1. Intelligent Call Routing
AI-driven systems analyze customer data, intent, and historical interactions to direct customers to the most suitable agent or channel, reducing wait times and improving resolution quality.
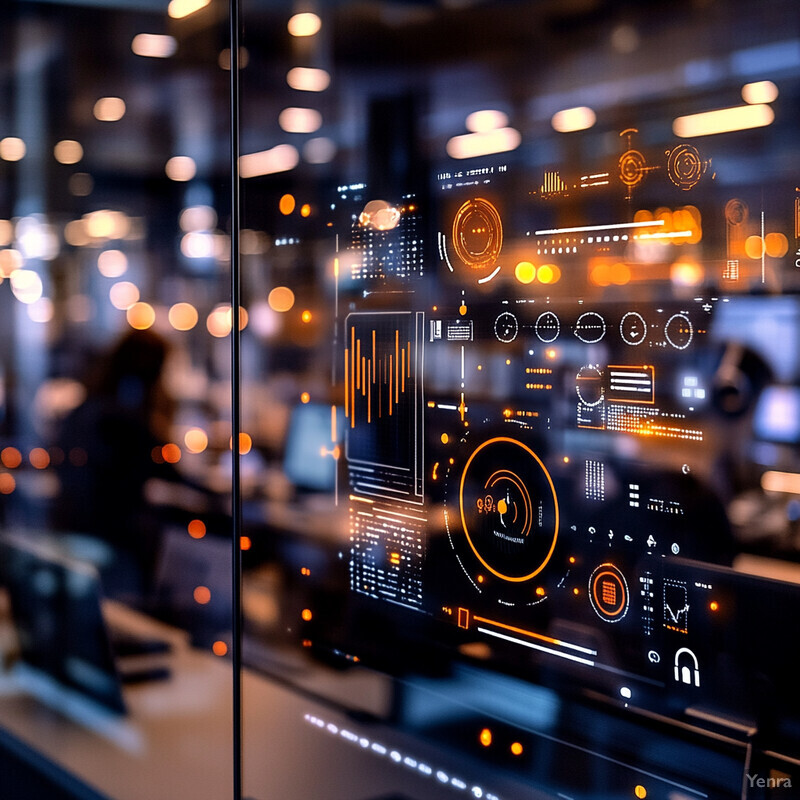
Traditionally, calls were routed based on simplistic rules such as language choice, region, or basic IVR selections. With the advent of AI, contact centers can route each call or message to the most appropriate agent by analyzing factors like the customer’s history, communication style, and the complexity of the query. Machine learning models continuously learn from past interactions to predict which agent is best suited—whether it’s someone with specialized technical knowledge or an agent who excels in empathetic customer care. This ensures that customers are connected more quickly and efficiently to someone who can help them effectively, thereby reducing wait times, improving first-call resolution (FCR) rates, and ultimately boosting overall customer satisfaction.
2. Predictive Call Volume Forecasting
Advanced machine learning models forecast contact volumes across channels, enabling more accurate staffing and scheduling for optimal workforce management.
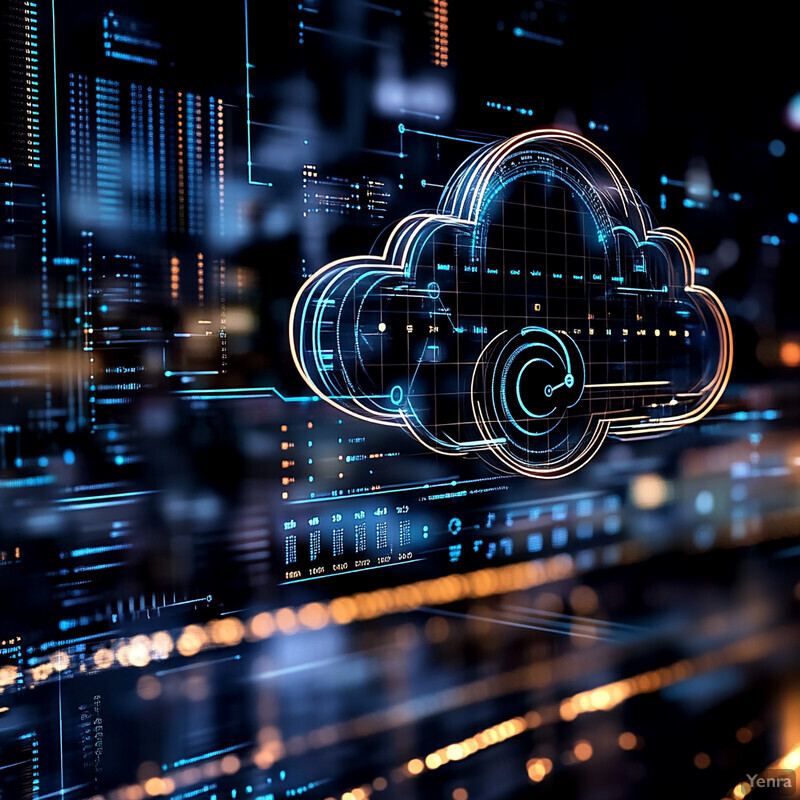
Long gone are the days of guesswork in staffing and scheduling. AI-driven forecasting tools analyze historical patterns, seasonal trends, promotional events, and even external data like weather or news cycles. By leveraging sophisticated time-series analysis and pattern recognition, contact centers gain precise predictions of inbound queries, enabling them to schedule the right number of agents at the right times. Accurate forecasting ensures consistent service quality, avoids agent underutilization, and prevents customer frustration due to long queues. This directly translates to better workforce optimization and more consistent adherence to service-level agreements (SLAs).
3. AI-Powered Chatbots and Virtual Assistants
Natural language understanding and dialogue management systems handle routine inquiries, provide instant responses, and escalate complex issues to human agents, freeing staff for higher-level tasks.
