1. Predictive Demand Forecasting
AI-driven models analyze historical rides, seasonal trends, local events, and weather patterns to accurately predict when and where ride requests will spike, enabling better resource allocation.
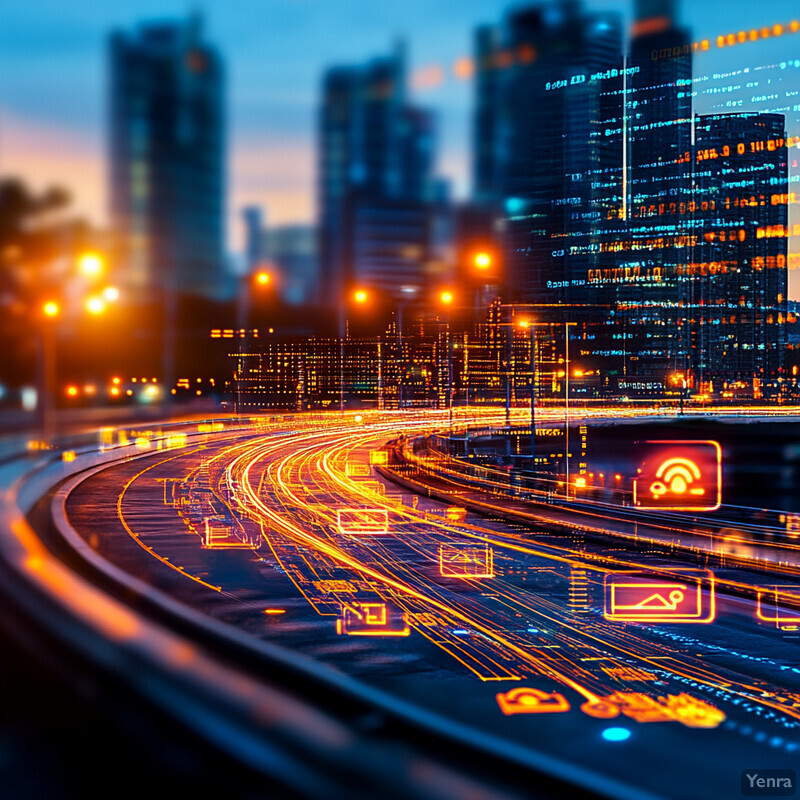
AI-powered predictive demand forecasting involves training machine learning models on historical ride request data, weather information, local event schedules, and seasonal trends to anticipate future rider demand. By analyzing these data points, the system can determine when, where, and how frequently ride requests will occur. This enables fleet managers to strategically position drivers in locations and at times most likely to yield rides, reducing idle time and enhancing reliability. With better forecasting, ridesharing services can ensure quicker pickup times, minimized wait periods, and a more consistent level of service, ultimately improving overall rider satisfaction and operational efficiency.
2. Dynamic Route Optimization
Advanced route-planning algorithms use real-time traffic data, road closures, and congestion levels to constantly update and optimize drivers’ paths, reducing travel time and improving fuel efficiency.
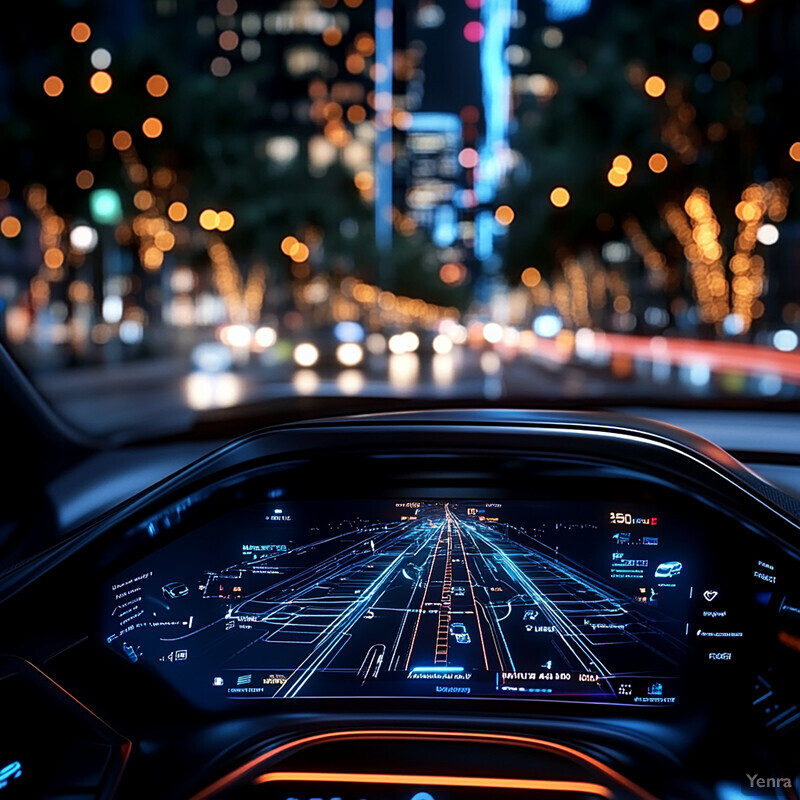
Dynamic route optimization leverages real-time data streams—such as traffic conditions, construction updates, accidents, and GPS signals—to constantly refine the travel path of a ridesharing vehicle. Advanced AI algorithms consider various factors like shortest possible routes, road closures, and congestion patterns to recommend the most efficient journey. This continuous recalibration ensures that drivers spend less time stuck in traffic, conserve fuel, and save on operational costs, while passengers benefit from reduced travel times. As the system learns from past trips and conditions, its routing suggestions become increasingly accurate and adaptive, providing a smoother, more reliable commuting experience.
3. Multi-Rider Matching Algorithms
Intelligent matching systems group passengers heading along similar routes, balancing ride pooling preferences with minimal detours, increasing seat utilization and cutting operating costs.
