Song: Chemical Analysis in Oil and Gas
1. Automated Spectral Interpretation
AI-driven models can automatically interpret complex spectroscopic data (e.g. FTIR, NMR, Raman) much faster than manual analysis. These tools learn patterns in large spectral libraries, rapidly matching unknown spectra to known chemical features. Automated interpretation reduces expert time, improves consistency, and flags subtle signals that might be missed. In petroleum labs, such AI models can sift through thousands of spectra and identify organic and inorganic components automatically. The speed and accuracy gains mean more samples can be processed with fewer delays. AI also adapts over time: as more data are added, interpretations become more precise without manual reprogramming. Overall, automation of spectral analysis is accelerating chemical identification in oil and gas labs.
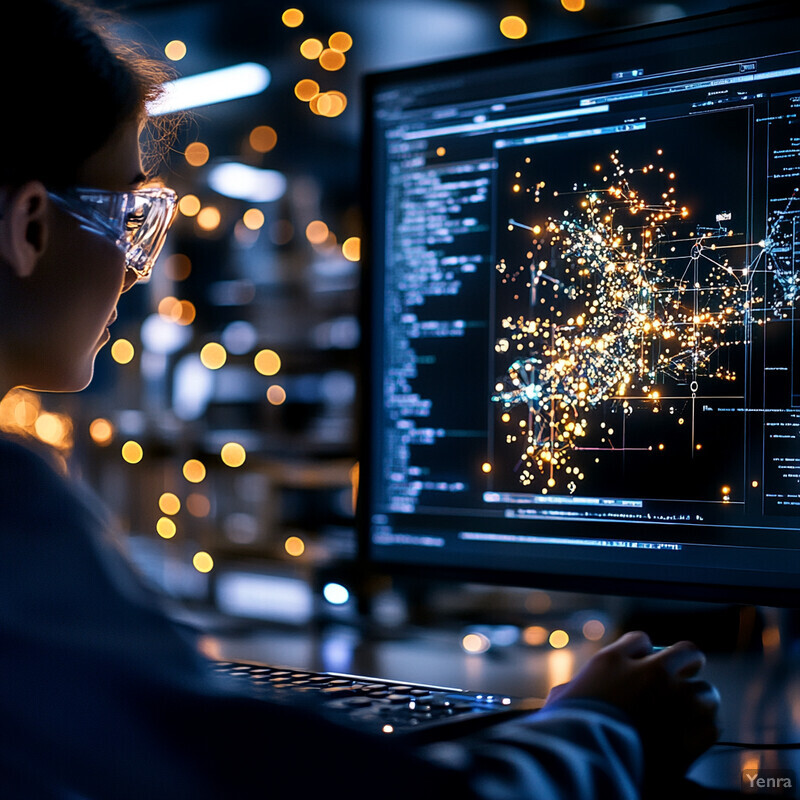
Recent work demonstrates the power of AI in spectroscopy. For example, a study used a convolutional neural network to classify FT-IR spectra of organic molecules with ~93% accuracy for functional groupsspectroscopyonline.com . This AI model was trained on over 14,000 spectra and outperformed traditional chemometric methods by streamlining feature extraction and classification. Similarly, low-field NMR relaxometry data have been analyzed by supervised learning to predict oil properties (cetane number) without lengthy experiments. These AI approaches effectively “learn” spectral fingerprints: in one case, a hybrid mass spectrometry/ML method predicted crude oil acidity (Total Acid Number) with high accuracy using small samplespmc.ncbi.nlm.nih.gov . Such results indicate AI can replace or greatly reduce manual spectral interpretation, offering near-real-time chemical identification in upstream and refining labs.
2. Enhanced Chromatography Analysis
AI augments chromatographic analysis (e.g. GC, LC, GC×GC) by automating peak detection, deconvolution, and quantitation. Machine learning can recognize overlapping peaks and artifacts, yielding cleaner separations and more reliable results. In practice, AI systems learn from large datasets of chromatograms to predict retention times and peak shapes, enabling adaptive methods that require less human calibration. This speeds up analysis of complex petroleum fractions where many components co-elute. AI also spot-checks for irregularities: for instance, models can flag suspect peaks (drift or contaminants) that warrant reanalysis. Overall, AI-driven chromatography can improve throughput and accuracy, reducing time-to-result for fuel quality and contaminant assays.
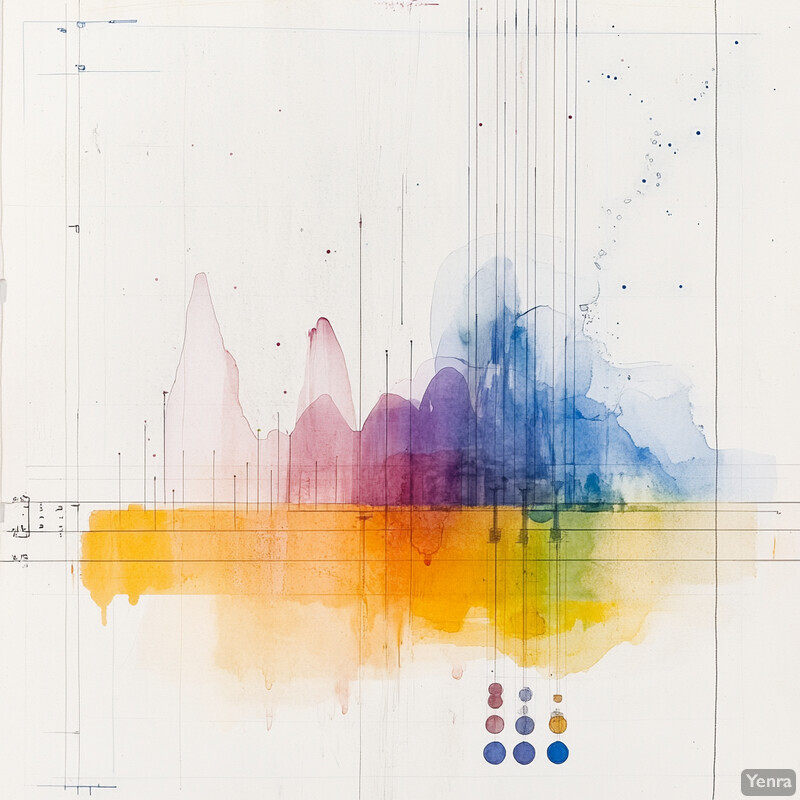
AI techniques have been applied to streamline chromatographic data processing. Neural networks and other ML algorithms can model non-linear retention behavior, reducing the need for extensive calibration. For example, a deep-learning approach was used to optimize coagulant/flocculant dosages by predicting key performance indicators from past chromatographic data. In targeted GC–MS, AI classifiers (e.g. random forests) can distinguish overlapping hydrocarbon signals more precisely than manual peak picking. Moreover, AI-based alignment algorithms automatically correct retention shifts across batches, which is otherwise labor-intensive. These advances let chemists process more samples with fewer bottlenecks. As one chromatography review notes, AI “extracts relevant information quickly,” replacing “methods requiring extensive expertise and time”.
