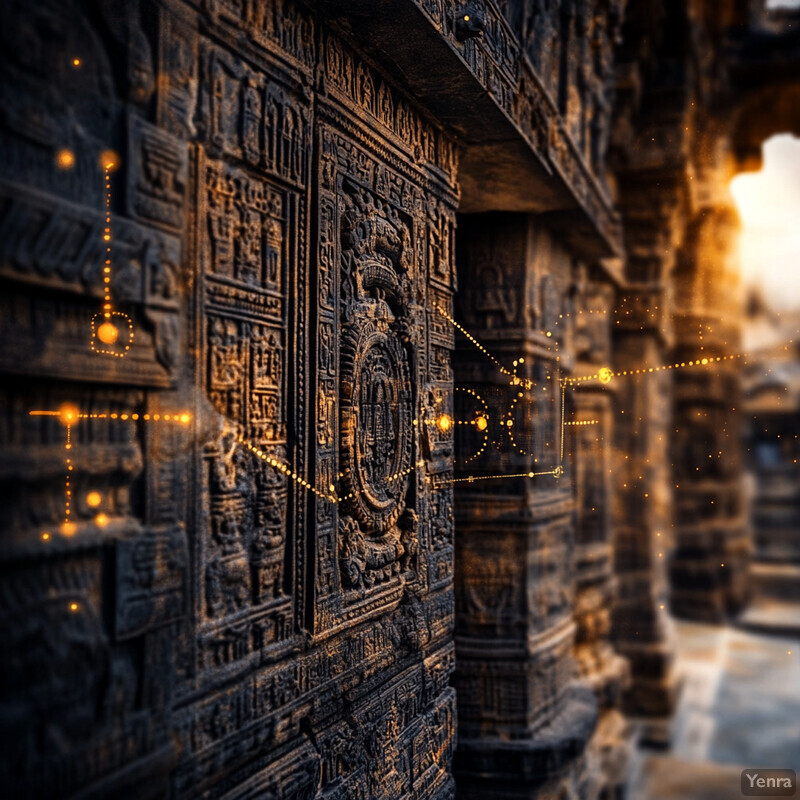
From recurring geometric shapes in a pottery tradition to intricate iconography in religious statuary, decorative patterns can serve as cultural fingerprints. AI can systematically analyze complex imagery to recognize subtle visual cues that connect an object to a particular craft tradition, artisan network, or cultural identity. These insights not only aid identification but also deepen our understanding of how certain designs traveled across regions and periods. By shining a spotlight on the visual language inscribed in artifacts, AI fosters a richer comprehension of cultural dialogues and aesthetic values.
15. Temporal and Cultural Contextualization
AI tools can cross-reference a piece’s attributes (like materials, decoration style, or inscriptions) with known historical events, movements, or influences, placing artifacts in a richer cultural narrative.