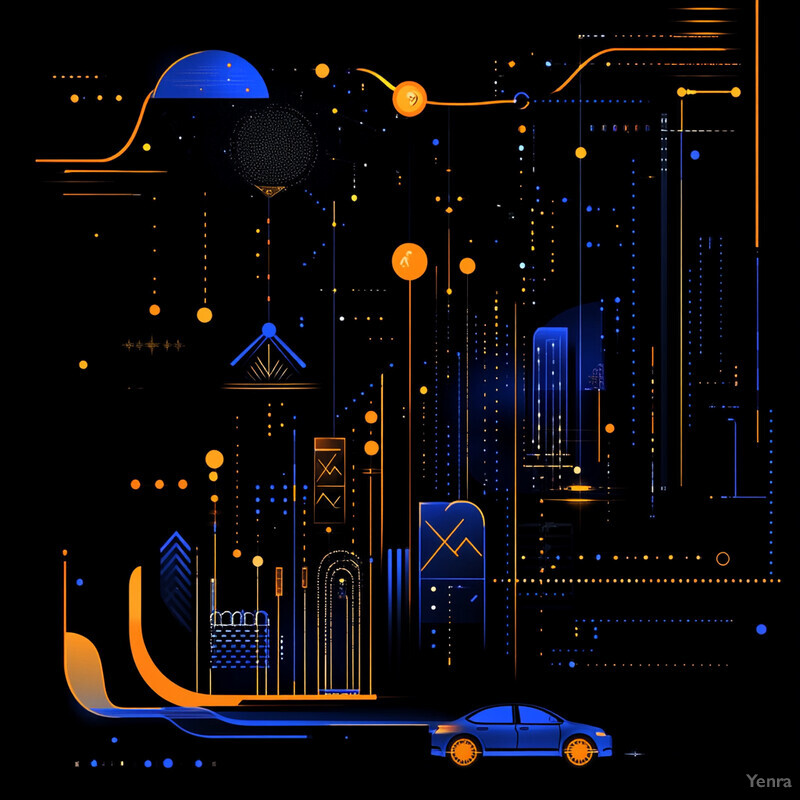
AI-driven matching algorithms analyze passenger locations, destinations, and time constraints to group riders into shared trips that minimize detours. These algorithms consider compatibility factors such as travel direction, pickup feasibility, and timing windows, ensuring that the combined journey remains efficient for everyone involved. By increasing the average occupancy per trip, these solutions reduce the cost per passenger, alleviate congestion, and lower emissions. Over time, as the matching model learns from user feedback and historical outcomes, the service can refine pairing logic to improve comfort, convenience, and fairness, ultimately delivering a more appealing ridesharing experience.
4. AI-Based Dispatching
Sophisticated dispatch engines leverage machine learning to select the ideal driver for each trip based on proximity, vehicle type, driver performance, and customer preferences.
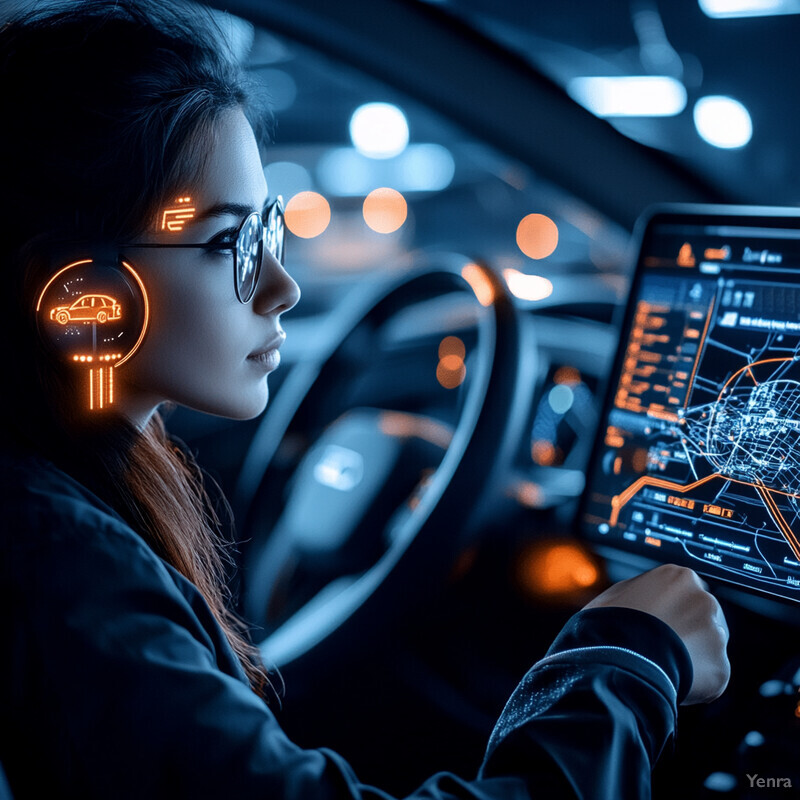
Sophisticated AI-based dispatch systems assign rides to the ideal driver for each request, considering multiple criteria beyond simple proximity. The algorithms assess a driver’s historical performance, trip acceptance rates, vehicle type, current location, and even driving style. By selecting the most suitable driver for a given request, the system balances supply and demand more efficiently, ensuring faster pickups and higher rider satisfaction. Over time, these dispatching systems learn the nuanced preferences of regions, specific routes, and user cohorts, further refining their decision-making to continually improve the passenger experience and operational fluidity.
5. Dynamic Pricing Strategies
Machine learning models set fares that reflect real-time demand, driver supply, and competing services, ensuring balanced pricing that attracts riders while incentivizing drivers.
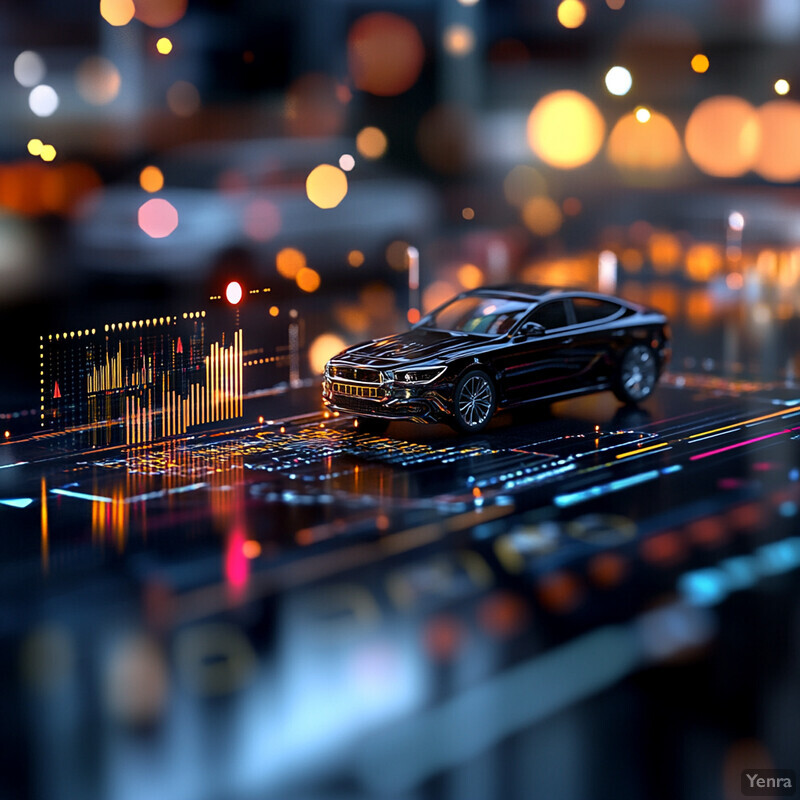
AI-driven dynamic pricing uses machine learning models to intelligently set fares in real time, reflecting current market conditions, local demand surges, driver availability, competitive offerings, and special events. By constantly adjusting prices, the system prevents severe imbalances in supply and demand, attracting more drivers to areas of high need, and encouraging riders to schedule travel during off-peak times. Dynamic pricing optimizes revenue for the service provider and ensures that fares remain fair and responsive to changing conditions. Ultimately, this approach fosters a stable ecosystem, where both drivers and riders benefit from balanced, transparent pricing that aligns with real-time operational realities.