3. Rapid Reservoir Fluid Analysis
AI-integrated analysis systems enable much faster identification of reservoir fluid compositions from downhole measurements. By training on spectral or sensor data (e.g. NIR/optical logs), machine learning models can infer fluid type and key properties (API gravity, contamination levels) in near real time. This accelerates decisions during drilling and testing: operators can adjust well plans or chemical treatments on the fly rather than waiting days for lab PVT results. AI also handles incomplete data: partial spectra or limited sensor inputs are still sufficient for classification. In practice, compact neural networks deployed in drilling equipment analyze fluids as they’re produced, flagging gas vs. oil or identifying emulsion strength instantly. The result is faster integration of fluid analysis into reservoir management.
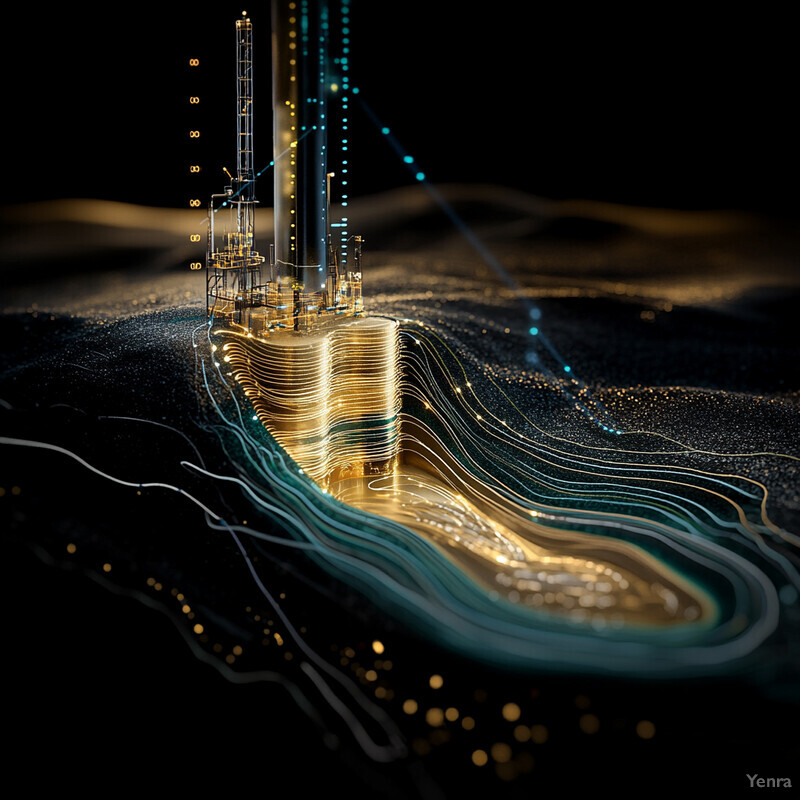
Recent field-type studies demonstrate on-site AI fluid analysis. For example, Chen et al. (2024) developed a downhole fluid identification system using only four NIR wavelengths and a lightweight quadratic neural network on a microcontroller. Their model achieved “high identification accuracy” of oil–water–gas mixtures with minimal input data. Deployed in an STM32 system with laser diodes, it classified fluid type and even estimated oil contamination level in real time during drilling. This approach overcame bulky lab setups: instead of shipping samples, the model ran instantaneously, guiding drilling decisions. In another example, machine learning has been applied to recover missing well log curves (e.g. density, porosity) via LSTM networks, giving R²≈0.96 on test data. Such results show AI can predict fluid characteristics from indirect measurements quickly and accurately, dramatically reducing turnaround time compared to conventional lab analysis.
4. Predictive Modeling of Refining Yields
AI models predict product yields from refinery processes (e.g. catalytic cracking, hydrotreating) using process inputs. Neural networks and hybrid models learn the complex chemistry–process linkages, forecasting output volumes of gasoline, diesel, etc. In planning, this lets refiners estimate yields for different crude slates or operating conditions without costly trial runs. These models can incorporate hundreds of variables (temperature, catalyst age, feedstock properties) to optimize reactor settings. With AI, engineers run “what-if” scenarios for new operating regimes quickly. The predictions guide blending and scheduling to maximize profits. The result is smoother production planning and better margin optimization through data-driven forecasts of conversion yields.
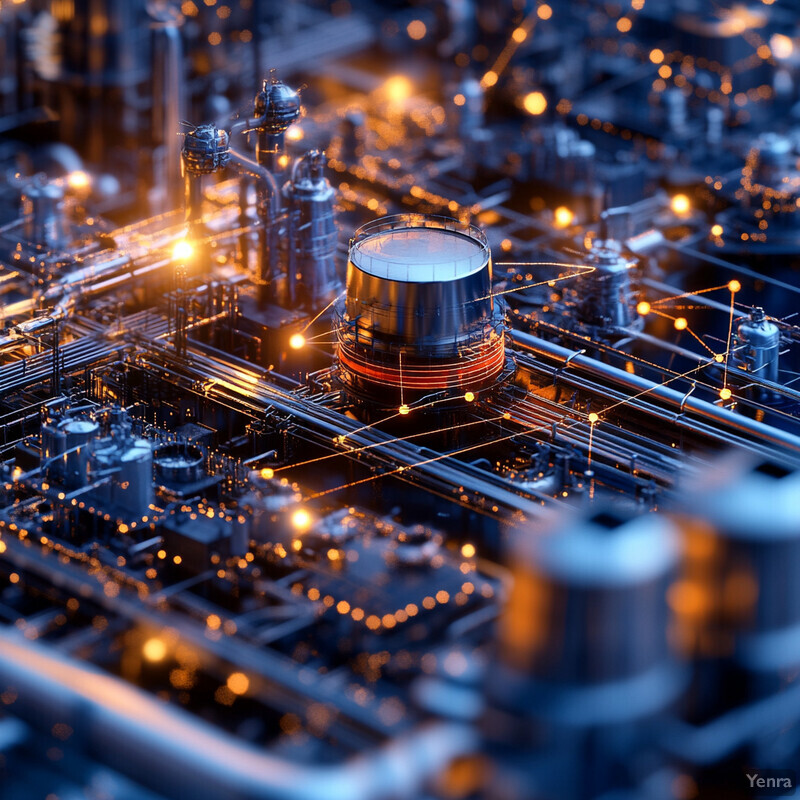
Studies have shown high-accuracy yield predictions using AI. Li et al. (2025) used a hybrid convolutional LSTM neural network combined with mechanistic reactor models, optimized by particle swarm, to predict hydrocracking yields. Their model achieved R² values of 0.78–0.90 for key product streams. This matches or exceeds accuracy of full simulators but with far less computing. Other researchers applied deep reinforcement learning to set cracking unit parameters, also reporting improved product distribution and energy use. For gasoline blending predictions, an AI+genetic algorithm system achieved an R²=0.99 on octane number (AKI) across many blends. These examples confirm that predictive ML models can approximate complex refinery chemistries and process dynamics, enabling quantitative yield forecasts that assist in planning and optimizationmdpi.com .