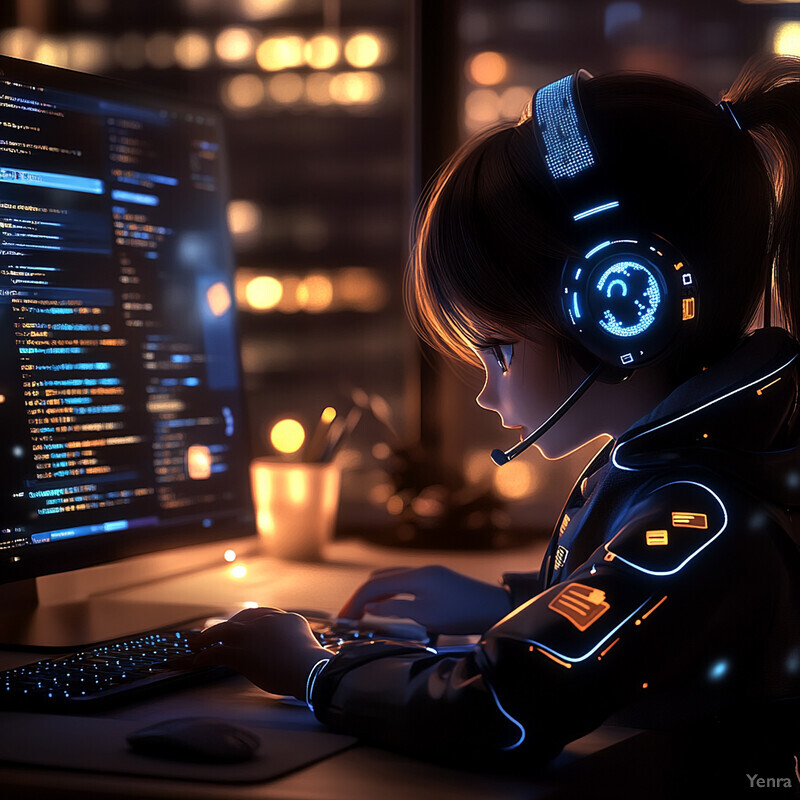
Modern contact centers leverage AI chatbots that can understand natural language, process user intent, and carry on context-aware conversations. Rather than static, menu-driven bots, these AI assistants learn from every customer interaction to provide more relevant answers, troubleshoot common issues, and even guide customers through complex processes step-by-step. By handling repetitive and straightforward inquiries—such as resetting passwords, tracking orders, or providing FAQs—these virtual assistants free human agents to focus on higher-value interactions. This balanced workload results in faster resolutions, reduced operational costs, and improved customer satisfaction.
4. Sentiment Analysis and Emotion Detection
AI models detect customer sentiment and tone in real-time, allowing agents to adjust their approach, improve empathy, and better manage sensitive or challenging conversations.
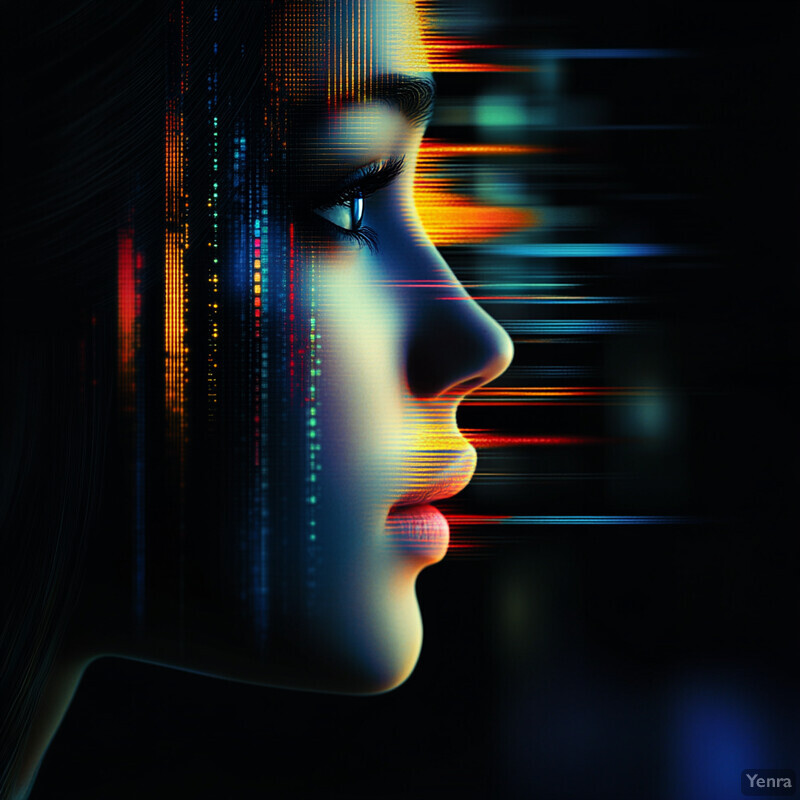
Beyond the words spoken or written, the emotional undertone of a conversation greatly impacts the customer experience. AI-powered sentiment analysis tools detect subtle cues—tone, speed of speech, word choice, and even silences—to gauge a customer’s emotional state. When agents are aware of a customer’s frustration, confusion, or satisfaction level in real time, they can adapt their responses to be more empathetic, reassuring, or proactive. This heightened emotional intelligence leads to more meaningful interactions, fosters trust, and enhances the likelihood of turning a negative experience into a positive one.
5. Speech Recognition and Transcription
Automated speech-to-text engines accurately transcribe calls, supporting real-time agent prompts, post-call analytics, and detailed quality assessments.
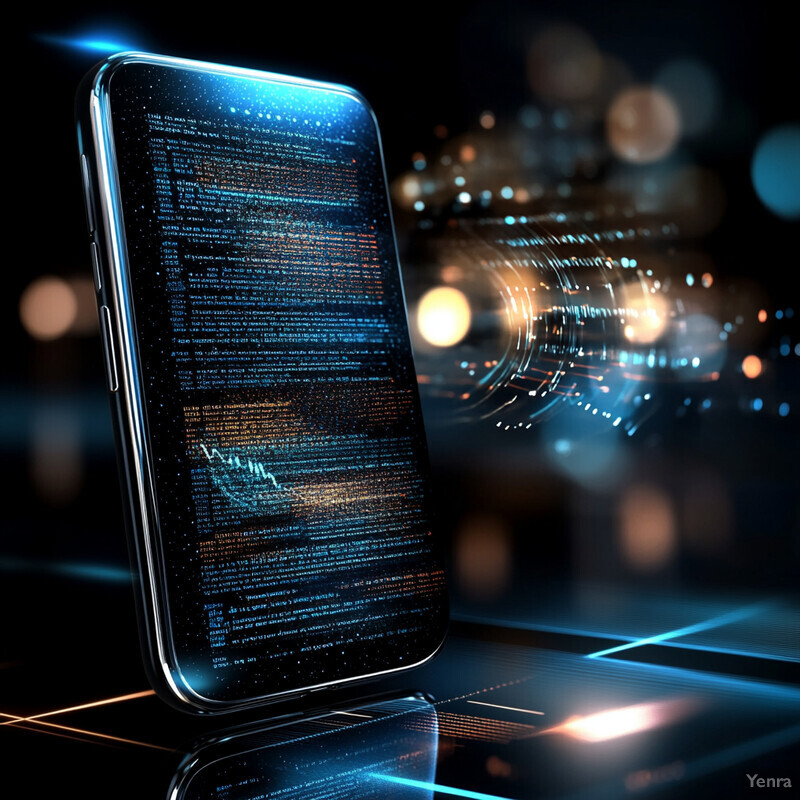
AI-driven speech recognition engines have evolved to transcribe customer calls into text with remarkable accuracy. These transcriptions are not only used post-interaction for analysis and compliance reviews but can also assist agents in real time by providing relevant information from the knowledge base during a call. Transcripts help ensure consistent quality by making it easier to review interactions, confirm whether agents followed guidelines, and identify areas needing improvement. They also enable streamlined documentation, reducing the administrative burden on agents and leaving them more time to focus on delivering great service.