6. Demand-Supply Rebalancing
AI tools suggest areas where drivers should position themselves to meet upcoming demand, reducing idle time and improving rider wait times.
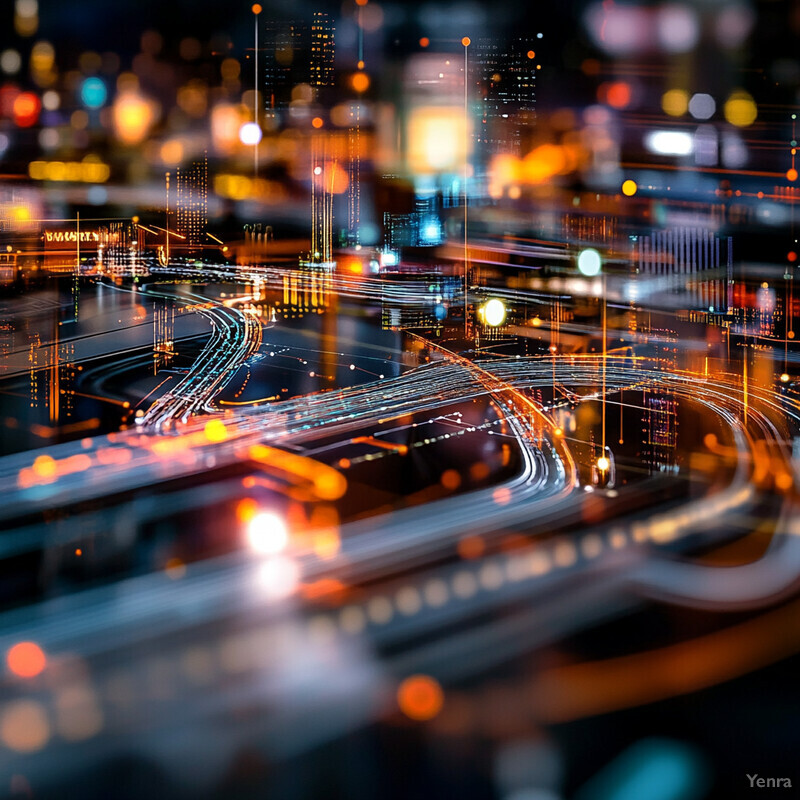
Demand-supply rebalancing models proactively guide drivers to regions that are expected to experience an uptick in ride requests. By analyzing historical data, current booking trends, traffic conditions, and external factors such as events or weather shifts, AI tools can preemptively position drivers before demand actually peaks. As a result, riders find available cars faster, reducing wait times and improving service reliability. This strategic maneuvering also lessens driver downtime and idle cruising, cutting operational expenses and benefiting the environment through decreased fuel consumption and emissions.
7. Traffic Pattern Recognition
Neural networks continuously learn traffic patterns, identifying the best times and routes for ridesharing vehicles to travel, minimizing delays and improving on-time performance.
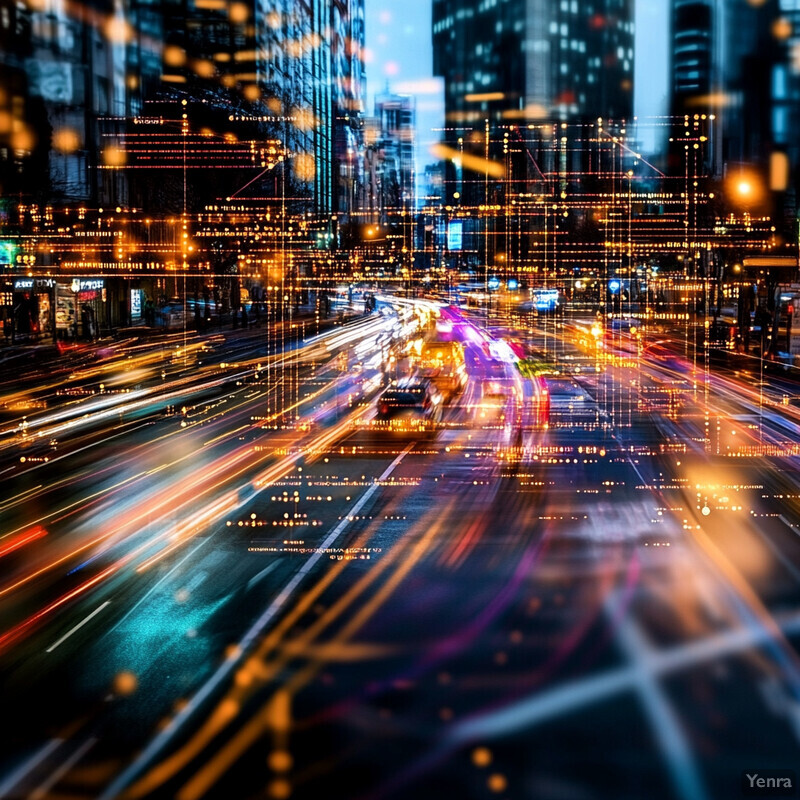
Through continuous analysis of large datasets, AI identifies and learns from recurring traffic patterns—morning rush hour congestion, midday lulls, weekend events, or sports matches that impact local flow. Armed with this intelligence, the system can guide drivers toward paths that consistently offer smoother passage. By anticipating rather than just reacting to traffic conditions, ridesharing services can deliver faster, more predictable travel experiences. Over time, the AI refines its understanding of these patterns, allowing it to accurately forecast future traffic scenarios, resulting in enhanced efficiency and rider satisfaction.
8. Improved ETA Accuracy
By ingesting live GPS data, historical averages, and situational factors, AI delivers highly accurate arrival time predictions, improving rider trust and trip planning.