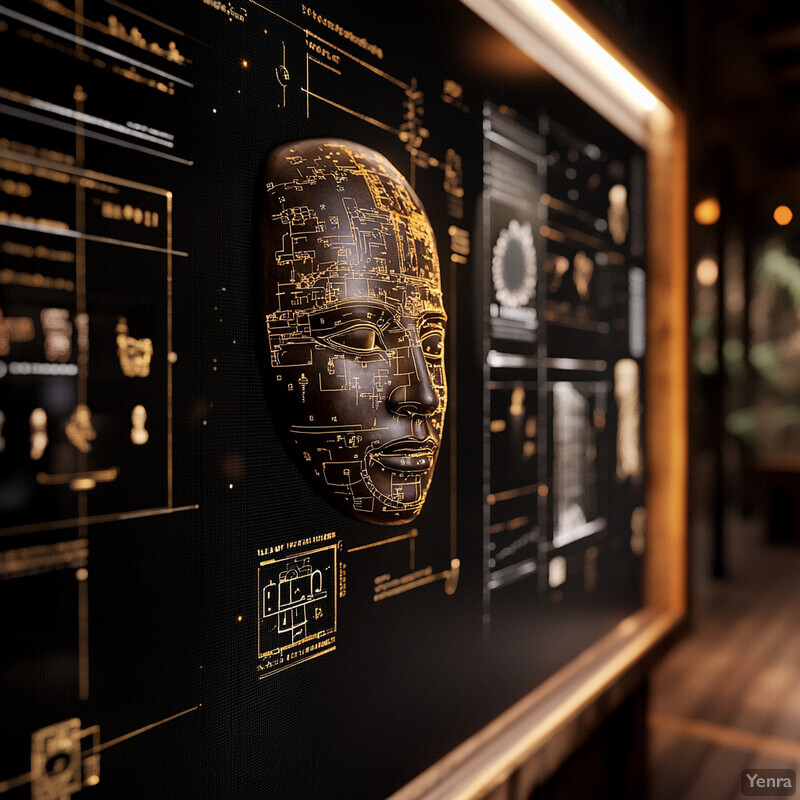
An artifact is not just an isolated object; it belongs to a web of historical events, social changes, and cultural influences. AI tools can draw on interdisciplinary datasets—encompassing historical records, anthropological studies, linguistic analyses, and archaeological surveys—to place an object into a broad cultural narrative. By correlating attributes of the artifact with known historical phenomena, these systems provide context that transcends the artifact’s physical properties. This integrated approach empowers researchers to appreciate not only what the artifact is, but also what it meant to the people who made, used, and revered it.
16. Automated Metadata Generation for Digital Archives
When scanning and digitizing collections, AI can automatically generate tags, keywords, and contextual data, making digital archives more user-friendly and searchable for researchers.
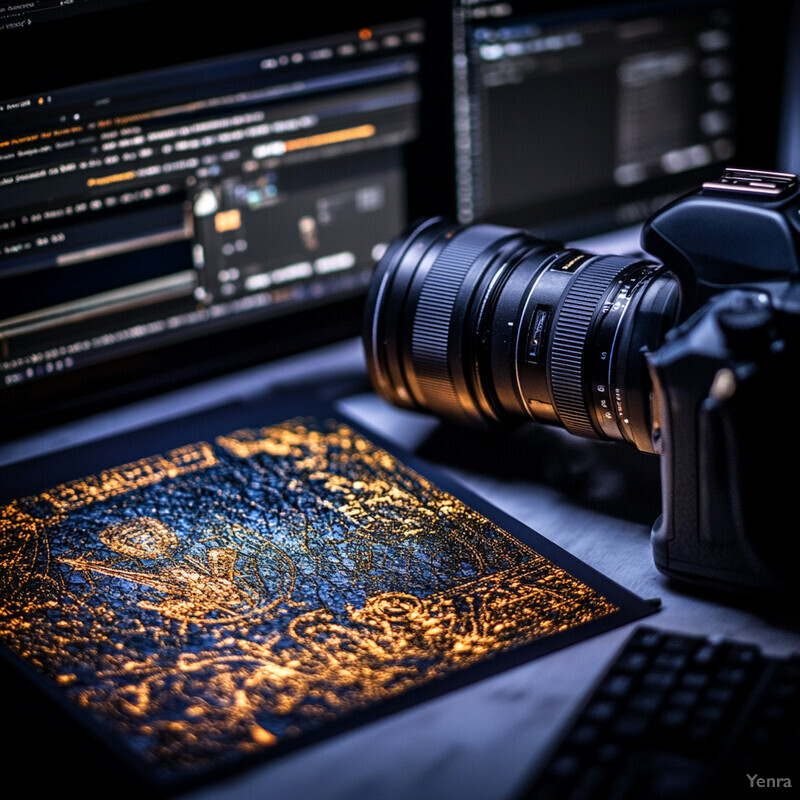
As cultural institutions continue to digitize their collections, creating accurate metadata (e.g., descriptions, tags, cultural affiliation) is both essential and time-intensive. AI can streamline this process by analyzing an artifact’s images and associated data, then automatically generating descriptive tags, contextual information, and classification details. This automation saves countless hours of manual labor and makes digital archives more intuitive to navigate. In turn, researchers and the public gain easier access to a wealth of cultural resources, fostering greater transparency and inclusivity in the field of heritage studies.
