1. Enhanced Patient Recruitment and Enrollment
AI-driven analytics can rapidly screen large patient databases (electronic health records, registries, claims data) to identify suitable participants, reducing recruitment time and improving the likelihood of meeting inclusion criteria.
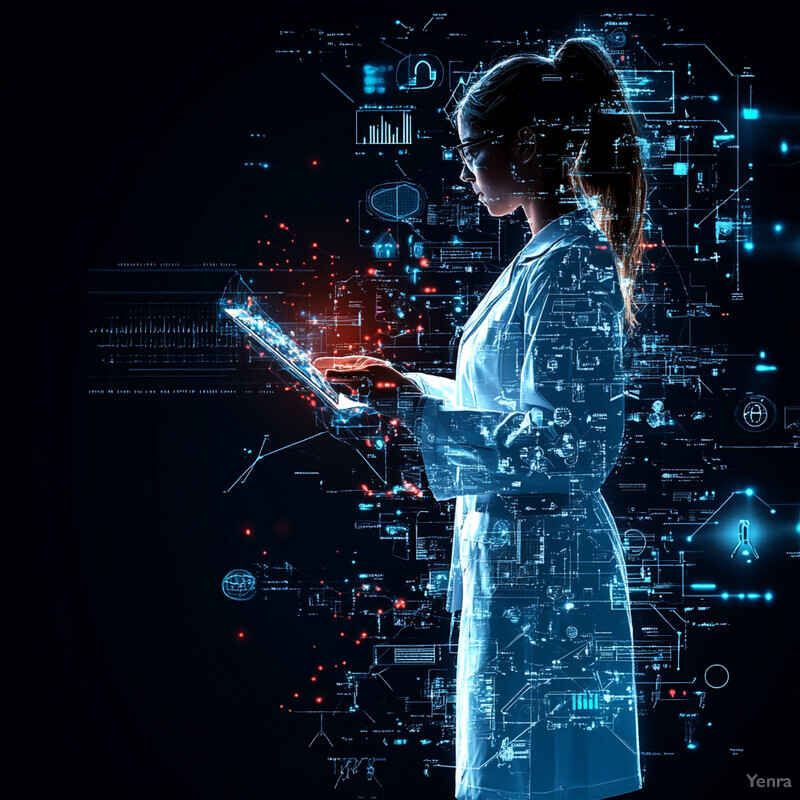
Recruiting suitable patients is often one of the longest and most costly steps in a clinical trial. AI-powered tools can scan large pools of patient data—such as electronic health records, claims data, and patient registries—to rapidly identify individuals who meet inclusion and exclusion criteria. By automating the initial screening process, AI helps sponsors avoid tedious manual reviews and ensures that potential participants are not overlooked. This approach leads to more precise and timely enrollment, reducing the risk of trial delays and increasing the likelihood that the study population accurately reflects the target patient demographic. Ultimately, faster and more targeted recruitment enhances the overall efficiency and quality of clinical trials.
2. Optimized Site Selection and Feasibility Studies
Machine learning algorithms analyze historical performance, patient demographics, and regulatory conditions to recommend ideal trial sites with high potential for enrollment and data quality.
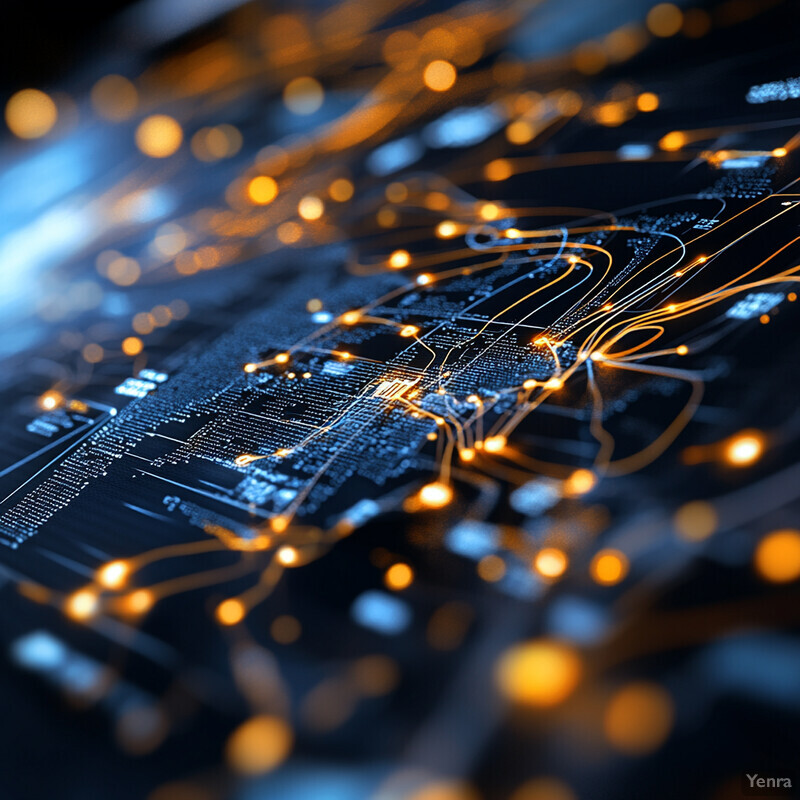
Selecting the right trial sites is critical for timely enrollment and reliable data. Traditional site selection often relies on investigators’ past performance and personal relationships. AI streamlines this by analyzing a wide range of data points, including site-level metrics, patient demographics, health outcomes, and geographic considerations. Machine learning algorithms can forecast which locations are most likely to enroll a sufficient number of participants, adhere to protocols, and ensure high data quality. By predicting site feasibility and performance, sponsors and CROs can focus resources on the most promising locations, reduce the number of underperforming sites, and potentially shorten overall trial timelines.
3. Predictive Dropout and Retention Modeling
Predictive models can identify patients at high risk of discontinuing participation.