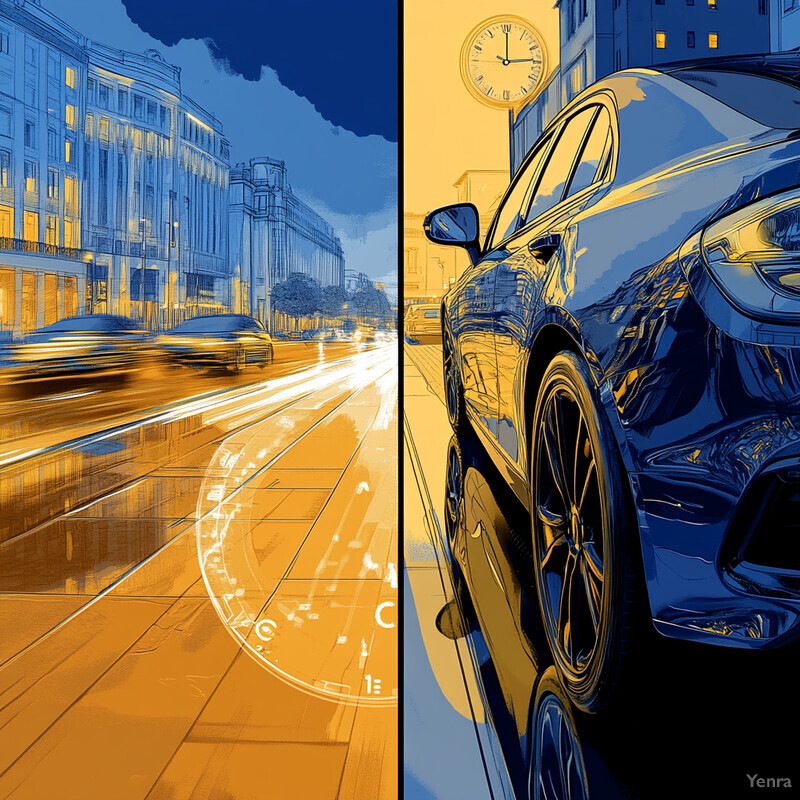
Precise Estimated Time of Arrival (ETA) predictions rely on machine learning models that integrate route length, current and predicted traffic congestion, driver speed behavior, and historical averages. With every trip completed, the system gathers new data to refine future estimates. Accurate ETAs build trust, as riders can reliably plan their schedules around the ride’s arrival and journey duration. For drivers, improved ETAs mean they can manage multiple rides more confidently, optimizing their schedules. Overall, accurate ETAs significantly enhance the transparency and usability of ridesharing platforms, increasing user satisfaction and operational effectiveness.
9. Vehicle Utilization Optimization
Routing and scheduling solutions maximize vehicle occupancy and reduce empty miles, boosting the efficiency and profitability of fleets.
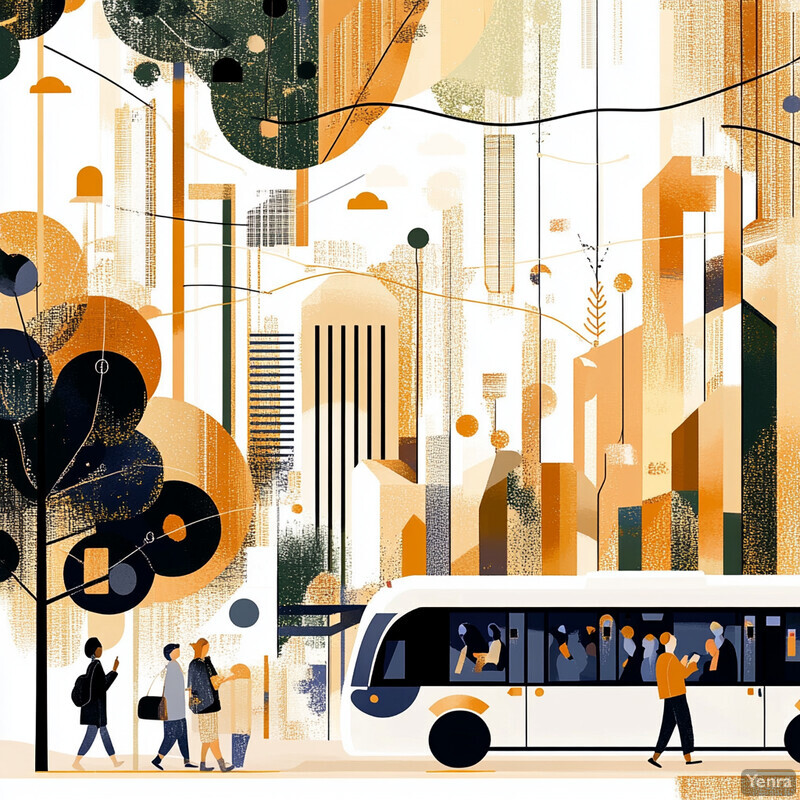
Maximizing vehicle utilization involves assigning trips that minimize empty seats and unproductive miles driven between pickups. AI optimization ensures that cars are kept as close to full capacity as possible, without compromising on convenience or trip quality. By reducing the frequency of empty car movements, operational costs drop, while drivers achieve more consistent earnings. Additionally, improved utilization cuts down on needless travel, reducing environmental impact. Over time, these optimization models become increasingly adept at fine-tuning how vehicles are deployed, balancing efficiency with user experience for a robust, sustainable operation.
10. Vehicle-to-Vehicle Coordination
AI-powered communication protocols between ridesharing vehicles help them avoid congestion clusters, coordinate spacing, and optimize placement within their operational regions.
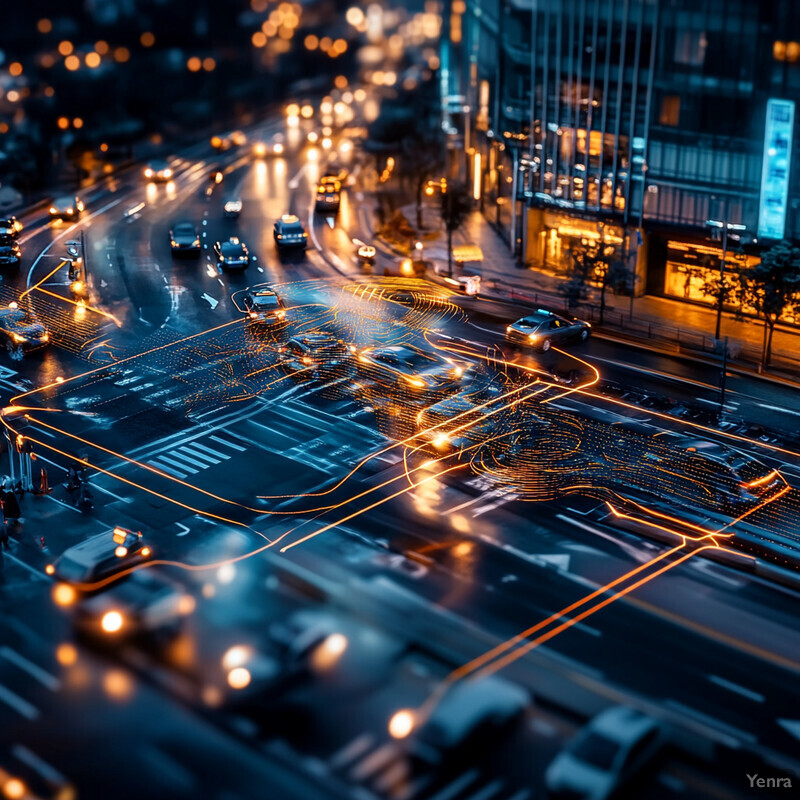
In a future where multiple ridesharing vehicles operate concurrently in a given region, AI can facilitate direct communication and coordination among them. By sharing information about their routes, passenger counts, and traffic conditions, vehicles can avoid clustering in the same areas and reduce congestion. This coordination ensures more balanced coverage, shorter wait times for riders, and fewer wasted trips for drivers. As these systems evolve, they can dynamically adjust routes and vehicle positioning, resulting in a fleet that acts like a coordinated, intelligent network rather than a loose collection of individual drivers.
