1. Dynamic Yield Modeling
AI-driven predictive models can accurately estimate future yields for different crop rotations, helping farmers choose the best sequences that maximize output.
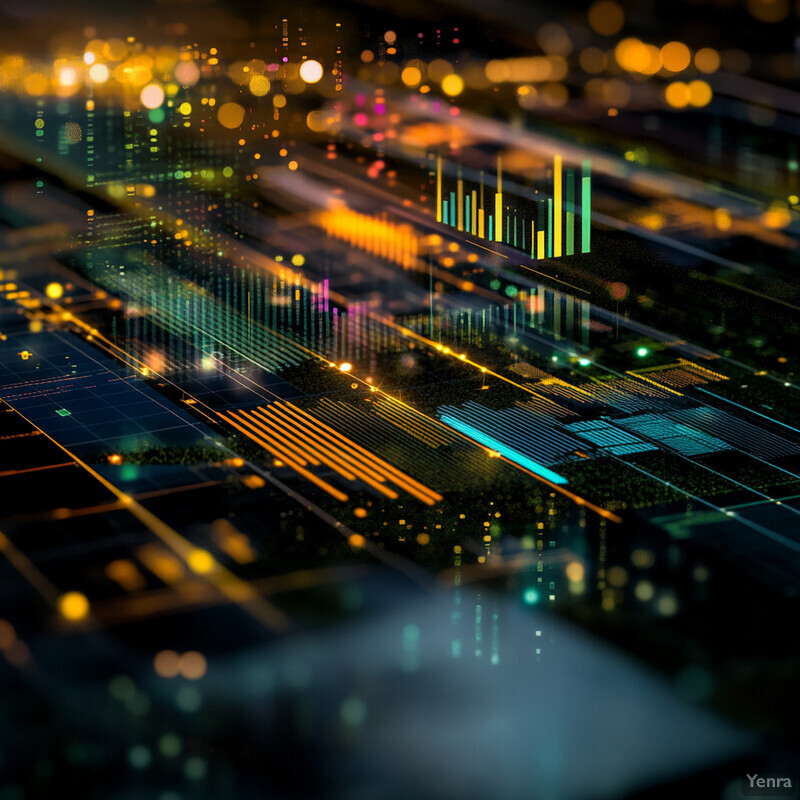
AI-driven predictive models leverage historical yield data, local soil composition, weather forecasts, and regional agricultural trends to anticipate the performance of various crops over multiple seasons. By processing vast datasets, these models identify the most productive sequences of crops in a rotation, considering factors such as planting density, soil nutrient uptake rates, and pathogen pressure. Farmers can use these simulations to select crop rotations that balance short-term gains with long-term sustainability. Over time, as new data flows in, the models continuously refine their predictions, ensuring that farmers can maintain or improve yield outcomes in shifting environmental and market conditions.
2. Soil Health Analysis
Advanced machine learning techniques integrate data from soil sensors, past crop performance, and environmental conditions to determine how different rotations affect soil nutrient levels, pH balance, and organic matter.
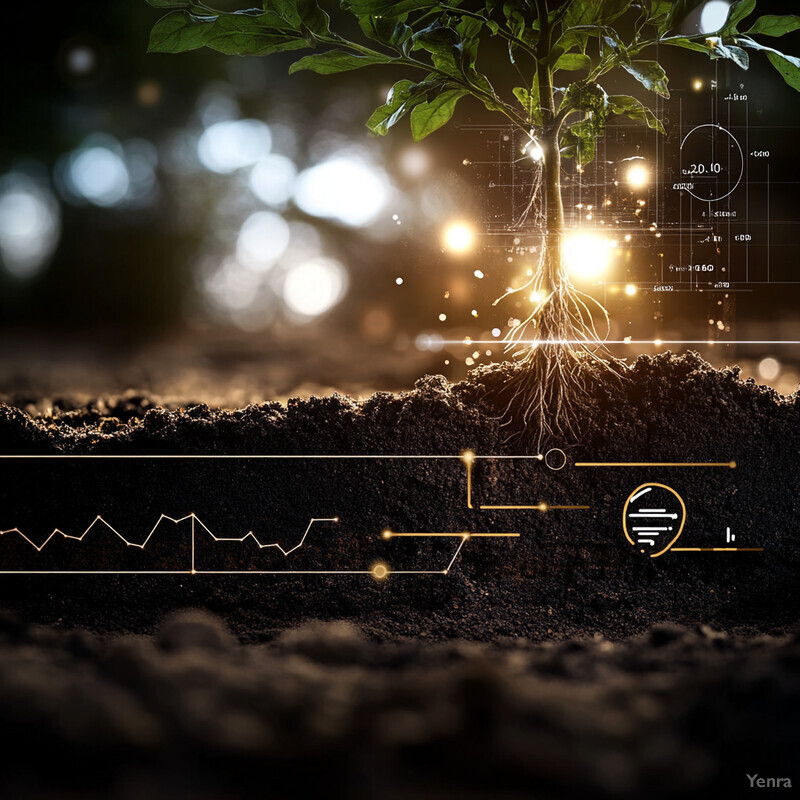
Modern machine learning techniques integrate sensor inputs—such as soil moisture, pH levels, and nutrient concentrations—with historical rotation records and agronomic best practices. AI tools can detect subtle trends that human observers might miss, such as gradual declines in soil organic matter or hidden mineral deficiencies that emerge over multiple crop cycles. By pinpointing how specific crop rotations influence soil texture, structure, and biological activity, farmers receive precise, data-driven guidance on maintaining healthy soil. This long-range perspective helps them design rotations that improve fertility, enhance beneficial microbial communities, and safeguard the land’s productive capacity.
3. Pest and Disease Forecasting
AI models use historical and real-time data on pest populations, weather patterns, and crop vulnerability to predict outbreaks and recommend crop rotations that disrupt pest life cycles.
