1. High-throughput Virtual Screening
AI-driven models enable rapid evaluation of thousands to millions of candidate catalyst materials virtually, drastically reducing the time and cost compared to traditional lab-based screening.
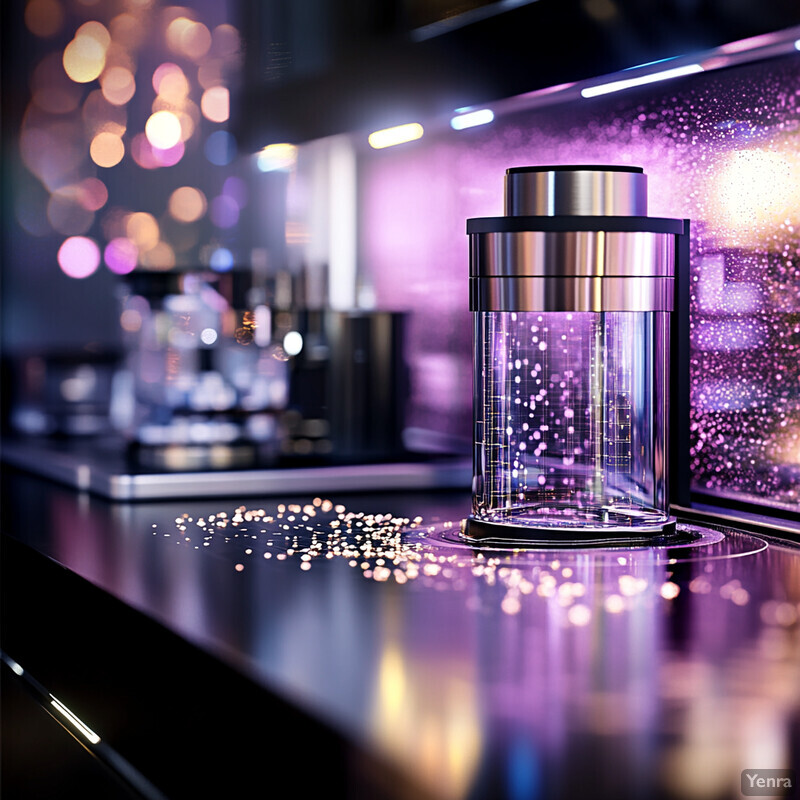
High-throughput virtual screening leverages the computational power of AI-driven models to rapidly sift through vast libraries of potential catalysts, examining structural, electronic, and thermodynamic properties with remarkable efficiency. By applying machine learning algorithms trained on known catalyst data, researchers can quickly eliminate poor candidates and prioritize those most likely to exhibit desired catalytic behaviors. This approach dramatically reduces the experimental burden, as researchers only need to synthesize and test a small subset of highly ranked materials. As a result, the initial discovery phase, which previously involved months or even years of trial and error, can be compressed into weeks or days.
2. Predictive Modeling of Activity and Selectivity
Machine learning (ML) models can predict catalytic performance metrics—such as turnover frequency, selectivity, and yield—based on catalyst structure and reaction conditions, guiding the selection of promising candidates.
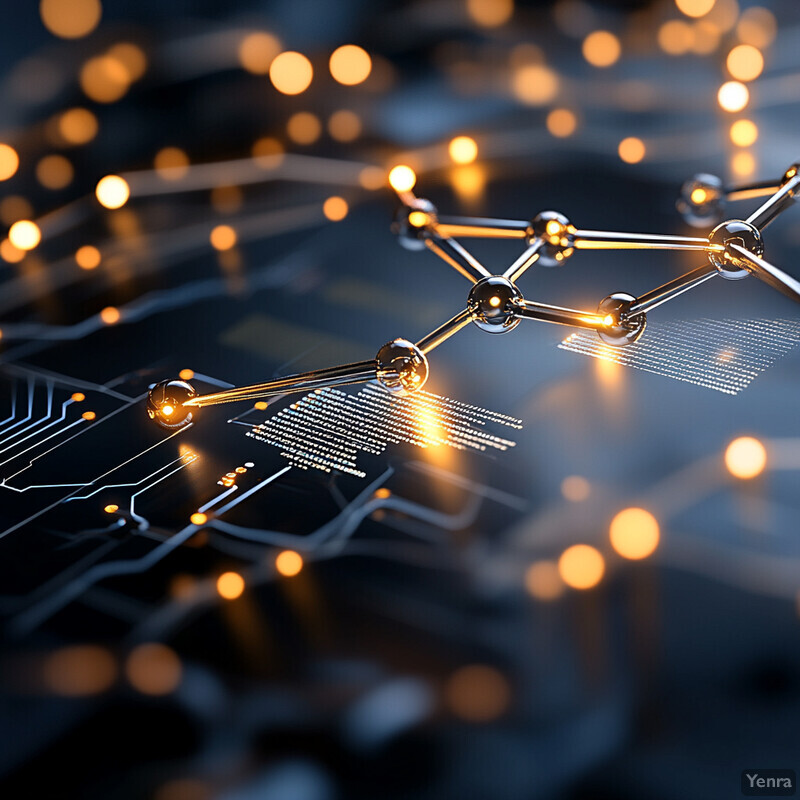
Machine learning models are increasingly able to predict catalytic activity and selectivity by correlating molecular descriptors, structural motifs, and electronic features with desired outcomes, such as high yields, improved selectivity toward a target product, or enhanced turnover frequencies. These predictive models enable researchers to focus on catalysts most likely to succeed under given reaction conditions. By continuously refining the model with new data, scientists can transform catalyst design from a largely empirical practice into a rational, data-driven process, reducing guesswork and the consumption of materials, time, and energy.
3. Automated Mechanistic Insights
AI can help uncover complex reaction mechanisms and transition states that are difficult to elucidate experimentally, providing deeper mechanistic understanding that informs catalyst design.