6. Real-Time Agent Coaching
AI-driven agent assist tools provide on-the-fly suggestions, knowledge-base references, and compliance reminders during live interactions, helping agents resolve issues faster and more consistently.
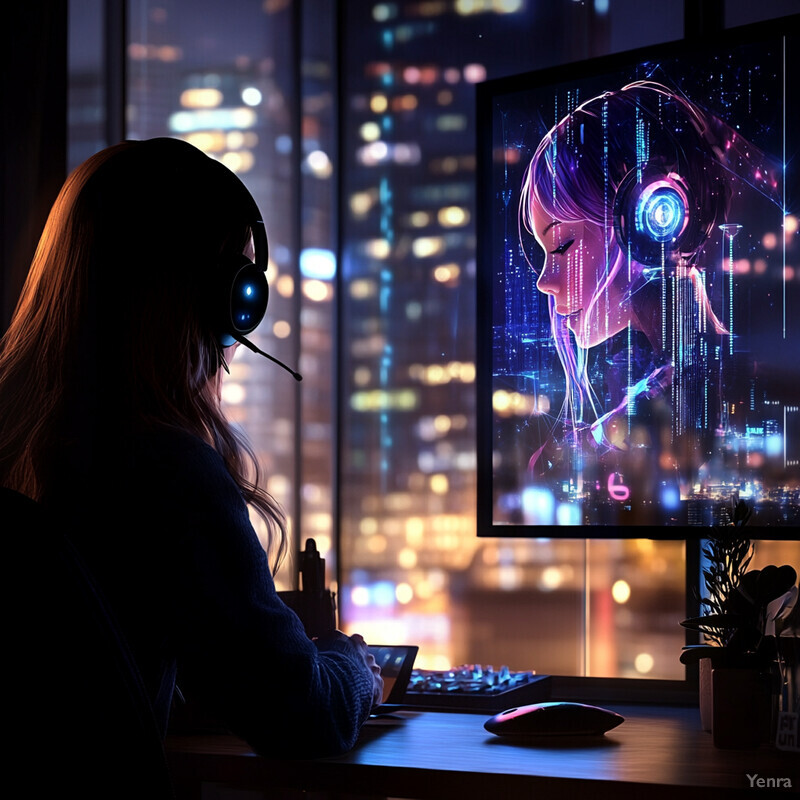
In the dynamic environment of a contact center, agents often need immediate guidance. AI-driven agent assist tools monitor ongoing conversations, identify key terms, compliance issues, or knowledge gaps, and prompt agents with relevant information instantaneously. For instance, if a customer mentions a new product issue, the system might push related troubleshooting steps or warranty information directly to the agent’s interface. This proactive support reduces resolution times, ensures compliance with policies, and helps even newer agents perform at a higher level, ultimately improving the consistency and quality of customer experiences.
7. Automated Quality Assurance (QA)
Machine learning algorithms evaluate 100% of interactions for compliance, script adherence, and customer satisfaction indicators, enabling continuous improvement at scale.
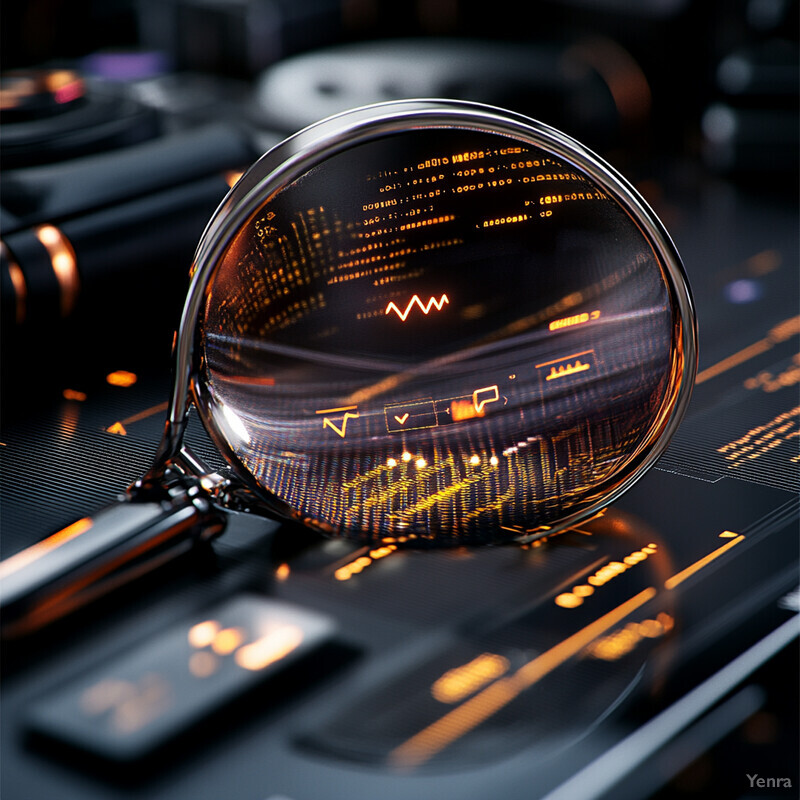
Whereas traditional QA involved manually sampling a small percentage of calls or chats, AI enables the evaluation of nearly every interaction at scale. Machine learning models detect compliance with scripts, adherence to regulatory requirements, and gauge the overall quality of the agent’s engagement. These tools highlight patterns of success and pinpoint common failure points, allowing supervisors to focus on coaching rather than tedious manual scoring. This comprehensive quality check ensures continuous improvement, as contact centers can quickly identify and correct systemic issues, ultimately improving performance across all agents.
8. Advanced Personalization
Predictive analytics leverage historical context, browsing behavior, and CRM data to tailor offers, solutions, and product recommendations to individual customers.