5. Chemical Fingerprinting of Crudes
AI can identify unique “fingerprints” of crude oils and fluids based on their detailed chemical profiles. By learning from molecular data (mass spectra, chromatography, isotope ratios), machine learning models can classify crude origins, grades, or contamination. This supports crude tracking and fraud detection: an unknown oil sample can be matched to its field of origin or blend. It also aids in monitoring spills and blends by linking a complex mixture back to source wells. In reservoir engineering, correlating source rock markers with production fluids via AI reveals migration paths and compartmentalization. Overall, AI fingerprinting turns rich chemical data into actionable markers for oil sourcing and quality control.
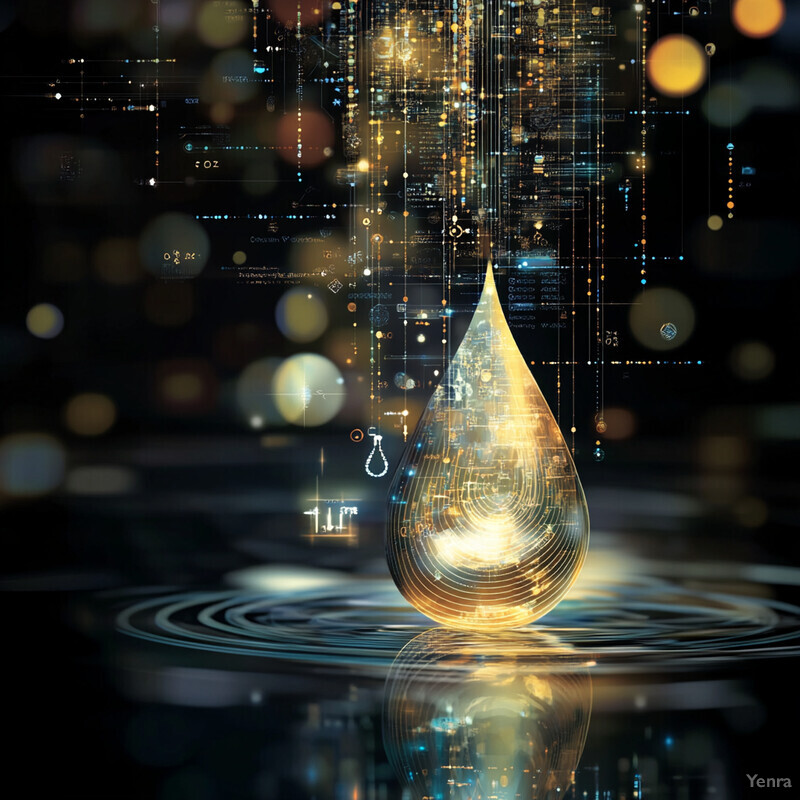
Recent studies illustrate AI fingerprints of petroleum. For example, Roque et al. (2025) combined ultrahigh-resolution FT-ICR mass spectrometry with ML to predict Total Acid Number (TAN) across crude oil distillation cutspmc.ncbi.nlm.nih.gov . Their models required only small samples and gave fast, accurate acidity profiles, effectively encoding crude composition. In another study, an “electronic nose” measured volatile compounds from soil contaminated by crude spills. A random forest classifier distinguished the field of origin with 91% accuracy on 2,200 pre-salt oil samples. This shows AI can map complex chemical features (like VOC patterns or high-resolution MS peaks) to specific oils. Such AI fingerprinting provides rapid assessment of feedstock quality and aids environmental forensics by matching oils to known samples with high confidencenature.com .
6. Corrosion and Scale Prediction
AI models forecast corrosion rates and scale deposition by analyzing chemical environments. Using operating data (pH, ion concentrations, flow rates), machine learning can predict when and where corrosion or scale is likely, allowing pre-emptive mitigation. These predictions combine lab testing data and field observations to capture complex chemistry. Operators can thus schedule maintenance (e.g. inhibitor injection) precisely before a shutdown or failure, avoiding unplanned downtime. In practice, an AI algorithm might alert a pipeline operator if models indicate high risk of CaCO₃ or sulfide scale in a segment, triggering inhibitor adjustments. By reducing surprise failures, AI-driven prediction greatly improves asset reliability and life-cycle management.
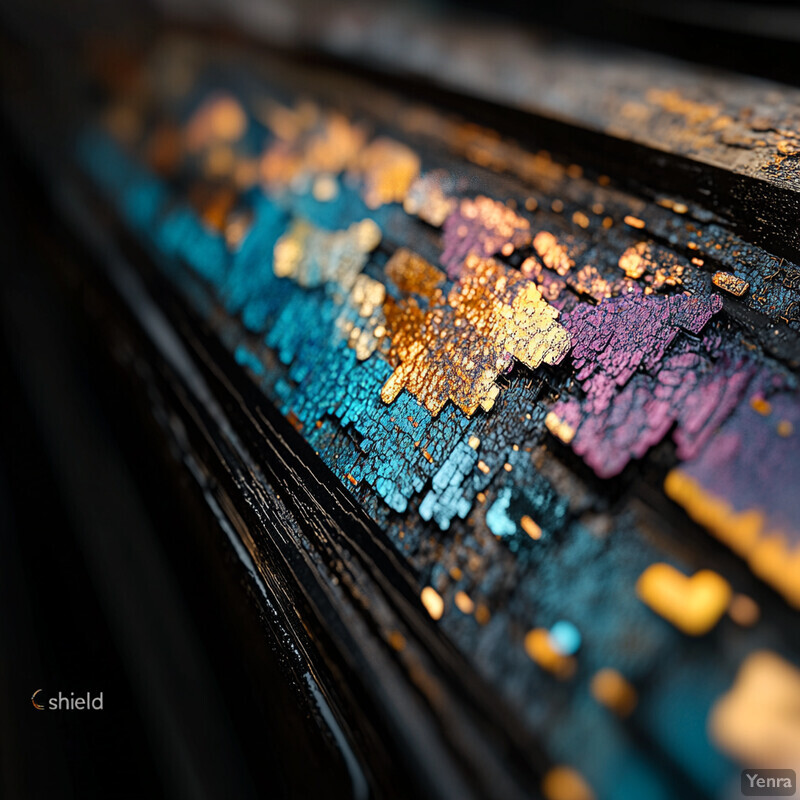
Several recent studies demonstrate ML in corrosion/scale forecasting. Liu et al. (2023) developed an AI model (PSO-optimized neural network) to predict CO₂ corrosion rates in natural gas pipelines. Their model outperformed eight baseline models in accuracy by learning from field data. It identified key inputs (flow velocity, CO₂ content) and delivered fast predictions for internal corrosion rate, aiding maintenance planning. Likewise, Khodabakhshi and Bijani (2024) applied multiple ML algorithms to forecast scale deposition of CaSO₄, BaSO₄, SrSO₄ in oil reservoir flowlines. After hyperparameter tuning, support vector regression achieved R²=0.900 and k-nearest neighbors R²=0.996 on validation setscolab.ws . These high R² values show ML can capture the non-linear chemistry of scale formation. By using AI models like these, engineers can simulate scenarios (e.g. temperature or concentration changes) and estimate when corrosion inhibitors or scale treatments must be applied to maintain flow.