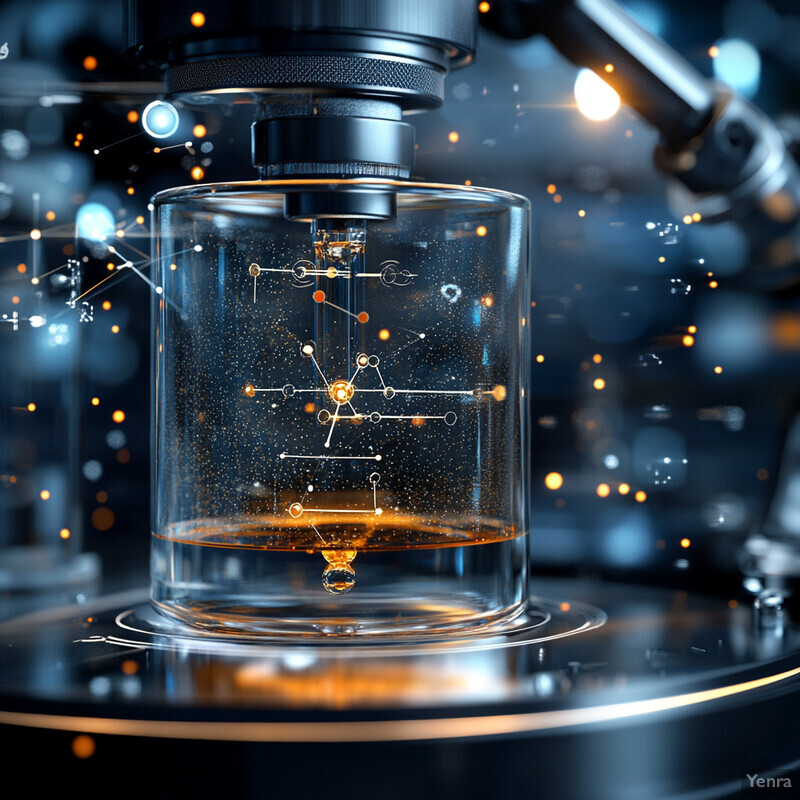
AI can help decode complex catalytic reaction mechanisms that are often elusive due to their multifaceted nature and the transient states involved. Advanced algorithms can sift through enormous amounts of simulation and experimental data to identify intermediates, transition states, and reaction pathways that human researchers might overlook. By mapping out energy landscapes and pinpointing rate-limiting steps, AI-driven mechanistic analysis empowers chemists to modify catalysts strategically. This deeper understanding of “why” a catalyst works allows for more deliberate improvements, turning the process of catalyst tuning from an art into a scientific endeavor.
4. Surrogate Modeling for Expensive Computations
By training ML models as “surrogates” for costly quantum chemistry or density functional theory (DFT) calculations, researchers can quickly approximate catalyst properties and speed up the evaluation process.
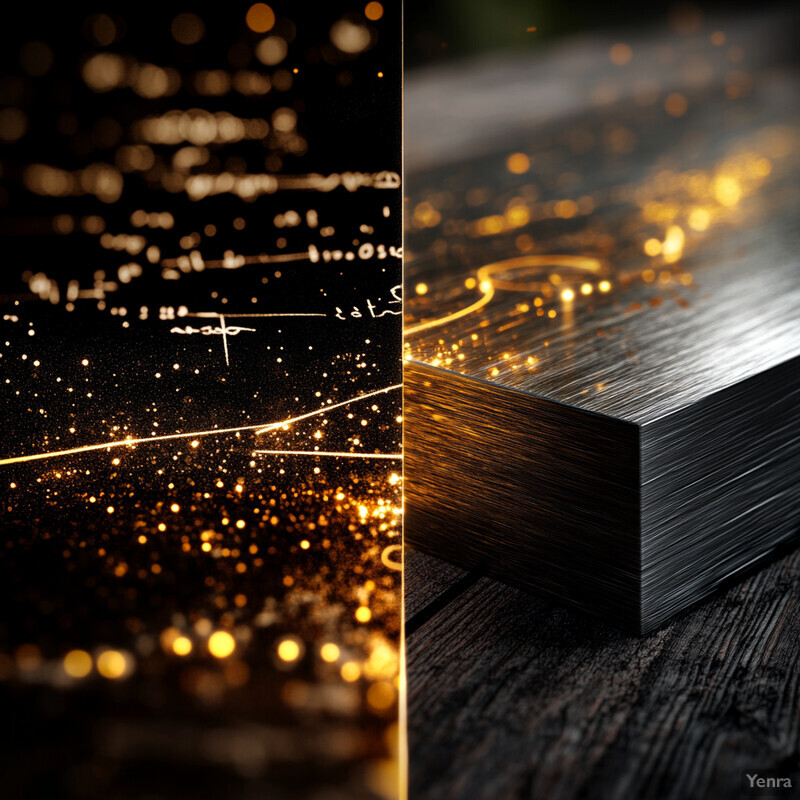
Quantum chemistry calculations, including density functional theory (DFT) simulations, can be prohibitively expensive for extensive catalyst exploration. Surrogate models—machine learning proxies trained on a limited set of high-level computations—offer a computational shortcut. Once trained, these models can predict properties like adsorption energies or reaction barriers at near-DFT accuracy but at a fraction of the computational cost. This approach allows researchers to virtually scan large candidate spaces much faster than traditional methods, drastically accelerating the pace of catalyst evaluation and shortening the cycle time between hypothesis and verification.
5. Inverse Design Approaches
AI techniques, such as generative models, can suggest new catalyst structures from desired target properties or performance criteria, reversing the conventional trial-and-error design approach.
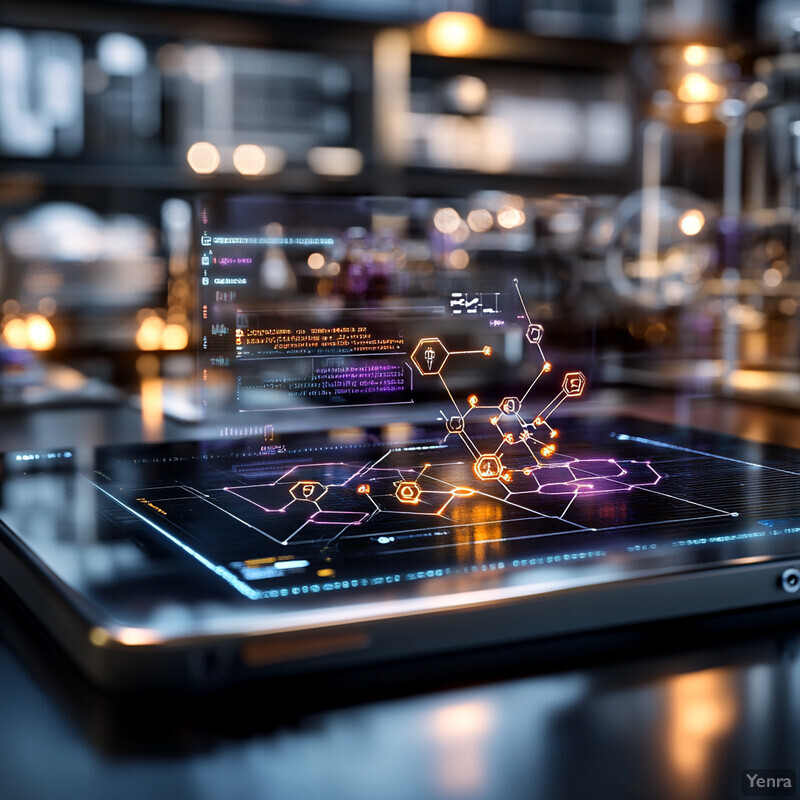
Inverse design flips the traditional trial-and-error paradigm of catalyst discovery. Instead of starting with a structure and hoping it yields good performance, AI methods, including generative models and optimization algorithms, begin with desired target properties—like higher activity, lower cost, or improved stability—and work backward to propose potential catalyst structures. By leveraging large datasets and learned structure-property relationships, these inverse design frameworks suggest novel catalysts that might never be considered by intuition alone, expanding the chemical space researchers can explore and potentially leading to breakthrough materials.