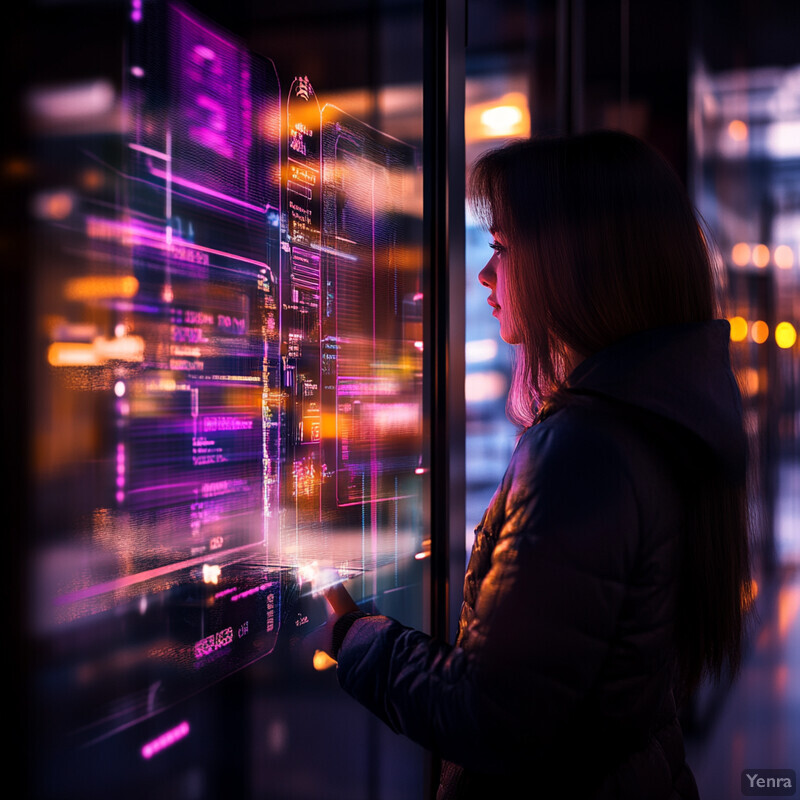
Modern customers expect personalized interactions. AI empowers agents and self-service systems to leverage customer histories, preferences, purchase behavior, and past interactions to deliver tailor-made solutions. For example, if a customer previously called about a billing issue, the AI can surface that context immediately, enabling the agent to pick up seamlessly where the last discussion ended. By presenting relevant offers, recommending complementary products, or recalling prior challenges, the customer feels recognized and valued, reinforcing loyalty and improving overall satisfaction.
9. Intelligent Self-Service
AI enhances IVR systems and self-service portals, making them more intuitive, context-aware, and capable of handling complex inquiries without human intervention.
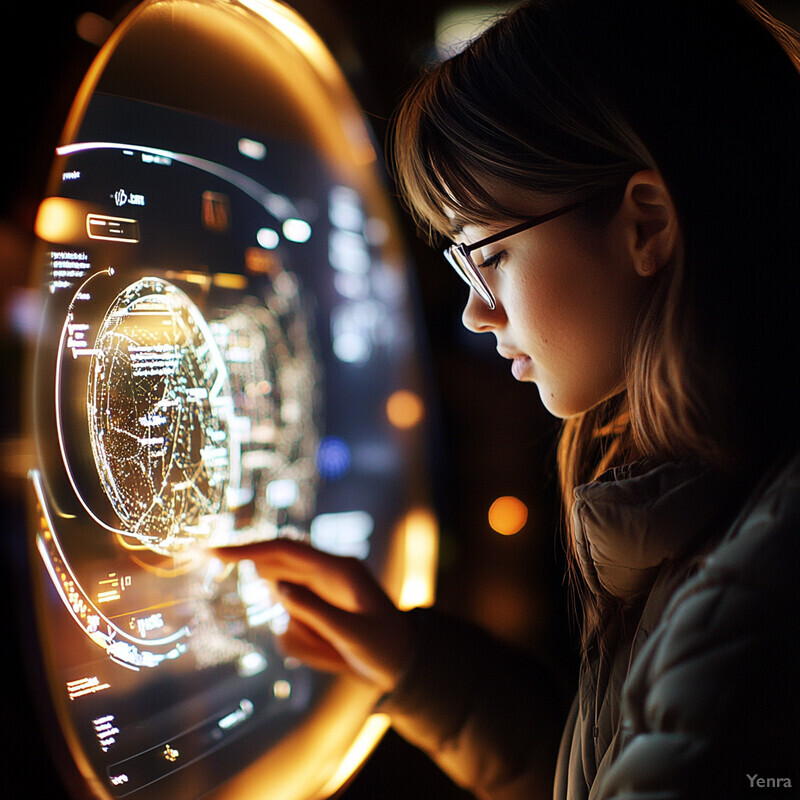
Sophisticated AI has transformed static IVR menus into intelligent self-service portals. Customers can express their problems in their own words, and the system’s natural language understanding capabilities guide them to the right answers, tutorials, or actions. Instead of a linear “Press 1 for billing” experience, users can say, “My last payment didn’t go through,” and the system will respond contextually. This improvement reduces customer effort, increases resolution speed, and cuts down on operational costs by deflecting simple queries from human agents—freeing them to focus on more complex issues.
10. Knowledge Base Optimization
AI-driven content curation and maintenance keep internal and customer-facing knowledge resources up-to-date, searchable, and contextually relevant to speed resolution.
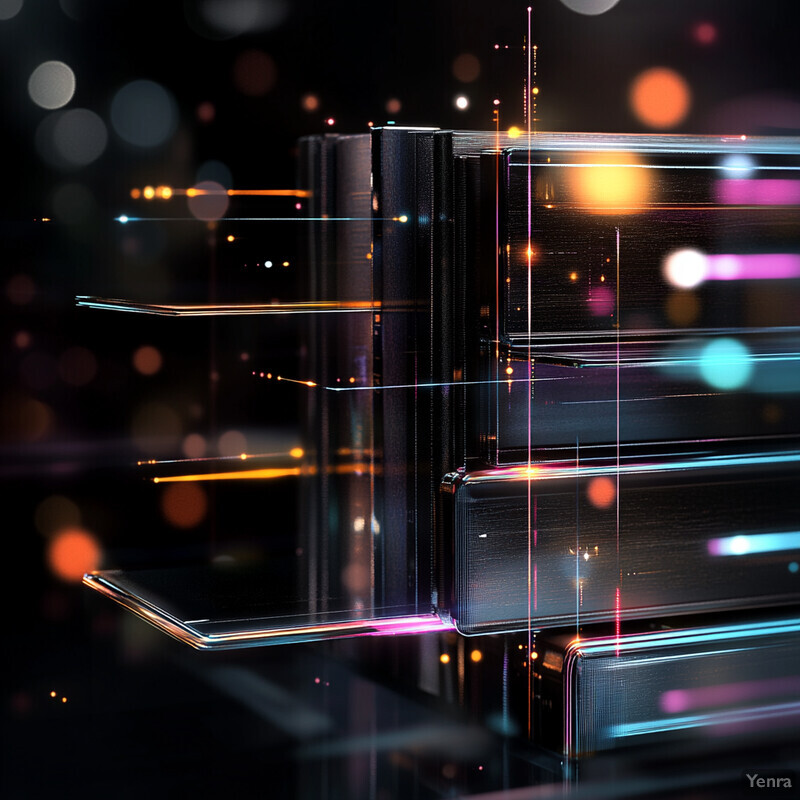
Contact centers rely on a robust knowledge base (KB) to help agents and customers find the correct information quickly. AI takes KB management to the next level by analyzing search patterns, user feedback, and resolution outcomes to continually refine and update content. These systems can suggest reorganizing articles, flag outdated information, and highlight gaps in the knowledge repository. By making sure the KB is always fresh, relevant, and accessible, agents spend less time hunting for information and more time providing efficient, accurate support, improving both agent performance and customer satisfaction.