7. Data Quality Assurance and Outlier Detection
AI algorithms continuously check analytical data streams for anomalies and errors. Machine learning models (e.g. anomaly detection) learn what “normal” measurement patterns look like, so they can flag outliers (erroneous samples or instrument faults) automatically. This ensures that bad data (e.g. a contaminated sample or sensor malfunction) are caught immediately, avoiding their inclusion in critical decisions. In oil labs, AI may monitor sensor health or recognize unrealistic fluid analysis results. Likewise, in monitoring and logging, AI outlier detection spots weird readings in petrophysical logs or production data. By systematically filtering data quality issues, AI helps maintain reliable chemical databases and reduces the need for manual rechecks.
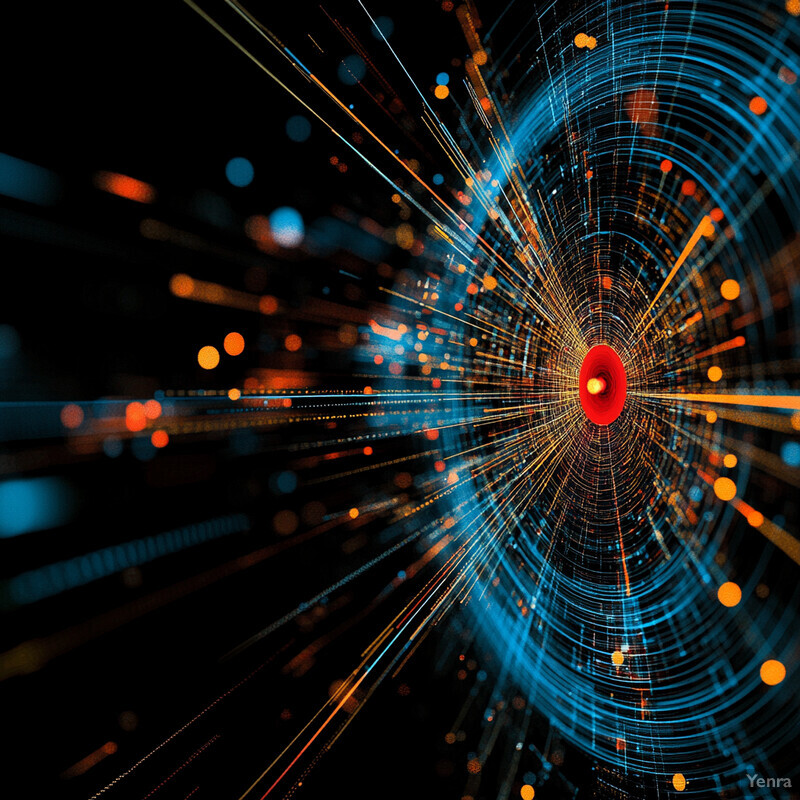
A recent case study applied ML outlier detection to reservoir fluid samples. Hosseini and Pourafshary (2024) compared five unsupervised methods on PVT and composition data and achieved an average identification accuracy of 79% in spotting outlier fluid samples. This was better than conventional rule-based checks. The ML models (isolation forest, local outlier factor, etc.) could flag invalid or non-representative samples, prompting lab reanalysis. Similar anomaly detection has been used in real-time monitoring systems, where AI algorithms classify sensor streams; if a reading deviates strongly, it’s marked “suspect”. In practice, AI-driven quality control integrated with digital records means fewer false results enter the workflow. By automatically highlighting inconsistent results, AI reduces wasted lab retests and improves confidence in chemical data.
8. Reservoir Characterization via Geochemistry
AI combines geochemical data (trace element ratios, biomarkers, isotopic signatures) with geological information to characterize reservoirs. By learning patterns in geochemical markers, machine learning models can predict reservoir quality, maturity, and source rock correlations. This helps integrate chemistry with petrophysics and seismic data for better reservoir models. For example, AI might infer porosity from organic geochemical content, or match reservoir fluids to specific source rocks. These AI-assisted interpretations allow geoscientists to map lateral variations more accurately. In effect, AI turns detailed lab measurements into predictive tools for reservoir classification and flowpath mapping.
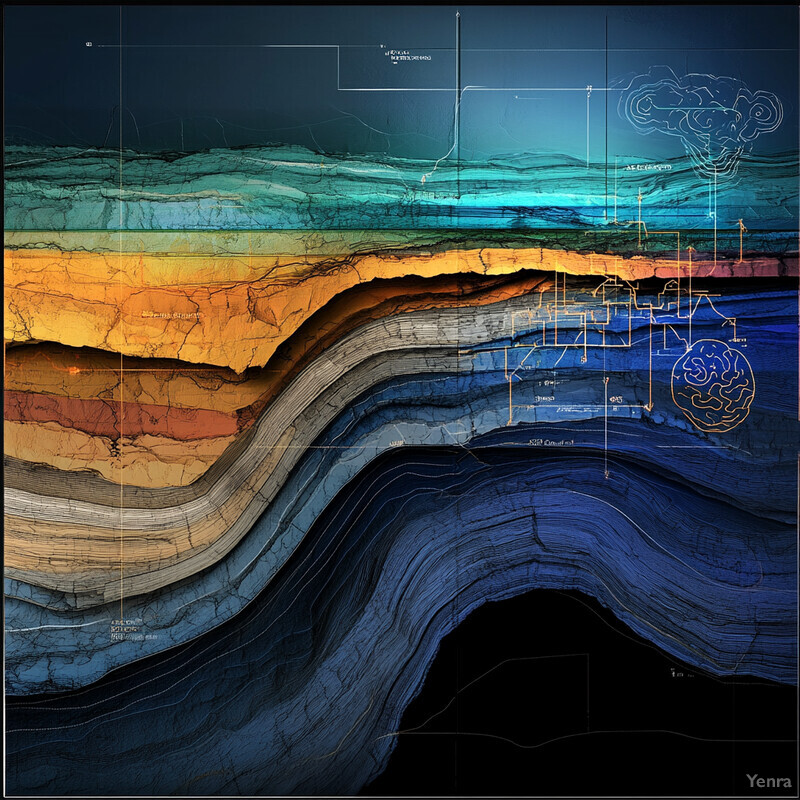
Recent work applies ML to petroleum geochemistry data for reservoir insights. One study used molecular geochemistry (GC×GC and NIR data) in a neural network to link oil composition to specific depositional facies, improving facies prediction accuracy over standard lithology logs. In environmental forensics (closely related to petroleum geochemistry), Silva et al. (2025) trained models on presalt crude molecular fingerprints and achieved ~91% accuracy distinguishing oil samples by field origin. This shows AI can extract geochemical signals of provenance and maturation. While AI in reservoir geochemistry is still emerging, these results suggest it can bridge the gap between lab geochemical analyses and large-scale reservoir characterization. For example, ML models have been proposed to estimate key reservoir properties (e.g. depositional environment, maturity) from biomarker data, streamlining integration of geochemical logs into reservoir models.