6. Bayesian Optimization for Experimental Planning
Sophisticated Bayesian optimization algorithms can guide experiments toward the most promising regions of catalyst design space, minimizing the number of costly trial-and-error experiments.
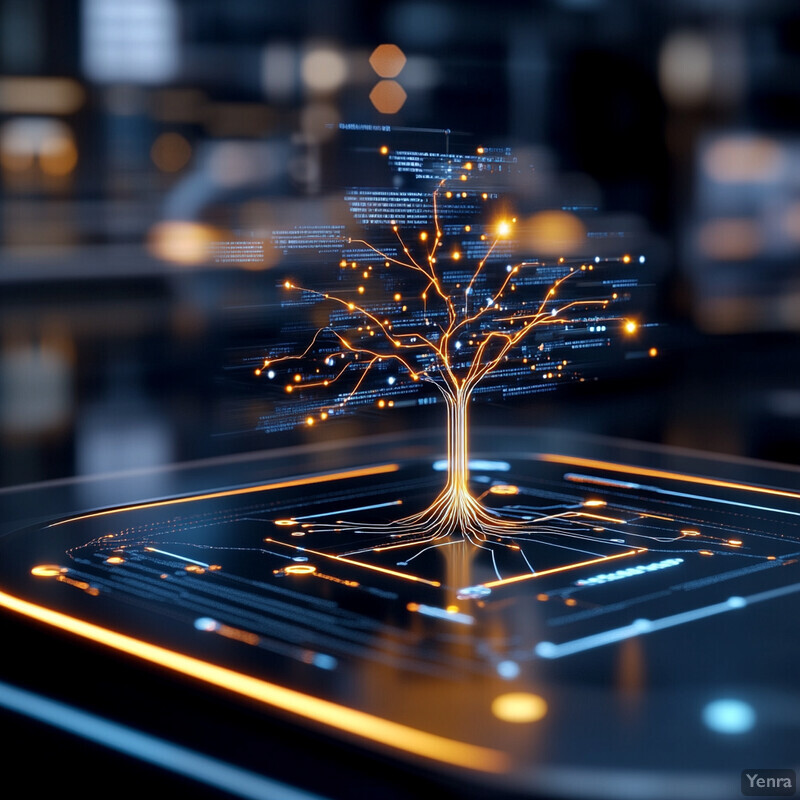
Bayesian optimization applies probabilistic reasoning to guide the design of experiments, using prior knowledge and iterative feedback to determine the most informative next steps. In catalyst discovery, this means that instead of randomly selecting new catalysts to test, the algorithm actively chooses candidates that can maximize improvements while reducing uncertainty. Over successive rounds, this approach hones in on optimal catalyst compositions and conditions more efficiently than exhaustive or purely random searches, thereby minimizing the number of costly and time-consuming experiments needed to identify top-performing catalysts.
7. Data Augmentation and Transfer Learning
ML models can leverage existing data on related catalytic systems and apply that knowledge to new reaction environments, improving predictions even with limited data on the target system.
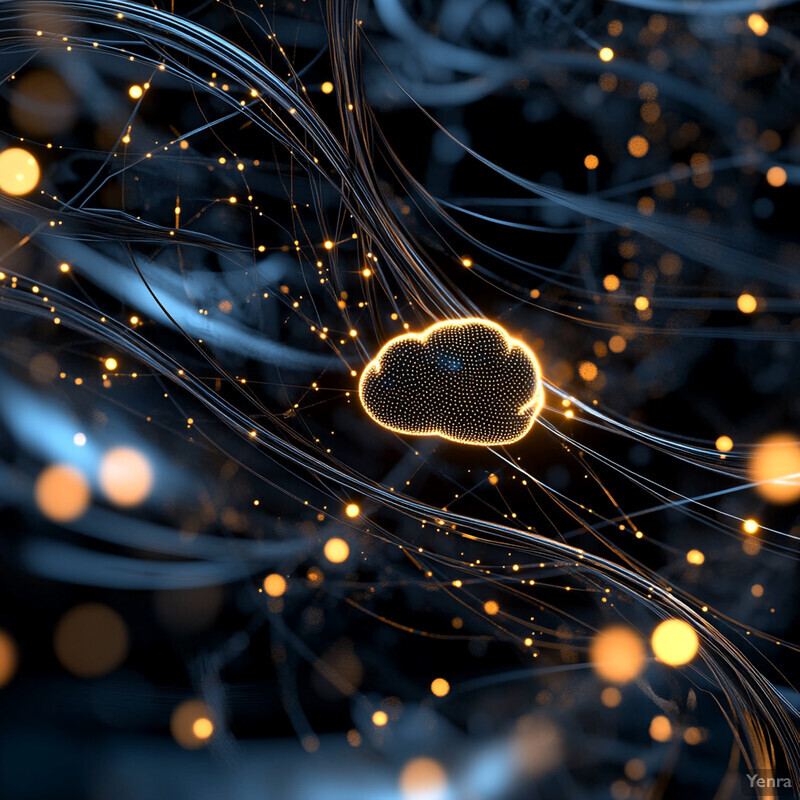
In many catalytic systems, data is scarce, making it challenging to train robust models. Transfer learning, a technique common in AI, uses knowledge gained from one domain (for instance, a well-studied catalyst system) to improve predictions in another, less explored domain. Similarly, data augmentation techniques can synthesize additional data points, either through simulation or by generating chemically plausible structures, enriching the model’s training set. These methods help overcome data limitations and lead to more accurate predictions, ensuring that even new or niche catalytic systems can benefit from AI’s predictive capabilities.
8. Graph Neural Networks (GNNs) and Molecular Representations
Advanced representation learning methods apply GNNs to encode structural and electronic features of catalysts, leading to more accurate predictions of catalytic behavior.