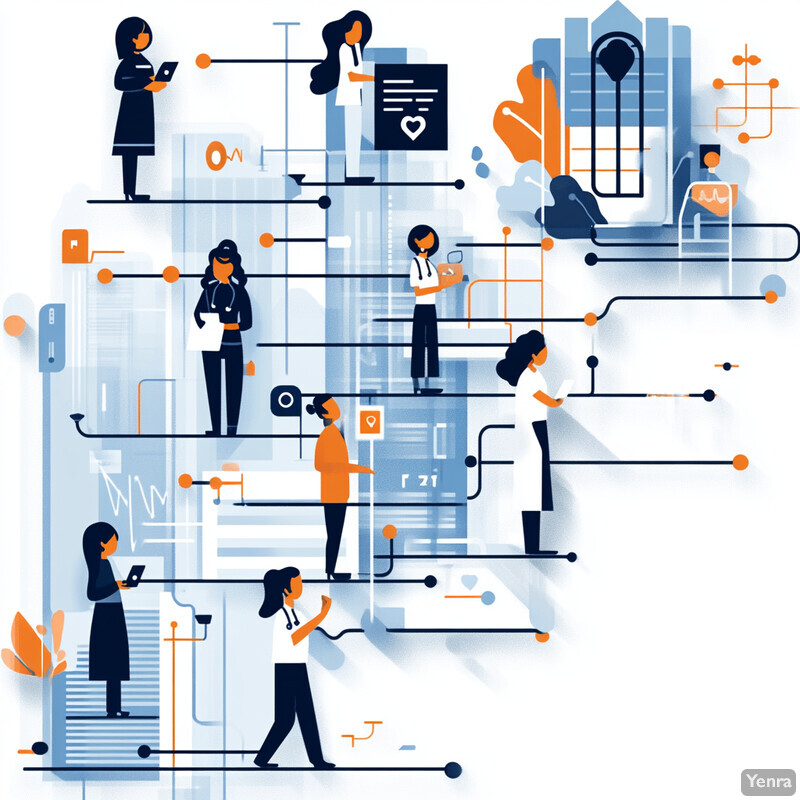
Patient dropout remains a key challenge in clinical trials, potentially compromising the integrity of results and increasing costs. AI can be leveraged to build predictive models that flag participants at high risk of early withdrawal based on patterns in their demographic data, past adherence behavior, and responses to early treatment. With this insight, sponsors can proactively implement retention strategies—such as personalized education materials, regular check-ins, or additional support services—to keep participants engaged. By anticipating and mitigating dropout risk, AI ultimately helps maintain a stable participant population, preserving statistical power and data integrity.
4. Adaptive Trial Design Simulation
AI tools can simulate various trial designs, sample sizes, and endpoint scenarios, enabling researchers to adjust protocols in real-time for more efficient, adaptive clinical trials.
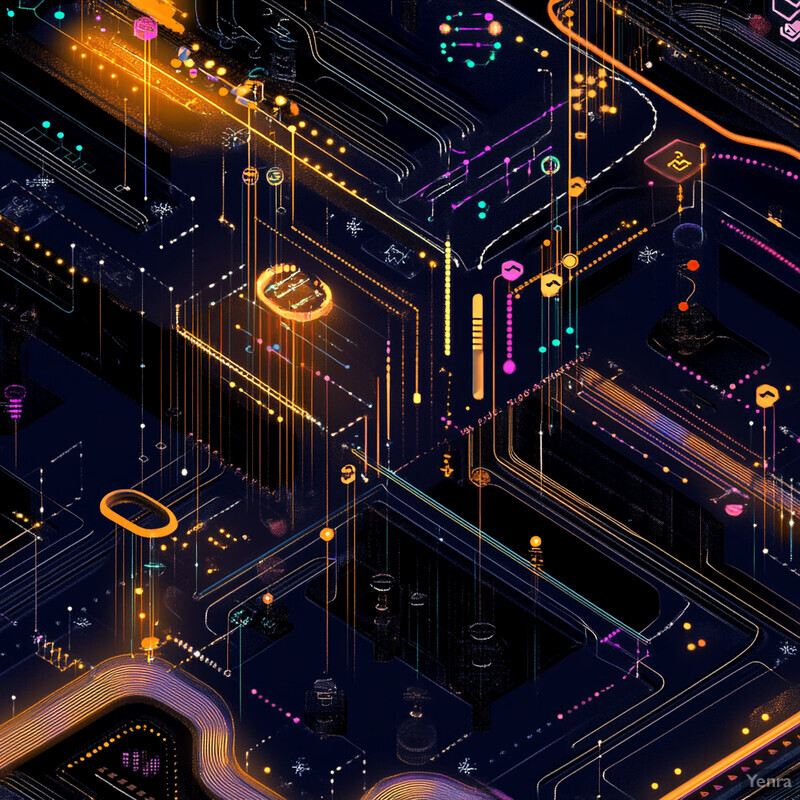
Designing a clinical trial often involves extensive scenario testing to ensure that the study is well-powered, ethically sound, and able to demonstrate meaningful outcomes. AI-driven simulation tools allow researchers to model various trial designs, sample sizes, and endpoint strategies before starting a study. For example, machine learning can use historical trial data and real-world evidence to predict how changes in inclusion criteria or randomization ratios might affect enrollment time, resource use, and overall success. By enabling flexible and informed decision-making, adaptive design simulations help sponsors refine protocols, reduce time and costs, and increase the chances of a successful trial outcome.
5. Precision Matching of Patients to Trials
NLP and deep learning models help match participants to the most appropriate trials based on genetic, phenotypic, and demographic factors, increasing the likelihood of therapeutic efficacy.
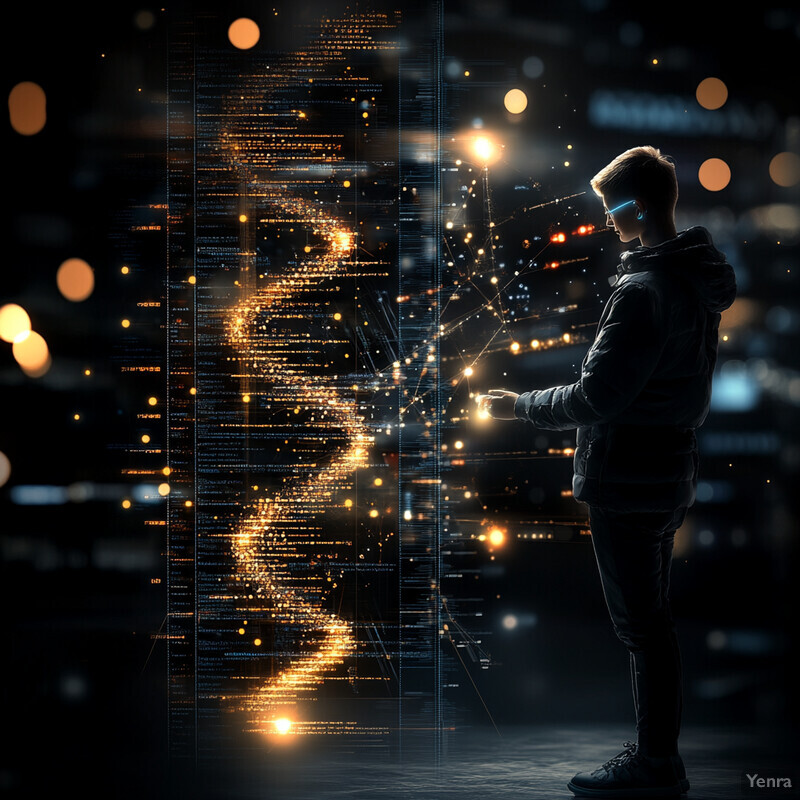
Ensuring the right patient is enrolled in the right trial is critical for evaluating treatment efficacy. AI, particularly through techniques like natural language processing and deep learning, can analyze complex patient data—including genetic profiles, comorbidity patterns, and previous treatment histories—to match individuals with the most suitable studies. This “precision matching” approach enhances the likelihood of demonstrating clinical benefit by selecting participants whose conditions align with the therapeutic mechanisms under investigation. It can also improve patient satisfaction, as individuals are more likely to receive interventions that are relevant and potentially effective for their specific health status.