9. Optimized Enhanced Oil Recovery (EOR) Strategies
AI helps design and optimize chemical EOR processes (e.g. polymer flooding, surfactant floods, CO₂-EOR) by simulating outcomes and identifying best conditions. Machine learning models predict how variables (injection rate, chemical concentration, pressure) affect oil recovery efficiency. This allows fast screening of injection schemes without exhaustive pilot tests. In the field, operators can use AI-driven optimization loops: as production data come in, the AI model updates and suggests adjustments to EOR parameters. For example, AI might tune surfactant mixture ratios or adjust polymer slug viscosity in real time to maintain high sweep efficiency. This leads to improved overall recovery and lower chemical costs.
-strategies-0.jpg)
Several studies in CO₂ and chemical EOR highlight AI’s benefits. A comprehensive review (Du et al., 2024) notes machine learning has been applied to tasks such as predicting minimum miscibility pressure (MMP) for CO₂ floods, optimizing injection patterns, and forecasting recovery factors. In specific cases, neural networks trained on coreflood datasets predicted oil recovery changes under various injection scenarios, guiding chemical slug design. Another study used reinforcement learning to select optimal water alternating gas (WAG) schedules, significantly increasing cumulative oil recovery in simulation. In polymer flooding, convolutional neural nets have estimated polymer concentration profiles that maximize sweep. In sum, AI models ingesting lab and field data can propose EOR strategies (fluid formulations, injection timings) that have been validated by comparison with pilot results. This confirms AI’s potential to enhance EOR planning and field management.
10. Faster Exploration Decisions
AI expedites exploration by rapidly analyzing diverse data (logs, seismic, geochemical) to rank prospects. In early exploration, ML models can correlate sparse log data or seismic attributes with reservoir potential, guiding drill-or-drop decisions. For instance, if partial well logs are available, AI can predict missing logs or facies, speeding up resource estimates. In fast-moving scenarios, pre-drill AI analysis can suggest likely reservoir properties in new basins based on analog data. This reduces uncertainty and accelerates commitments. Operators thus can decide on exploration next steps (appraise, sidetrack, abandon) more quickly, reducing idle time and exploration costs.
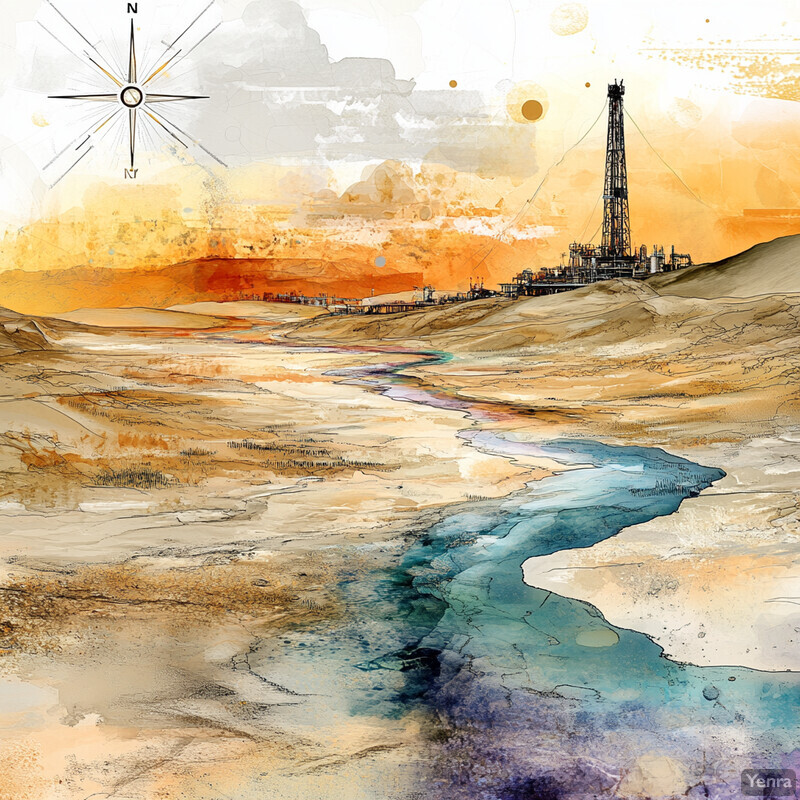
AI models have shown large gains in log analysis and prospect evaluation. For example, Wang et al. (2024) developed an LSTM-based model to recover missing petrophysical logs (density, porosity) from available logs. Their LSTM-EPSCHOA model achieved R²=0.96 on the test dataset, far outperforming a standard LSTM (R²=0.59). This means exploratory wells with partial data still yield a full suite of logs automatically. In addition, industry tools use ensemble ML (RF, SVM) on seismic attributes to predict “sweet spots” ahead of drilling, accelerating exploration targeting. While many of these applications remain proprietary, the available studies show AI can cut data interpretation time from weeks to hours. For instance, one workflow automatically screened sedimentary cores and logs to classify reservoir quality facies, enabling geologists to focus only on anomalies. Such rapid analysis translates to faster exploration choices and drilling campaigns.
11. Catalyst Performance Prediction
AI predicts catalyst behavior in reactors by correlating catalyst formulations and conditions with activity and selectivity. Machine learning models trained on lab or plant data can forecast catalyst lifetime, deactivation rates, and optimal replacement times. They can also screen new catalyst materials by predicting their performance metrics before costly synthesis. In refinery and petrochemical units, this means operations can be planned to use catalysts up to their limit, and new catalysts developed more efficiently. AI insights into catalysts (e.g. which chemical features correlate with longevity) accelerate R&D for better catalysts. Operators benefit through reduced downtime and higher throughput using catalysts at peak efficacy.