11. Automated Post-Interaction Summaries
AI summarizes calls and chats into concise notes, saving agent time on administrative tasks and ensuring consistent documentation for future reference.
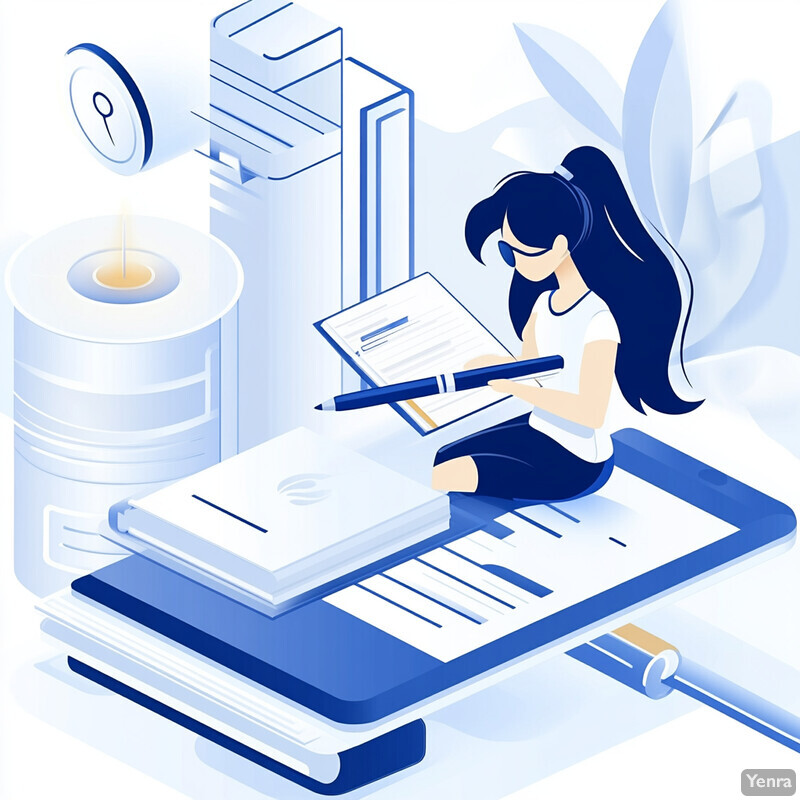
After each customer interaction, agents typically spend time creating summaries and notes for future reference. AI-driven summarization tools listen to or read the entire conversation and automatically generate concise, coherent summaries. This reduces repetitive administrative work, increases agent productivity, and maintains a high level of documentation quality. Managers and agents reviewing these summaries later can quickly grasp the gist of what transpired, making it easier to follow up, train new staff, or inform product and process improvements based on identified trends.
12. Proactive Customer Outreach
Predictive analytics and behavioral modeling identify when and why customers might need assistance, prompting proactive outreach that can resolve issues before they escalate.
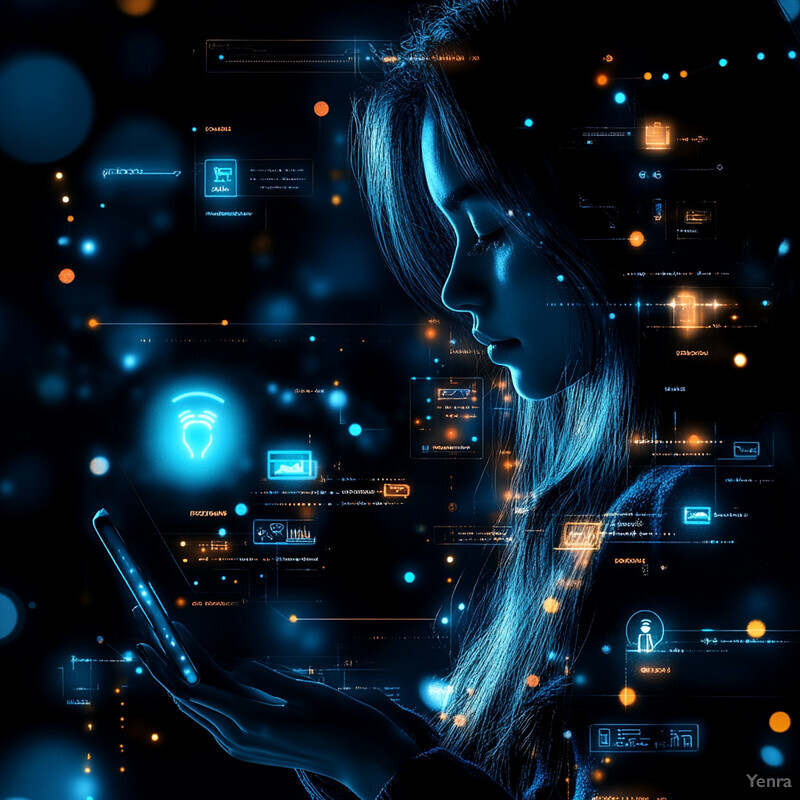
Rather than waiting for customers to contact the center with problems, AI can predict future needs or issues and initiate outreach. For example, if the system notices a pattern of device malfunctions after a recent firmware update, it can proactively message affected users with troubleshooting steps or arrange a callback. By resolving issues before they escalate, contact centers not only save time and resources but also boost customer satisfaction and trust. Proactive communication shows customers that the company understands their challenges and values their time.
13. Dynamic Script Optimization
Machine learning identifies which phrases, offers, and communication styles lead to the best outcomes, continuously refining agent scripts and conversation flows.