6. Automated Data Cleaning and Quality Assurance
Machine learning algorithms can continuously monitor trial data for inconsistencies, errors, and outliers, automating the data cleaning process and ensuring higher-quality, more reliable datasets.
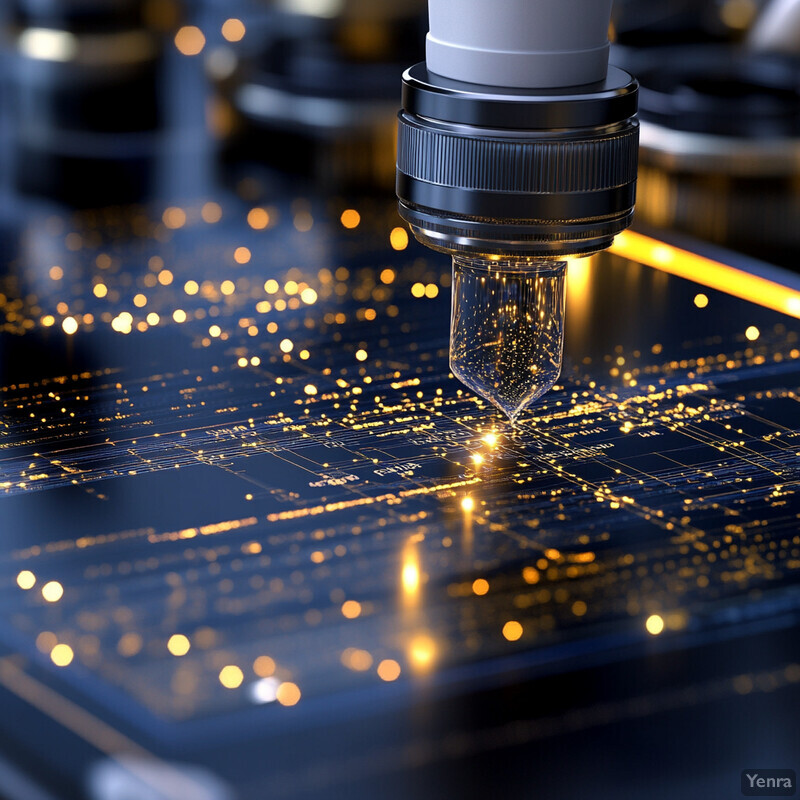
Clinical trials produce vast amounts of data from multiple sources, and ensuring its accuracy is a critical but labor-intensive task. AI-driven tools can continuously monitor incoming data for discrepancies, outliers, missing values, and potential errors. By automatically flagging and sometimes correcting issues in real-time, AI significantly reduces the manual workload of data managers and statisticians. This allows for quicker resolution of problems and more reliable, high-quality datasets. As a result, sponsors benefit from cleaner data that can be readily analyzed and used to draw robust, credible conclusions.
7. Real-Time Safety and Adverse Event Monitoring
AI-driven pharmacovigilance systems detect potential adverse events earlier by analyzing ongoing trial data streams, allowing for prompt corrective measures and protecting patient safety.
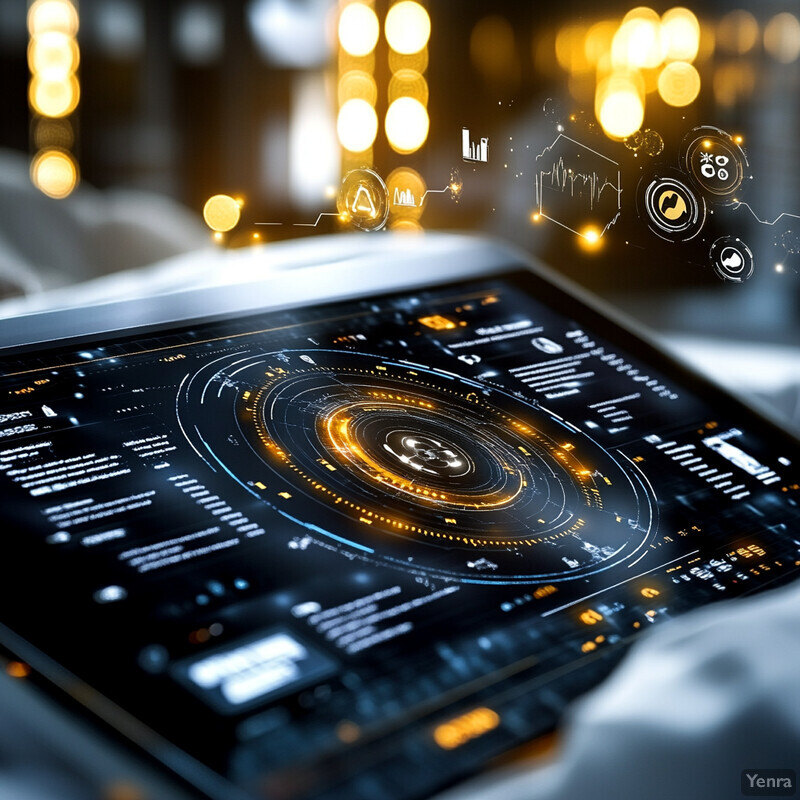
Patient safety is paramount in clinical research, and timely detection of adverse events is essential. AI systems, trained on large corpora of medical literature, historical trial databases, and ongoing patient-reported outcomes, can quickly detect signals that may indicate a potential safety risk. By continuously monitoring trial data streams, these systems can identify unusual patterns of symptoms, laboratory values, or medication tolerability that warrant closer scrutiny. Early recognition of safety signals enables sponsors and regulators to intervene promptly—adjusting dosages, altering trial protocols, or pausing a study if necessary—to protect participants and maintain ethical research standards.
8. Intelligent Protocol Design and Optimization
AI can assist in writing and refining clinical trial protocols by analyzing existing literature, regulatory guidelines, and historical trial data, resulting in clearer, more efficient study designs.