17. Rarity and Significance Detection
By comparing an artifact against a massive database of known pieces, AI can highlight unusual features that might indicate rarity, significance, or even the presence of a previously unknown cultural artifact type.
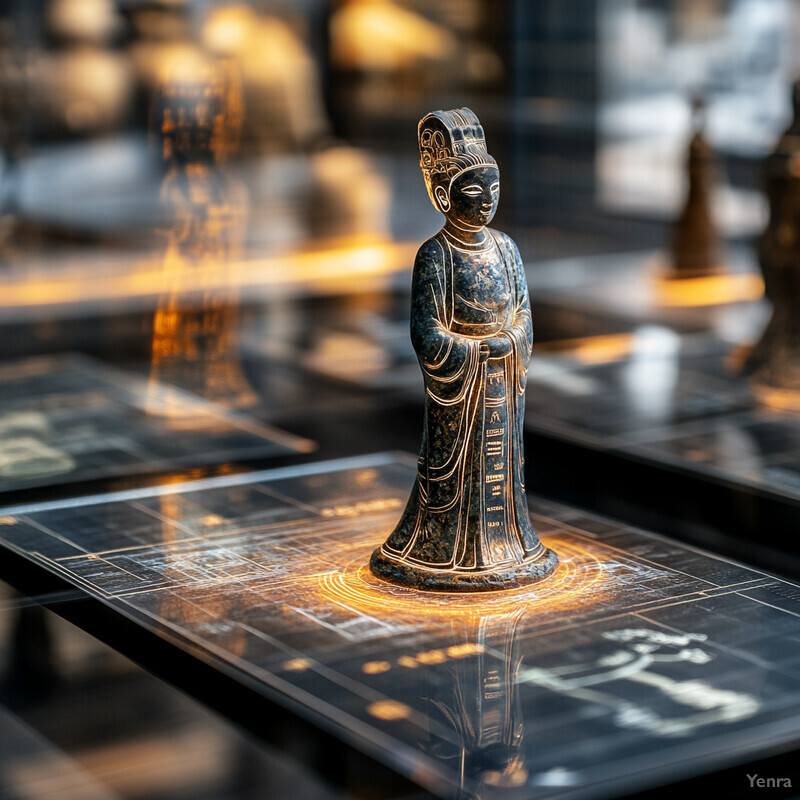
Not all artifacts are created equal—some are relatively common, while others are exceedingly rare or culturally pivotal. By comparing an artifact’s attributes against expansive reference databases, AI can gauge its uniqueness. Subtle differences in technique, materials, or iconography might hint at exceptional historical significance or reveal previously unknown artifact categories. This helps scholars prioritize their research efforts, draw attention to items that can revolutionize understanding of a given culture, and ensure that the most noteworthy discoveries receive the attention they deserve.
18. Identification of Fakes and Forgeries
With machine learning models trained on known authentic and fraudulent objects, AI can detect subtle anomalies in craftsmanship, materials, or iconography that suggest a modern forgery masquerading as an ancient piece.