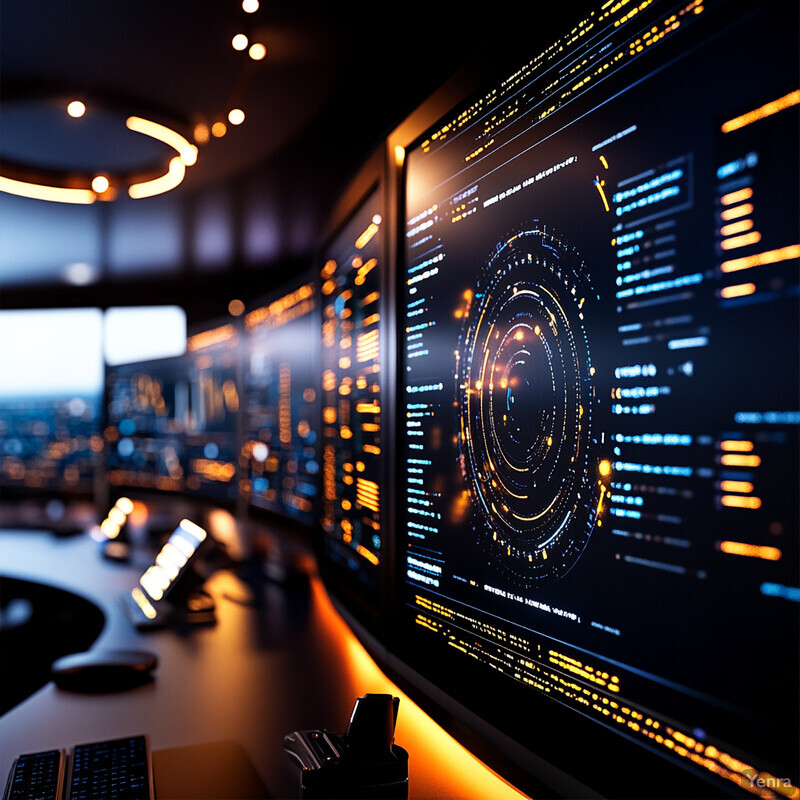
Agent scripts should not be static documents frozen in time. AI analyzes successful and unsuccessful conversations, identifying which phrases, order of presentation, or offers resonate best with customers. By constantly iterating and testing new approaches, these systems refine the dialogue flow to improve conversion rates, shorten call durations, and enhance overall customer engagement. Scripts become living documents, continuously adapting to evolving customer expectations and market conditions, ensuring that agents are always equipped with the most effective communication strategies.
14. Adaptive Learning for Agents
AI-powered training programs personalize learning modules for each agent based on their performance gaps, accelerating skill development and improving service delivery.
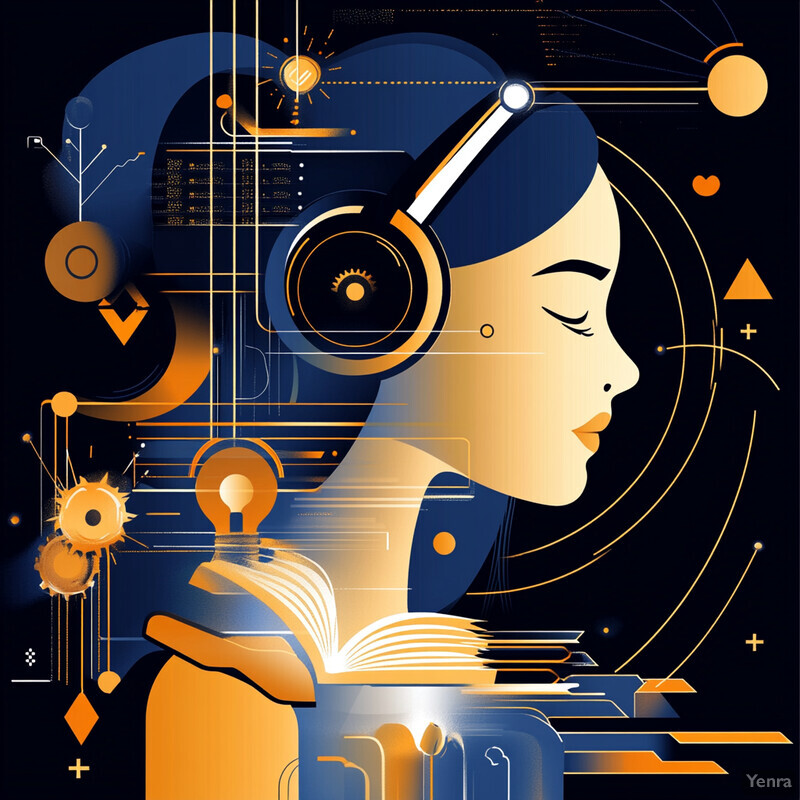
Every agent has unique strengths and weaknesses. AI-driven learning management systems personalize training modules and coaching sessions based on individual performance metrics, call outcomes, and knowledge gaps. Whether it’s improving technical troubleshooting skills, developing better negotiation tactics, or learning how to navigate a particular CRM system more efficiently, these tailor-made learning pathways ensure that agents can continuously improve. Over time, this investment in targeted skill development leads to better customer interactions, improved agent retention, and higher overall service quality.
15. Fraud Detection and Security
Voice biometrics and anomaly detection models flag suspicious activity, helping to safeguard customer data and maintain compliance with security standards.
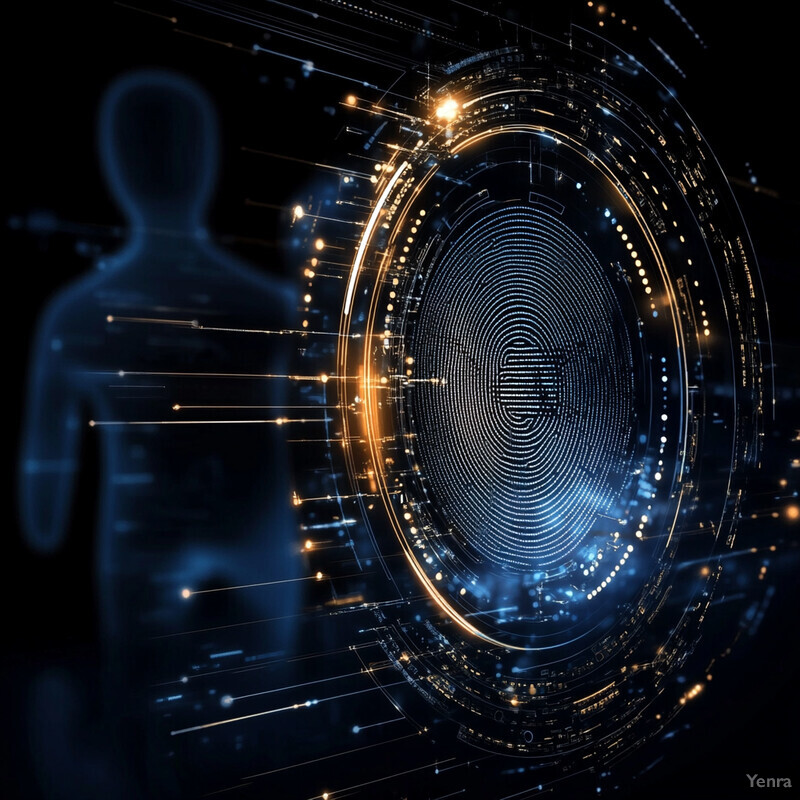
AI plays a critical role in securing customer data and transactions. Voice biometrics and machine learning models can authenticate callers by their voice patterns, flagging suspicious attempts from unauthorized individuals. Additionally, anomaly detection algorithms identify unusual account activity or interaction patterns, alerting agents and supervisors to potential fraud before any damage is done. This proactive approach to security helps maintain customer trust and ensures compliance with data protection regulations, as well as safeguarding the company’s reputation.