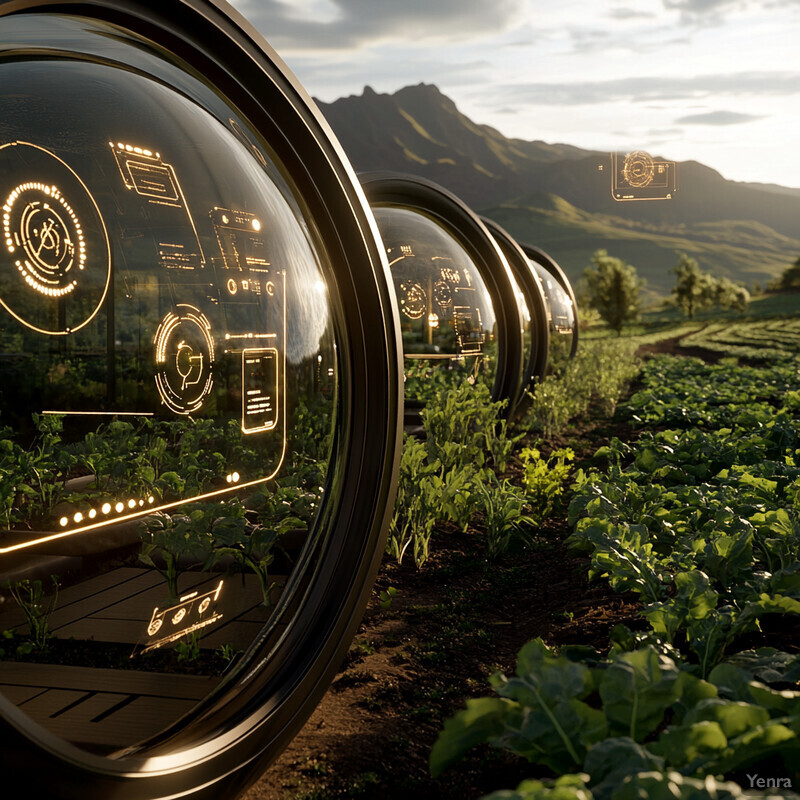
Using pattern recognition and predictive analytics, AI can analyze historical pest and disease outbreak data, linking it with weather patterns, soil conditions, and crop susceptibilities. By recognizing conditions that favor certain pathogens or insect populations, AI can recommend rotations that break these organisms’ life cycles. For example, alternating between susceptible and resistant crops can slow the spread of a fungal disease or disrupt a pest’s breeding timeline. This foresight reduces reliance on pesticides and fungicides, lowering input costs and environmental impacts while preserving crop health.
4. Nutrient Optimization
By analyzing patterns in nutrient uptake, AI can guide the rotation of legumes and other soil-enriching crops to maintain or improve soil fertility while minimizing external fertilizer use.
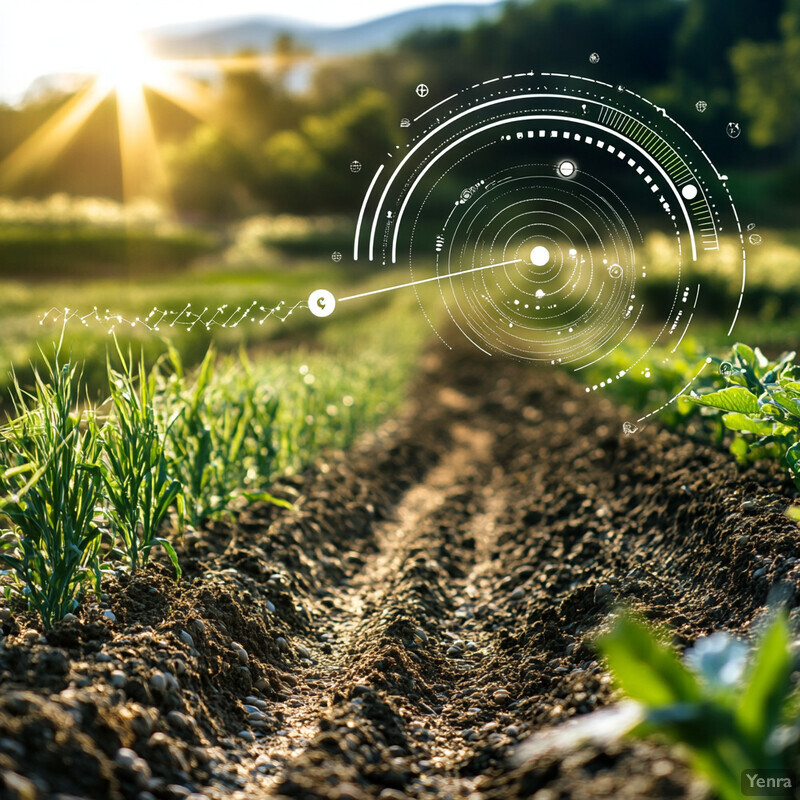
Crops differ in the nutrients they extract and return to the soil, and AI systems can map these relationships with remarkable detail. By understanding how certain rotations, such as including nitrogen-fixing legumes before nutrient-demanding cereals, can restore soil fertility, farmers can reduce synthetic fertilizer usage and costs. AI-driven nutrient models can suggest the ideal sequence to replenish depleted elements, balance nutrient ratios, and maintain soil vitality. As a result, farmers are guided towards rotations that naturally recycle nutrients, improve plant health, and minimize pollution from runoff.
5. Climate-resilient Planning
Machine learning algorithms simulate how different crop sequences will perform under various future climate scenarios, helping farmers select rotations that are more resilient to droughts, floods, or temperature extremes.
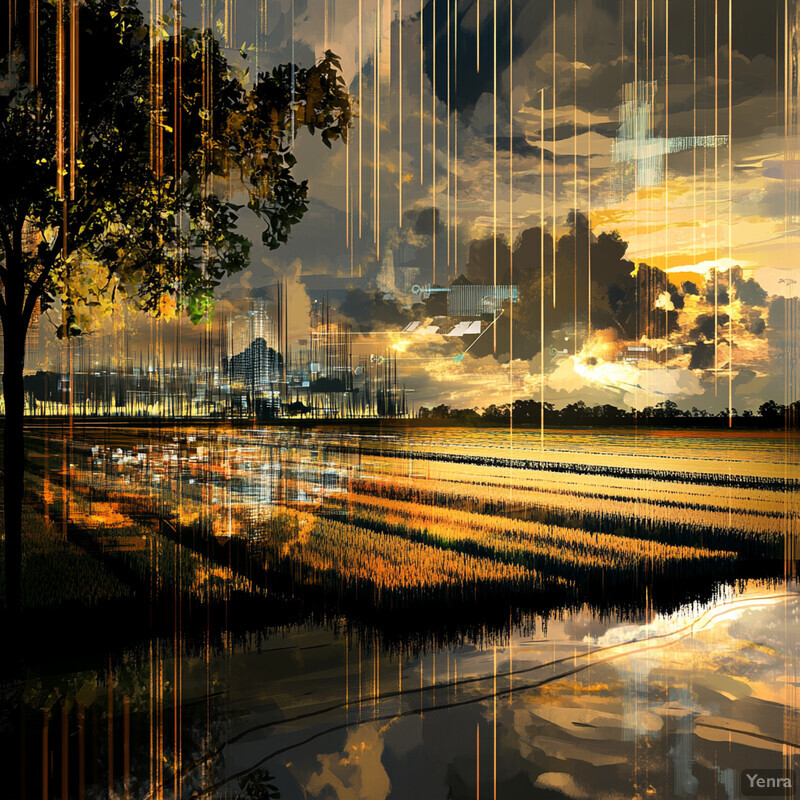
Climate change introduces uncertainties such as erratic rainfall, temperature extremes, and shifting growing zones. AI models address these uncertainties by running simulations under multiple climate scenarios—drought, intense rainfall, or extended heatwaves—and identifying rotations that yield stable results under such conditions. These analyses highlight crops that can withstand stress or rotations that mitigate the impact of climate variability, allowing farmers to future-proof their operations. The outcome is a rotation strategy that builds long-term resilience against weather extremes and secures consistent productivity.