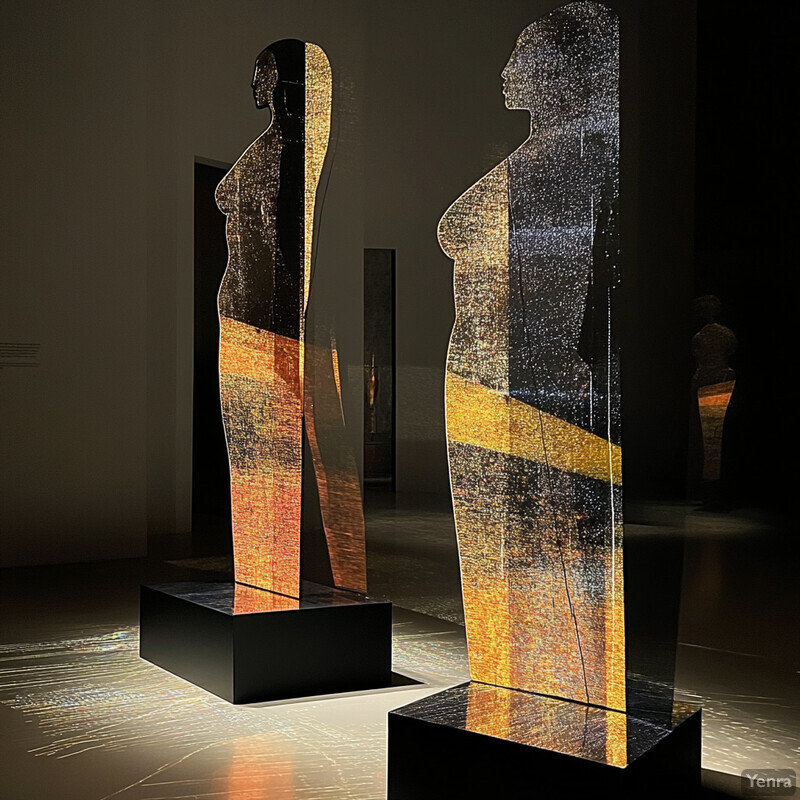
The cultural heritage market is vulnerable to forgery and fraud. AI helps expose these illicit practices by identifying patterns that differ from authentic works. Models trained on genuine examples learn to detect anomalies in material choice, manufacturing methods, stylistic consistency, and even microscopic details not easily perceived by the human eye. Spotting these red flags helps maintain the integrity of collections, assures buyers and museums of authenticity, and protects the cultural record from contamination by misleading objects.
19. Adaptive Learning From New Data
As new artifacts are discovered and documented, AI systems can continuously update their models. This iterative learning process helps refine identifications and ensures that new cultural knowledge is quickly integrated.
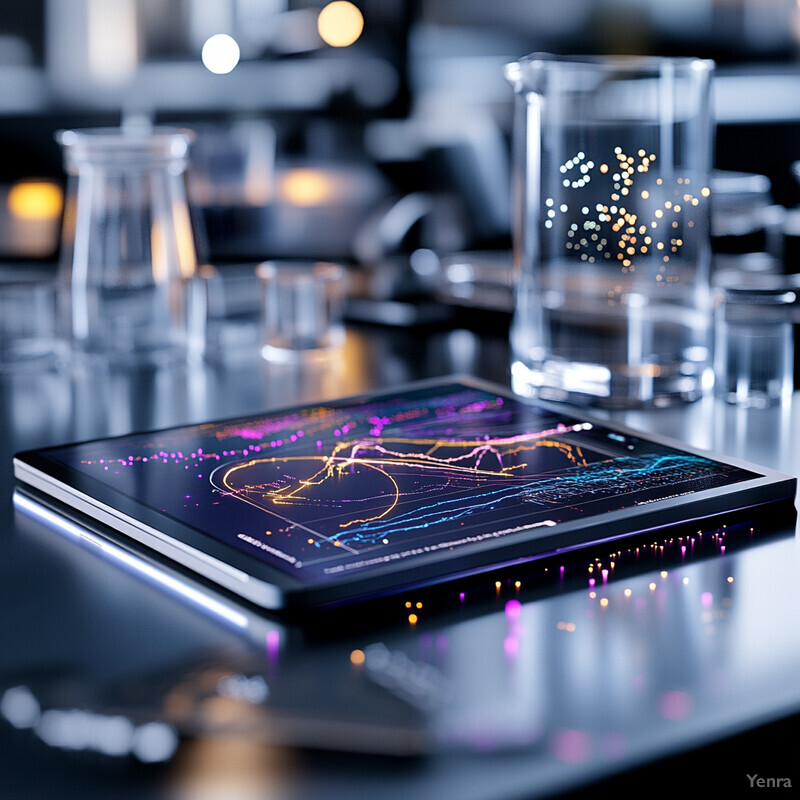
Cultural artifact identification is not static; as new pieces are discovered and fresh research emerges, our collective understanding evolves. AI systems excel at adaptive learning, updating their models regularly as they ingest newly digitized artifacts, corrected classifications, or revised historical interpretations. This continual refinement ensures that AI-driven identification remains accurate, relevant, and aligned with the latest scholarship. Ultimately, this adaptability improves the quality of cultural databases, enriches academic discourse, and encourages collaboration across disciplines.