16. Multilingual Support
Natural language processing (NLP) models translate and handle inquiries in multiple languages, broadening the contact center’s reach and consistency in a global marketplace.
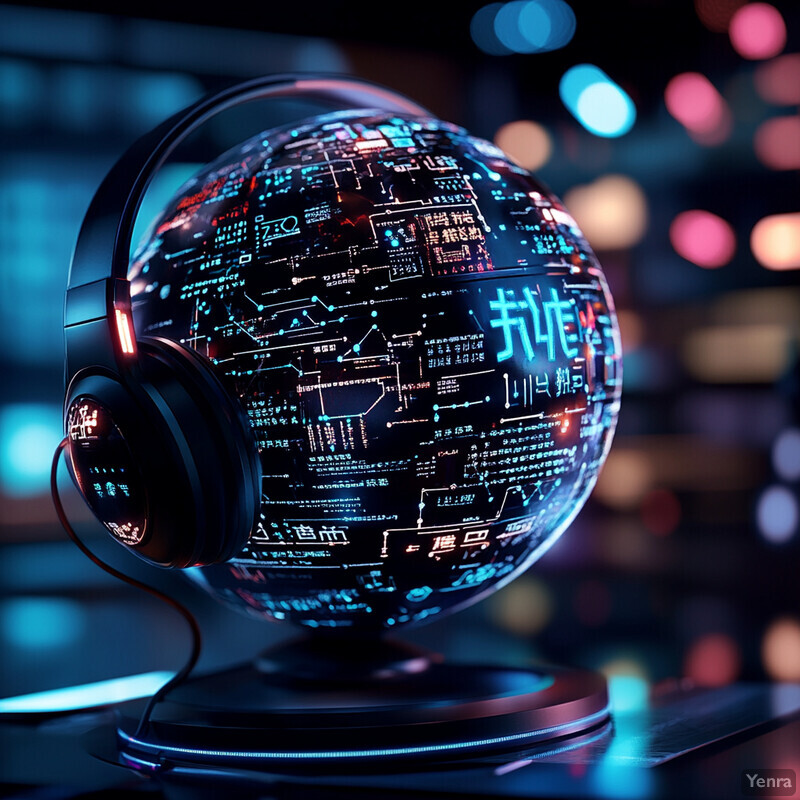
With a global customer base, supporting multiple languages is essential. Advanced NLP models can handle queries in various languages, translate messages in real-time, and ensure consistent quality of service worldwide. This reduces the need for maintaining large, specialized agent teams for each language. Instead, AI can serve as a linguistic bridge, making it more efficient to serve a broad, international customer population. The result is a more inclusive experience for non-native speakers and a stronger global presence for the brand.
17. Customer Journey Mapping and Analytics
AI integrates data from all touchpoints to reveal end-to-end customer journeys, identifying friction points and guiding optimization strategies.
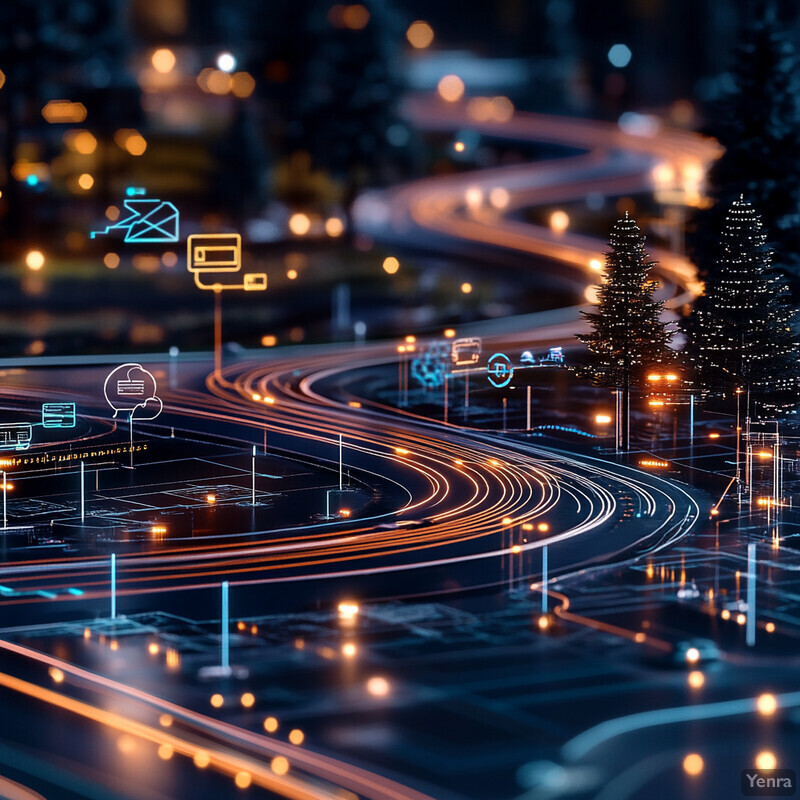
Customers often interact through multiple touchpoints—email, social media, live chat, and phone calls—before their issues are resolved. AI-driven analytics integrate and analyze data from all these channels to provide a cohesive view of the customer’s journey. By identifying common bottlenecks, drop-off points, and frequently asked questions, contact centers gain insights into where processes can be streamlined. Understanding the full lifecycle of a customer’s experience helps in optimizing not just the contact center operations but also product development, marketing strategies, and overall user experience.
18. Workforce Optimization and Capacity Planning
Predictive algorithms align staffing levels with anticipated customer demand and agent performance, balancing efficiency, cost, and service quality.