6. Real-time Remote Sensing Integration
AI-driven tools leverage satellite imagery and drone data to track crop health indicators (e.g., leaf chlorophyll content, canopy cover) and adjust rotation plans on the fly based on early indicators of stress or disease.
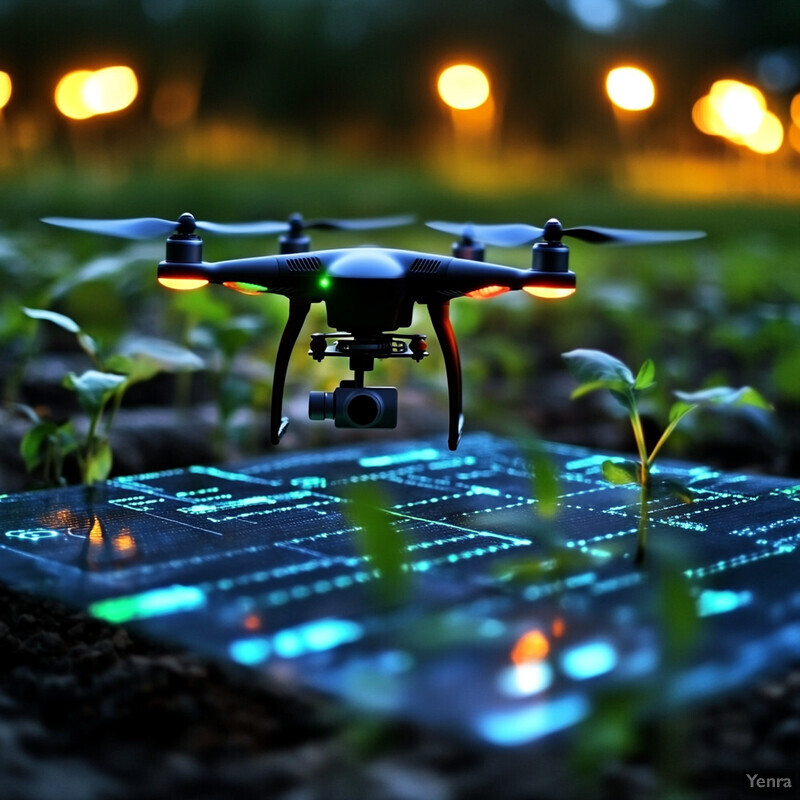
Satellite imagery, drone scans, and in-field IoT devices offer a stream of data on crop health indicators like leaf area index, canopy temperature, and chlorophyll content. AI tools interpret this real-time data, detecting early signs of stress, disease, or nutrient deficiencies. By understanding how each crop responds to current conditions, the AI can adjust rotation plans mid-season if necessary. For example, if a current crop exhibits early symptoms of a nutrient deficiency, the system might recommend a rotation that restores soil balance in the following season. This dynamic, data-driven decision-making minimizes yield losses and optimizes the rotation schedule continuously.
7. Precision Irrigation Scheduling
By linking rotation plans to water needs, AI can optimize irrigation schedules for each crop sequence, ensuring that water resources are used efficiently, even as climatic conditions change.
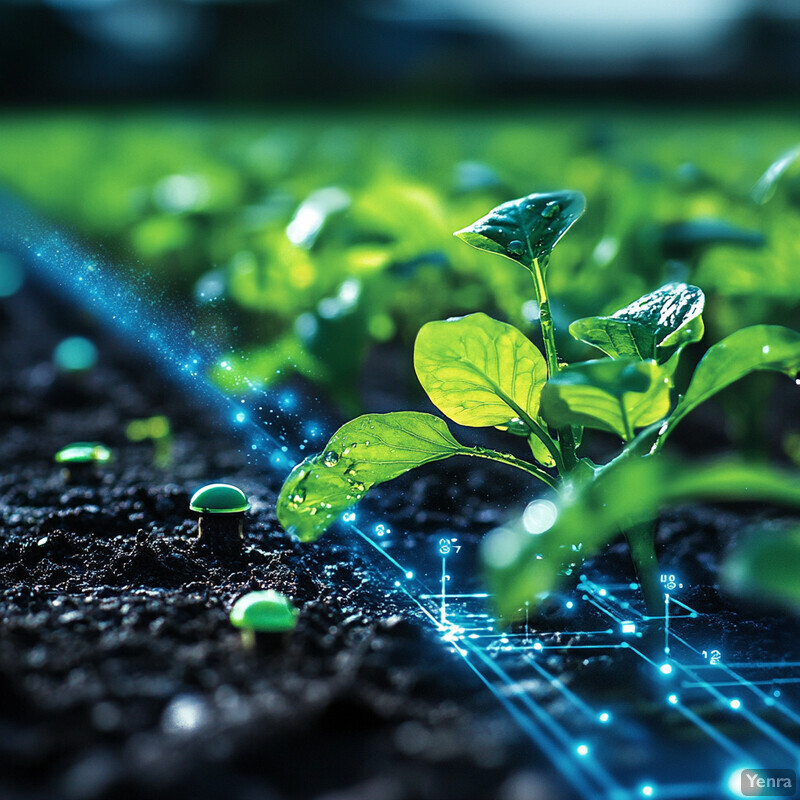
Different crops have unique water requirements, and their demands vary throughout the rotation cycle. AI models use weather forecasts, soil moisture sensors, and evapotranspiration rates to calculate precise irrigation needs. By linking these models to crop rotation plans, farmers can schedule watering more strategically, ensuring each crop receives just enough water at the right growth stage. This approach conserves water resources, reduces energy use, prevents nutrient leaching, and ensures healthy plant development. In turn, rotations become more efficient, contributing to both cost savings and environmental stewardship.
8. Weed Management Strategies
AI models consider different crop rotation patterns’ effects on weed species dominance, suggesting rotation plans that naturally suppress weeds and reduce herbicide dependence.