11. Fleet Composition Analysis
Machine learning models determine the optimal mix of different vehicle types—such as sedans, SUVs, or vans—based on regional demand patterns and rider preferences.
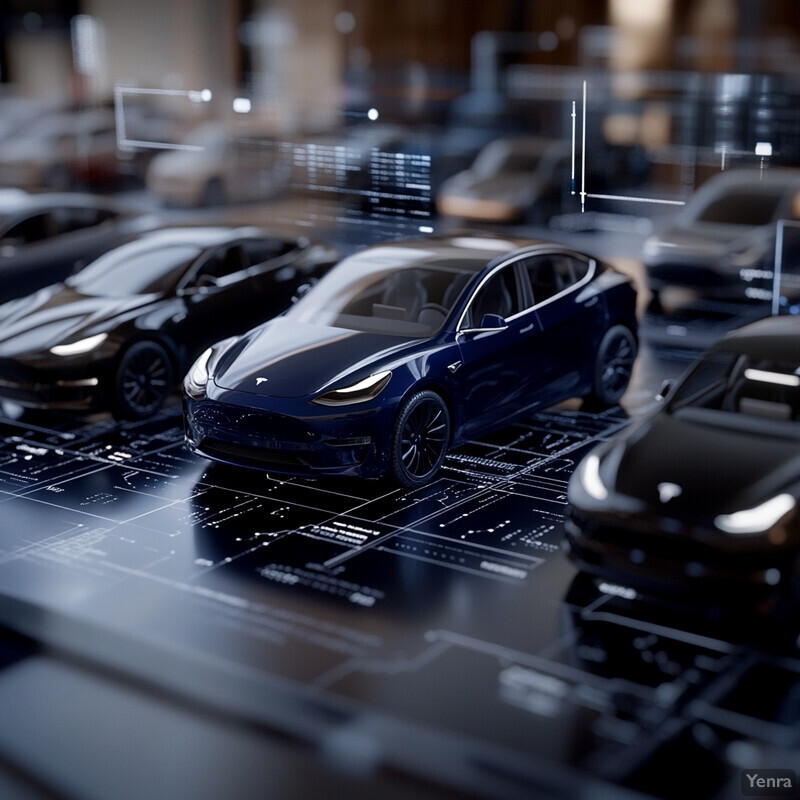
Determining the optimal mixture of vehicle types—standard cars, vans, luxury vehicles, or electric models—requires detailed analysis of rider demographics, travel patterns, and service areas. AI-driven insights guide decisions on what percentage of the fleet should be comprised of each vehicle category. By aligning fleet composition with demand (e.g., more vans for airport shuttles, more electrics in eco-conscious cities), companies can offer more tailored and cost-effective services. This strategic approach also impacts maintenance planning, branding, fuel expenditure, and environmental goals, ultimately contributing to a more responsive and appealing ridesharing network.
12. Sustainability and Emissions Reduction
AI-driven route planning and occupancy maximization help decrease total miles traveled and emissions, with some models prioritizing greener routes or vehicles.
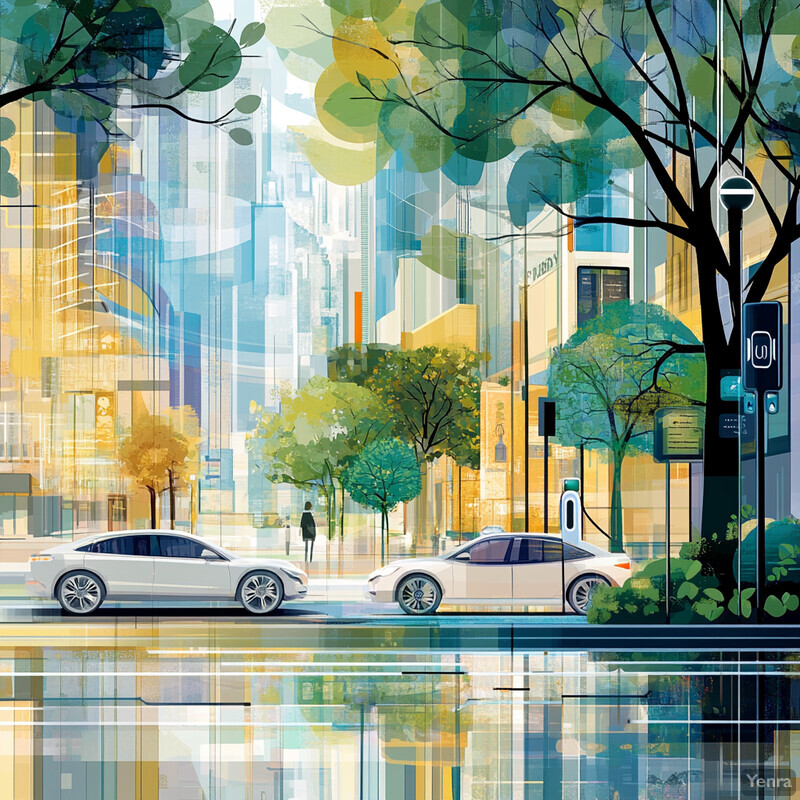
Sustainability efforts benefit significantly from AI’s capability to optimize routes, reduce idle time, and increase shared occupancy. As the system promotes pooling and more efficient travel paths, total vehicle miles traveled decline, leading to lower greenhouse gas emissions. AI can also weigh eco-friendly routing options, prioritize electric vehicles where possible, and nudge drivers and riders toward greener choices. Over time, these optimizations contribute to a cleaner urban environment, diminished traffic congestion, and better public health outcomes, which can enhance a ridesharing company’s reputation and align its services with broader sustainability goals.
13. Surge Management and Mitigation
AI systems anticipate surge conditions and strategically deploy vehicles, reducing the severity and frequency of surge pricing while still meeting demand spikes.