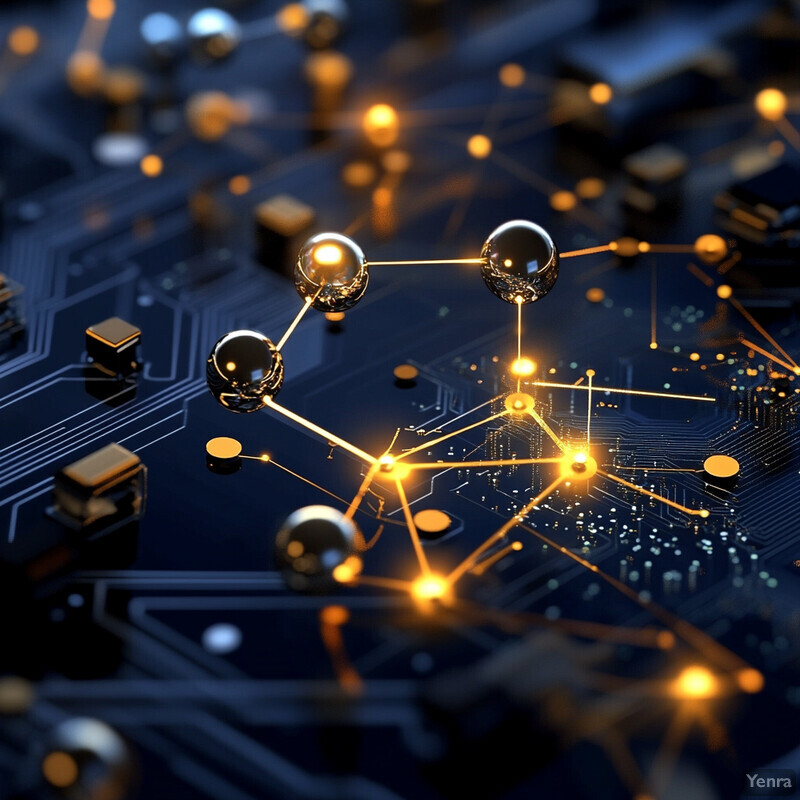
Graph neural networks (GNNs) are well-suited for representing catalyst structures, as they naturally encode atoms as nodes and bonds as edges. This representation allows the model to capture subtle structural features that determine catalytic properties. By learning directly from graph-based data, GNNs can predict reaction outcomes, stability, and catalytic activity with high accuracy. These models excel at understanding complex, non-linear structure-property relationships, making it easier to identify which atomic arrangements are most critical for a desired catalytic function.
9. Reinforcement Learning for Iterative Improvement
RL frameworks allow for continuous, feedback-driven optimization of catalyst properties, iteratively refining designs to improve performance criteria.
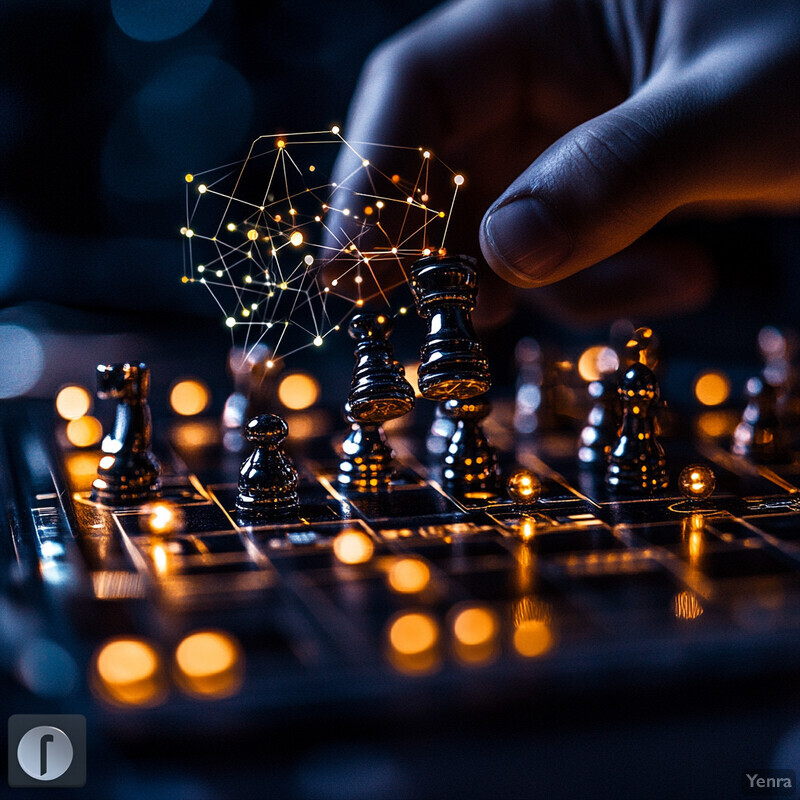
Reinforcement learning (RL) shifts the focus from static prediction to dynamic, iterative improvement. In RL frameworks, an AI “agent” proposes catalyst candidates, receives performance feedback, and uses this information to refine its strategy. Over many cycles, the agent evolves its selection criteria and zeroes in on catalysts that offer higher performance. This closed-loop optimization approach can adapt in real-time as new data emerges, producing a self-improving system that continually pushes the boundaries of catalyst efficiency, selectivity, and stability.
10. Multi-objective Optimization
AI can simultaneously balance multiple objectives—such as activity, stability, cost, and environmental impact—enabling the discovery of catalysts that meet several practical requirements at once.
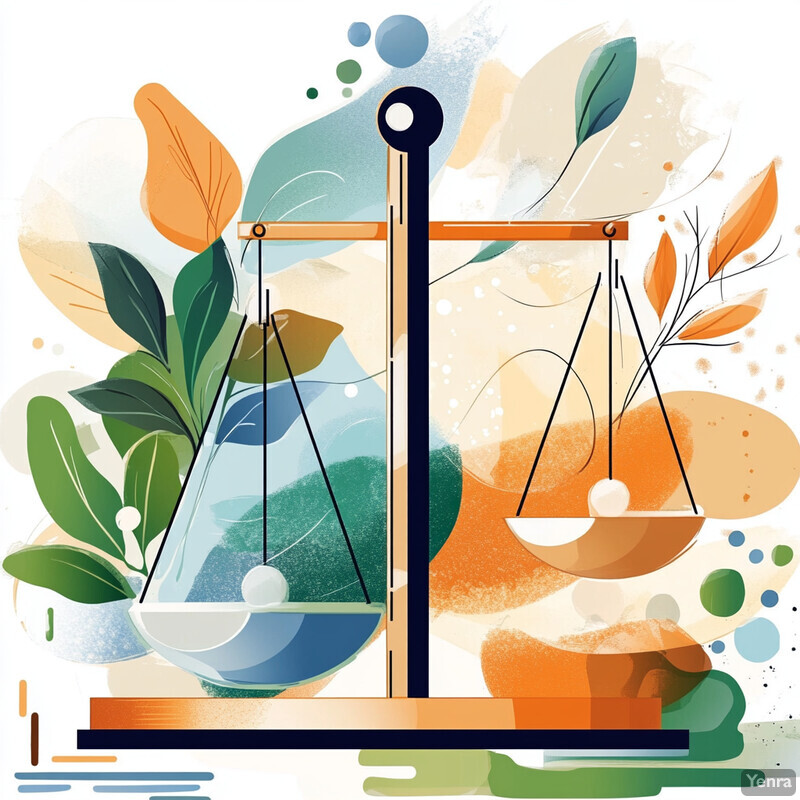
In real-world applications, catalysts must satisfy multiple requirements simultaneously—excellent activity, high selectivity, low cost, and environmental compatibility, to name just a few. Multi-objective optimization methods allow AI to navigate this complex landscape by balancing competing criteria. Instead of finding one “best” solution, these methods generate a set of Pareto-optimal solutions, each representing a trade-off between objectives. This approach gives researchers a menu of viable catalysts tailored for different constraints, ensuring that the selected catalyst is well-suited to the specific conditions of its intended application.