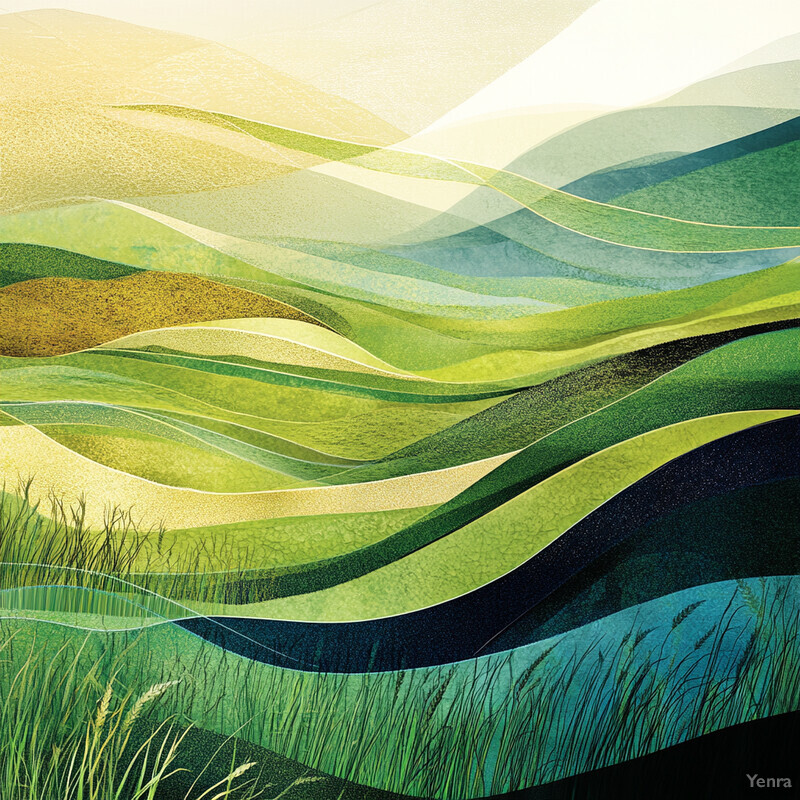
Weed species adapt to consistent cropping patterns, flourishing when the same crops are grown repeatedly. AI-driven analysis of weed ecology can pinpoint rotations that disrupt weed life cycles, limit seed bank accumulation, and minimize the need for herbicides. By introducing crops with different planting times, root structures, or canopy architectures, farmers can create conditions unfavorable for dominant weed species. Over time, these AI-informed rotations foster a more balanced agro-ecosystem, maintaining yields while curbing pesticide reliance and mitigating resistance development in weed populations.
9. Market Trend Forecasting
Advanced predictive analytics help farmers anticipate commodity prices and consumer demand, incorporating these factors into rotation decisions to balance agronomic goals with financial profitability.
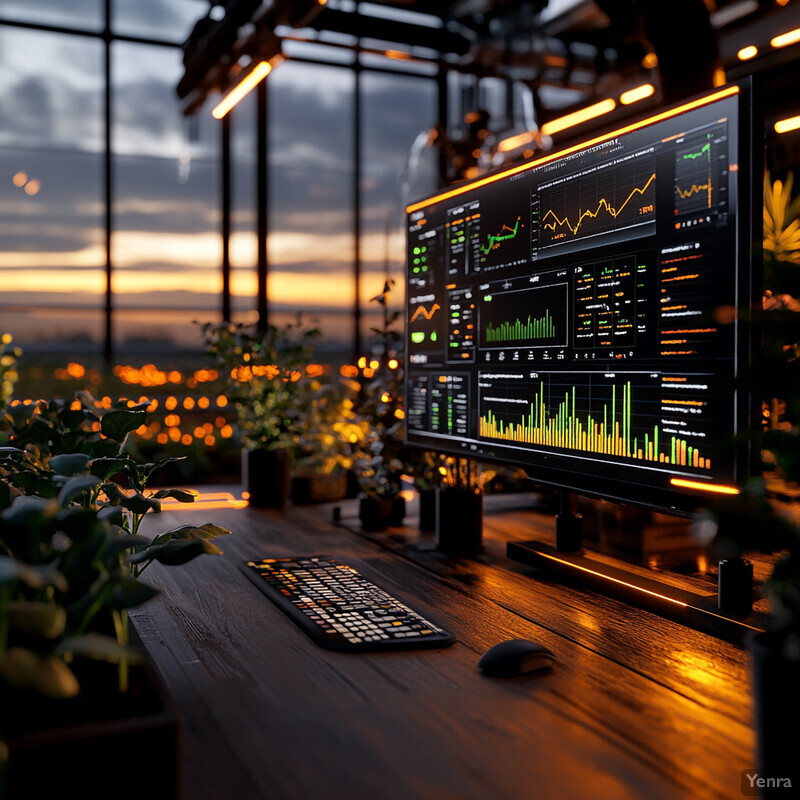
Agricultural markets are influenced by a plethora of factors: consumer preferences, global supply chains, geopolitical events, and economic cycles. AI systems can monitor these factors in real-time, predicting shifts in commodity prices and identifying high-value opportunities. By integrating these market projections into rotation planning, farmers can choose sequences that align agronomic viability with profitability. For instance, if emerging markets favor certain grains or pulses, farmers can rotate towards these high-demand crops. The result is an informed strategy that optimizes both yield and income.
10. Carbon Sequestration Optimization
AI tools can forecast how different crop rotations influence carbon sequestration rates in the soil, enabling farmers to choose rotations that bolster their environmental sustainability credentials.
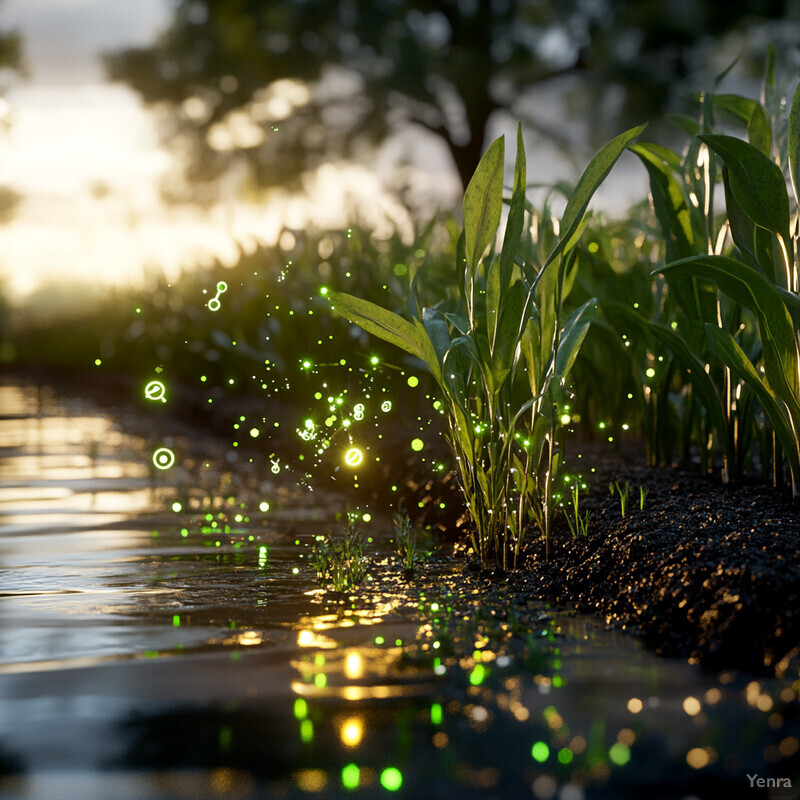
As sustainability and carbon footprint reduction become top priorities, AI can help farmers design rotations that maximize carbon storage in soils. By comparing the root systems, residue decomposition rates, and soil-cover qualities of different crops, AI highlights sequences that increase organic matter accumulation. Over time, these rotations help sequester more carbon, improving soil health, enhancing water retention, and contributing to climate change mitigation efforts. This approach aligns agricultural practices with environmental stewardship, meeting both regulatory and consumer expectations for sustainability.