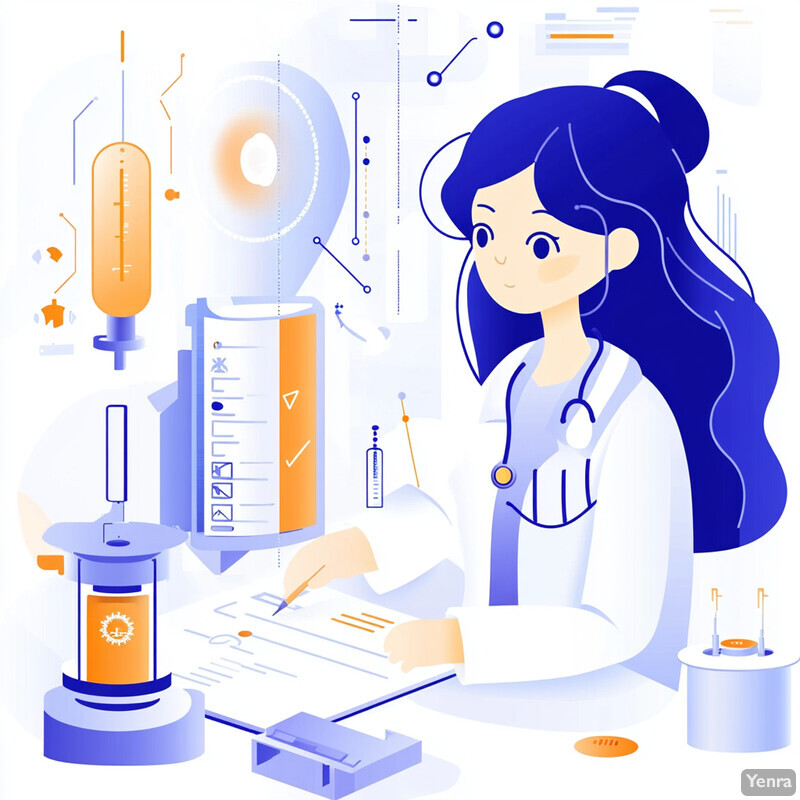
Writing a clinical trial protocol involves synthesizing scientific goals, regulatory requirements, logistical constraints, and patient considerations. AI can assist in this complex process by analyzing large volumes of prior studies, published literature, and regulatory guidance documents. Natural language processing tools can help identify common sources of confusion or inefficiency in protocols, and machine learning can recommend adjustments to eligibility criteria or endpoint definitions. By streamlining protocol development and ensuring its logical coherence, AI helps accelerate study start-up times and improves the clarity of research objectives, ultimately supporting more effective and credible trials.
9. Efficient Regulatory Document Processing
NLP can extract and interpret data from complex regulatory submissions, automatically flagging compliance issues or inconsistencies, accelerating the approval process.
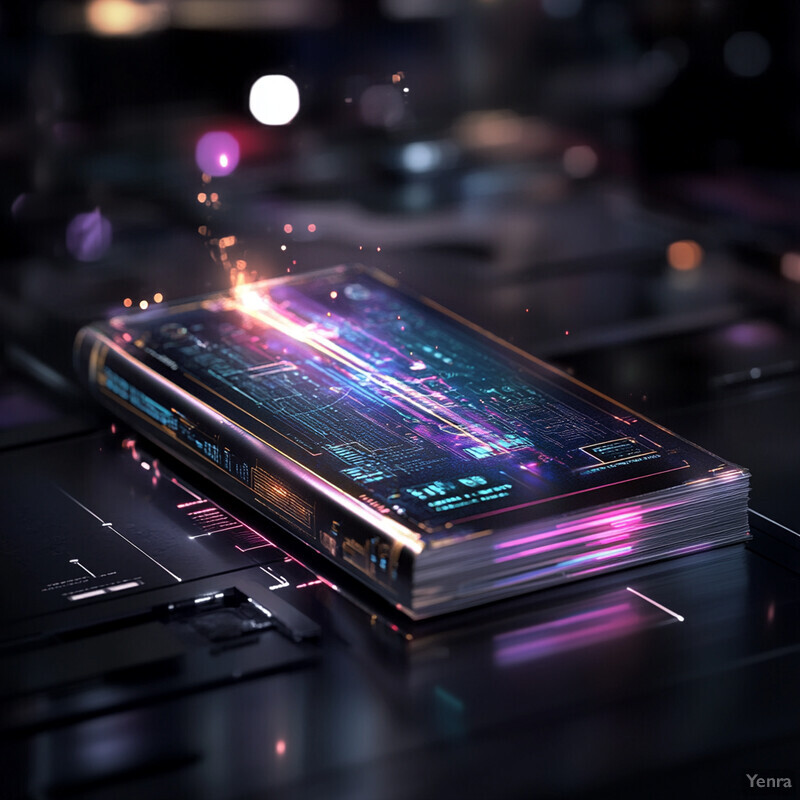
Regulatory submissions and approvals are famously time-consuming processes. AI, particularly advanced NLP models, can review and interpret large amounts of text in clinical trial applications, labeling submissions, patient narratives, and safety reports. These tools can identify inconsistencies, highlight areas that may require additional clarification, and ensure that the appropriate documentation is present. Such automation reduces the manual administrative burden on regulatory affairs specialists, shortens the feedback cycle with regulators, and may ultimately expedite approvals, bringing new therapies to patients more quickly.
10. Biomarker Discovery and Endpoint Refinement
AI-driven image recognition, genomics, and proteomics analyses can identify novel biomarkers and refine endpoints, making trials more targeted and outcomes more meaningful.
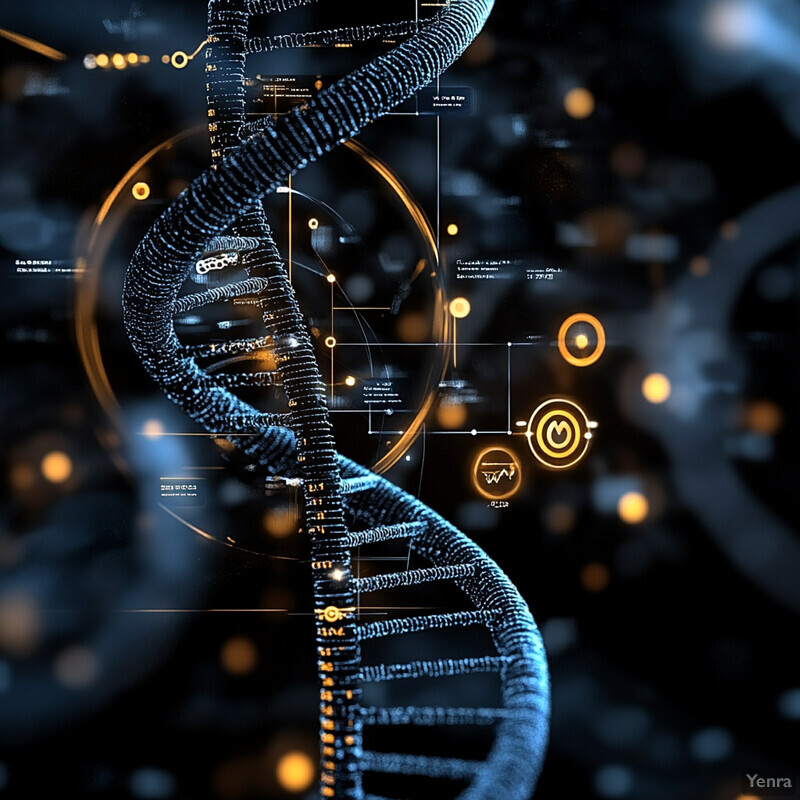
Identifying meaningful biomarkers and refining trial endpoints can significantly enhance the relevance and impact of a study. AI-driven analytical methods, including pattern recognition and machine learning, can sift through complex molecular and imaging datasets to discover new biomarkers that predict treatment response or disease progression. By identifying these biomarkers early, sponsors can adjust endpoints mid-study to focus on the most clinically valuable measures. This improved targeting can lead to more accurate assessments of therapeutic efficacy, reduce the time needed to detect meaningful outcomes, and open the door to precision medicine approaches.