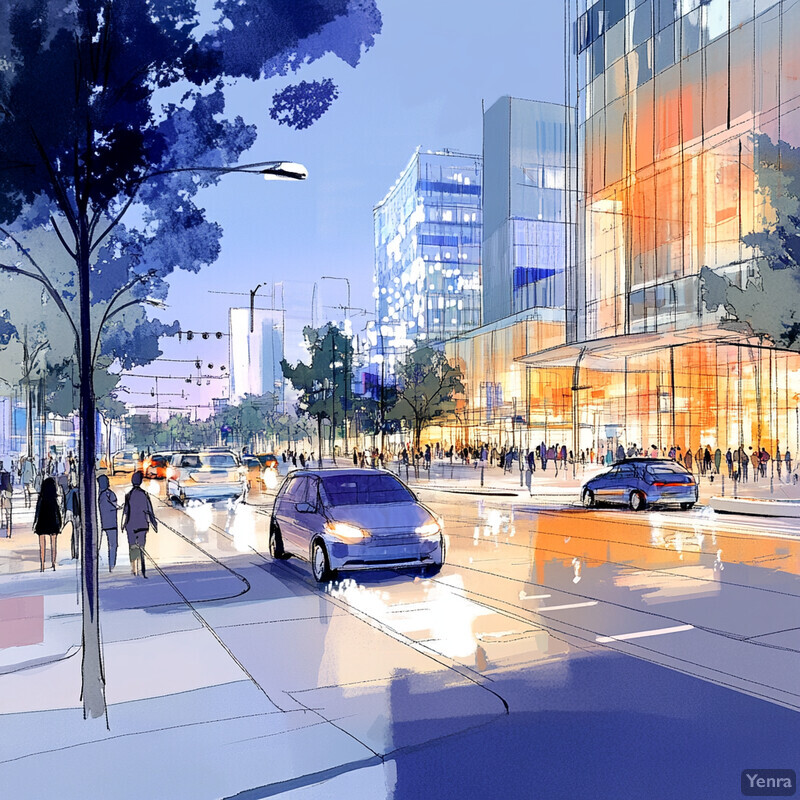
Instead of reacting after a sudden spike in demand occurs, AI can anticipate surge conditions by monitoring factors like weather changes, public transit disruptions, and major city events. By deploying additional drivers or incentivizing existing ones to move toward areas of expected high demand, the system can mitigate extreme price hikes and long wait times. Consequently, riders benefit from more stable pricing and quicker service, even during peak periods. Over time, effective surge management leads to higher user retention, a competitive edge, and a more reliable perception of the ridesharing service.
14. Maintenance and Downtime Prediction
Predictive maintenance models monitor vehicle performance data, identifying when cars need servicing to prevent breakdowns and reduce operational downtime.
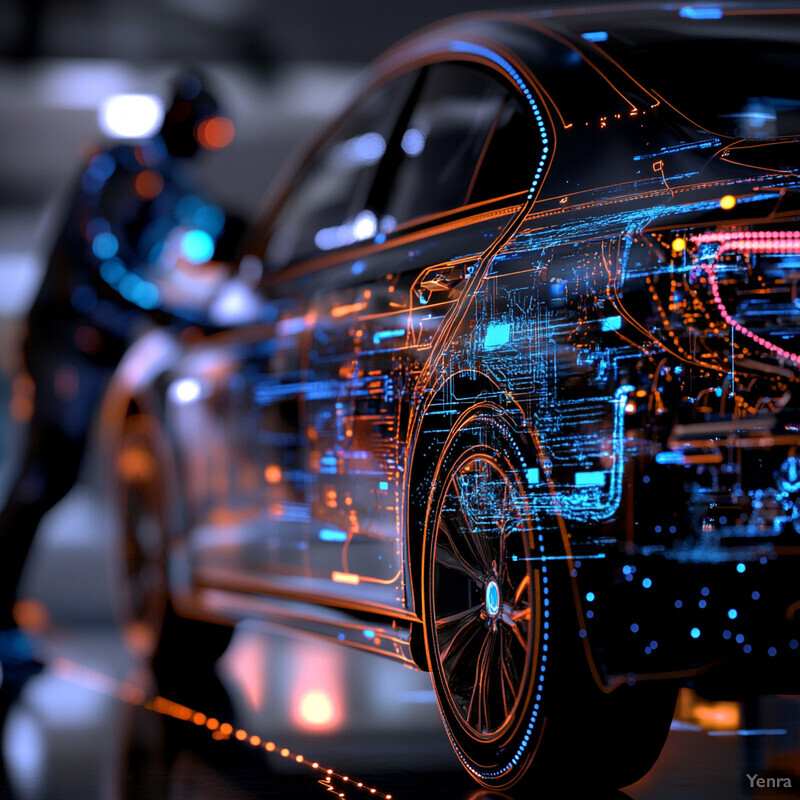
Predictive maintenance models monitor vehicle metrics such as mileage, fuel efficiency, engine health indicators, and brake wear. Using machine learning, these systems identify patterns that precede mechanical issues, allowing operators to schedule maintenance proactively. By addressing potential problems before they become severe, ridesharing services minimize unexpected breakdowns, reduce downtime, and ensure a consistent availability of well-maintained vehicles. The result is a smoother operation, lower long-term repair costs, and improved rider safety, as vehicles remain in optimal condition more consistently.
15. Personalized Travel Recommendations
Machine learning algorithms analyze a rider’s past trips and preferences to suggest the most convenient pooling options, pickup points, or specialized services.
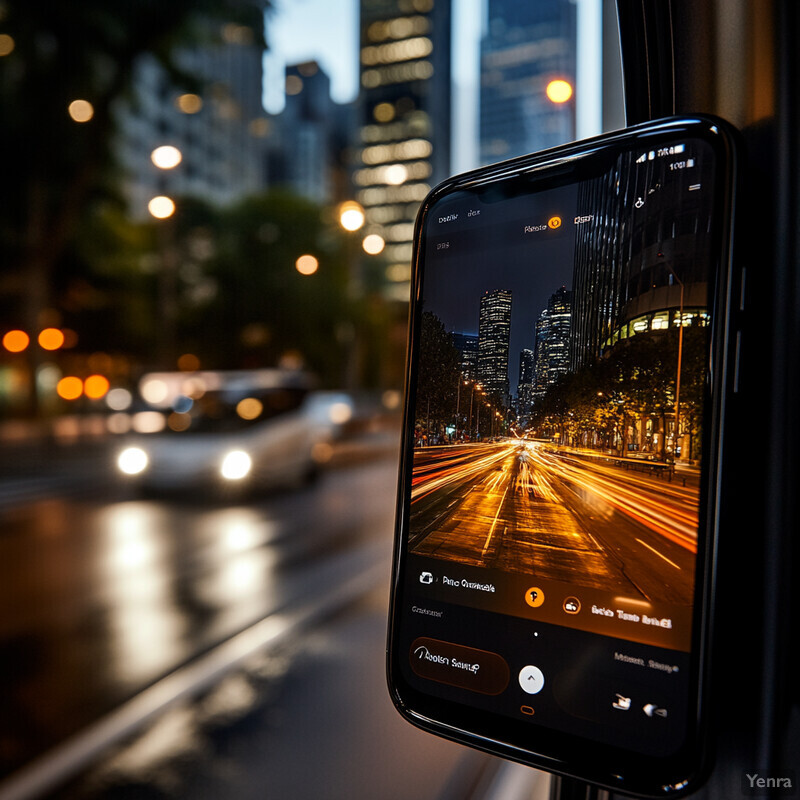
AI systems can analyze a rider’s past behavior, travel history, preferences for certain routes or vehicle types, and even the times of day they typically travel. Using this data, platforms can offer personalized suggestions—such as recommending a pickup point that reduces wait time, suggesting a cost-effective rideshare option, or highlighting special amenities. Personalized recommendations make the experience feel tailored to each rider, increasing user loyalty and satisfaction. By continuously learning from individual behaviors, the platform can refine its suggestions, ultimately creating a more intuitive and user-centric service.