11. Multi-objective Optimization
AI systems can simultaneously evaluate a range of objectives—yield stability, soil health, economic return, environmental impact—and recommend rotation sequences that best meet a farmer’s holistic goals.
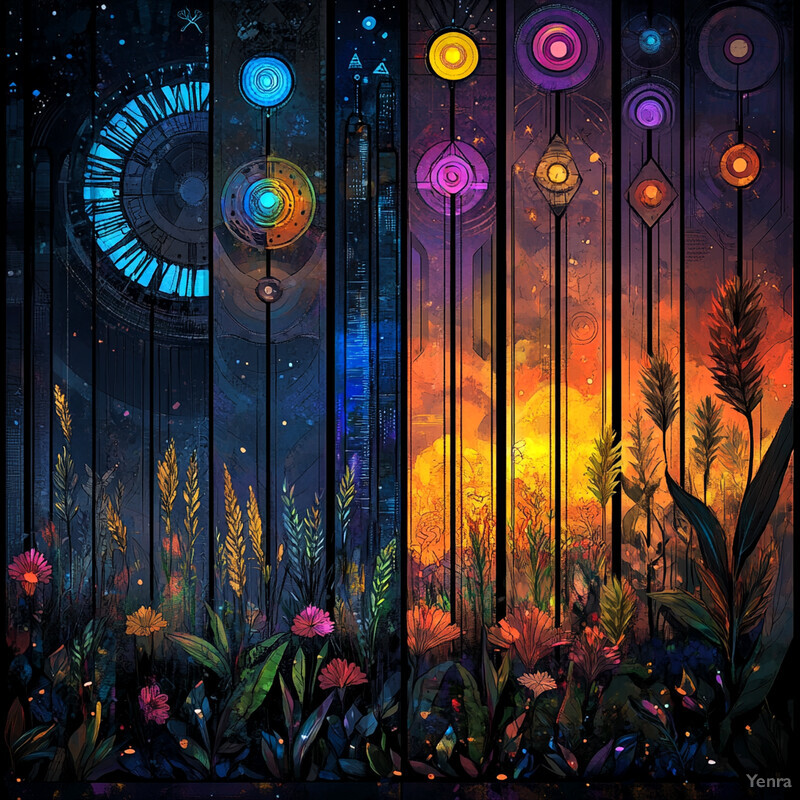
Farmers often juggle multiple goals—maximizing yield, minimizing environmental impact, optimizing input use, and ensuring long-term soil health. AI excels at handling such complexity by simultaneously analyzing numerous variables. These systems use advanced algorithms to weigh competing objectives and suggest rotations that strike the best possible balance. Such holistic planning helps farmers find “win-win” scenarios, where improving one aspect of their operation does not unduly sacrifice another, leading to more stable, profitable, and ecologically sound farming practices.
12. Disease-resistant Cultivar Selection
By analyzing genomic and phenotypic data, AI can suggest which crop cultivars, rotated properly, may reduce disease risk and sustain plant health over multiple seasons.
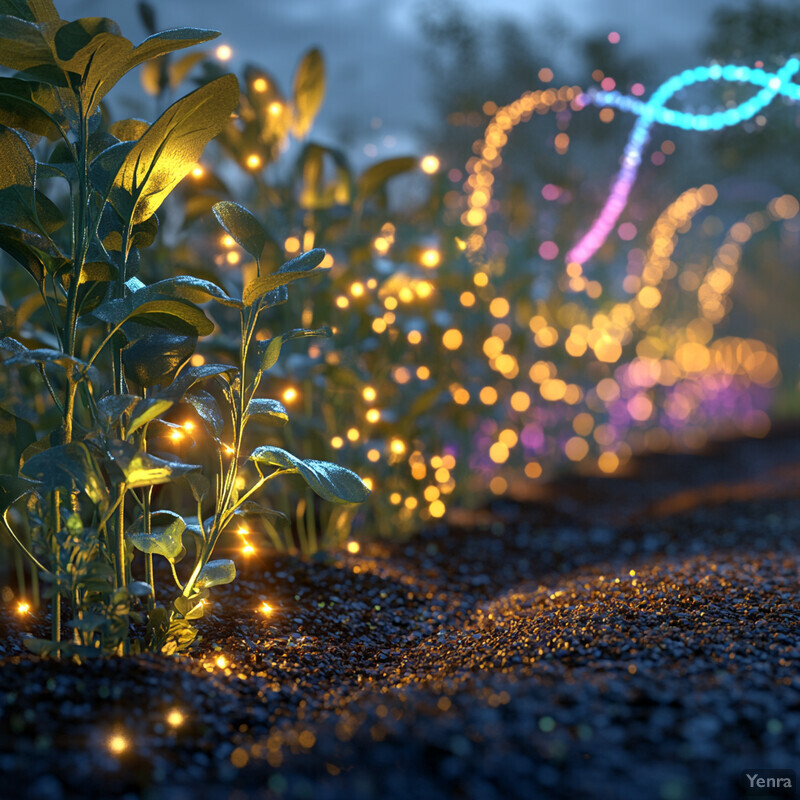
Crop susceptibility to diseases can vary greatly between cultivars. AI models that incorporate genomic data, plant breeding records, and on-farm performance can identify which cultivars thrive under certain rotations. By selecting the right cultivar for each crop in the sequence, farmers reduce the incidence of disease and lower their dependence on chemical controls. Over time, these informed choices lead to more robust rotation systems that continuously improve as new cultivars become available, ensuring ongoing adaptation to evolving pathogens.
13. Integrated Cropping-Livestock Systems
AI models help blend crops that support livestock feed production in a rotation plan, improving farm self-sufficiency and reducing reliance on external feed sources.