11. Automated Monitoring of Trial Operations
Intelligent platforms can track trial progress in real-time—monitoring enrollment rates, data completeness, and budget—and alert managers to inefficiencies or delays.
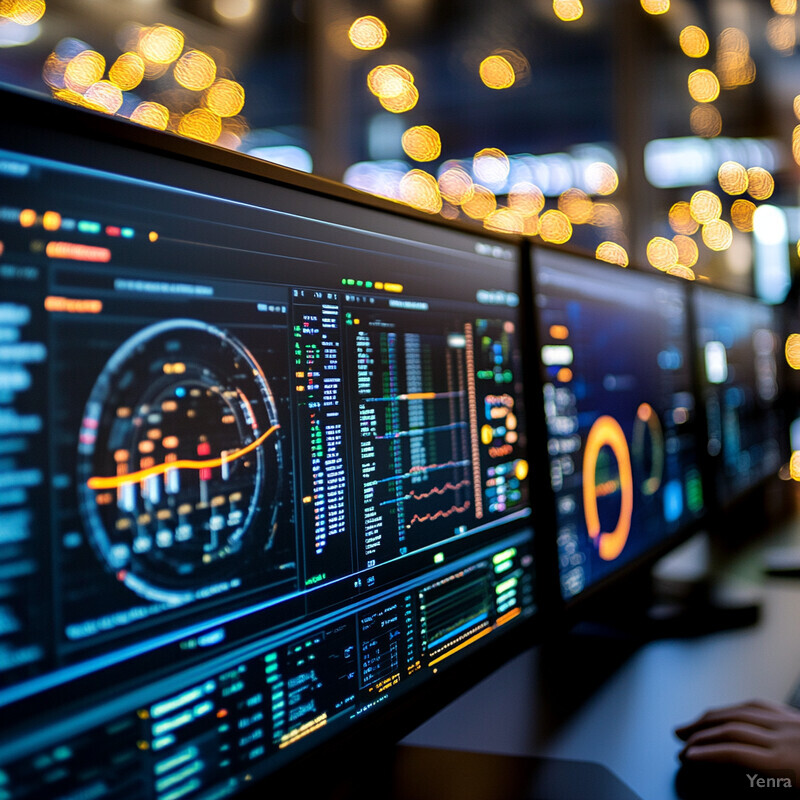
Managing the day-to-day operations of a clinical trial is a multi-faceted endeavor involving participant enrollment, data collection, budgeting, and timeline adherence. AI-powered dashboards can track trial metrics in real-time, from patient accrual rates and data query resolution times to spend against the study budget. By proactively identifying operational bottlenecks or early signs of delays, these tools enable managers to take corrective actions quickly. Such dynamic oversight increases overall efficiency and helps keep trials on schedule and within budgetary constraints.
12. Dynamic Risk-Based Monitoring
Machine learning models can guide monitors to focus on high-risk sites and data points, decreasing the need for universal on-site monitoring and reducing trial costs.
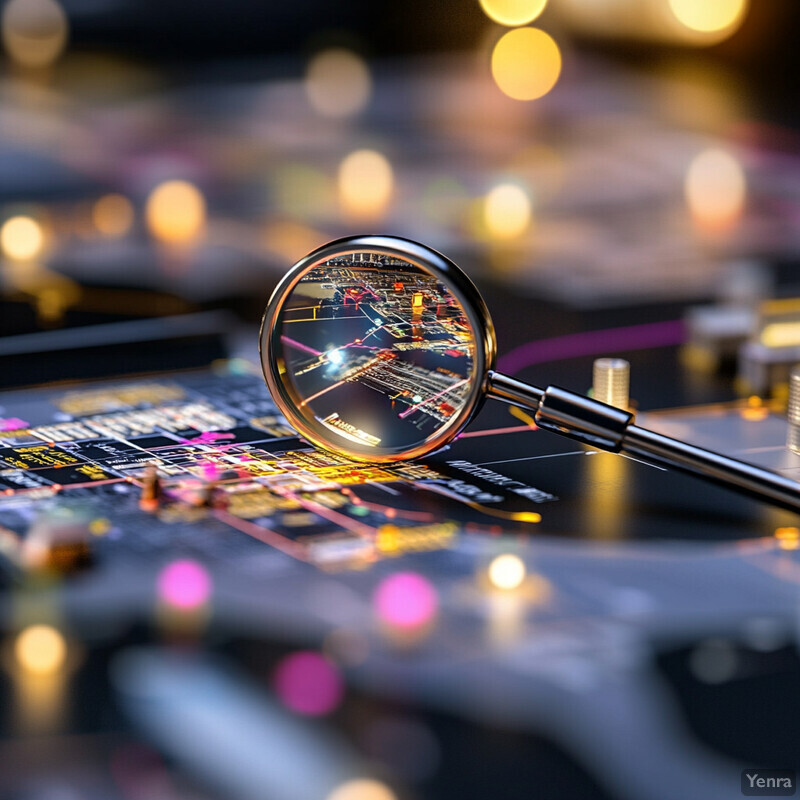
In traditional trials, on-site monitoring can be costly and time-consuming, with monitors manually reviewing data to ensure accuracy and compliance. Risk-based monitoring (RBM) strategies leverage AI algorithms that focus resources on sites or data points that are most likely to have issues. By detecting anomalies—such as unusually fast enrollment, missing data patterns, or suspiciously uniform outcomes—machine learning tools guide monitors to target their efforts where it matters most. This reduces unnecessary travel, cuts costs, and improves data quality by enabling a more strategic and evidence-based approach to oversight.
13. Intelligent Patient Engagement Tools
AI-powered chatbots and virtual assistants can enhance patient experience by providing immediate responses to inquiries, reminders for medication schedules, and feedback on reported symptoms.