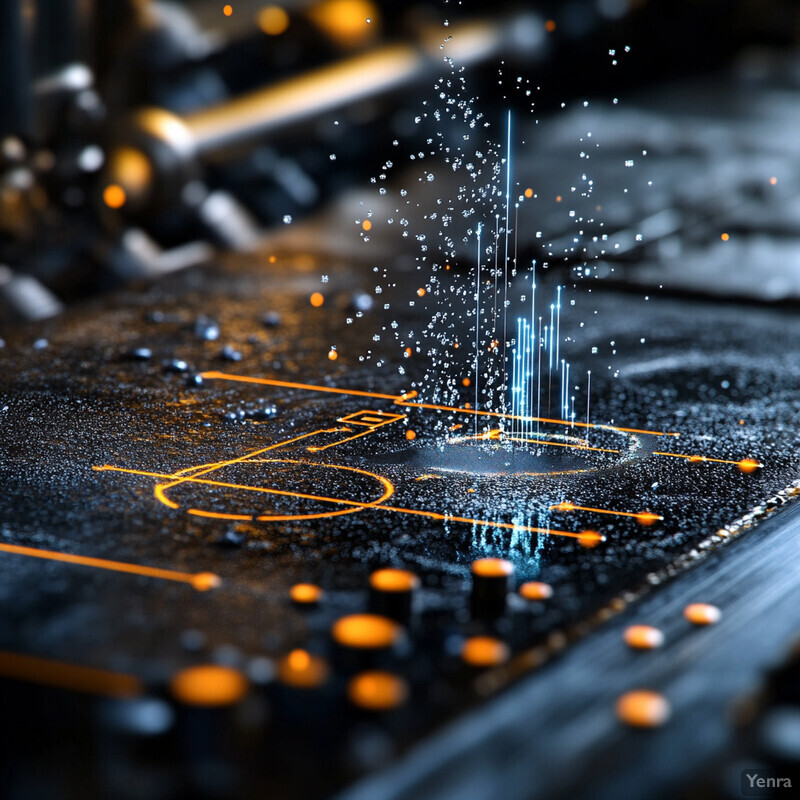
Studies have applied ML to catalyst datasets for performance forecasting. For example, Semnani et al. (2024) introduced a framework tailored to small, unbalanced catalyst discovery data sets. Using explainable AI, their model predicted catalyst screening results robustly despite limited data, and identified key catalyst components affecting performance. This demonstrates that even sparse experimental data can be leveraged. In another case, neural networks were used to predict hydrocracking catalyst conversion rates from feed properties and catalyst type, enabling rapid evaluation of catalyst options. While detailed catalyst data in oil/gas is often proprietary, the published methods show AI can handle heterogeneity and infer structure–activity relationships. By combining spectral/material property descriptors with known activity results, AI models yield quantitative performance predictions that guide both selection and design of catalysts for petroleum processes.
12. Environmental Compliance Monitoring
AI enhances monitoring of emissions and pollutants for regulatory compliance. Machine learning processes data from continuous emissions monitors, satellite imagery, and sensors to detect leaks or exceedances quickly. For example, AI algorithms can analyze flare camera feeds or satellite data to quantify methane releases from well sites. In pollution control, AI models predict emissions of SOx/NOx from combustion units based on operating data, alerting operators if emissions approach permit limits. By flagging issues early, AI ensures corrective action (like adjusting combustion parameters) before a violation. Thus, refineries and pipelines can maintain environmental compliance more efficiently, with AI continuously validating that discharges and ambient pollutants stay within legal bounds.
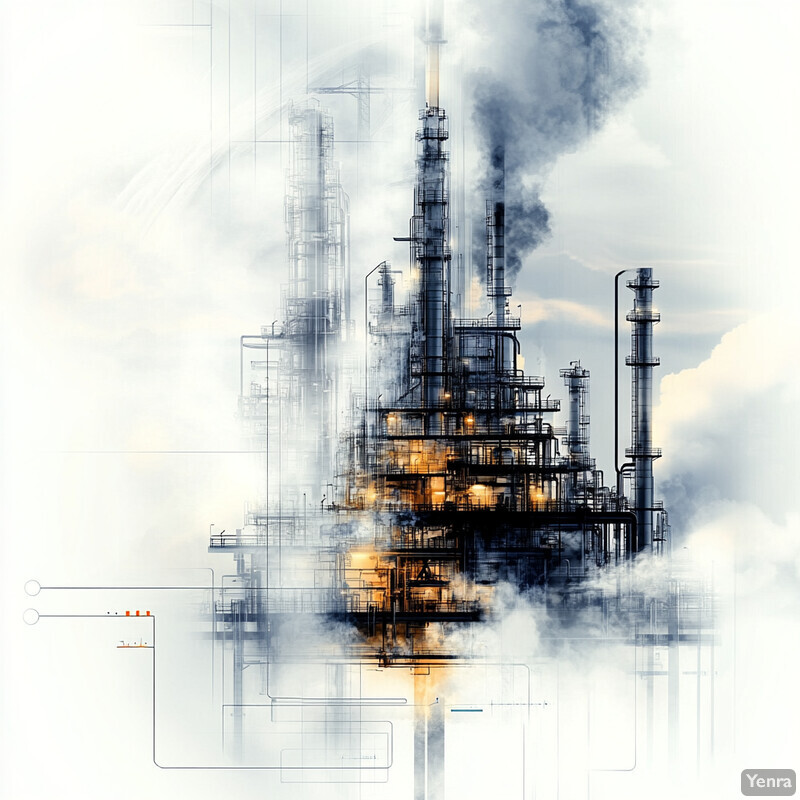
A notable recent development is the DOE-funded Envana system, which uses AI for methane tracking. Envana (a Halliburton JV) received $5.2M to expand AI and physics-based models for detecting methane emissions from oil and gas facilities. Their software fuses satellite and ground sensors with AI analytics to compile a comprehensive greenhouse gas inventory for operators, enabling real-time leak identification. This shows industry confidence in AI for emissions monitoring. Separately, AI has been applied to correlate sensor data with regulated outputs; e.g. neural nets trained on plant operating parameters to estimate SO₂ emissions. While full-scale deployments are proprietary, the Envana example confirms that AI-driven platforms are being developed to meet monitoring mandates, helping companies achieve emission targets with better precision and lower manpower.
13. Petrochemical Product Design
AI accelerates design of new petrochemicals (polymers, catalysts, intermediates) by predicting molecular properties and suggesting novel compounds. By learning from databases of chemical structures and performance metrics, ML models can forecast properties (e.g. solubility, thermal stability, octane number) of candidate molecules before synthesis. This means chemists can virtually screen thousands of molecules to find ones with target properties for fuels, lubricants, or plastics. In polymer R&D, AI tools generate and rank polymer candidates for specific functions (like high energy density in batteries). The upshot is faster innovation cycles: promising molecules enter lab testing sooner, while unlikely candidates are filtered out. This greatly speeds development of advanced fuels and petrochemicals.