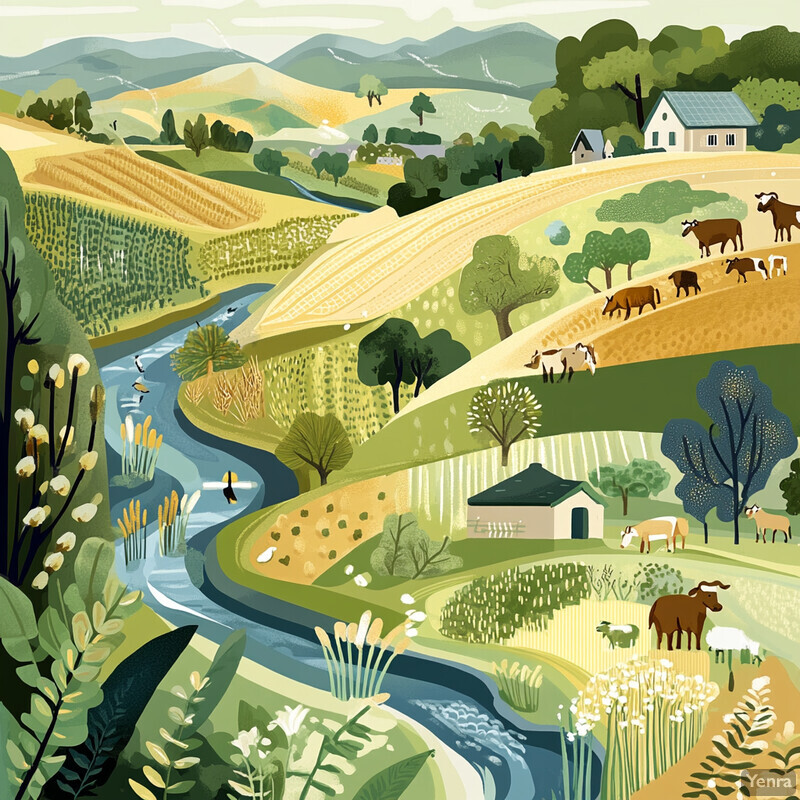
Many farms incorporate both crop and livestock production. AI tools can help integrate forage and feed crops into rotations that sustain animal nutrition while improving soil health. By analyzing manure inputs, crop residue utilization, and rotational grazing schedules, these models ensure that the system runs efficiently as a whole. Such integration creates synergies: manure enriches the soil for crop rotations, and diverse crop cycles provide reliable feed sources. This approach reduces reliance on purchased feed, cuts costs, and enhances the sustainability and resilience of mixed farming systems.
14. Resource Allocation Planning
By projecting labor, equipment usage, and input requirements for various rotation scenarios, AI helps optimize resource allocation and reduce operational costs.
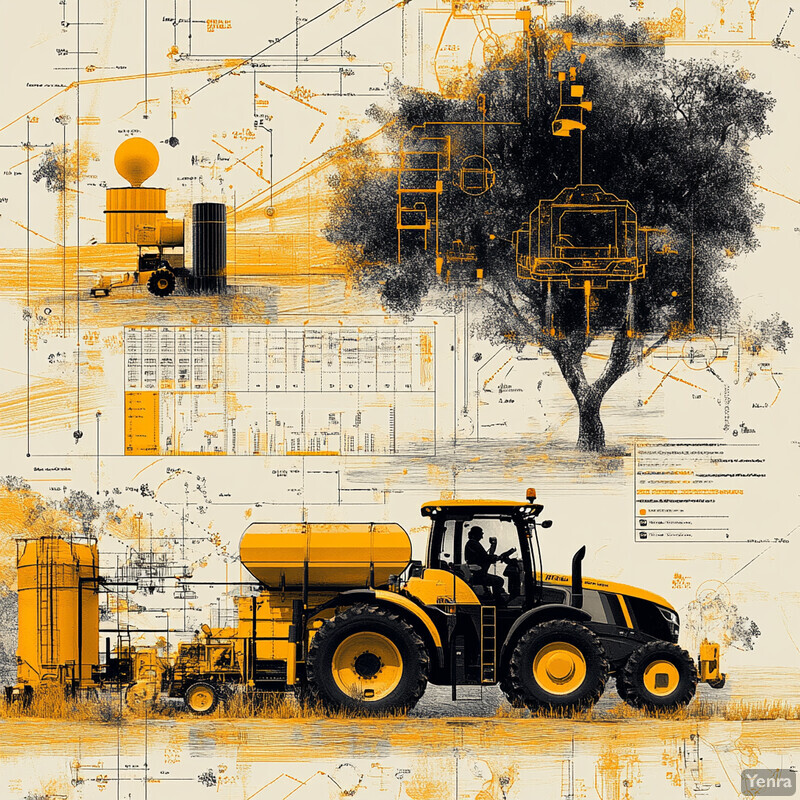
Beyond agronomic factors, farm operations involve machinery scheduling, labor distribution, and financial budgeting. AI can model how various rotations influence labor demand peaks, equipment usage, and input deliveries. By finding sequences that spread out workloads and capitalize on equipment efficiencies, AI-driven planning lowers operational costs and streamlines management. Farmers end up with rotation plans that not only improve the land but also ensure smoother, more profitable day-to-day operations.
15. Long-term Soil Erosion Prevention
AI-based simulations assess how rotating certain soil-anchoring crops can reduce erosion over time, guiding farmers toward rotations that maintain land quality.
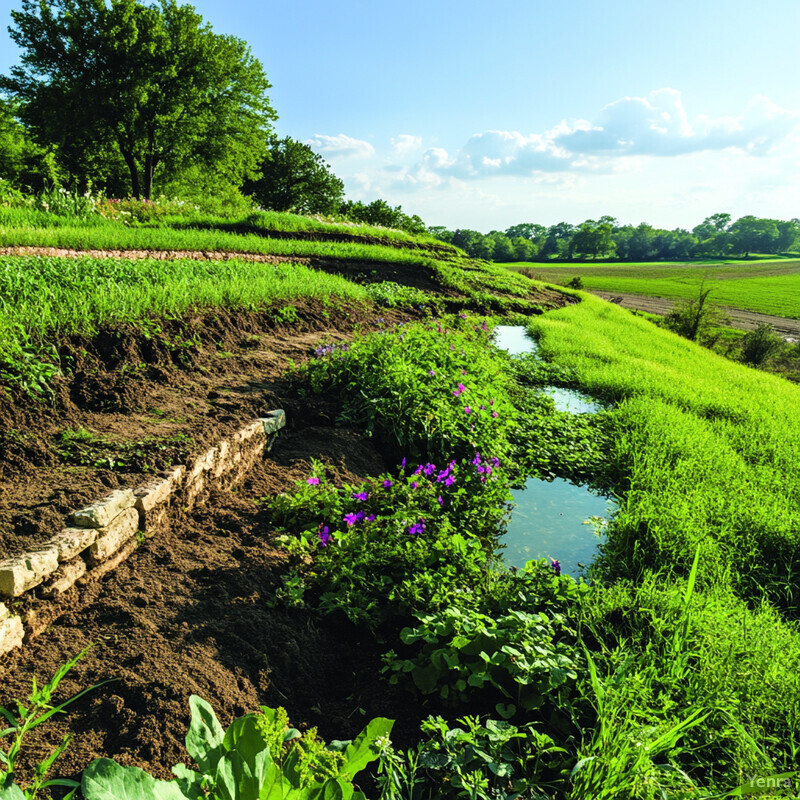
Crop rotation can play a critical role in preventing soil erosion by maintaining vegetation cover, improving soil structure, and increasing organic matter. AI systems analyze topographic data, rainfall patterns, and crop root characteristics to suggest rotations that protect against wind and water erosion over the long term. This ensures that the farmland remains productive and the broader environment benefits from reduced sediment run-off into waterways. Over multiple cycles, these soil-conserving rotations help maintain productive land assets for future generations.