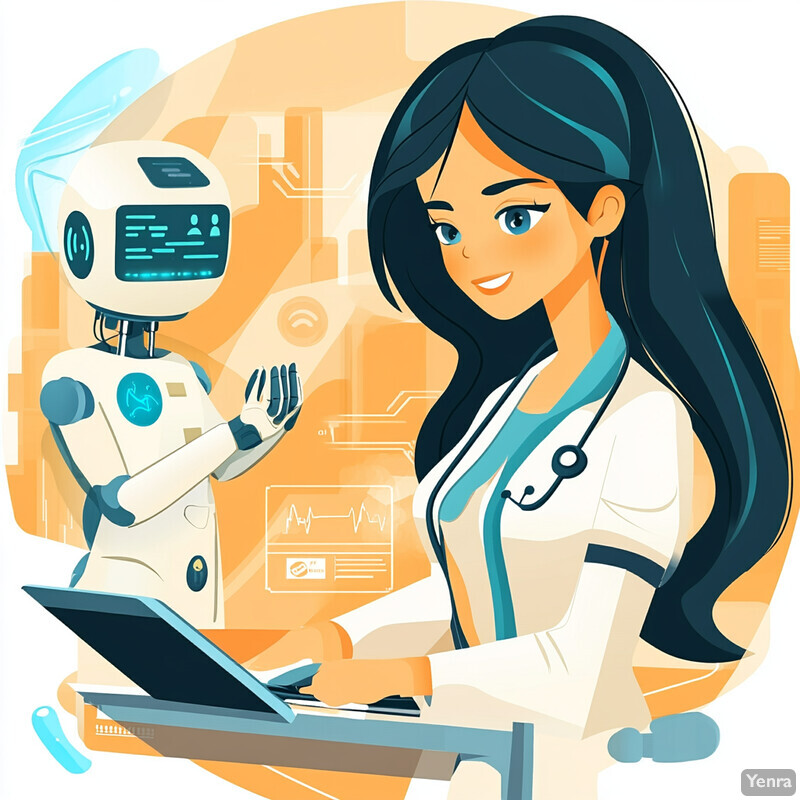
Participant engagement is essential for adherence to study protocols and maintaining data integrity. AI-driven chatbots, mobile apps, and virtual assistants can provide immediate, personalized support to trial participants. These tools might send reminders for medication schedules, explain upcoming procedures, or offer reassurance and education around adverse events. By facilitating two-way communication and providing assistance on-demand, AI-based engagement solutions enhance patient satisfaction, lower dropout rates, and ultimately strengthen the reliability of the trial data collected.
14. Supply Chain and Inventory Management
Predictive analytics models can optimize drug supply logistics—forecasting required quantities, timing, and distribution—preventing shortages, overstocking, and waste.
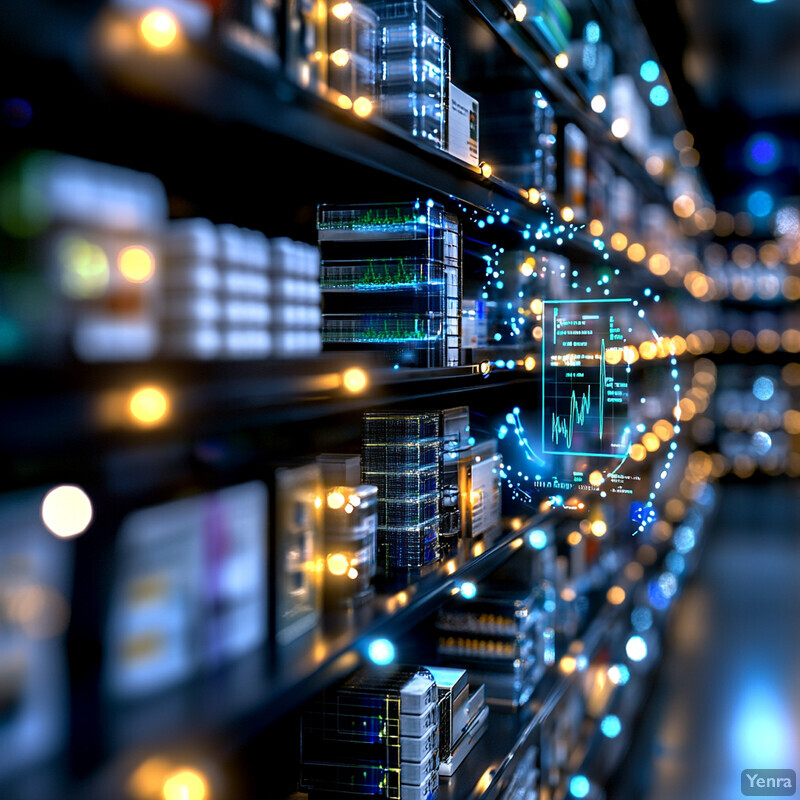
Ensuring that the right investigational product is available at the right time in the right quantity is a complex challenge. AI tools can forecast drug supply needs by analyzing enrollment trends, site performance, and consumption rates. This predictive modeling helps sponsors anticipate when and where supplies are needed, minimizing drug wastage and preventing stock-outs. With improved logistical efficiency, trials avoid costly interruptions and ensure that participants consistently receive the treatments required, enhancing data continuity and study credibility.
15. Automated Informed Consent and Education
AI-powered systems can deliver personalized explanations of trial protocols, risks, and benefits, potentially improving patient understanding and the quality of informed consent.
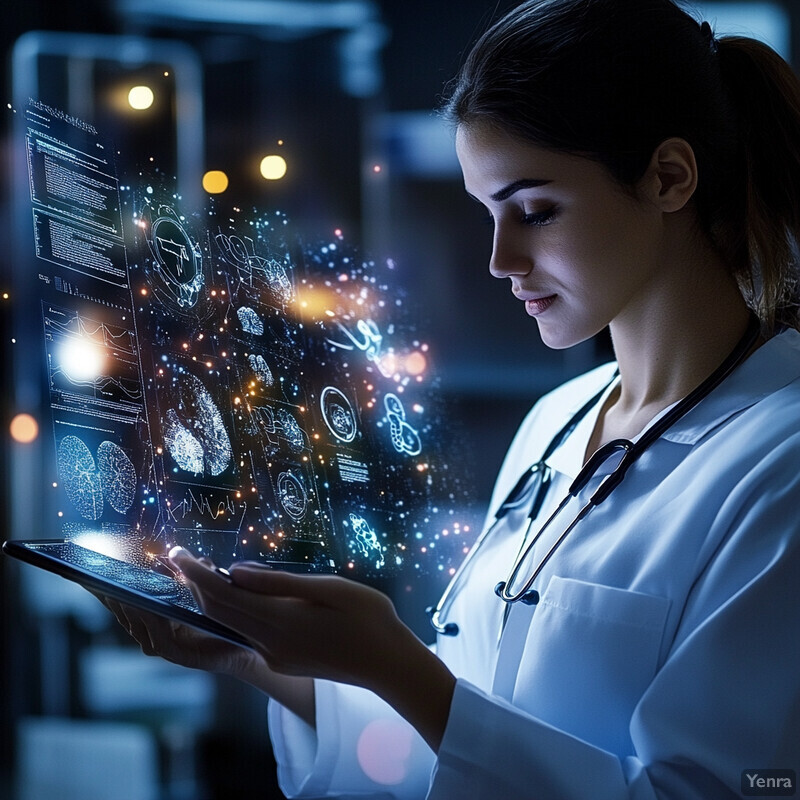
Informed consent is a critical ethical and regulatory requirement. However, complex trial protocols and medical jargon can make the consent process confusing for some participants. AI-driven platforms can provide simplified, personalized explanations of trial risks, benefits, and procedures, using multimedia elements like videos, graphics, and tailored text. By ensuring that patients clearly understand what participation entails, these tools help preserve ethical standards, improve participant comfort, and enhance the overall quality of the consent process.