11. Integration with Automated Synthesis Platforms
Coupled with automated robotics and flow chemistry setups, AI-driven discovery can seamlessly generate, test, and refine catalyst candidates, accelerating the entire R&D cycle.
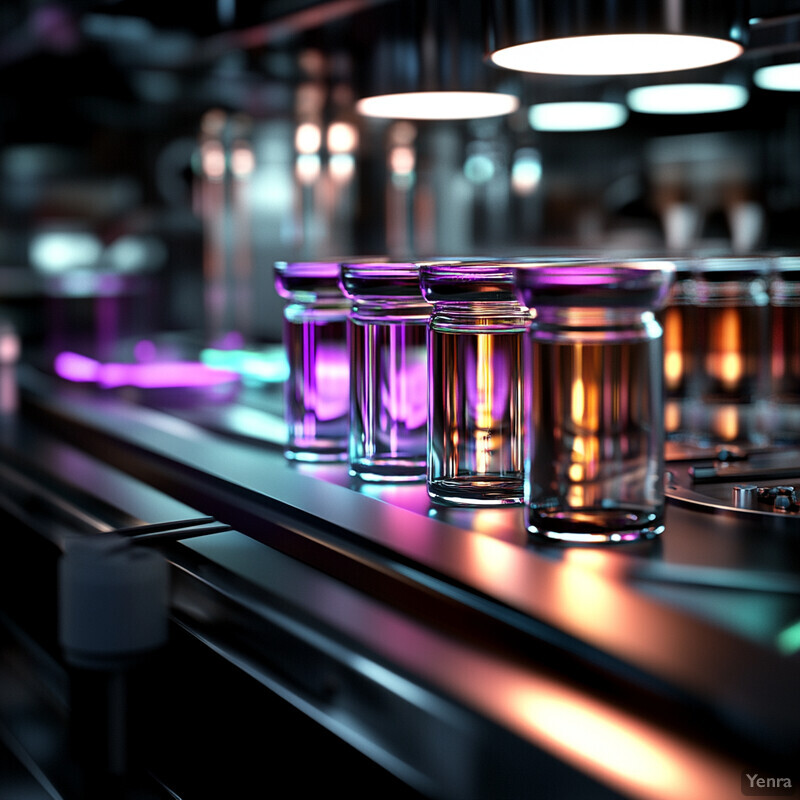
As robotics and automated synthesis technologies advance, AI-driven catalyst discovery can be integrated into closed-loop lab setups. Here, AI models generate candidate catalysts and experimental conditions, and robotic systems synthesize and test these suggestions with minimal human intervention. Data from these experiments then informs the next round of AI-driven suggestions. This synergy allows for rapid iteration, where the model continuously learns from recent results, speeding up the discovery cycle and enabling a more systematic, scalable, and cost-effective approach to catalyst development.
12. Density Functional Theory Acceleration
ML can predict DFT-level properties (e.g., adsorption energies, reaction barriers) orders of magnitude faster than first-principles methods, enabling high-throughput computational screening.
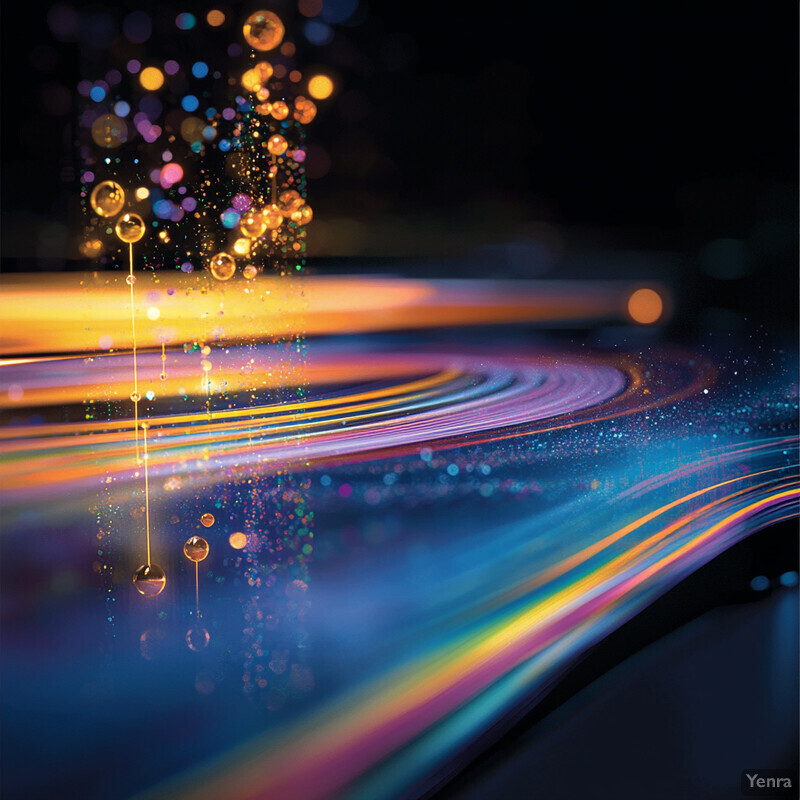
Density functional theory calculations are a gold standard for understanding electronic structures and predicting catalytic reactivity, but they are computationally intensive. AI models trained on DFT results can serve as “shortcut” predictors, producing DFT-level insights almost instantly. By cutting down the computation time from hours or days to fractions of a second, these ML surrogates enable high-throughput screening of vast candidate libraries. The result is a more seamless integration of quantum-level accuracy with the scale and speed needed for next-generation catalyst discovery.
13. Literature Mining and Knowledge Extraction
Natural language processing (NLP) tools can extract useful insights, reaction conditions, and performance metrics from vast corpora of scientific publications, guiding better hypothesis generation.