16. Risk Mitigation under Uncertainty
Machine learning approaches can incorporate weather volatility, disease emergence, and market fluctuations to design robust rotation strategies that minimize downside risks.
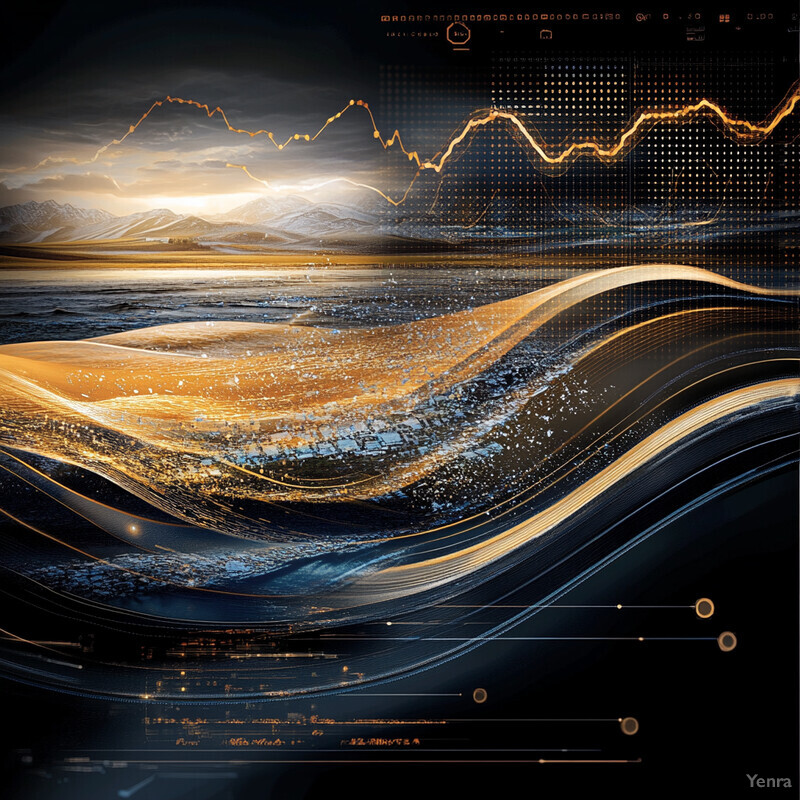
Agriculture is inherently uncertain, with volatility arising from weather, pests, markets, and more. AI models embrace this uncertainty, using probabilistic methods and scenario planning to recommend rotations that minimize downside risks. By examining “what-if” situations—such as unexpected droughts or price collapses—these systems guide farmers toward robust strategies that hold up under diverse conditions. In doing so, rotations become not just a production practice, but a risk management tool that improves the farm’s overall resilience and financial stability.
17. Continuous Improvement via Feedback Loops
As farmers implement AI-recommended rotations, performance data feeds back into the model, continually refining suggestions and improving system accuracy.
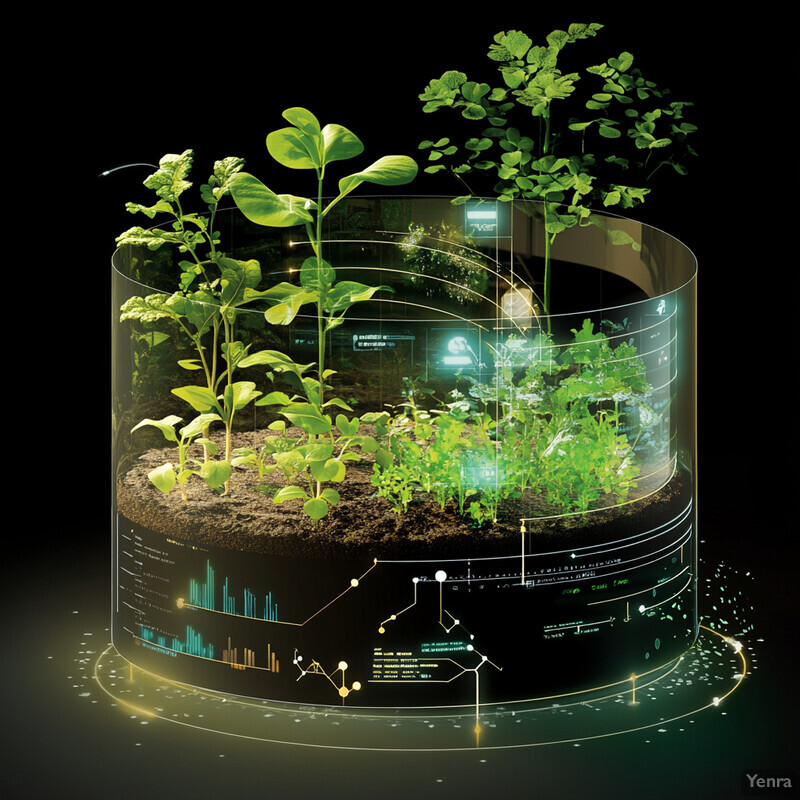
AI systems grow smarter over time as they ingest new data from field trials, sensor updates, and yield records. Every season’s outcomes feed back into the models, refining predictions and recommendations for the next cycle. This iterative learning process leads to continuous improvements in rotation planning. Farmers benefit from real-time insights that reflect the most current conditions, and the models steadily gain accuracy, becoming indispensable tools for long-term farm management.
18. Sustainable Nutrient Cycle Management
AI-based nutrient flow models identify how rotating legumes, cover crops, and cash crops can recycle nitrogen and other nutrients effectively, reducing reliance on synthetic inputs.