16. Seamless Integration of Real-World Evidence (RWE)
AI can mine real-world data sources (EHRs, claims, wearables) to supplement trial findings, providing richer context and more robust evidence for decision-making and regulatory submissions.
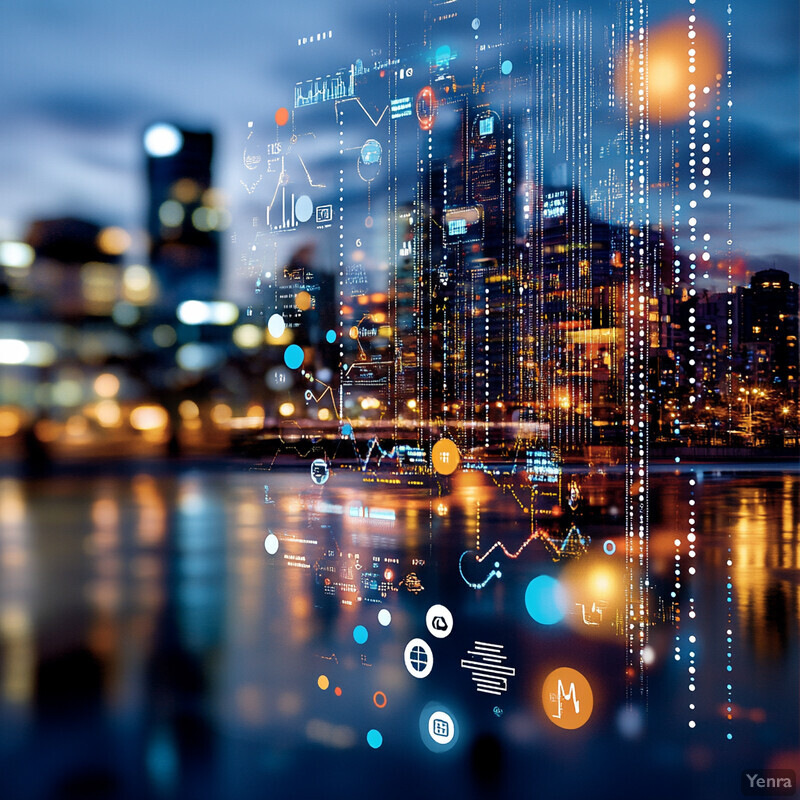
Clinical trials increasingly rely on real-world evidence (RWE) from sources like electronic health records, insurance claims, and wearable devices. AI can integrate and analyze these diverse data streams, contextualizing trial results within broader patient populations and real-world settings. This integration enables sponsors and regulators to better assess the generalizability of trial findings, streamline post-marketing surveillance, and identify potential subgroups that may benefit most from the studied intervention. By blending RWE with traditional clinical endpoints, AI creates a more complete picture of therapeutic value and safety.
17. Contextual Data Harmonization
Machine learning techniques help standardize heterogeneous data formats and terminologies across multiple trial sites, ensuring consistency and simplifying analysis.
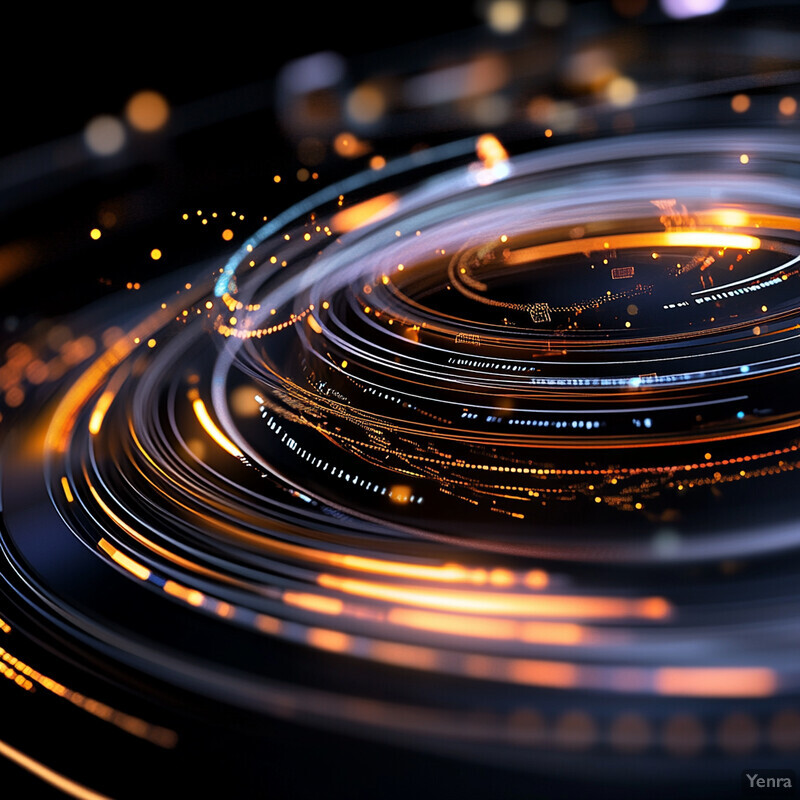
Clinical data often originate from multiple sources and come in varied formats, making integration a challenge. AI-driven solutions help harmonize these disparate datasets by mapping different terminologies, formats, and standards into a unified model. By ensuring that all trial data—ranging from laboratory values and imaging results to patient-reported outcomes—speak the same “language,” sponsors can run analyses more efficiently and with greater accuracy. The result is a cleaner, more coherent dataset that reduces the complexity of post-trial analyses and regulatory submissions.
18. Adaptive Endpoint Detection and Analysis
As data accrues, AI can refine endpoints mid-trial, identifying the most clinically relevant measures of efficacy or safety, thus improving the likelihood of meaningful outcomes.