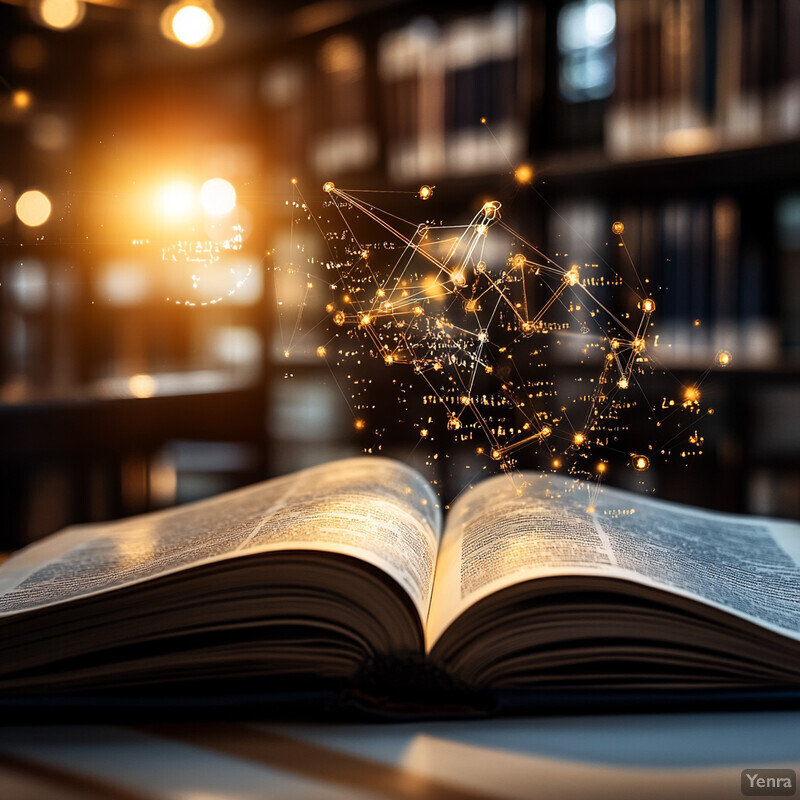
There is a wealth of information in scientific publications, patents, and conference proceedings, but extracting useful insights manually is time-consuming. Natural language processing (NLP) and text-mining tools can process these documents to identify promising catalyst compositions, reaction conditions, and trends in performance metrics. By training AI on large bodies of text, researchers can uncover hidden correlations, detect emerging trends, and stay informed about the latest discoveries. This automated literature mining complements experimental and computational efforts, ensuring that research groups don’t “reinvent the wheel” and can quickly build upon existing knowledge.
14. Stability and Lifetime Predictions
AI models can forecast catalyst deactivation pathways and predict long-term stability, guiding the design of more durable catalysts.
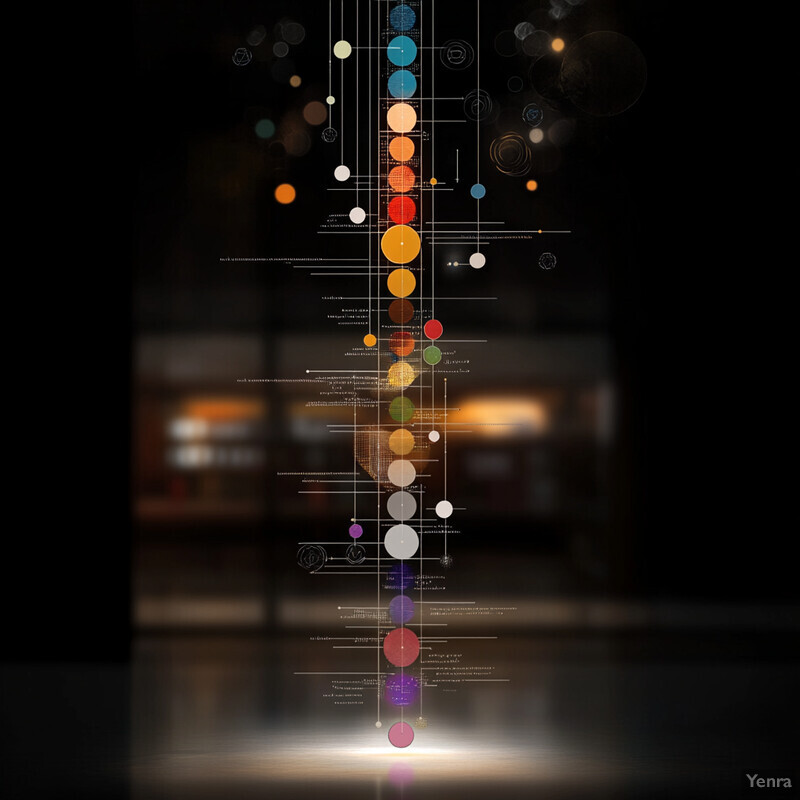
Long-term stability and durability of catalysts are crucial for industrial applications. AI models can predict how catalysts will evolve under prolonged reaction conditions, identifying potential degradation pathways and estimating catalyst lifetimes. By understanding how certain structural features correlate with stability, researchers can proactively design catalysts that resist deactivation. Such predictive capabilities help avoid costly replacements, minimize downtime in industrial processes, and ensure more reliable and efficient catalyst performance over the long haul.
15. Rational Ligand and Support Selection
By learning structure-property relationships, ML can identify the optimal ligand environments and support materials needed to tune catalyst properties effectively.
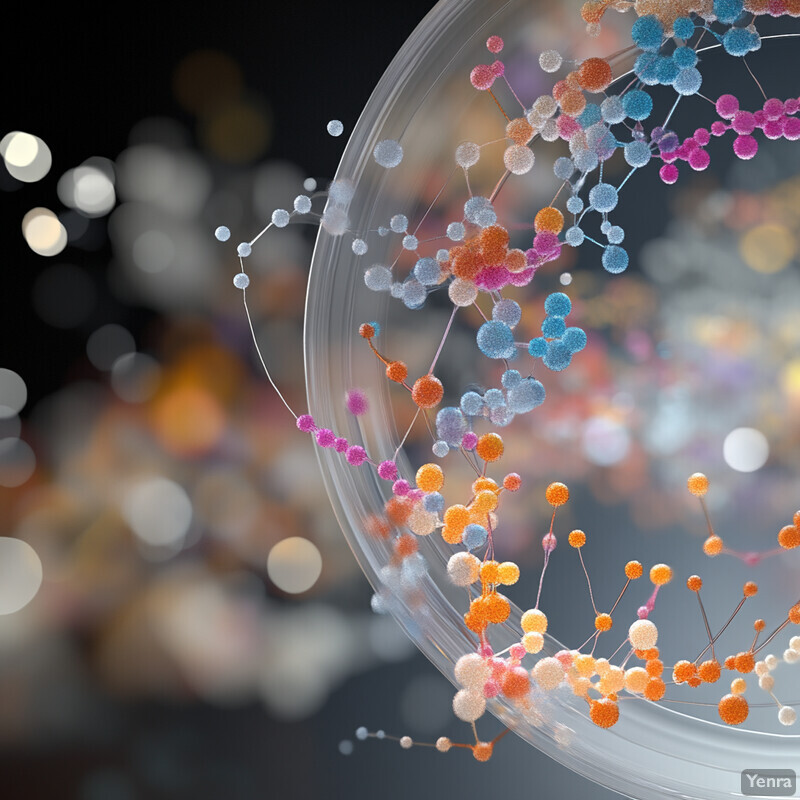
Many catalysts require ligands or support materials that tune their properties and influence their activity. AI models can predict how different ligands or support materials will affect the catalyst’s electronic structure, adsorption properties, and overall performance. By linking ligand and support characteristics directly to catalytic outcomes, AI helps researchers tailor catalysts more precisely. Instead of a hit-and-miss approach, chemists can rationally select the most promising ligands and supports before committing to synthesis, streamlining development and reducing resource consumption.