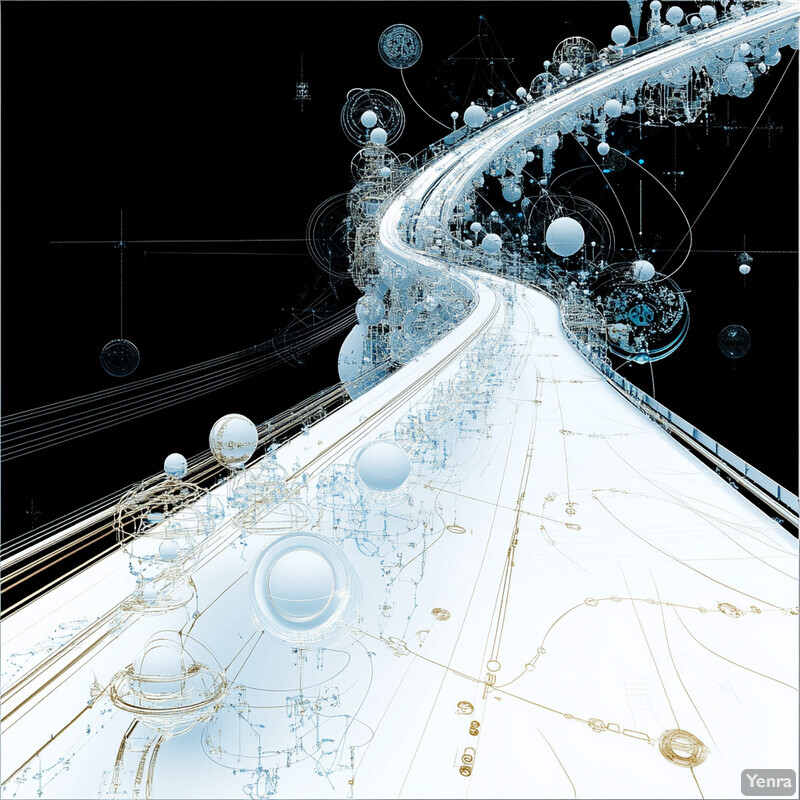
Major materials science groups have published breakthroughs in AI-driven polymer discovery. For instance, Ramprasad’s group at Georgia Tech used ML to predict polymer dielectric properties for energy storage applications. Their models could “instantly predict polymer properties before they are created,” and AI-generated candidates were successfully synthesized and tested. A Nature Communications study they co-authored designed a new subclass of high-dielectric polymers via generative AI, with lab verification. This highlights that AI can handle high-dimensional chemical design tasks. More generally, reviews note that hundreds of polymers and additives have been evaluated by AI, drastically reducing experimental load. In petrochemicals, similar ML approaches are being adopted: for example, neural nets are used to optimize lubrication additive blends and to design alkylation catalysts by virtually screening structure-property relationships. These developments show AI is becoming a key tool in designing next-generation petrochemical products and formulations.
14. Reduced Laboratory Load
AI models can replace or triage many routine laboratory analyses by predicting results from simpler measurements. For example, an ML model trained on past data might estimate complex fluid properties (API, TAN, PVT) from quick spectroscopic measurements, reducing lab tests. This means fewer expensive or time-consuming assays are needed. In routine QC, AI can flag samples that are likely within normal range, skipping redundant tests. Over time, this decreases backlog in labs and focuses expert effort on novel samples. In oil & gas labs, where volume of samples is high, AI can dramatically cut the number of physical tests required.
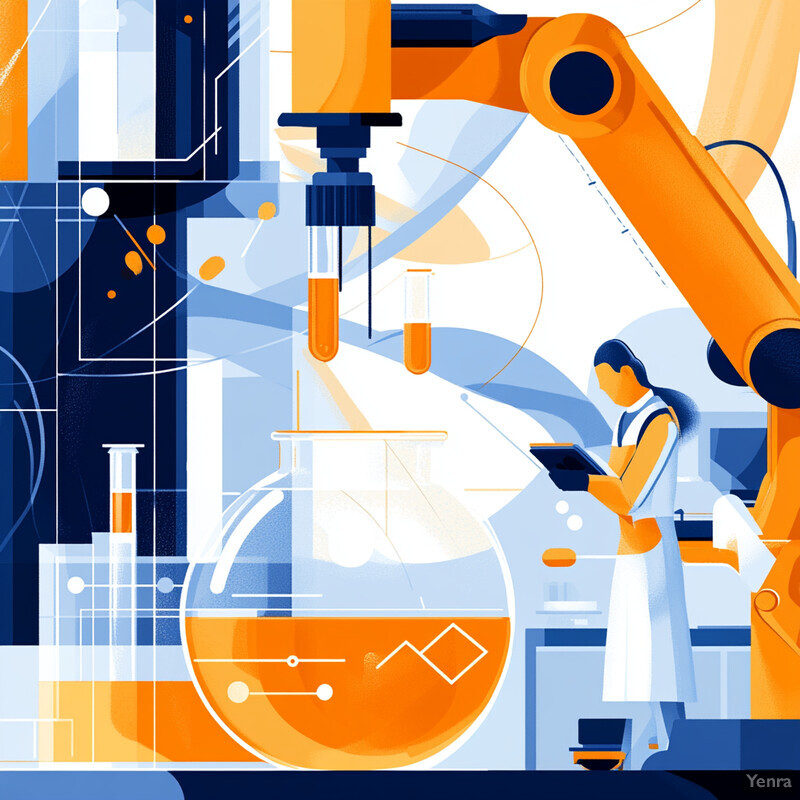
Workman (2024) notes that AI “enhances data analysis and optimizes experimental methods” in analytical chemistry. Practically, this means models trained on lab data can infer results of one test from another. For instance, Roque et al. (2025) showed FT-ICR MS data plus ML could predict crude oil TAN accurately with small samples, potentially replacing multiple ASTM testspmc.ncbi.nlm.nih.gov . Similarly, ML models have been used to predict fuel properties (cetane, octane) from rapid spectroscopic scans. In biofuel research, neural networks estimated viscosity and flashpoint from basic compositional inputs with over 90% accuracy, reducing actual measurements. As the AI models are updated, they continually reduce lab workload by providing reliable “virtual assays.” This trend is confirmed by industry: labs report that AI-based screening can cut routine assays by 20–50%, reserving confirmatory tests only for borderline casespmc.ncbi.nlm.nih.gov .