20. Cross-Disciplinary Correlations
AI can integrate data from anthropology, archaeology, linguistics, materials science, and art history, correlating varied information streams to provide a multifaceted understanding of an artifact’s cultural background. This synthesis leads to more holistic identifications and interpretations.
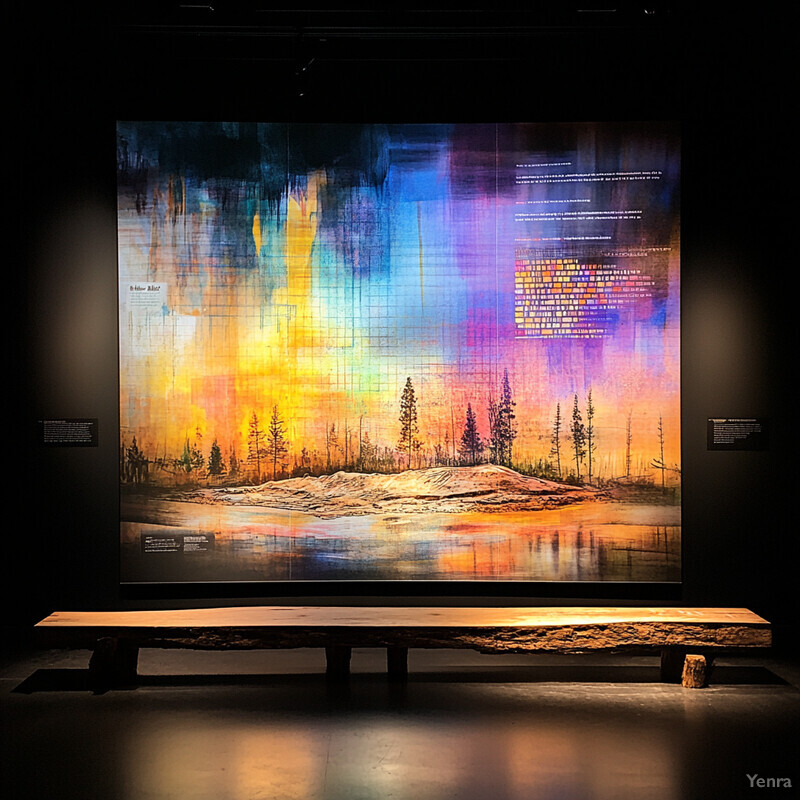
Cultural artifacts do not exist in a vacuum; they are shaped by geography, technology, politics, religion, and the creative impulses of their makers. AI models can integrate information from varied fields—like archaeology, anthropology, art history, linguistics, materials science, and even climate studies—to draw comprehensive connections. This synthesis transcends the boundaries of any single discipline, allowing for more holistic interpretations. The result is a richer, fuller understanding of an artifact’s role within its cultural ecosystem, illuminating how past societies interacted, innovated, and expressed their identities through material culture.