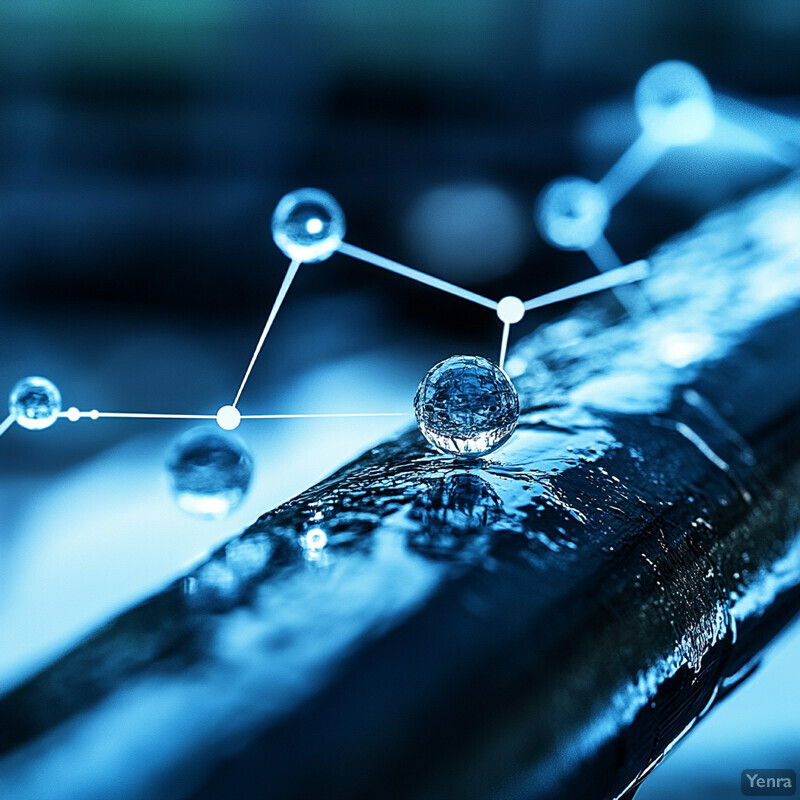
Over the course of a trial, new information may emerge, suggesting that certain endpoints are more clinically meaningful than originally anticipated. AI can dynamically adjust and refine endpoints mid-study by analyzing ongoing data streams and patient responses. For example, if early results indicate that a secondary outcome measure is providing particularly strong signals of therapeutic benefit, the trial team may shift focus to that endpoint. This adaptability ensures that studies remain relevant, maximizing the potential for meaningful results and supporting more informed decision-making about the investigational therapy.
19. Early Signal Detection for Efficacy Trends
Predictive models can identify subtle trends in patient response data earlier than traditional methods, enabling quick protocol adjustments or informed go/no-go decisions.
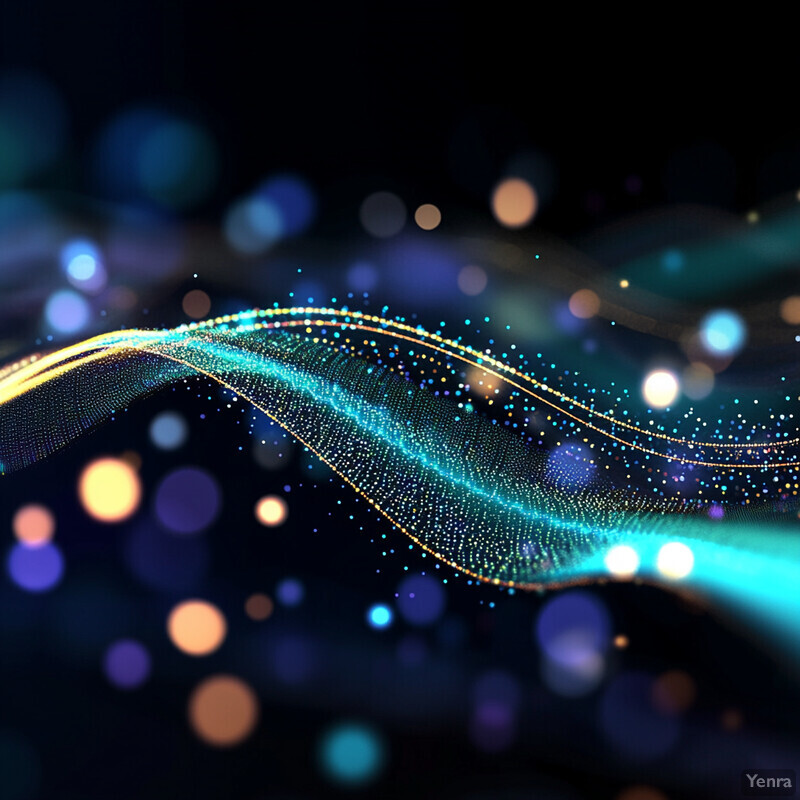
Identifying early signals of efficacy can help sponsors decide whether to continue, modify, or terminate a trial. AI can detect subtle patterns in symptom improvement, biomarker changes, or patient-reported outcomes that might be missed by human analysts. By recognizing these signals early, sponsors can reallocate resources, refine study focus, or make go/no-go decisions sooner, saving time and money. Early detection of promising efficacy trends can also hasten the delivery of potentially life-saving treatments to patients who need them most.
20. Automated Clinical Study Reports and Summaries
NLP and summarization algorithms can draft and review final study reports and regulatory documents, accelerating reporting timelines and ensuring comprehensive, consistent documentation.
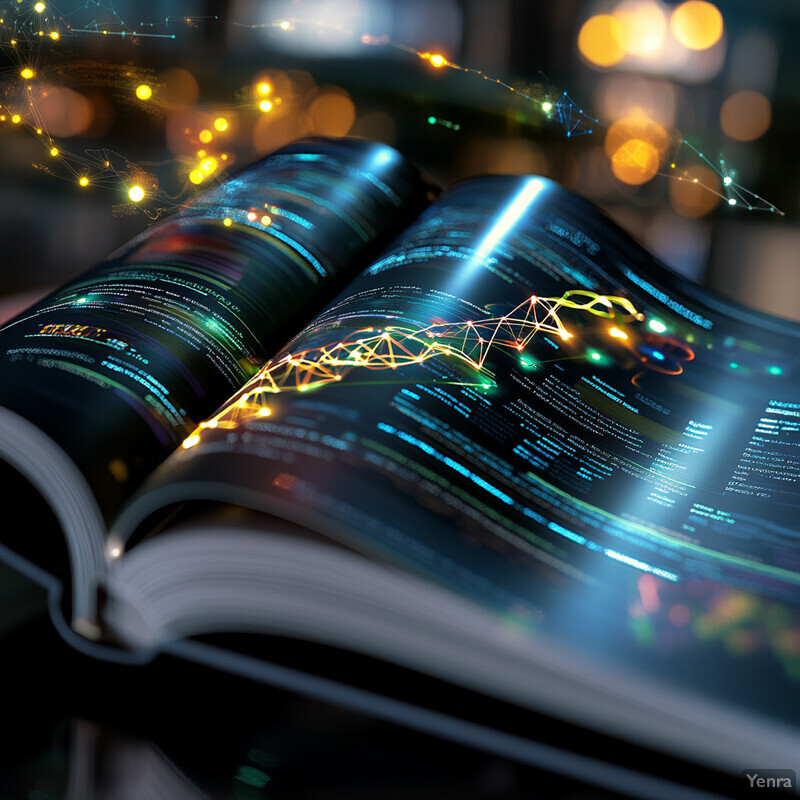
Preparing final study reports and regulatory documents is time-consuming and requires meticulous attention to detail. AI tools powered by NLP can help draft these documents by aggregating and summarizing trial results, statistical analyses, and relevant literature references. Automated solutions can also highlight data inconsistencies, check compliance against reporting standards, and suggest clearer phrasing. By streamlining the reporting process, AI reduces the administrative burden on clinical teams, shortens the time to regulatory submissions, and ensures more consistent, high-quality documentation throughout the trial lifecycle.