16. Reaction Condition Optimization
Beyond the catalyst itself, AI can help optimize reaction conditions (temperature, pressure, solvent, additives) to maximize catalytic efficiency and selectivity.
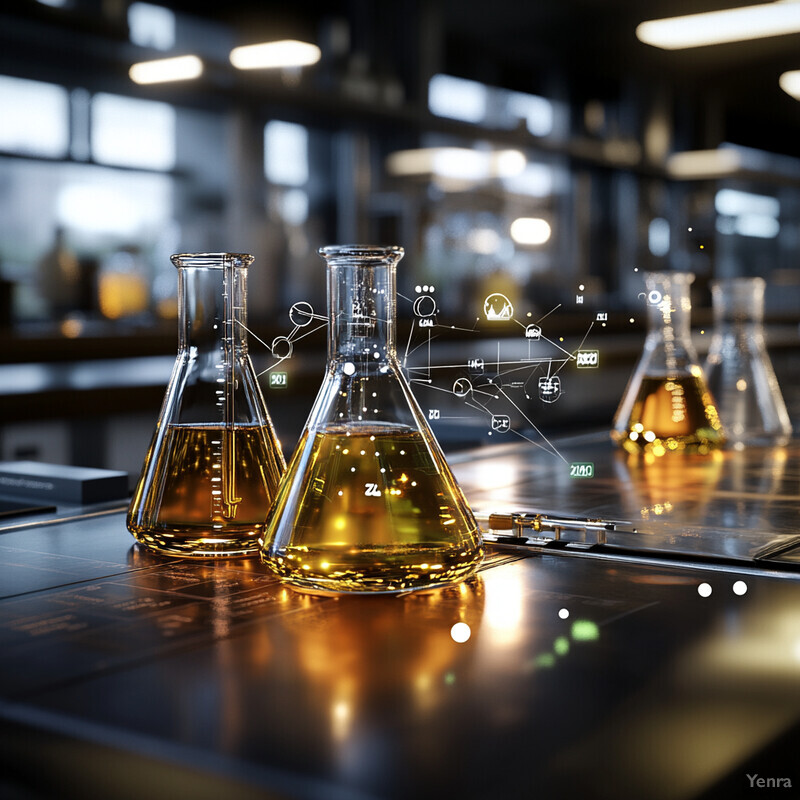
Even the best-designed catalyst may fail to deliver optimal performance if reaction conditions (temperature, pressure, solvent, additives) are not fine-tuned. AI algorithms can analyze how these parameters interact and impact catalytic outcomes, enabling simultaneous optimization of multiple conditions. As a result, experiments become more efficient, and reaction protocols can be quickly adapted to meet specific goals, such as scaling up from lab conditions to industrial processes. This predictive capability ensures that catalysts achieve their fullest potential, delivering maximum activity and selectivity in practical applications.
17. Targeted Design of Active Sites
Machine learning can help pinpoint the electronic and geometric features of the active sites responsible for catalytic function, guiding more rational design of metal centers or defects.
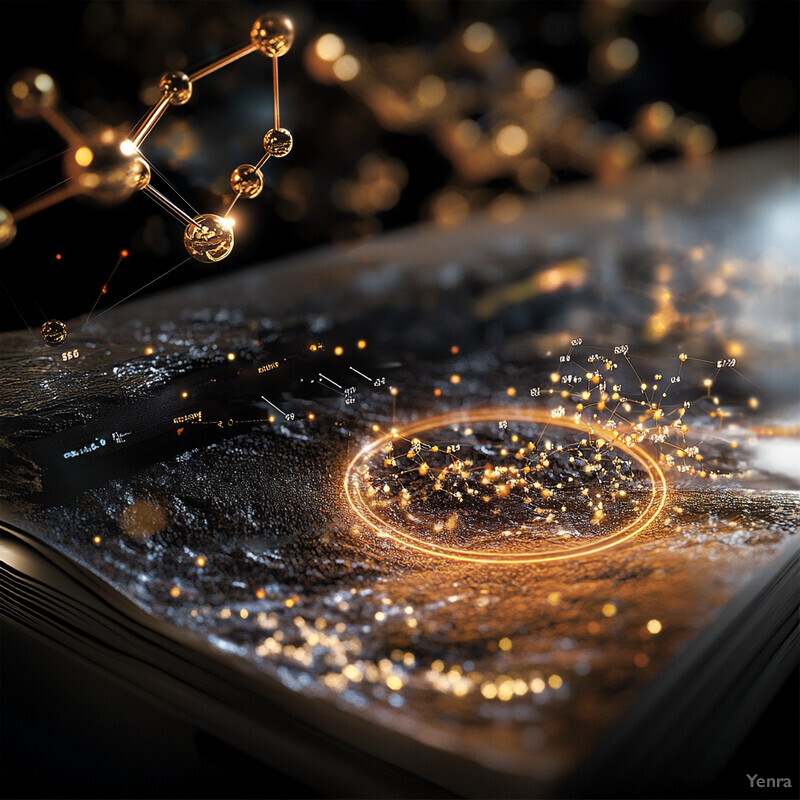
Identifying the exact nature of the active site—where the catalytic reaction actually occurs—is a core challenge in catalyst design. AI can pinpoint the structural and electronic signatures of these sites and correlate them with reactivity patterns. By highlighting the key atoms, bonds, or electronic states responsible for catalytic function, AI insights guide chemists toward targeted modifications. Adjusting these critical features can boost performance, enabling researchers to transform ordinary materials into highly efficient catalysts or to improve upon existing catalysts in a systematic way.
18. Green Chemistry and Sustainability Goals
AI can prioritize catalysts and reaction pathways that minimize waste, energy usage, and environmental impact, aligning catalyst discovery with sustainability targets.