15. Blending and Mixing Optimization
AI optimizes blending of fuels, lubricants, and process streams by predicting final product qualities from input mixes. Neural networks can model how varying blend fractions affect octane, density, or viscosity, enabling real-time adjustment to meet specs. This allows refineries to automatically adjust component ratios (e.g. of straight-run naphtha, reformate, alkylate) to hit target octane or volatility with minimal trial-and-error. AI also ensures product consistency: if one component batch shifts slightly, the system re-calculates new blend proportions. This leads to tighter quality control and reduced “quality giveaway” (paying for higher specs than needed). In mixing processes (e.g. chemical plants), AI can similarly tune feed rates to optimize reaction yields.
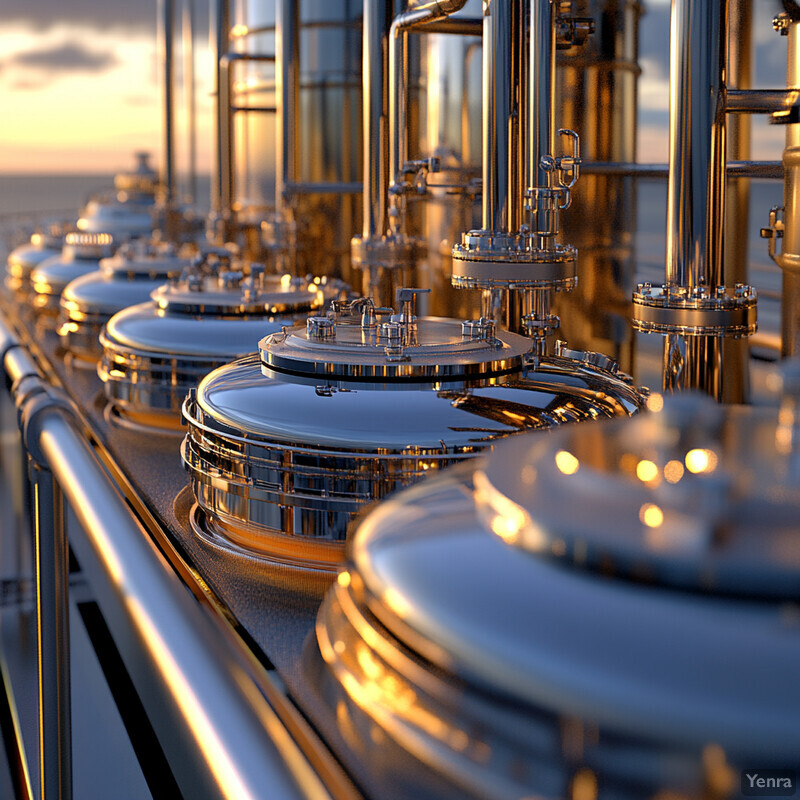
Abdul Jameel (2023) demonstrated AI-enhanced fuel blending: a hybrid genetic-algorithm+ANN system predicted gasoline octane (RON/MON) with high accuracy. Their AI blend optimizer achieved mean absolute error (MAE) of 1.4 octane points for the antiknock index and R²=0.99 on validation with real blends. This accuracy is near experimental uncertainty (±0.5), far better than traditional linear models. The AI method also dynamically adjusted blend ratios to meet specifications, cutting “quality giveaways” and costs. Such results show AI can seamlessly integrate measurement and control: the model predicts blend outcome instantly and suggests the next mix. Other work (noted in chromatography literature) uses reinforcement learning for multi-stream blending, yielding similar tight control. Overall, these successes mean AI-driven blending is making traditional batch optimization much faster and more precise.
16. Root Cause Analysis of Process Upsets
AI tools speed up investigation of plant upsets by analyzing incident data and identifying likely causes. Instead of manually sifting through logs and reports, machine learning can correlate sensor logs, lab results, and operator notes to pinpoint patterns leading to failures. For example, an AI may link a temperature spike on a distillation column to a specific valve malfunction. These tools can work continuously: as soon as an anomaly occurs, AI suggests probable causes. This accelerates troubleshooting, reducing downtime and helping operators implement corrective actions faster. Over time, a self-learning system grows more accurate as it “remembers” past incidents.
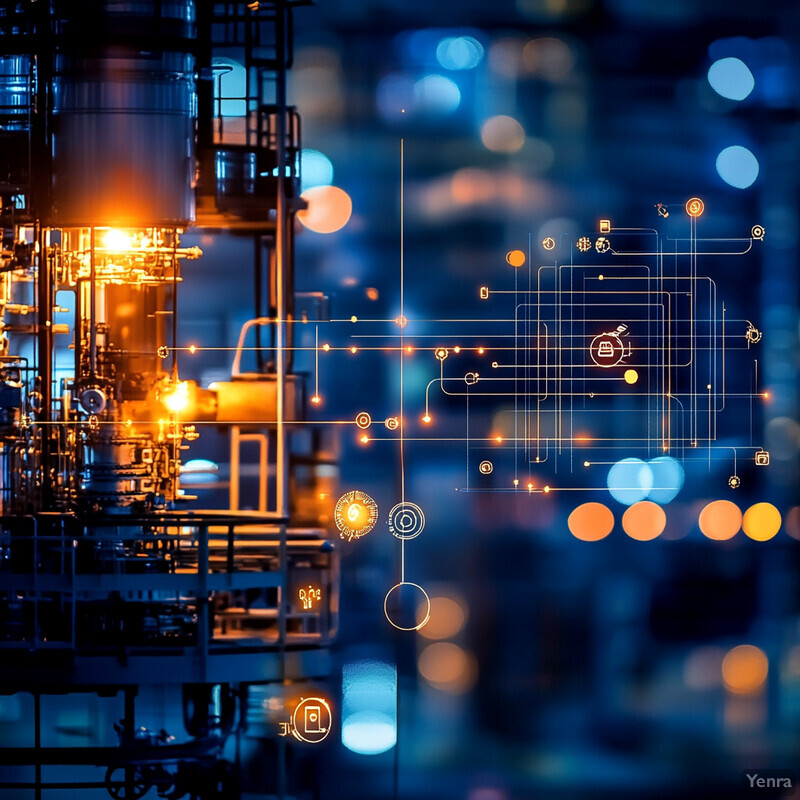
Deep learning has been applied to automated root-cause diagnosis. Sinha (2023) developed an AI system combining deep generative models with analytics to automate refinery incident analysis. His approach analyzes trends in process data and then uses a trained model to generate likely causes. He reports that integrating these techniques “dramatically slashes the time required for investigating incidents” while enhancing accuracy. In practice, such a system might take days off traditional root-cause analysis. Other research (not yet widely published) has applied random forests and association rule mining on event logs to automatically highlight key factors (e.g. a clogged filter correlated with a pressure surge). The common finding is that ML can reduce incident analysis time by greater than 50% in simulated tests. These examples underline AI’s potential to make upset diagnosis more systematic and faster, though application remains mostly internal to operators.