16. Driver Coaching and Performance Feedback
AI-based feedback systems assess driving patterns, fuel efficiency, and passenger satisfaction, providing personalized tips to drivers to enhance performance and safety.
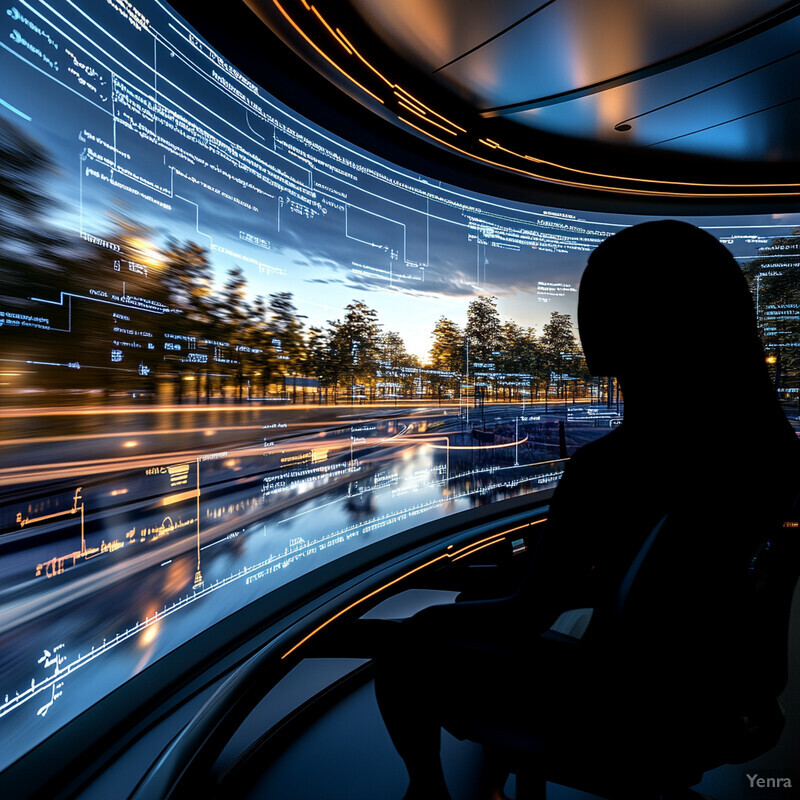
AI-based performance analytics provide drivers with detailed feedback on their driving habits, safety metrics, customer satisfaction scores, and operational efficiency. The system may highlight excessive speeding, harsh braking, poor route choices, or inefficient idling. Through regular and constructive feedback, drivers gain insights into how they can improve their overall service quality, fuel consumption, and customer experience. Over time, consistent coaching leads to a more professional driver community, which enhances the brand’s reputation, reduces accidents, and fosters trust and loyalty among riders.
17. Enhanced Safety and Security Measures
AI algorithms detect unusual driving patterns, erratic behavior, or route deviations in real time, enabling quick responses to potential safety risks.
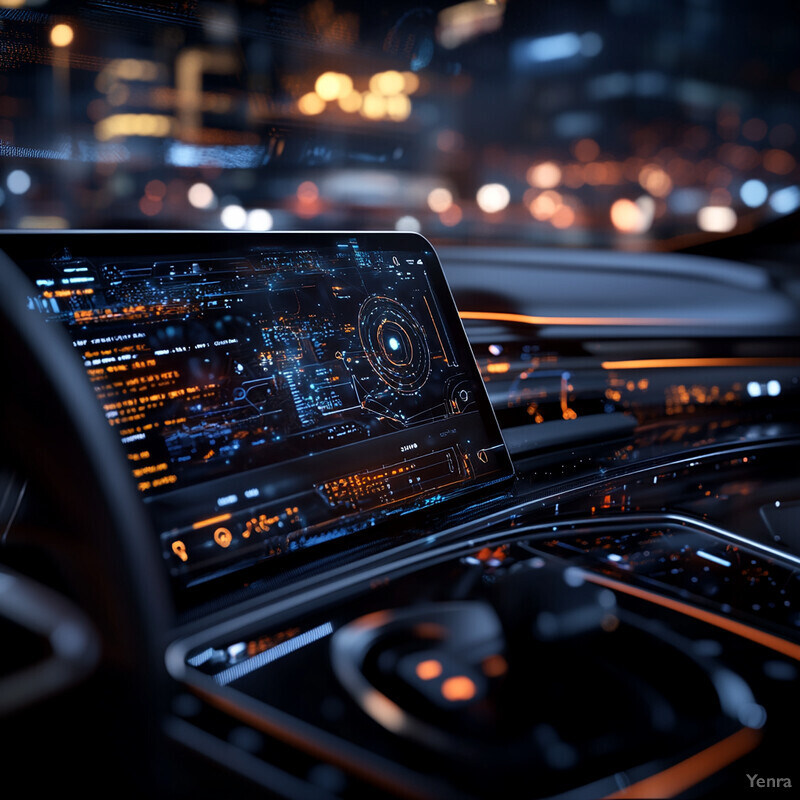
AI-driven safety protocols monitor driving patterns, detecting sudden deviations in route, erratic speed changes, or unexpected stops that might indicate a hazard. Additionally, computer vision can analyze onboard camera feeds to identify suspicious behavior or potential threats. Should something unusual occur, the system can alert operators in real-time, prompt additional verification steps, or even directly contact emergency services if needed. This proactive approach to security not only prevents harm but also instills confidence in riders, who can trust that the platform prioritizes their safety.
18. Fraud Detection and Prevention
Smart fraud detection systems identify suspicious patterns—like fictitious bookings or GPS spoofing—helping ensure trust and reliability within ridesharing ecosystems.