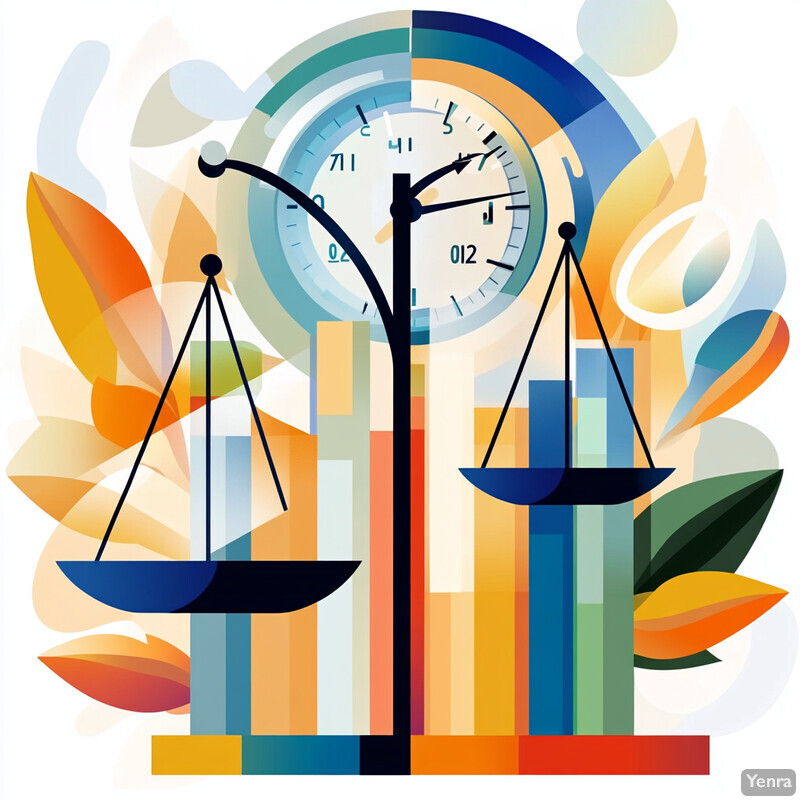
Determining how many agents to staff at any given time involves more than simple guesswork. AI-driven workforce management tools consider myriad variables, from forecasted volume and agent skill sets to planned vacations, training schedules, and expected wait times. Machine learning models learn from past experiences and adjust their projections dynamically. With accurate capacity planning, centers maintain consistent service levels without overstaffing, reduce operational costs, and keep employees more engaged by ensuring a balanced workload.
19. Service Level Agreement (SLA) Adherence
Intelligent systems monitor interaction patterns to ensure that response and resolution times stay within agreed-upon service parameters, alerting managers to potential SLA breaches.
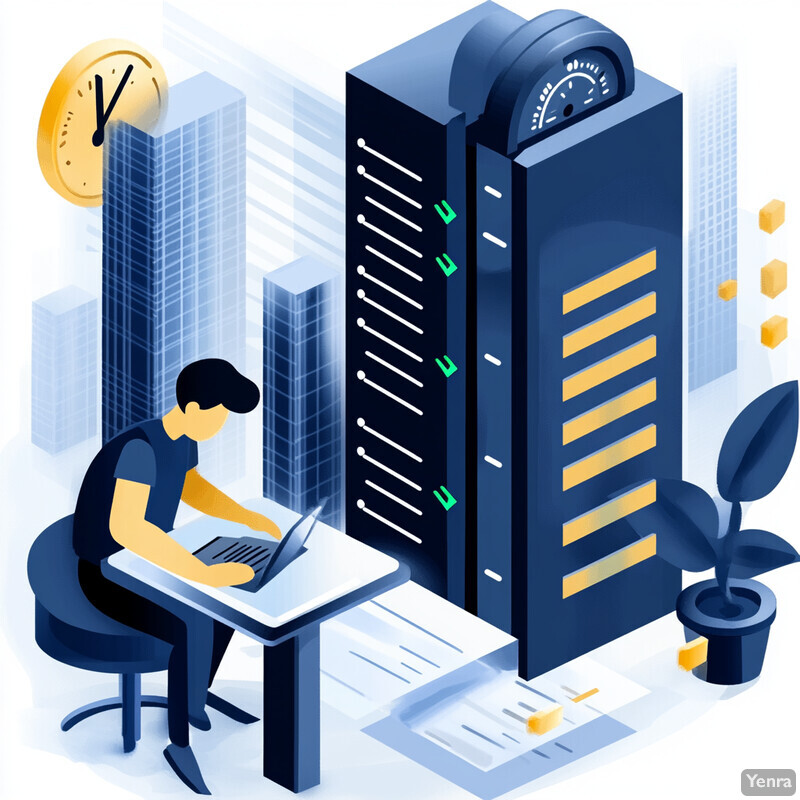
SLAs dictate turnaround times and quality standards that must be met. AI continuously monitors incoming and outgoing interactions, flagging potential delays, missed deadlines, or unresolved issues that risk breaching these agreements. By proactively alerting supervisors or automatically initiating recovery strategies—like escalating high-priority calls—AI ensures the contact center maintains performance metrics. This not only satisfies contractual obligations but also helps preserve strong client or partner relationships and the company’s reputation.
20. Cost Reduction and Revenue Enhancement
By streamlining workflows, minimizing repeat contacts, and delivering targeted upsell opportunities, AI-driven optimization helps reduce operational costs while boosting revenue.
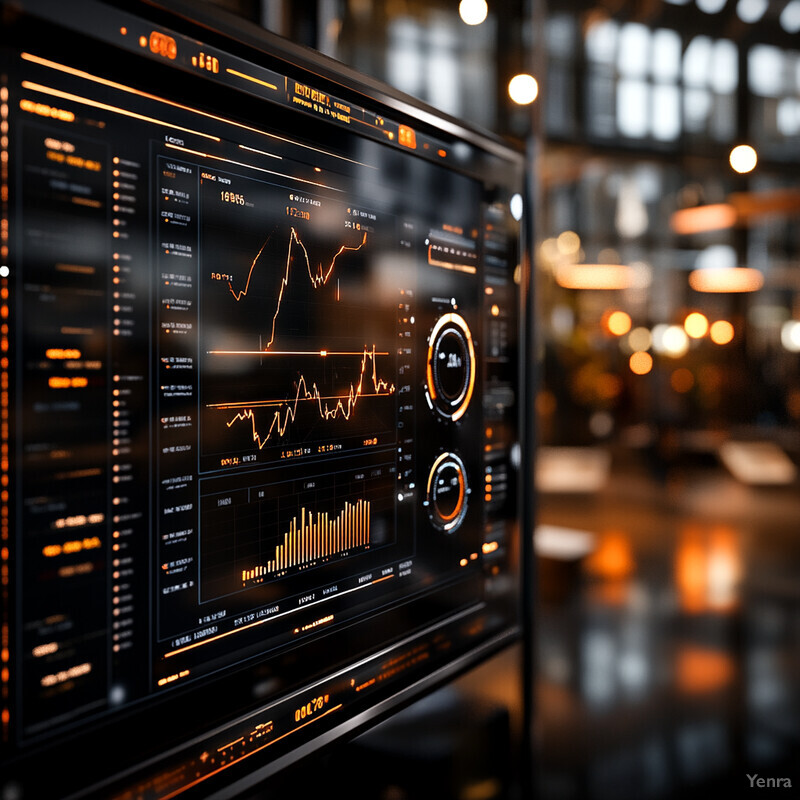
Ultimately, AI investments in the contact center drive tangible business results. By automating low-value tasks, improving agent efficiency, predicting and preventing issues, and personalizing customer experiences, organizations can significantly lower operational expenses. At the same time, providing better service and timely offers leads to increased customer loyalty, higher conversion rates, and more cross-selling opportunities. Over time, these optimized processes translate into a healthier bottom line, ensuring that the contact center doesn’t just serve customers well, but also supports the company’s long-term growth and profitability.