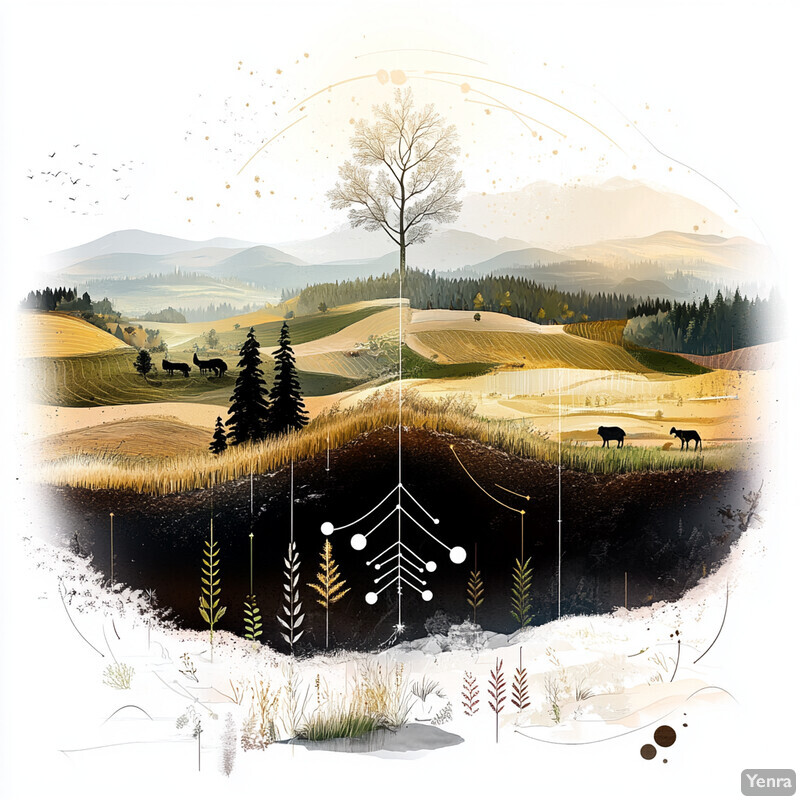
Nutrient cycling is at the heart of soil health and crop productivity. AI-based nutrient flow models track how nitrogen, phosphorus, and other key elements move through the system, identifying bottlenecks or imbalances. By suggesting rotations that introduce cover crops to fix nitrogen or cereals that efficiently utilize soil nutrients, AI helps maintain a self-sustaining nutrient cycle. Farmers thus reduce their reliance on synthetic fertilizers, lowering both costs and environmental impacts, while maintaining or improving yield quality and quantity.
19. Localized Hyper-customized Planning
Region-specific AI models factor in microclimatic data, soil types, local pests, and water availability, resulting in tailored rotation recommendations unique to each field.
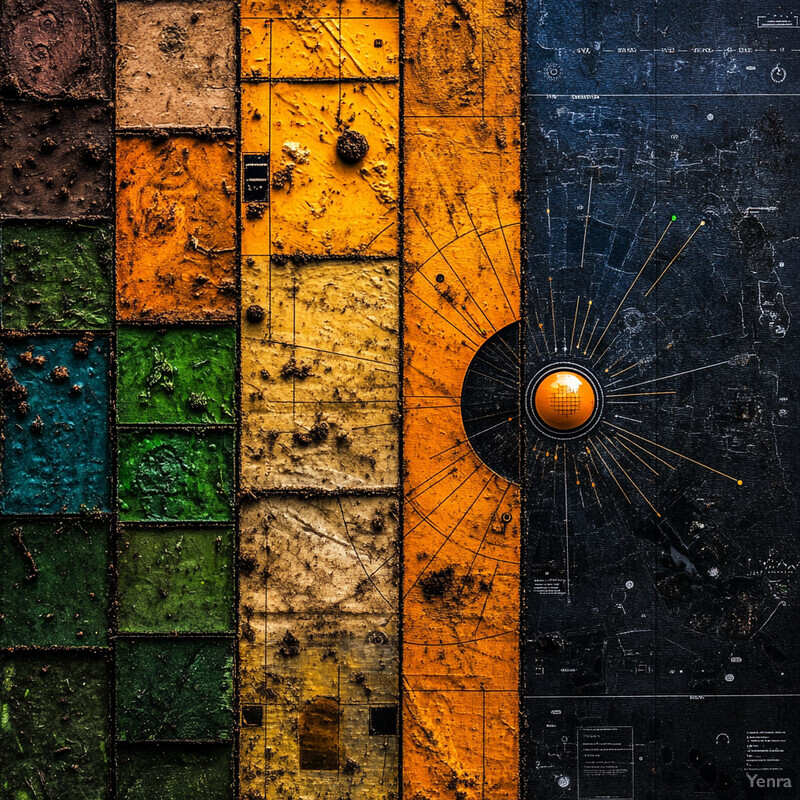
No two farms are identical. AI enables hyper-localized rotation recommendations by factoring in unique field conditions such as microclimates, slope gradients, soil textures, and pest pressures. Farmers receive rotation suggestions tailor-made for their specific parcels, ensuring the best use of available resources. This localized approach acknowledges that what works on one farm may not work next door, ultimately improving efficiency, productivity, and sustainability at a granular level.
20. Enhanced Training and Decision Support
Through user-friendly interfaces and decision dashboards, AI-powered crop rotation planning tools help farmers, agronomists, and advisors visualize complex data and implement sophisticated strategies more confidently.
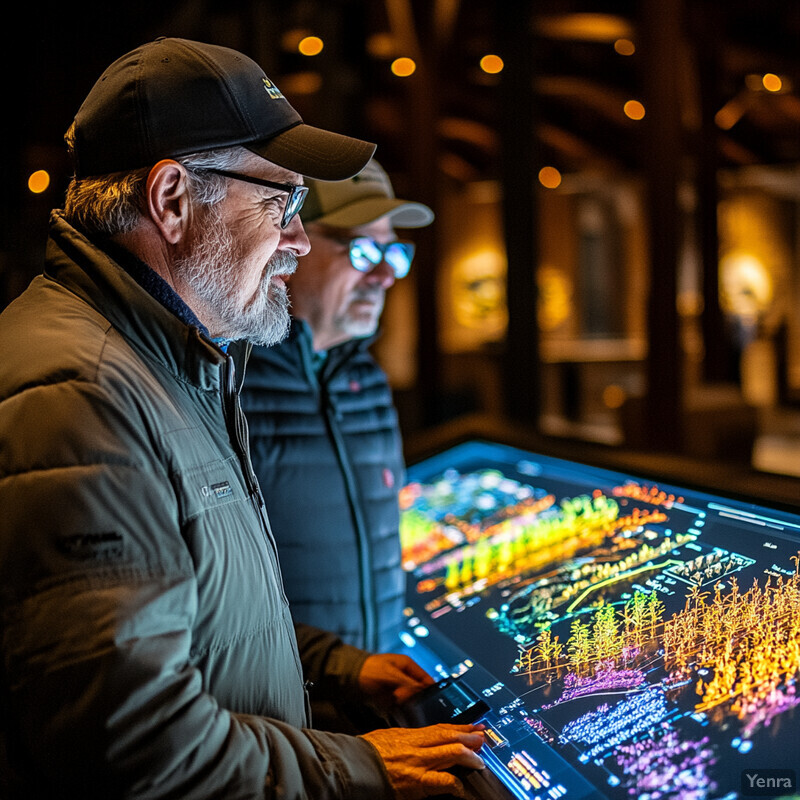
AI-powered platforms can present complex rotation recommendations through intuitive dashboards and visualization tools. Farmers, agronomists, and advisors can easily understand potential outcomes, weigh trade-offs, and select optimal rotations. By turning raw data and complex analytics into accessible insights, these tools empower users to make informed decisions. The result is a more educated farming community, armed with the knowledge and confidence to adopt innovative, AI-driven rotation strategies that lead to healthier soils, higher yields, and stronger farm resilience.