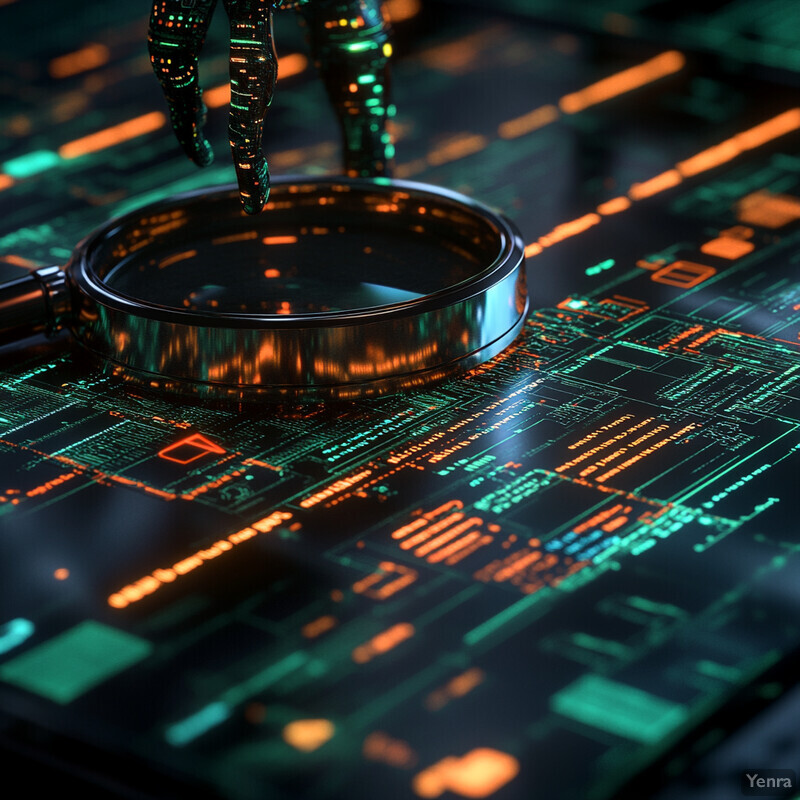
Ridesharing platforms face challenges such as fake driver profiles, fictitious bookings, or fraudulent payment methods. AI-based fraud detection systems learn to recognize anomalies in behavior—such as unusual travel patterns, duplicate accounts, or inconsistent payment information. When suspicious activity is flagged, the platform can take immediate action, whether by temporarily suspending an account, requesting additional verification, or involving law enforcement. As these models improve, they maintain platform integrity, protect driver and rider interests, and preserve the credibility of the ridesharing ecosystem.
19. Integrating Public Transit Data
By combining ridesharing data with public transportation schedules, AI can offer seamless multimodal trip planning, reducing wait times and encouraging carpooling.
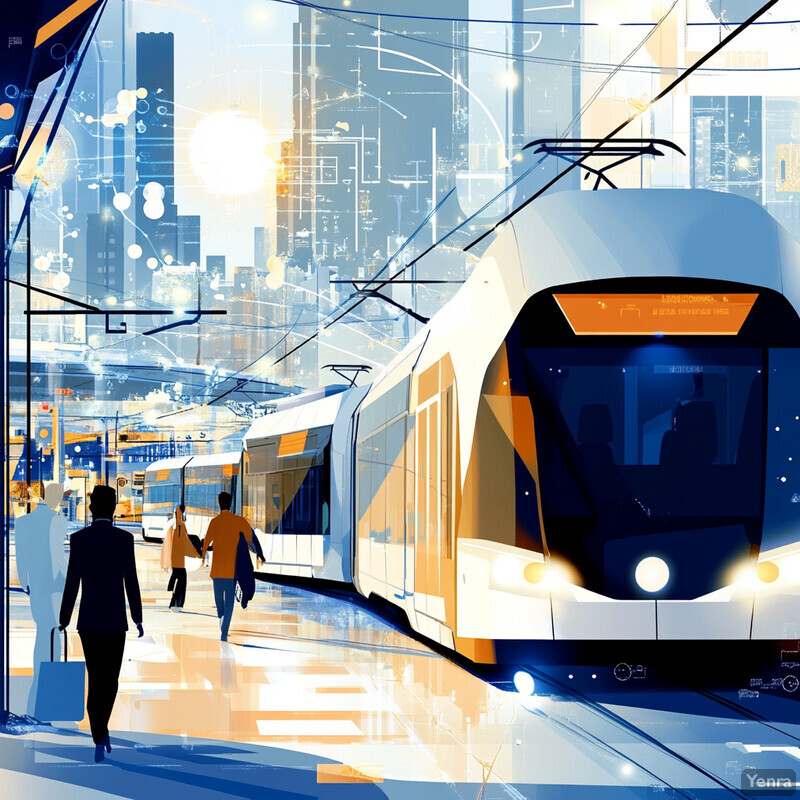
By incorporating schedules and real-time data from buses, trains, and other public transport, AI can present riders with multimodal trip options that combine ridesharing with public transit. Such integration helps passengers plan complex journeys more efficiently, often resulting in lower costs and reduced total travel time. AI can optimize these combinations, ensuring that a rideshare vehicle arrives just before a train departs or recommending a drop-off point near a bus stop. Encouraging riders to use public transport for longer stretches reduces traffic congestion, lowers emissions, and creates a seamless, citywide mobility network.
20. Simulation and Scenario Testing
Before implementing new policies or pricing structures, AI simulates various scenarios to predict outcomes, allowing data-driven decisions that optimize efficiency and rider satisfaction.
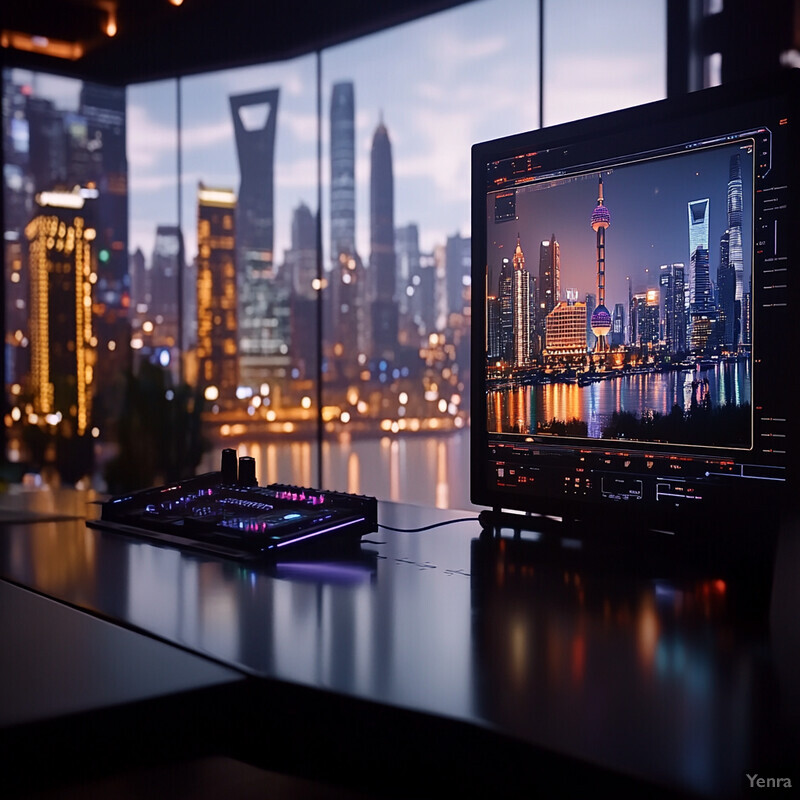
Before implementing a new pricing model, introducing a different fleet composition, or expanding into a new neighborhood, ridesharing companies can use AI simulations to predict the outcomes of these changes. These simulations incorporate historical data, demographic information, and potential future variables to run “what-if” scenarios. By analyzing a range of possible outcomes, decision-makers can choose strategies that are most likely to yield positive results. This reduces the risk of costly missteps and ensures that adjustments—be it in pricing, routing, or vehicle allocation—are grounded in evidence-based predictions rather than guesswork.