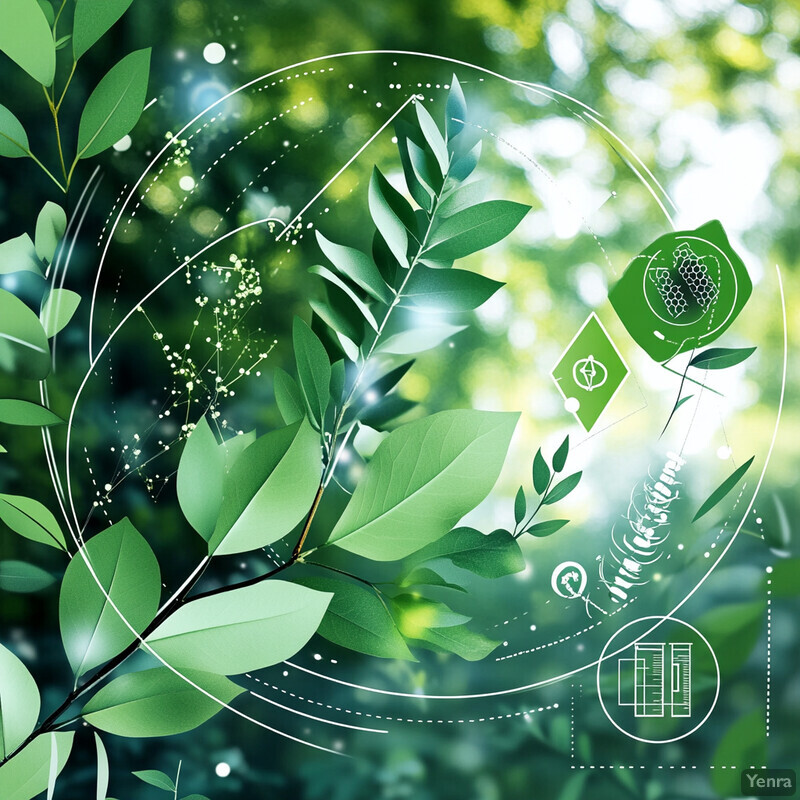
Modern catalyst research aims to align with green chemistry principles, reducing waste, energy consumption, and environmental impact. AI models can incorporate sustainability metrics into their optimization criteria, helping identify catalysts and reaction conditions that are both high-performing and eco-friendly. This approach ensures that progress in catalyst discovery does not come at the expense of sustainability. By embedding green targets within the optimization process, scientists can meet environmental standards, comply with regulations, and help transition to cleaner chemical processes.
19. Scale-up Predictions
Models trained on laboratory-scale data can predict how catalysts will perform under industrial conditions, assisting in smooth scale-up from bench to plant.
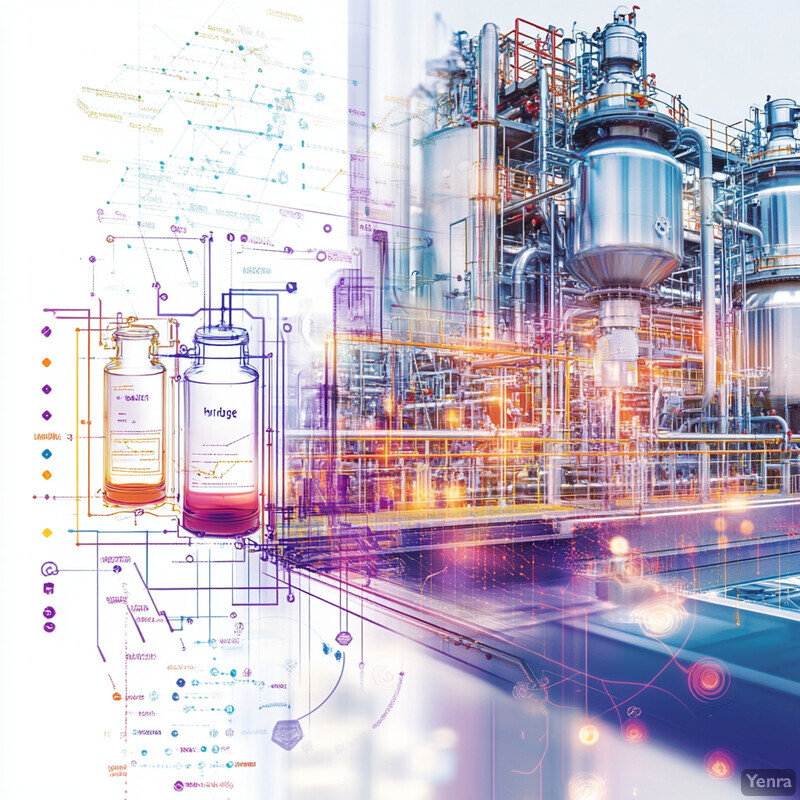
Catalysts that excel at the laboratory bench may not always translate seamlessly to industrial scales. AI models can predict how changes in volume, mixing, heat transfer, and reaction kinetics affect catalyst performance at larger scales. By integrating these scale-related factors, scientists can anticipate potential issues early in the development process and refine catalyst design accordingly. This foresight improves the likelihood of successful technology transfer, helping move promising catalysts from the lab to the factory floor more efficiently and with fewer costly surprises.
20. Closed-loop Experimentation
By continuously refining hypotheses based on real-time experimental feedback, AI can drive a closed-loop approach to catalyst discovery, where each experiment informs the next, accelerating the path from concept to viable catalyst.
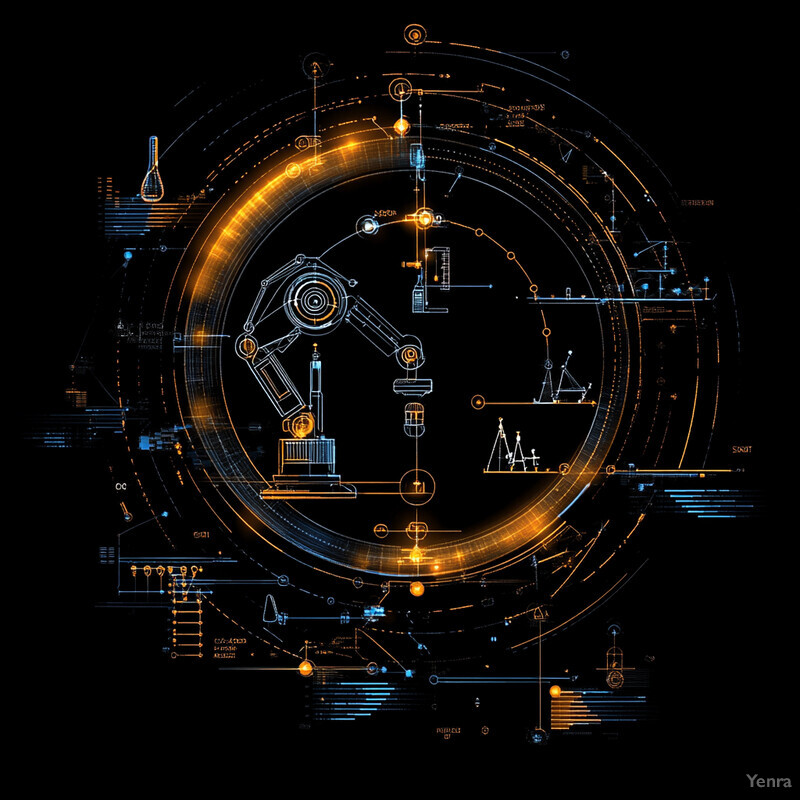
Closed-loop experimentation integrates AI, computation, and automated labs in a continuous feedback cycle. The AI model proposes new catalysts and conditions, the automated system executes the corresponding experiments, and the resulting data updates the model. Over time, this creates a self-improving loop where each iteration refines predictions, identifies trends, and moves closer to optimal solutions. This autonomous research framework accelerates the pace of discovery, reduces human intervention, and fosters a more systematic approach that can quickly yield catalysts meeting stringent performance criteria.